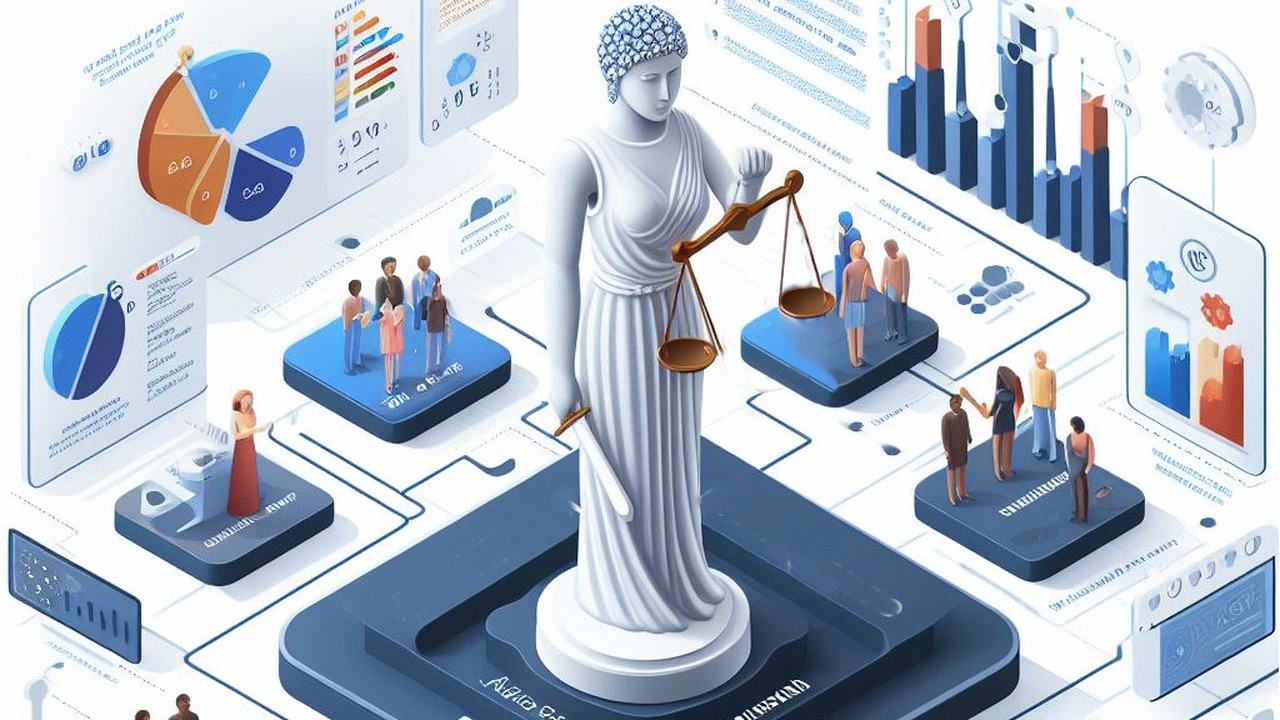
- By Justin Riddiough
- December 10, 2023
In the landscape of AI governance, ensuring fairness and preventing unintended discrimination are paramount. For those leading the implementation charge, the focus is on practices that eliminate bias, promote fairness, and establish robust data governance throughout the data lifecycle. Let’s dive into practical strategies and best practices.
Fairness: Eliminating Unintended Bias
Fairness is the bedrock of ethical AI. It involves ensuring that AI systems make consistent decisions regardless of changes in attributes and that the data used for training is representative. Here’s a roadmap for achieving fairness:
- Consistent Decision-making: Regularly test the AI system to ensure that changing an attribute does not lead to disparate outcomes.
Real-world Application: In a hiring AI, consistent decision-making ensures that candidates with the same qualifications are treated equally regardless of demographic attributes.
- Representative Training Data: Use diverse and representative datasets when training AI models to avoid biased decision-making.
Best Practice: Continuously audit and update training data to reflect evolving demographics and scenarios.
Data Governance: Embedding Fairness in the Data Lifecycle
Good data governance practices are instrumental in embedding fairness throughout the data lifecycle. Follow these best practices:
- Transparent Data Collection: Clearly communicate the purpose and methods of data collection to ensure informed consent.
Practical Example: In a healthcare AI system, transparent data collection practices build trust among patients by explaining how their data contributes to improved diagnostics.
- Ethical Data Handling: Implement ethical data handling practices, such as anonymization and aggregation, to protect individual privacy.
Compliance Reminder: Adhere to data protection regulations, ensuring that personal information is handled responsibly.
- Regular Audits: Conduct regular audits to identify and rectify any biases that may have crept into the dataset over time.
Real-world Application: In a financial AI system, regular audits help identify and rectify biases that may emerge due to shifts in economic trends.
Paving the Way to Ethical and Fair AI Governance
In the dynamic landscape of AI governance, prioritizing fairness and eliminating unintended discrimination is both an ethical imperative and a compliance necessity. By focusing on consistent decision-making, representative training data, and robust data governance, organizations can pave the way to ethical and fair AI governance.
Remember, fairness is an ongoing commitment. Regular assessments, transparent practices, and staying attuned to evolving ethical standards are essential for maintaining trust in AI systems.