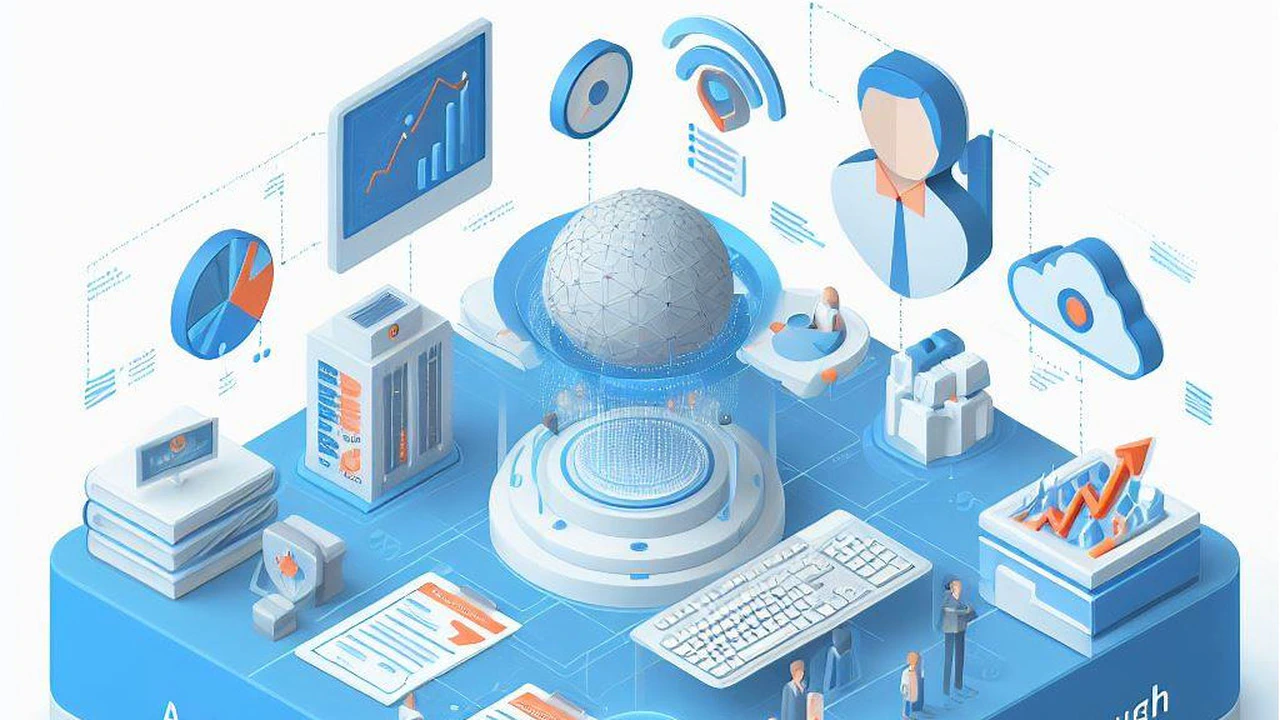
- By Justin Riddiough
- December 10, 2023
Implementing AI governance processes is critical for ensuring accountability, preserving human agency, and promoting inclusive growth. For those tasked with this responsibility, effective management and oversight are key. Let’s explore practical strategies and best practices to achieve these goals.
Accountability: Proper Management Oversight
Accountability is foundational to responsible AI use. It involves establishing proper management oversight throughout the AI system development lifecycle. Here’s how to ensure accountability:
- Clear Roles and Responsibilities: Define and communicate clear roles and responsibilities for all stakeholders involved in AI system development.
Real-world Application: In a manufacturing AI system, assigning responsibilities ensures that both data scientists and domain experts understand their roles in enhancing product quality.
- Regular Audits and Reviews: Conduct regular audits and reviews to ensure adherence to ethical standards and regulatory requirements.
Best Practice: Integrate audit processes seamlessly into the development pipeline for continuous accountability.
Human Agency and Oversight: Preserving Decision-making Abilities
Preserving human agency in decision-making is essential. AI systems should be designed to complement human capabilities, not diminish them. Follow these best practices:
- Explainable AI Models: Prioritize the development of explainable AI models that allow humans to understand and interpret the system’s decisions.
Practical Example: In a financial AI for risk assessment, an explainable model helps financial analysts validate and trust the system’s risk predictions.
- Human-in-the-Loop Systems: Implement systems where human intervention is possible, especially in critical decision-making scenarios.
Real-world Application: In a healthcare AI diagnosing diseases, a human-in-the-loop system allows medical professionals to provide insights and verify AI-generated diagnoses.
Inclusive Growth, Societal & Environmental Well-being: Beneficial Outcomes
AI governance should extend beyond individual systems to contribute to inclusive growth and societal and environmental well-being. Consider these practices:
- Ethical Use Considerations: Assess the potential impact of AI systems on society and the environment, emphasizing ethical considerations.
Best Practice: Integrate ethical impact assessments into the AI development process to identify and address potential harms.
- Monitoring Societal Impact: Continuously monitor the societal impact of AI systems post-deployment and iterate based on feedback.
Real-world Application: A city implementing AI for traffic management continuously monitors the impact on local communities and adjusts algorithms to minimize congestion and environmental impact.
Nurturing Responsible AI Governance
With AI governance, effective management and oversight are pivotal. By prioritizing accountability, preserving human agency, and ensuring beneficial outcomes for society and the environment, organizations can nurture responsible AI governance.
Remember, responsible AI governance is an evolving journey. Regular assessments, stakeholder collaboration, and staying attuned to ethical considerations are essential for maintaining trust and fostering positive impacts.