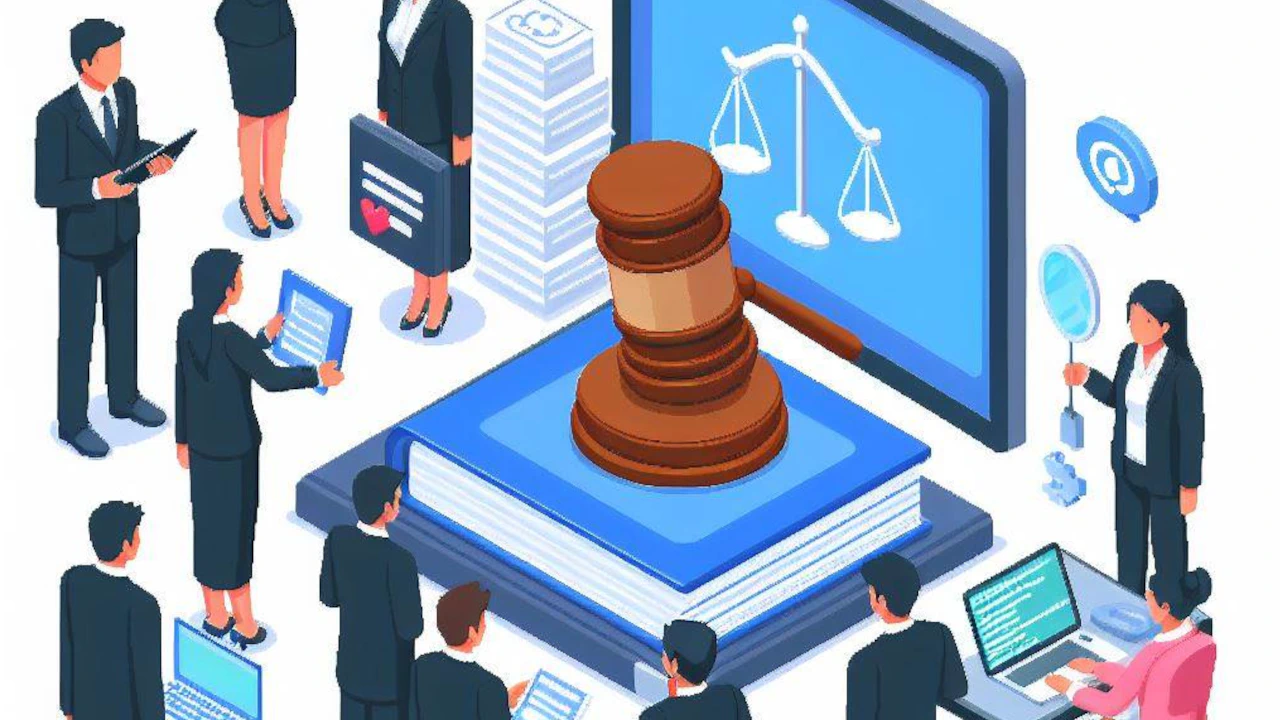
- By Justin Riddiough
- March 7, 2024
Open-source licenses are the foundation for collaboration and innovation in AI. They dictate how AI models, datasets, and code can be used, modified, and shared. Here’s a breakdown to help you navigate this crucial aspect of AI development:
The Open Source Initiative (OSI) is defining a comprehensive framework for open-source AI Deep Dive . This framework considers all aspects of an AI model, from training data to code, to guide the creation of appropriate legal licenses.
The Building Blocks of AI Models:
Each component of an AI model plays a crucial role in its functionality, and their licensing considerations can vary significantly. Here’s a breakdown:
-
Datasets (read about open datasets)
- Licensing Considerations: Datasets can be subject to various licenses, including copyright for curated data, creative commons for publicly available images or text, or specific database licenses.
- Open-Source Options: Look for datasets released under licenses like CC0 (public domain) or permissive licenses allowing reuse and modification for your AI project.
-
Training Code
- Licensing Considerations: The code used to train the model typically follows software licenses like MIT, Apache, or GPL. These licenses dictate how you can use, modify, and distribute the code itself.
- Open-Source Options: Choose code released under open-source licenses that align with your project’s needs. For instance, MIT grants flexibility, while GPL might require sharing your modifications if you distribute the trained model.
-
Trained Weights (read about open weights)
- Licensing Considerations: The legal status of trained weights can be less clear-cut compared to code. Some licenses might explicitly include or exclude weights, while others remain silent.
- Open-Source Options: Ideally, open-source projects provide access to both the training code and the trained weights. This allows full transparency and replicability of the model’s performance.
-
Deployment Code
- Licensing Considerations: Similar to training code, deployment code usually follows software licenses that dictate its use, modification, and distribution.
- Open-Source Options: Ensure the deployment code license aligns with how you intend to use the model. For commercial applications, licenses like Apache might be more suitable than restrictive licenses.
Common Open-Source Licenses:
- MIT License: A permissive license allowing free use, modification, and distribution of the AI model or code, with attribution to the original creators.
- GNU General Public License (GPL): Promotes open collaboration. If you modify and distribute an AI model under GPL, your modifications must also be open-source.
- Apache License: Offers a balance between open access and control. You can use the model in commercial products, but contributions back to the community are encouraged.
Finding Truly Open Projects:
Open-source doesn’t always mean completely unrestricted access. Here’s what to watch for:
- Data and Weights Availability: A truly open-source project provides access to both the training data and the trained model weights. Limited access might indicate a restricted project.
- Commercial Use Licenses: Some projects may require special licenses for commercial use of the AI outputs. Ensure these terms are compatible with your intended use.
- Custom Licenses: Be cautious of custom licenses claiming to be open source but lacking key elements of open access. Scrutinize project details to ensure genuine openness.
For a deeper dive into open models, open weights, and open data, along with a labeling system for these components, check out the AI Models website: labels .
Further Reading