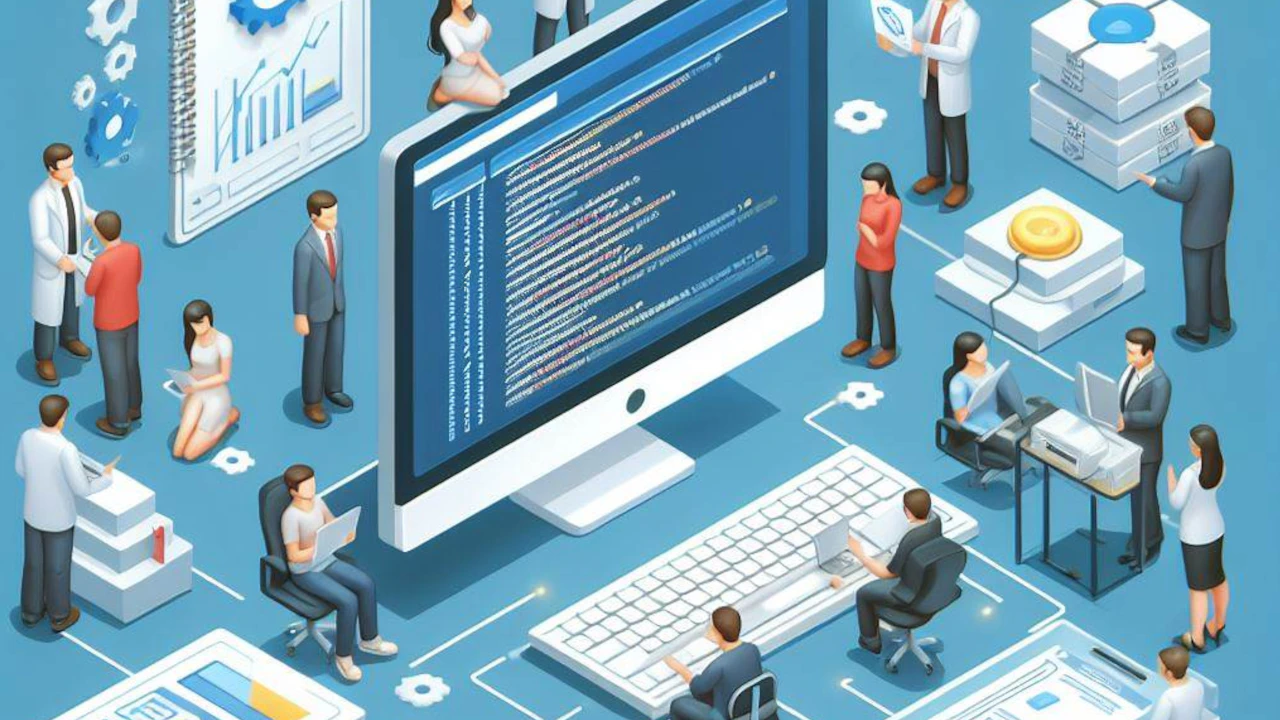
- By Justin Riddiough
- December 10, 2023
Version Control Systems
Version control is the backbone of collaborative software development, and in the realm of open-source AI, it plays a crucial role in managing code changes, tracking progress, and enabling seamless collaboration. One of the most widely used version control systems is Git.
Utilizing Systems Like Git for Code Versioning:
-
- Git allows teams to track changes in their source code efficiently. Learn the basics of Git, including commands for creating repositories, making commits, branching, and merging.
-
Collaborative Workflows:
- Explore collaborative workflows with Git, such as feature branching and pull requests. These practices streamline the integration of contributions from multiple developers.
-
Code Reviews:
- Leverage Git’s pull request or merge request features for code reviews. This ensures that changes are thoroughly reviewed before being merged into the main codebase.
-
Continuous Integration:
- Integrate Git with continuous integration (CI) tools like Jenkins or GitHub Actions. CI helps automate testing and ensures that changes don’t break existing functionality.
Reproducibility
Reproducibility is a cornerstone of reliable AI research. It ensures that experiments and results can be replicated by others, promoting transparency and reliability in the field. According to the OECD , reproducibility involves:
“The ability to re-use the same data or analysis to confirm the robustness of findings and results.”
Ensuring Reproducibility of AI Results:
-
Environment Setup:
- Document the software and hardware environment used for AI experiments. This includes specifying the versions of libraries, dependencies, and hardware configurations.
-
Containerization:
- Consider using containerization tools like Docker. Containers encapsulate the entire environment, making it easier for others to reproduce your experiments without compatibility issues.
-
Versioning Data:
- Apply version control principles to datasets. Use tools like DVC (Data Version Control) to track changes in datasets and ensure that data used in experiments is versioned and accessible.
-
Code Documentation:
- Document your code comprehensively. Explain the rationale behind design choices, algorithms used, and parameters selected. This documentation aids in understanding and reproducing the results.
-
Workflow Automation:
- Automate your workflow using tools like Make or workflow orchestration platforms. This ensures that the entire process, from data preprocessing to model training, is reproducible with a single command.
Note: By adopting robust version control practices and prioritizing reproducibility, open-source AI projects contribute to the reliability and credibility of research outcomes. These practices enable collaboration, facilitate peer review, and accelerate advancements in the field.