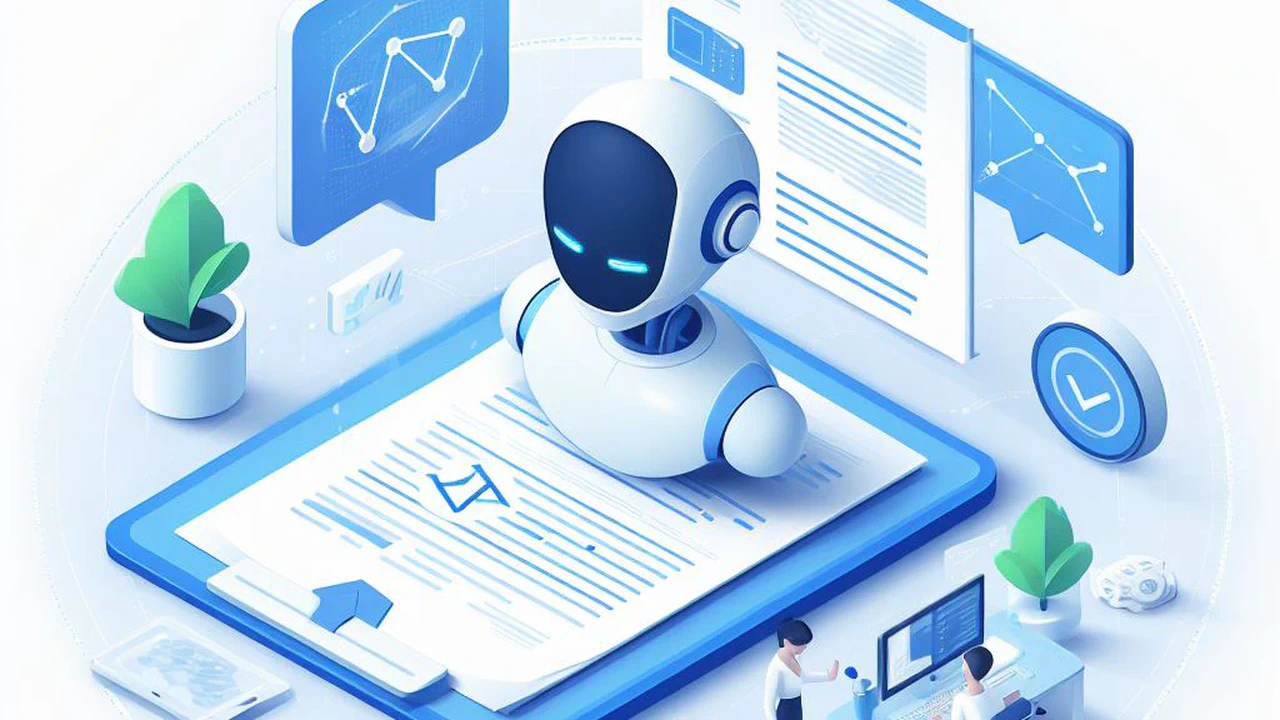
Papers is Alpha. This content is part of an effort to make research more accessible, and (most likely) has lost some details from the original. You can find the original paper here.
Introduction
Artificial intelligence (AI) is already in use across many areas of social and economic life, and new opportunities for AI to contribute to social good (AI4SG) have also been proposed and developed. For example, efforts like Microsoft’s AI for Earth program highlight the potential of AI to address the United Nation’s Sustainable Development Goals (SDGs). However, many challenges face the practical implementation of AI for social good efforts. Similarly, in the field of fairness, accountability, and transparency of AI, decades of research has only recently begun to be more thoroughly incorporated into practical settings, and many questions remain. In this paper we review challenges in translating principles into practices and propose recommendations towards closing this gap.
After introducing prior work on responsible AI principles and concerns about the practical application of these principles in Section 1, Section 2 proposes five explanations for the principles-to-practices gap. We discuss the complexity of AI’s impacts, confusion about the distribution of accountability, a social technical disciplinary divide, identifying and using tools, and organizational processes and norms as key issues in this gap.
In light of these concerns, Section 3 proposes the criteria of a framework that could help organizations turn responsible AI principles into practices. We propose that impact assessment is a promising approach towards meeting these criteria, as it has the potential to be sufficiently broad, operationalizable, flexible, iterative, guided, and participatory. As an exemplar, we focus on the new Institute of Electrical and Electronics Engineering’s (IEEE) 7010-2020 Recommended Practice for Assessing the Impact of Autonomous and Intelligent Systems on Human Well-being (henceforth IEEE 7010). IEEE 7010 is a standard that assesses the well-being implications of AI and employs a well-being impact assessment to do so. Finally, to help practitioners apply these recommendations. Section 4 applies a well-being impact assessment framework to a case study. The case study reviews challenges with AI’s use in ecosystem restoration and afforestation – an important aspect related to several SDGs – and demonstrates how an impact assessment framework may help to close the principles-to-practices gap in this case.
Principles
As of 2019, more than 20 firms[For example, Google, Microsoft, IBM, Sage, Workday, Unity Technologies, and Salesforce] have produced frameworks, principles, guidelines, and policies related to the responsible development and use of artificial intelligence (AI).[We opt for the phrase responsible AI' but this topic can also be termed as the
ethical AI,’ ’trustworthy AI’ or similar.] These documents are meant to address many of the social and ethical issues that surround AI, ranging from labor displacementand algorithmic biasto privacy, an increasingly important issue in the context of the COVID-19 virus. These governance documents typically address a set of social and ethical concerns, propose principles in response, and in some cases offer concrete reforms or internal governance strategies.
Research on the various AI documents produced by firms along with government actors and non-governmental associations has identified clear consensus in organizations’ ethical priorities. The social and ethical concerns highlighted most often surround general concern for public, customer, and employee welfare; algorithmic bias and fairness; transparency and explainability; trust in AI; and reliability and safety of AI products. While this scholarship often focuses on identifying consensus across organizations, it has also examined how companies define their responsibilitiesand whether there are issues neglected across documents.
Importantly, a key focus of the documents is on presenting a set of high-level principles for responsible AI. For example, Google’s AI principles include “Be socially beneficial,” “Avoid creating or reinforcing AI bias,” “Be built and tested for safety,” and “Be accountable to people,” among other principles. OpenAI discusses its focus on “Broadly Distributed Benefits,” “Long-Term Safety,” “Technical Leadership,” and its “Cooperative Orientation”. However, these and other high-level responsible AI principles can often be vague, host a multitude of possible interpretations, and may be difficult to translate into everyday practices.
Practices
Many scholars have raised concerns that companies less often provide detailed prescriptions of policies or practices meant to ensure that these principles are adhered to. In some cases, companies have established relatively clear strategies. Proposed practices include training, hiring, algorithm development frameworks and tools, and governance strategies. For example, Vodafone’s AI Framework provides some detail on specific actions it will take, such as adhering to its Code of Conduct and privacy commitments. SAP proposes as part of its Guiding Principles an AI Ethics Steering Committee and an AI Ethics Advisory Panel. IBM’s Everyday Ethics for AI provides a set of recommended actions and questions for its employees to address key concerns.
On the other hand, some principles are not accompanied by clear expressions of changes to practice. For example, documents from Tieto, Futurice, and Salesforce focus on abstract principles and commitments. Futurice proposes to “avoid creating or reinforcing bias,” while Salesforce claims “we test models with diverse data sets, seek to understand their impact, and build inclusive teams” and Tieto states it is “committed to harness AI for good, for the planet and humankind”. These and other generic principles like “Be socially beneficial“ beg the question of how exactly the companies are carrying out their commitments.
In the best case, companies may still be in the process of working out the details or may have communicated their intended strategies in other venues, for example, by publishing tools for responsible practice. Nevertheless, remaining at the “mission statement” level and the lack of practical detail are worrisome. We believe that the question of translating high-level principles into effective and responsible practices is a critical priority in the near-term future for AI. Closing the principles-to-practices gap is worthy of attention by companies developing AI, by those who might procure and deploy AI systems, and by other stakeholders and the public more broadly.
Principles without Practices?
Despite firms’ efforts towards establishing some design and development principles for AI systems, several breaches of law and the public trust have been reported in the last few years. Companies have come under significant scrutiny, in some cases facing significant negative media attention, along with customer criticism and employee petitions, walkouts, and resignations.
The question then is why principles have seemingly not been translated into effective practices? In fact, the process of translation is neither obvious nor automatic as clear. According to Mittelstadt (2019) “norms and requirements can rarely be logically deduced… without accounting for specific elements of the technology, application, context of use, or relevant local norms”. Barring practical guidance and absent “empirically proven methods… in real-world development contexts,”claims of responsible AI may amount to no more just that — claims.
As a result, some criticisms more deeply impugn the motives of firms. Greene et al.argue that companies attempt to shift responsibility onto designers and experts in order to minimize scrutiny of business decisions. Similarly, Hagendorffargues that companies are often driven by an economic logic, and that “engineers and developers are neither systematically educated about ethical issues, nor are they empowered, for example by organizational structures, to raise ethical concerns.” On this account, companies may be strategically promoting their principles to ameliorate customer trust and reputational concerns. In this way, they can appear actively engaged regarding AI’s ethical risks in the public eye, but while framing issues so as to minimize genuine accountability.
While some of these deeper criticisms may be true in part or for some organizations, we think a more multifaceted and charitable interpretationis both appropriate and likely to be beneficial toward seeking positive change. Organizations are best understood not as monolithic single actors, but as multiple coordinating and competing coalitions of individuals. Individuals within a single organization may have multiple or competing preferences and roles. Organizational motives should therefore be considered a complex composition of genuine ethical concern, economic logic, signaling and framing strategies, and promotion of both internal and external changes.
Researchers who have noticed the principles-to-practices gap have begun proposing strategies, often aimed at companies. These proposals include changes to software mechanisms (such as audit trails), hardware mechanisms (such as secure hardware enclaves), and institutional mechanisms (such as red team exercises). This work highlights that it is not only technical practices that must adapt, but also organizational practices.
Among the most comprehensive work assessing the principles-to-practices gap is the review by Morley et al. (2019), which systematically explores existing responsible AI tools and methodologies mapped against six components of the AI development lifecycle: 1) business and use-case development, 2) design phase, 3) training and test data procurement, 4) building, 5) testing, 6) deployment, and 7) monitoring. They identify 106 such tools and methodologies. Some such methods are relatively narrower in scope, such as those surrounding explainable AI, bias, or procurement (e.g., the AI-RFX Procurement Framework).
Other methodologies adopt a broader scope of focus, including impact assessments like the ISO 26000 Framework for Social Responsibilityand IEEE 7010. Relevant methods and approaches for responsible AI also come from outside of the AI domain and include privacy-by-design, value-sensitive design, the Responsible Research and Innovation (RRI) approach, and numerous others. In fact, the plethora of possible tools is itself a challenge which we discuss more in Section 2.
Explaining the Principles-to-Practices Gap
In short, despite the urgent attention to responsible AI in recent years, there are already many existing frameworks and a growing set of new methods aimed at addressing core ethical issues. Why then does the issue of translating principles to practices seem intractable? We offer a few candidate explanations that are neither exhaustive nor mutually exclusive.
The Complexity of AI’s Impacts
AI’s impacts on human well-being – positive or negative – are more complex than is sometimes assumed. Site-based research has identified that engineers are often focused on single products and the physical harm they may cause rather than broader kinds of harms, such as social, emotional, or economic harms. Even as conversations surrounding responsible AI increase, most work centers around a relatively small subset of issues, most often biasand transparencyin particular AI models. This approach involves exposing and then attempting to mitigate bias in algorithms as well as trying to improve interpretability or explainability given the black-boxed nature of certain AI models which can make decision-making processes opaque. Other commonly-emphasized issues include privacy, reliability, and safety.
However, these prominent issues most familiar to engineers still constitute only a subset of social and ethical risks and impacts related to AI. Indeed, AI can be understood to impact a wide variety of aspects of human well-being, such as human rights, inequality, human-human relationships, social and political cohesion, psychological health, and more. AI can also impact natural ecosystems and animal life.[Impacts on the environment and non-human animals may be intrinsically important, as well as instrumentally important to human well-being.] Moreover, many of these harms do not arise in a straightforward way from a single AI product, but from many AI systems influencing human social and economic life together and over time.
AI is not the only technology with complex implications on human well-being. Yet its rapid rise is leading to calls for urgency, and some aspects of AI surface a unique combination of ethical concerns. For example, compared to other general-purpose technologies like electricity or the internet, AI is notable for its autonomy, its capacity to `learn,’ and its power in making accurate predictions, all while embedded in software and ambient systems and therefore invisible to many affected by it. As a result, AI systems are becoming increasingly ubiquitous, and can act in the aggregate to influence human and social well-being in subtle but pervasive ways.
For example, algorithms on social media designed to steer consumers to entertaining video clips have also led to so-called filter bubbles that may foster political polarization, misinformation and propaganda, targeting of minority groups, and election interference. AI as instantiated in autonomous vehicles has potentially massive implications for physical infrastructure, energy and environment, traffic fatalities, work productivity, urban design, and unemployment. In short, addressing AI principles in full seriousness requires an expansive scope of attention to the full set of issues influencing human well-being. This requires looking well beyond a narrow set of topics such as bias, transparency, privacy, or safety and treating them as independent issues. Instead, the full range of topics and their complex interdependencies needs to be understood. However, such a task can be enormously difficult.
The Many Hands Problem
It is clear that responsibly designing and applying AI is therefore both a technical challenge and a social one (implicating social, economic, and policy questions). For example, creating a facial recognition system for policing that minimizes racial bias (by some technical measure) is inseparable from questions on the legitimacy of the use of that system in a particular social and policy setting. However the question of distributing accountability for addressing these issues remains open and contested. Engineers and computer scientists may see their responsibility as focused on the quality and safety of a particular product rather than on larger scale social issues, and may be unaware of the wider set of implications. Business managers and companies may see their responsibility as fiduciary, in producing high-quality products and revenue. This potentially creates holes in responsibility for addressing key well-being impacts of AI.
In addition to uncertainty regarding one’s scope of professional accountability, engineers and computer scientists who focus on design of systems may have limited influence within their organizations. They may expect business managers, liability officers, or corporate social responsibility staff to assess broader social and ethical issues. Social scientists and ethicists tapped specifically for these issues may find themselves similarly handicapped, perhaps in an external advisory role without real say. The result is the `many hands’ problem, where responsibility for responsible AI is distributed and muddled. The many stakeholders involved in shaping AI need to be both functionally able and willing to resolve the accountability question with a concrete division of labor. If companies fail to resolve these challenges, they may continue to face public scrutiny as well as financial and legal risks and reputational harms. Moreover, they may harm their employees, consumers, or the public. Figuring out how to distribute responsibility for AI’s impacts on well-being is therefore as critical as it is difficult. It may involve challenging long-held assumptions and shifting norms.
The Disciplinary Divide
Another related challenge is the plurality of professional disciplines with roles to play in responsible AI. Discourse on responsible AI has been advanced not only by engineers and computer scientists, but also by sociologists, ethicists, historians and philosophers of technology, policy scholars, political decision-makers, journalists, members of the public, and more. Yet the composition of these diverse stakeholders directs attention to the likelihood that they may bring very different perspectives to the table. They may differ in their technical and ethical education, their framing of problems and solutions, their attitudes and values towards responsible AI, and their norms of communication.
Consider attempts to apply the principle of fairness in attempting to minimize bias. Arguably, a thoughtful AI engineer today might identify a normative principle like ‘fairness,’ specified in a corporate responsible AI policy, pick a plausible fairness metric to instantiate it (noting there are ineliminable trade-offs between different metrics), apply it, and communicate these decisions transparently. However, even these laudable efforts cannot begin to satisfy the extensive societal questions related to fairness, discrimination, and inequality that trouble many social scientists and ethicists.
More specifically, approaching social issues like bias and fairness too narrowly leads to what Selbst et al. (2018) call category or abstraction errors. For example, computer scientists and engineers developing AI systems can fail to consider how an AI system will be implemented in different social contexts, influence human behavior in those contexts, or lead to long-term ripple effects, all of which can threaten the assumptions on which the AI system is built. This is especially difficult as predicting a technology’s usage and impact is known by historians of science and technology to be difficult. More fundamentally, AI developers may err in even considering social concepts like fairness to be computationally definable and technically soluble.
Consider an algorithm designed to minimize racial bias that is used to inform a judge’s decision about criminal sentencing. An algorithm designed and trained on test data from one jurisdiction may translate poorly to another region. It may influence the judge’s decisions in unexpected ways, as a judge may overtrust or undertrust the algorithm, or even hold values contrary to those reflected in the algorithm. For example, the algorithm may favor predictive accuracy, while the judge favors leniency and second chances. The consequences for criminal justice outcomes when such a system is used in complex contexts is unclear, and may feed back in unexpected or problematic ways if an AI is trained on data the system has itself helps to generate. To reiterate, there are many questions about responsible AI that cannot be straightforwardly addressed with a narrow technical lens.
On the other hand, social scientists may bring a lens that is broader but faces an inverse problem to the problem faced by engineers. Frameworks for considering social and ethical consequences of AI more in line with the thinking of social scientists can be unhelpfully complex and vague, and therefore fail to translate into action. For example, ethicists recognize that concepts like justice are complex, while political scientists know that values surrounding justice are politically contested. Yet AI engineers must define some measure of justice to implement it.
In addressing issues like inequality, social scientists may propose large structural changes to economic and social systems, some of which are difficult to achieve (e.g., reforming the motives of corporations) and others possibly far-fetched (e.g., changing the structure of capitalism). These structural changes may be significantly outside of the scope of control of AI engineers. Also unhelpful are conceptions of AI based on sweeping, overly futuristic, or unrealistic generalizations. These abstractions can fail to provide the specificity needed to think clearly about addressing harms to human well-being. Again, while the intentions may be laudable, translating them to practice can be unfeasible or at best unclear. In the best case, it is difficult to resolve the awkwardness of attempting to apply technical fixes to fundamentally socio-technical problems. Something is lost in translation.
The Abundance of Tools
As we have seen, there are already many tools and methodologies for addressing responsible development and use of AI. While creating more and better such tools and methodologies is a worthy pursuit, in one sense there are already too many. Even those tools that do exist have arguably not been tested sufficiently to demonstrate which are most effective and in which contexts. An over-abundance problem makes it difficult for individuals to sort through and assess the utility of a given tool, or to weigh it against the many other available tools. People’s time, attention, and cognitive capacity is limited, leading to search and transaction cost problems. As a result, individuals and organizations may fail to take advantage of the useful tools and methodologies that are already out there.
In addition, many tools and methodologies are not supported by practical guidance. A published journal paper or open source code may explain basic functionality but not contain sufficient instructions to apply, customize, or troubleshoot tools and methodologies, especially in a variety of organizational contexts and use cases. This means that only tools that are well-documented, perhaps those created by well-resourced companies or universities and backed up by online communities, may be feasible to use. Individuals without high levels of expertise and specific training may have little luck even with these prominent tools.
Further, because of the disciplinary divide, methodologies developed in part or in whole by disciplines outside of engineering and computer science (such as responsible research and design ethics) may have a harder time gaining traction. If these extra-disciplinary ideas are not documented and translated for use in AI development settings, there may be little uptake. More work is needed to test tools empirically, to streamline access and guidance, and to help with sorting between tools and methods. Organizations may need an overarching framework to help integrate these lower-level tools and methodologies.
The Division of Labor
The last explanation for the principles-to-practices that we discuss is how organizations structure their job responsibilities and workflow related to AI. Again related to the disciplinary divide, a major concern is that the computer scientists and engineers more directly responsible for an AI system’s development may be functionally separated from other workers likely to be tasked with thinking about the system’s broader implications – such as higher-level business managers, the C-suite, and corporate social responsibility and compliance staff. For simplicity, we refer to these crassly as technical' and
non-technical’ teams.
For instance, several companies have proposed external AI ethics advisory or governance boards. External boards (and likely internal ones) may constitute functionally distinct units of the organization that interact only occasionally with primary AI system designers. The same functional separation may apply even when non-technical teams are internal to an organization. Non-technical employees may have limited ability to understand or modify an AI system’s design if interaction with technical teams happens at an arm’s distance. Staff without disciplinary expertise in engineering and computer science and even those with technical expertise but not involved in the system’s creation may not be able to imagine improvements to the system’s development or deployment. They may make underinformed or overly simplistic decisions, for example, prohibiting the use of an AI system that could be modified; or recommending the use of an AI system when they do not fully understand its risks. This functional separation therefore limits their ability to support responsible AI development that adequately considers the full range of impacts on human well-being.
On the other hand, engineers and computer scientists in technical teams may also not be privy to the deliberations of their non-technical counterparts if there is functional organizational separation. If technical employees are exempt from this dialogue, they will not be able to participate in how their colleagues weigh considerations of corporate responsibility, profit, policy, and social and ethical impacts. They may not learn how to incorporate these concepts and trade-offs into their design processes. Technical teams may also fail to imagine ways in which the system they are creating could be improved, or how other systems, tools, or methodologies could be applied to better safeguard and improve human well-being. In sum, functional separation of technical and non-technical experts in organizations limits the potential to communicate effectively, understand issues robustly, and respond to considerations of AI’s impacts on well-being.
Summarizing the Concerns
In this section, we have reviewed five sets of concerns that we believe help to explain why AI principles do not easily translate into concrete and effective practices: 1) that AI’s social and ethical implications for human well-being are broader, more complex, and more unpredictable than is often understood; 2) that accountability for ethical consequences is divided and muddled; 3) that the orientations of experts in different disciplines lead to emphases that are too narrow, too broad, and generally difficult for translation and interdisciplinary communication; 4) that existing methodologies and tools for responsible AI are hard to access, evaluate, and apply effectively; and 5) that organizational practices and norms which divide technical from non-technical teams minimizes the chance of developing well-considered AI systems that can safeguard and improve human well-being.
Closing the Gap
Criteria of an Effective Framework for Responsible AI
Given the proposed explanations above, how can we begin to close the principles-to-practices gap? We think an overarching framework for responsible AI development can help to streamline practice and leverage existing tools and methodologies. What would be the desiderata of such a framework for responsible AI?[Akin to what Dignum calls `Ethics in Design’ As a starting point and based on the identified gaps, we suggest the following:
- Broad : it should consider AI’s impacts expansively, across many different ethical issues and aspects of social and economic life. Narrower tools and methodologies such as bias mitigation, privacy-by-design, and product design documentationcan then be subsumed under this more comprehensive framework. For example, after identifying the scope of an AI system’s impacts to human-wellbeing, designers could determine which lower-level sub-tools and methodologies are relevant.
- Operationalizable : it should enable users to cast conceptual principles and goals into specific strategies that can be implemented in real-world systems. This includes identifying relevant actions and decisions, assigned to the appropriate stage of an AI’s lifecycle, e.g., use case conception, system development, deployment, monitoring. This also means identifying accountable parties for these decisions at multiple levels of governance — engineers, designers, lawyers, executives. This helps to ensure that accountability for actions is assigned to those with the capacity to implement them.
- Flexible : it should be able to adapt to a wide variety of AI systems, use cases, implementation contexts, and organizational settings. A flexible framework has greater applicability to more kinds of AI systems and use cases, allowing for shared language and learning, while enabling sufficiently customization.
- Iterative : it should be applied throughout the lifecycle of an AI system and repeatedly as the AI system, implementation context, or other external factors change, not only at one point. Responsible AI is not one-and-done.
- Guided : it should be easy to access and understand, with sufficient documentation for users of moderate skill to apply, customize, and troubleshoot across different contexts. It should also be tested in different contexts with evidence of effectiveness made public.
- Participatory : it should incorporate the perspectives and input from stakeholders from a range of disciplines as well asthose that may be impacted by the AI system, especially the public. Translating principles “into business models, workflows, and product design” will be an ongoing effort that requires engineers, computer scientists, social scientists, lawyers, members of the public, and others to work together.
A framework that meets these criteria balances the need for technical specificity with an equally important need for conceiving of AI’simpacts on human well-being in their full breadth and complexity, understanding we cannot fully predict all of AI’s possible ramifications. That is, while some prominent strategies for responsible AI assume there are only a small set of issues to address, such as bias, transparency, and privacy, we have argued that AI’s impacts are more complex.
We think that impact assessments are a promising strategy towards achieving these criteria. Impact assessments have been used historically in human rights, in regulatory contexts, and more recently to study the impact of AI or algorithms. We focus specifically on the recently published IEEE 7010 standard as an exemplarcreated specifically to assess AI’s impacts on human well-being.[IEEE is in the process of developing standards surrounding ethical AI on a variety of topics — bias, privacy, nudging, trustworthiness of news, and overall human well-being. While the authors of this paper were involved in helping to develop IEEE 7010, this paper reflects the individual views of the authors and not an official position of the IEEE.] We argue below that impact assessments like the well-being impact assessment from the IEEE 7010 standard could be adopted by companies pursuing responsible AI development as well as incorporated into the institutions which train future practitioners.
Impact Assessments for Responsible AI
Building on the IEEE 7010 standard, a well-being impact assessment is an iterative process that entails (1) internal analysis, (2) user and stakeholder engagement, and (3) data collection, among other activities. Internal analysis involves broadly assessing the possible harms, risks, and intended and unintended users and uses of an AI system. Here, developers and managers of an AI system carefully consider a wide range of an AI system’s potential impacts on human well-being, not limited to prominent issues like privacy, bias, or transparency.
Critically, assessing impacts requires not just speculating about impacts, but measuring them. Therefore, the user and stakeholder engagement stages of the assessment include learning from users of AI systems as well as others more indirectly impacted to determine how the system impacts their well-being. When developers have access to users, this may include asking them about possible or actual psychological impacts, economic impacts, changes to relationships, work-life balance, or health. . This is again in contrast to strategies which focus solely on technical fixes to issues like bias or privacy during the design stage alone and fail to account for the broader universe of well-being implications.
Finally, data collection based on the continuous assessment of the identified possible impacts is key. Here we refer to the collection and tracing of data related to the impact assessment, which may exceed collection of data related to the development of the AI system itself. Data can be collected through user surveys, focus, groups, publicly-available data sources, or directly as system outputs. In sum, we propose that adherence to – and rigorous documentation of – impact assessments will contribute to the continuous improvement of AI systems by ensuring that organizations are better able to understand and address AI’s many impacts on human well-being.
Not all tools entitled impact assessment' meet our definition. Many existing tools consider only a small scope of possible impacts. Some fail to measure impacts at all, instead focusing on anticipating impacts assumed to be important and applying best practices to avoid associated harms. Inversely, some tools that are not labelled
impact assessments’ might be classified as such under our definition, such as the European Commission’s Ethics Guidelines for Trustworthy AI. Notably, some frameworks have been proposed by the public sector (i.e., governments) and others by non-governmental organizations and companies.
Why is impact assessment as we have defined it a promising approach? First, it can be highly broad , measuring many aspects of human social and economic well-being and even environmental impacts. IEEE 7010 is an exemplar in its breadth. It identifies twelve domains as part of its well-being impact assessment: affect, community, culture, education, economy, environment, health, human settlements, government, psychological/mental well-being, and work.[All discussion of IEEE 7010 is adapted and reprinted with permission from IEEE. Copyrights IEEE 2020. All rights reserved.] For an AI system like a chatbot or autonomous vehicle, the impact assessment may lead to identification of numerous areas of concern like social relationships, the environment, psychological health, and economy. Thus while other responsible AI approaches take into account a far narrower range of concerns, emphasizing largely bias and transparency of algorithms, IEEE 7010’s well-being impact assessment is far more broad-ranging.
Impact assessments can also be highly operationalizable into specific strategies. In IEEE 7010, overarching domains like human well-being or environmental impacts are not just stated in abstract terms, but are measured through specific indicators based on rigorous research. The strategy involves internal analysis, followed by user and stakeholder engagement, both used to determine domains where an AI system can impact human well-being. Next, AI system creators can identify measurable indicators related to each domain, followed by measuring the impacts of their AI system on the selected indicators. For example, through using the well-being impact assessment, an AI developer might identify the environment as an important concern, and increases in air pollution as a specific possible impact. Using validated indicators to measure air pollution, the developer could then assess whether air pollution has increased or decreased.
Next, given the extensive range of possible impacts that can be measured, there is also ample room to customize an impact assessment and make it sufficiently flexible for particular use cases and organizational contexts. ALGO-CARE is one such example of an impact assessment applied specifically to algorithms in the criminal justice system. ALGO-CARE considers context-specific issues like whether human officers retain decision-making discretion and if proposed AI tools improve the criminal justice system in a demonstrable way. Similarly, users of IEEE 7010 would find that their impact assessment approach could be customized to focus on issues ranging from housing to human rights. Impact assessments also typically leave room to determine which actions are taken in response to identified impacts, meaning these responses can be applied in an iterative fashion, not only during the design phase. For example, concerns about impacts of an AI system on pollution could lead to changes not only during an AI system’s design, but also in terms of its implementation in the real world. Moreover, it is impossible to finish an impact assessment only during the design stage, as it requires measuring its impacts in real-world settings.
However, this breadth and flexibility suggest to us that guidance is the most challenging issue currently. Simply, there is no one-to-one mapping from identified impacts or problems with AI systems to individual technical or implementation `fixes’ and creating such a comprehensive mapping is likely not plausible. The breadth and complexity of an AI well-being impact assessment demonstrate the difficulties for any actor who attempts to single-handedly close the gap from principles to practices. Thus, we propose that developing guidance for particular sectors, types of AI systems, and use cases is a necessary and ongoing effort which could leverage a participatory process-driven impact assessment approach that engages different groups of stakeholders.[Importantly, the impact assessment tool selected need not be IEEE 7010’s well-being impact assessment; impact assessment frameworks with developed by the Canadian governmentand AI Noware examples of other promising tools already available.] In particular, developers, policymakers, philosophers, intended and unintended users of the technology being developed, and others could equally contribute in the AI impact assessment process, such as through interviews, focus groups, and participatory design methods.
We are hopeful that more scholars and organizations focused on responsible uses of AI will adopt an assessment approach that measures a wide range of impacts on human well-being and meets the criteria identified above. A key aspect of creating the supportive structure for effective impact assessments will be adopting new educational practices in institutions of higher education as well as organizational changes in firms. We turn to these issues briefly.
Supportive Practices in Institutions of Higher Education
Educational institutions also have an important role to play. Educational systems have undertaken meaningful efforts aimed at increasing ethical sensitivity and decision-making, but have not yet made the changes needed to support responsible AI practice. Of around 200 AI/ML/data science courses reviewed by Saltz et al. (2019), little more than 1 in 10 mentioned ethics in their syllabus or course description. Those that did focused overwhelmingly on bias, fairness, and privacy. While courses focused specifically on AI ethics cover a wider set of issues including consequences of algorithms, technically tractable issues like bias and privacy are still prominent. We suggest that AI ethics education focus not solely on a few prominent or technically tractable issues nor on general awareness building alone, but also on impact assessment as an overarching framework to understand AI’s impacts on human well-being.
AI ethics and design courses should also recruit and serve students of social sciences and humanities (and other `non-technical’ fields). Calls for more STEM education for these individuals often result in them taking a small number of basic computer science or statistics courses. We believe that more fundamental interaction with AI systems is important to build capacity in these students, who should be “capable of grasping technical details” in order to translate abstract principles and concepts from these fields into concrete computer and data ethics practices. In turn, students in social scientists and humanities can help to expand the scope of thinking of their counterparts in engineering and computer science. For example, AI ethics and design courses can facilitate interdisciplinary teamwork that involves the use of impact assessments. Such an approach would allow students to understand the range of AI’s impacts and practice applying relevant tools and methodologies in response. Interdisciplinary teaming could also occur through student extracurricular clubs and contests (not limited to grand prizes) to encourage this kind of cross-disciplinary learning and practice.
Supportive Practices in Business Organizations
Analogous to the educational setting, companies developing or deploying AI should move towards the integration of technical and non-technical teams rather than functional separation of roles, for reasons discussed in the previous section. These integrated teams could include technical developers as well as other individuals tasked with considering impacts of an AI system who may have social science, humanities, business, law, or ethics expertise, or who can represent a typical user’s perspective effectively. Such a change requires establishing practices that are integrated with engineering and software lifecycles and part of the ongoing dialogue characteristic of development processes. Already, organizations have proposed including a residential non-technical thinker tasked with responsible AI — an `ethics engineer’ or ‘responsible AI champion’.
However, we would urge that these integrated teams not remain at an arm’s distance in a way that maintains bifurcated expertise areas and roles. Instead, technical and non-technical team members should aim learn each other’s languages and work jointly. For example, an AI development team could include ethnographers, policy scholars, or philosophers, all tasked with applying a broad impact assessment as the AI system is being created and implemented. While these changes to organizational practice may be difficult, requiring individuals to stretch their boundaries, we believe that a deep level of integration is necessary to bridge the disciplinary divide.
Organizations could also engage in interdisciplinary and interdepartmental cross-training, potentially supported by responsible AI champions or external experts. For example, organizations could facilitate red team exercisesor hypothetical case studies that draw on the impact assessment approach. Practicing even on hypothetical cases allows social science-oriented practitioners and technically-oriented practitioners to learn from one another about how they can define problems, consider solutions, define terminology, etc. This can help diverse disciplinary practitioners begin to learn and establish common language and identify gaps and opportunities in each other’s practice.
In summary, we have argued that impact assessments are a promising strategy to address the gaps between principles and effective practices for responsible AI. However, applying an impact assessment might feel like an abstract exercise to those who have not done it. To demonstrate how closing the principles-to-practices gaps with an impact assessment might occur, we move now to a case study.
Case Study: Impact Assessments to Support Responsible AI for Forest Ecosystem Restoration
In this section, we set out to explore how the recommendations introduced above could be implemented within a particular setting. We hope this case study will help practitioners in adapting our research findings to the unique sociotechnical context within which their own work is situated. In the example case study below, we look at AI systems that are being used to address forest ecosystem restoration.
Case Study Background
As is characteristic of the SDGs, achieving goals in one area – like the environment – also has effects on multiple other goals, such as addressing health and poverty targets. Forest restoration is one such aspect of the SDGs. While it has clear importance to SDG 13 (Climate Action) and SDG 12 (Responsible Consumption and Production), forest ecosystem restoration is addressed most directly by SDG 15 (Life on Land). SDG 15 states a global ambition to “Protect, restore and promote sustainable use of terrestrial ecosystems, sustainably manage forests, combat desertification, and halt and reverse land degradation and halt biodiversity loss”.
Forest ecosystem restoration is therefore essential for many reasons. Forests have the most species diversity on the planet, with some 80% of land-based species. Forests also reduce the risk of natural disasters such as floods, droughts, and landslides and help protect watersheds. Further, forests are critical for mitigating land-based carbon emissions by increasing carbon sequestration, critical for climate change prevention goals. Project Drawdown, for example, has calculated that the restoration and protection of tropical forests could lead to 61.23 gigatons of carbon reduction by 2050.
Achieving these goals requires the restoration of forest ecosystems through the cultivation of trees, known as afforestation. Applied afforestation projects typically involve three stages - planning, execution, and monitoring of ecosystem restoration. Several AI technologies have been used in afforestation efforts and their use is increasing. During planning, AI systems have been used to predict forest carbon sequestration potential through the use of satellite and drone image data. AI can also facilitate execution of afforestation through computer vision algorithms used in identifying appropriate planting sites, monitoring plant health, and analyzing trends. Lastly, in the monitoring stage of restoration projects, AI can be used to identify where deforestation may have been conducted illegally, as well as assess risks due to fire, disease, insects, or other causes.
Current Challenges
While AI thus has great potential to contribute to SDG efforts and social good in this case, there are complications with translating the aforementioned goals into responsible practices. We focus on one specific challenge leading to a gap in responsible AI practice – the issue of multi-stakeholder coordination.
According to international governance efforts like the UN SDGs, the UN Forum on Forests, Agenda 21, and the Future We Want (the outcome document of the Rio+20 Conference) there is a need for holistic, multi-stakeholder engagement to address forest ecosystem restoration adequately. This is due to the existence of multiple groups with critical interests in forest ecosystems. Local communities and businesses may engage in harvesting timber, farming, and industrial exploitation to produce resources and support local economies. Natives living off the land have an essential stake, as their livelihood may depends on hunting animals and harvesting plants and other materials. Government officials tasked with maintaining forests or woodlands need to monitor the quantity and kind of trees to harvest, and NGOs focused on conservationism may attend to animal life and biodiversity as well. Finally, policymakers must also worry about carbon sequestration and climate change efforts.
Though the goals of these groups are not always in conflict, they can come from different perspectives and have competing priorities. Therefore, AI-driven systems used for afforestation that do not take into account these “multiple ecological, economic, social and cultural roles” important to various stakeholdersmay lead to blind spots and unintended harms. For example, an AI system that uses imaging data to determine carbon sequestration potential could optimize climate change goals in a narrow sense, but fail to account for social-ecological aspects of the land important to indigenous groups, or ignore endangered species important to conservationists. This could engender a lack of coordination and collaboration among stakeholders and lead to costly delays and conflict, as parties are unwilling to accept afforestation efforts or even work actively against them.
As a result, carbon sequestration targets optimized in the short term could fall short in the long term as afforestation progress fails to translate into a sustainably managed multi-stakeholder effort. Failing to develop and implement AI systems for ecosystem restoration in a participatory fashion is thus an example of how the laudable goal of improving environmental well-being can fail to translate into responsible and effective practices.
Applying Impact Assessment
It is therefore important for developers of AI systems to consider that numerous groups have stakes in forest ecosystem restoration. As discussed by Rolnick et al. in the case of AI, “Each stakeholder has different interests, and each often has access to a different portion of the data that would be useful for impactful [machine learning] applications. Interfacing between these different stakeholders is a practical challenge for meaningful work in this area.” Landowners, policymakers, public and private sector organizations, local communities, and others need to have a voice in the application of AI to forest ecosystem restoration.
How would AI impact assessments such as the IEEE 7010 well-being impact assesssment help in this instance? As discussed in Section 3, the assessment process involves a broad internal analysis by the organizations developing AI systems for forest ecosystem restoration. This would involve trying to understand the variety of possible stakeholders and intended or unintended impacts of their products. A company that develops AI to identify target areas for afforestation given carbon sequestration potential might recognize possible impacts on species diversity, the local economy, and the general well-being of native groups.
In order to have a more accurate picture – as well as to build consensus among stakeholders – the company would then begin the user and stakeholder engagement process. This would involve talking to local governments procuring the company’s AI systems about the need for a holistic implementation of afforestation efforts. Critically, it would involve soliciting the input of the numerous stakeholders mentioned such as conservation groups, landowners, scientists, government officials, local businesses, and native populations. For example, a method like participatory action research or other participatory design methodscould be used to facilitate this engagement.
This process, which should be ongoing and iterative throughout the management of the forest ecosystem, should surface a number of clear concerns about possible implications of the afforestation efforts. For example, the company may have originally been optimizing a target through their AI system such as SDG indicators 15.1.1, “Forest area as a proportion of total land area,” or 15.3.1, “Proportion of land that is degraded over total land area.” However, the impact assessment process should lead to the flexible identification of new indicators critical to having a broader understanding of the broader social, economic, and ecological context.
These new indicators – reflecting economic, health, and governance concerns as well as environmental ones – could include, for example, SDG indicators 3.3.5, “Number of people requiring interventions against neglected tropical diseases,” 1.5.2, “Direct disaster economic loss in relation to global gross domestic product,” or 11.3.2 “Proportion of cities with direct participation structure of civil society in urban planning and management that operate regularly and democratically.” These and other indicators, not necessarily picked from the SDG indicators, would therefore operationalize possible dimensions and impacts of the forest ecosystem management effort – disease, natural disasters, and participatory governance – as specific measurable indicators. The company in collaboration with partners would endeavor to measure these impacts, not merely carbon sequestration or forest area as a proportion of land area.
Finally, the company in collaboration with partners, would have several ways to use this new and deeper understanding of the well-being implications of their AI system. One such approach would be embedding this expert domain knowledge garnered from the participatory process into the architecture of the AI system itself. For example, an AI system that previously optimized carbon sequestration potential as part of its objective function could incorporate new data regarding tropical diseases or natural disasters as additional constraints or targets in the optimization of its model.
However, not all efforts to address the identified well-being impacts need be strictly technical in nature. Changes to organizational practices and governance strategies are likely called for. For example, the company might find that accounting for species diversity directly within the model is not sufficiently nuanced. Instead, the company could bring initial recommendations about carbon sequestration target areas to a multi-stakeholder governance board. The board could then offer feedback on the suitability of the recommendations given species diversity or native land usage. While the impact assessment process and identification of solutions would initially feel unfamiliar and complex, the company would gradually develop best practices and guidance towards a more responsible application of its AI system for forest ecosystem restoration.
While this case study – the use of AI for forest ecosystem restoration – is based on real uses of AI and associated real-world challenges, the specific indicators and actions taken by the company are hypothetical. We do not mean to suggest that there are not companies or governments already taking thoughtful approaches to multi-stakeholder governance in this area. However, to the best of the authors’ knowledge, current sustainability efforts have not yet incorporated impact assessments of AI-driven technological solutions applied to ecosystem restoration. We hope this case study helps demonstrate how impact assessments are a promising tool to close the principles-to-practices gap towards responsible AI.
Conclusion
In this paper, we reviewed and synthesized explanations for the gap between high-level responsible AI principles and the capacity to implement those principles in practice. We identified five explanations for the gap, related to the complexity of AI’s impacts on well-being, the distribution of accountability, socio-technical and disciplinary divides, a lack of clarity and guidance around tool usage, and functional separations within organizations that preclude effective interdisciplinary practices.
Next, we considered the criteria of a framework likely to help close the principles-to-practices gap, and identified that impact assessment is one such approach. An impact assessment approach to responsible AI, unlike some alternative approaches, has the potential to be broad, operationalizable, flexible, iterative, guided, and participatory. After reviewing the benefits of impact assessment and the well-being impact assessment approach of IEEE 7010, we suggested changes that educational institutions and companies can make to supportive effective and responsible AI practices.
Finally, we considered the use of AI in forest ecosystem restoration efforts. In the face of complex impacts and possible conflicts between stakeholders that could inhibit sustainable forest management efforts, impact assessment offers promise for those wishing to close the principles-to-practices gap.
Bibliography
1@article{Obaidy2015,
2 url = {http://www.sciencedirect.com/science/article/pii/S2210537914000778},
3 keywords = {Sensor networks},
4 issn = {2210-5379},
5 doi = {http://dx.doi.org/10.1016/j.suscom.2014.09.004},
6 abstract = {Abstract In this work we are presenting the design of an intelligent hybrid optimization algorithm which is based on Evolutionary Computation and Swarm Intelligence to increase the life time of mobile wireless sensor networks (WSNs). It is composed of two phases; Phase-1 is designed to divide the sensor nodes into independent clusters by using Genetic Algorithms (GAs) to minimise the overall communication distance between the sensor-nodes and the sink-point. This will decrease the energy consumption for the entire network. Phase-2 which is based on Particle Swarm Optimization (PSO) is designed to keep the optimum distribution of sensors while the mobile sensor network is directed as a swarm to achieve a given goal. One of the main strengths in the presented algorithm is that the number of clusters within the sensor network is not predefined, this gives more flexibility for the nodes’ deployment in the sensor network. Another strength is that sensors’ density is not necessary to be uniformly distributed among the clusters, since in some applications constraints, the sensors need to be deployed in different densities depending on the nature of the application domain. Although traditionally Wireless Sensor Network have been regarded as static sensor arrays used mainly for environmental monitoring, recently, its applications have undergone a paradigm shift from static to more dynamic environments, where nodes are attached to moving objects, people or animals. Applications that use \{WSNs\} in motion are broad, ranging from transport and logistics to animal monitoring, health care and military. These application domains have a number of characteristics that challenge the algorithmic design of WSNs. },
7 volume = {5},
8 pages = {54 - 63},
9 year = {2015},
10 journal = {Sustainable Computing: Informatics and Systems },
11 author = {Mohaned Al. Obaidy and Aladdin Ayesh},
12 title = {Energy efficient algorithm for swarmed sensors networks },
13}
14
15@phdthesis{RASOL2007,
16 year = {2007},
17 school = {Faculty of Technology, De Montfort University},
18 author = {Rasol, Abdul Manaf Mohd},
19 title = {An Agent-Based Framework for Forest Planning},
20}
21
22@article{march_business_1962,
23 file = {Snapshot:C\:\\Users\\dssch\\Zotero\\storage\\GRUTL49L\\S0022381600016169.html:text/html},
24 pages = {662--678},
25 year = {1962},
26 month = {November},
27 author = {March, James G.},
28 journal = {The Journal of Politics},
29 urldate = {2019-01-23},
30 number = {4},
31 doi = {10.1017/S0022381600016169},
32 url = {https://www.journals.uchicago.edu/doi/abs/10.1017/S0022381600016169},
33 issn = {0022-3816},
34 volume = {24},
35 title = {The {Business} {Firm} as a {Political} {Coalition}},
36}
37
38@incollection{schomberg_vision_2013,
39 file = {Snapshot:C\:\\Users\\dssch\\Zotero\\storage\\RT5XKWFN\\9781118551424.html:text/html},
40 pages = {51--74},
41 keywords = {collective responsibility, grand challenges, responsible research and innovation (RRI)},
42 doi = {10.1002/9781118551424.ch3},
43 year = {2013},
44 author = {Schomberg, René von},
45 publisher = {Wiley-Blackwell},
46 booktitle = {Responsible {Innovation}},
47 urldate = {2018-08-24},
48 language = {en},
49 abstract = {This chapter outlines a vision behind responsible research and innovation (RRI), taking a largely European policy perspective. It provides a definition of the concept and proposes a broad framework for its implementation under research and innovation schemes around the world. The author makes the case that RRI should be understood as a strategy of stakeholders to become mutually responsive to each other, anticipating research and innovation outcomes aimed at the “grand challenges” of our time, for which they share responsibility. Research and innovation processes need to become more responsive and adaptive to these grand challenges. This implies, among others, the introduction of broader foresight and impact assessments for new technologies, beyond their anticipated market-benefits and risks.},
50 url = {https://onlinelibrary.wiley.com/doi/abs/10.1002/9781118551424.ch3},
51 isbn = {978-1-118-55142-4},
52 copyright = {Copyright © 2013 John Wiley \& Sons, Ltd},
53 title = {A {Vision} of {Responsible} {Research} and {Innovation}},
54}
55
56@article{autor_why_2015,
57 file = {Autor - 2015 - Why Are There Still So Many Jobs The History and .pdf:C\:\\Users\\dssch\\Zotero\\storage\\62IZ8C9Y\\Autor - 2015 - Why Are There Still So Many Jobs The History and .pdf:application/pdf},
58 pages = {3--30},
59 year = {2015},
60 month = {August},
61 author = {Autor, David H.},
62 journal = {Journal of Economic Perspectives},
63 urldate = {2018-08-23},
64 number = {3},
65 language = {en},
66 doi = {10.1257/jep.29.3.3},
67 url = {http://pubs.aeaweb.org/doi/10.1257/jep.29.3.3},
68 shorttitle = {Why {Are} {There} {Still} {So} {Many} {Jobs}?},
69 issn = {0895-3309},
70 volume = {29},
71 title = {Why {Are} {There} {Still} {So} {Many} {Jobs}? {The} {History} and {Future} of {Workplace} {Automation}},
72}
73
74@techreport{ibm_everyday_2018,
75 pages = {26},
76 year = {2018},
77 month = {September},
78 author = {{IBM}},
79 institution = {IBM},
80 url = {https://www.ibm.com/watson/assets/duo/pdf/everydayethics.pdf},
81 title = {Everyday {Ethics} for {Artificial} {Intelligence}},
82 address = {New York, NY},
83}
84
85@techreport{sap_saps_2018,
86 pages = {12},
87 note = {https://news.sap.com/2018/09/sap-guiding-principles-for-artificial-intelligence/},
88 year = {2018},
89 month = {September},
90 author = {{SAP}},
91 institution = {SAP},
92 url = {https://www.sap.com/docs/download/2018/09/940c6047-1c7d-0010-87a3-c30de2ffd8ff.pdf},
93 title = {{SAP}’s guiding principles for artificial intelligence},
94 address = {Waldorf, Germany},
95}
96
97@techreport{google_ai_2018,
98 pages = {3},
99 year = {2018},
100 month = {June},
101 author = {{Google}},
102 institution = {Google},
103 url = {https://www.blog.google/technology/ai/ai-principles/},
104 title = {{AI} at {Google}: our principles},
105 address = {Mountain View, CA},
106}
107
108@techreport{openai_openai_2018,
109 pages = {1},
110 year = {2018},
111 month = {April},
112 author = {{OpenAI}},
113 institution = {OpenA},
114 url = {https://blog.openai.com/openai-charter/},
115 title = {{OpenAI} {Charter}},
116 address = {San Francisco, CA},
117}
118
119@techreport{selbst_fairness_2018,
120 file = {Full Text PDF:C\:\\Users\\dssch\\Zotero\\storage\\2VTRNA53\\Selbst et al. - 2018 - Fairness and Abstraction in Sociotechnical Systems.pdf:application/pdf;Snapshot:C\:\\Users\\dssch\\Zotero\\storage\\Z8W9XSM6\\papers.html:text/html},
121 keywords = {Fairness-Aware Machine Learning, Interdisciplinary, Sociotechnical Systems},
122 year = {2018},
123 month = {August},
124 author = {Selbst, Andrew D. and Boyd, Danah and Friedler, Sorelle and Venkatasubramanian, Suresh and Vertesi, Janet},
125 institution = {Social Science Research Network},
126 urldate = {2019-03-22},
127 number = {ID 3265913},
128 language = {en},
129 abstract = {A key goal of the fair-ML community is to develop machine-learning based systems that, once introduced into a social context, can achieve social and legal outcomes such as fairness, justice, and due process. Bedrock concepts in computer science—such as abstraction and modular design—are used to define notions of fairness and discrimination, to produce fairness-aware learning algorithms, and to intervene at different stages of a decision-making pipeline to produce "fair" outcomes. In this paper, however, we contend that these concepts render technical interventions ineffective, inaccurate, and sometimes dangerously misguided when they enter the societal context that surrounds decision-making systems. We outline this mismatch with five "traps" that fair-ML work can fall into even as it attempts to be more context-aware in comparison to traditional data science. We draw on studies of sociotechnical systems in Science and Technology Studies to explain why such traps occur and how to avoid them. Finally, we suggest ways in which technical designers can mitigate the traps through a refocusing of design in terms of process rather than solutions, and by drawing abstraction boundaries to include social actors rather than purely technical ones.},
130 url = {https://papers.ssrn.com/abstract=3265913},
131 title = {Fairness and {Abstraction} in {Sociotechnical} {Systems}},
132 type = {{SSRN} {Scholarly} {Paper}},
133 address = {Rochester, NY},
134}
135
136@article{greene_better_2019,
137 file = {Greene et al. - 2019 - Better, Nicer, Clearer, Fairer A Critical Assessm.pdf:F\:\\Google Drive\\Documents\\Academics\\GA Tech\\AI Ethics Study\\Literature\\Similar\\Greene et al. - 2019 - Better, Nicer, Clearer, Fairer A Critical Assessm.pdf:application/pdf},
138 pages = {10},
139 year = {2019},
140 author = {Greene, Daniel and Hoffmann, Anna Lauren and Stark, Luke},
141 journal = {Critical and Ethical Studies of Digital and Social Media},
142 language = {en},
143 abstract = {This paper uses frame analysis to examine recent high-profile values statements endorsing ethical design for artificial intelligence and machine learning (AI/ML). Guided by insights from values in design and the sociology of business ethics, we uncover the grounding assumptions and terms of debate that make some conversations about ethical design possible while forestalling alternative visions. Vision statements for ethical AI/ML co-opt the language of some critics, folding them into a limited, technologically deterministic, expert-driven view of what ethical AI/ML means and how it might work.},
144 title = {Better, {Nicer}, {Clearer}, {Fairer}: {A} {Critical} {Assessment} of the {Movement} for {Ethical} {Artificial} {Intelligence} and {Machine} {Learning}},
145}
146
147@techreport{musikanski_ieee_2018,
148 year = {2018},
149 month = {December},
150 author = {Musikanski, Laura and Havens, John and Gunsch, Gregg},
151 institution = {IEEE},
152 title = {{IEEE} {P7010} {Well}-being {Metrics} {Standard} for {Autonomous} and {Intelligent} {Systems}™},
153 address = {New York, NY},
154}
155
156@article{mittelstadt_ai_2019,
157 file = {arXiv.org Snapshot:C\:\\Users\\dssch\\Zotero\\storage\\BTDBL5WD\\1906.html:text/html;Mittelstadt - 2019 - AI Ethics -- Too Principled to Fail.pdf:F\:\\Google Drive\\Documents\\Academics\\GA Tech\\AI Ethics Study\\Literature\\Similar\\Mittelstadt - 2019 - AI Ethics -- Too Principled to Fail.pdf:application/pdf},
158 keywords = {Computer Science - Computers and Society, Computer Science - Artificial Intelligence},
159 note = {arXiv: 1906.06668},
160 year = {2019},
161 month = {June},
162 author = {Mittelstadt, Brent},
163 journal = {arXiv:1906.06668 [cs]},
164 urldate = {2020-01-13},
165 abstract = {AI Ethics is now a global topic of discussion in academic and policy circles. At least 63 public-private initiatives have produced statements describing high-level principles, values, and other tenets to guide the ethical development, deployment, and governance of AI. According to recent meta-analyses, AI Ethics has seemingly converged on a set of principles that closely resemble the four classic principles of medical ethics. Despite the initial credibility granted to a principled approach to AI Ethics by the connection to principles in medical ethics, there are reasons to be concerned about its future impact on AI development and governance. Significant differences exist between medicine and AI development that suggest a principled approach in the latter may not enjoy success comparable to the former. Compared to medicine, AI development lacks (1) common aims and fiduciary duties, (2) professional history and norms, (3) proven methods to translate principles into practice, and (4) robust legal and professional accountability mechanisms. These differences suggest we should not yet celebrate consensus around high-level principles that hide deep political and normative disagreement.},
166 url = {http://arxiv.org/abs/1906.06668},
167 title = {{AI} {Ethics} -- {Too} {Principled} to {Fail}?},
168}
169
170@article{morley_what_2019,
171 file = {Morley et al. - 2019 - From What to How An Initial Review of Publicly Av.pdf:F\:\\Google Drive\\Documents\\Academics\\GA Tech\\AI Ethics Study\\Literature\\Similar\\Morley et al. - 2019 - From What to How An Initial Review of Publicly Av.pdf:application/pdf},
172 keywords = {Artificial intelligence, Machine learning, Data governance, Digital ethics, Ethics of AI, Governance, Applied ethics},
173 year = {2019},
174 month = {December},
175 author = {Morley, Jessica and Floridi, Luciano and Kinsey, Libby and Elhalal, Anat},
176 journal = {Science and Engineering Ethics},
177 urldate = {2020-01-13},
178 language = {en},
179 abstract = {The debate about the ethical implications of Artificial Intelligence dates from the 1960s (Samuel in Science, 132(3429):741–742, 1960. https://doi.org/10.1126/science.132.3429.741; Wiener in Cybernetics: or control and communication in the animal and the machine, MIT Press, New York, 1961). However, in recent years symbolic AI has been complemented and sometimes replaced by (Deep) Neural Networks and Machine Learning (ML) techniques. This has vastly increased its potential utility and impact on society, with the consequence that the ethical debate has gone mainstream. Such a debate has primarily focused on principles—the ‘what’ of AI ethics (beneficence, non-maleficence, autonomy, justice and explicability)—rather than on practices, the ‘how.’ Awareness of the potential issues is increasing at a fast rate, but the AI community’s ability to take action to mitigate the associated risks is still at its infancy. Our intention in presenting this research is to contribute to closing the gap between principles and practices by constructing a typology that may help practically-minded developers apply ethics at each stage of the Machine Learning development pipeline, and to signal to researchers where further work is needed. The focus is exclusively on Machine Learning, but it is hoped that the results of this research may be easily applicable to other branches of AI. The article outlines the research method for creating this typology, the initial findings, and provides a summary of future research needs.},
180 doi = {10.1007/s11948-019-00165-5},
181 url = {https://doi.org/10.1007/s11948-019-00165-5},
182 shorttitle = {From {What} to {How}},
183 issn = {1471-5546},
184 title = {From {What} to {How}: {An} {Initial} {Review} of {Publicly} {Available} {AI} {Ethics} {Tools}, {Methods} and {Research} to {Translate} {Principles} into {Practices}},
185}
186
187@article{floridi_unified_2019,
188 file = {Floridi and Cowls - 2019 - A Unified Framework of Five Principles for AI in S.pdf:F\:\\Google Drive\\Documents\\Academics\\GA Tech\\AI Ethics Study\\Literature\\Similar\\Floridi and Cowls - 2019 - A Unified Framework of Five Principles for AI in S.pdf:application/pdf},
189 year = {2019},
190 month = {June},
191 author = {Floridi, Luciano and Cowls, Josh},
192 journal = {Harvard Data Science Review},
193 urldate = {2020-01-13},
194 language = {en},
195 doi = {10.1162/99608f92.8cd550d1},
196 url = {https://hdsr.mitpress.mit.edu/pub/l0jsh9d1},
197 title = {A {Unified} {Framework} of {Five} {Principles} for {AI} in {Society}},
198}
199
200@article{hagendorff_ethics_2020,
201 month = {Feb},
202 year = {2020},
203 author = {Hagendorff, Thilo},
204 journal = {Minds and Machines},
205 abstractnote = {Current advances in research, development and application of artificial intelligence (AI) systems have yielded a far-reaching discourse on AI ethics. In consequence, a number of ethics guidelines have been released in recent years. These guidelines comprise normative principles and recommendations aimed to harness the “disruptive” potentials of new AI technologies. Designed as a semi-systematic evaluation, this paper analyzes and compares 22 guidelines, highlighting overlaps but also omissions. As a result, I give a detailed overview of the field of AI ethics. Finally, I also examine to what extent the respective ethical principles and values are implemented in the practice of research, development and application of AI systems—and how the effectiveness in the demands of AI ethics can be improved.},
206 doi = {10.1007/s11023-020-09517-8},
207 url = {https://doi.org/10.1007/s11023-020-09517-8},
208 issn = {1572-8641},
209 title = {The Ethics of AI Ethics: An Evaluation of Guidelines},
210}
211
212@inproceedings{schiff_whats_2020,
213 file = {Full Text PDF:C\:\\Users\\dssch\\Zotero\\storage\\6A6ZBZNN\\Schiff et al. - 2020 - What's Next for AI Ethics, Policy, and Governance.pdf:application/pdf},
214 pages = {153--158},
215 keywords = {corporate social responsibility, ai ethics, ai policy},
216 year = {2020},
217 month = {February},
218 author = {Schiff, Daniel and Biddle, Justin and Borenstein, Jason and Laas, Kelly},
219 publisher = {Association for Computing Machinery},
220 booktitle = {Proceedings of the {AAAI}/{ACM} {Conference} on {AI}, {Ethics}, and {Society}},
221 urldate = {2020-02-13},
222 abstract = {Since 2016, more than 80 AI ethics documents - including codes, principles, frameworks, and policy strategies - have been produced by corporations, governments, and NGOs. In this paper, we examine three topics of importance related to our ongoing empirical study of ethics and policy issues in these emerging documents. First, we review possible challenges associated with the relative homogeneity of the documents' creators. Second, we provide a novel typology of motivations to characterize both obvious and less obvious goals of the documents. Third, we discuss the varied impacts these documents may have on the AI governance landscape, including what factors are relevant to assessing whether a given document is likely to be successful in achieving its goals.},
223 doi = {10.1145/3375627.3375804},
224 url = {https://doi.org/10.1145/3375627.3375804},
225 shorttitle = {What's {Next} for {AI} {Ethics}, {Policy}, and {Governance}?},
226 isbn = {978-1-4503-7110-0},
227 title = {What's {Next} for {AI} {Ethics}, {Policy}, and {Governance}? {A} {Global} {Overview}},
228 series = {{AIES} '20},
229 address = {New York, NY, USA},
230}
231
232@techreport{vodafone_group_plc_vodafones_2019,
233 pages = {7},
234 year = {2019},
235 month = {June},
236 author = {{Vodafone Group Plc}},
237 institution = {Vodafone},
238 url = {https://www.vodafone.com/content/dam/vodcom/images/public-policy/artificial-intelligence-framework/55678_Vodafone_AI_Framework_AW1_V3.pdf},
239 title = {Vodafone's {AI} {Framework}},
240 address = {Newbury, United Kingdom},
241}
242
243@techreport{tieto_corporation_tietos_2018,
244 pages = {1},
245 year = {2018},
246 month = {October},
247 author = {{Tieto Corporation}},
248 institution = {Tieto Corporation},
249 url = {https://www.tieto.com/contentassets/964a20887f764aae944e4f029d05ff51/tieto-s-ai-ethics-guidelines.pdf},
250 title = {Tieto's {AI} ethics guidelines},
251 address = {Espoo, Finland},
252}
253
254@techreport{futurice_futurice_2018,
255 pages = {6},
256 year = {2018},
257 month = {November},
258 author = {{Futurice}},
259 institution = {Futurice},
260 url = {https://www.futurice.com/blog/introducing-the-futurice-principles-for-ethical-ai/},
261 title = {The {Futurice} {Principles} for {Ethical} {AI}},
262 address = {Helsinki, Finland},
263}
264
265@techreport{salesforce_salesforce_2019,
266 pages = {2},
267 year = {2019},
268 month = {March},
269 author = {{Salesforce}},
270 institution = {Salesforce},
271 title = {Salesforce {AI} {Ethics}},
272 address = {San Francisco, CA},
273}
274
275@article{jacobs_why_2018,
276 file = {Springer Full Text PDF:C\:\\Users\\dssch\\Zotero\\storage\\5ELMALNH\\Jacobs and Huldtgren - 2018 - Why value sensitive design needs ethical commitmen.pdf:application/pdf},
277 year = {2018},
278 month = {July},
279 author = {Jacobs, Naomi and Huldtgren, Alina},
280 journal = {Ethics and Information Technology},
281 urldate = {2020-03-02},
282 language = {en},
283 abstract = {Currently, value sensitive design (VSD) does not commit to a particular ethical theory. Critiques contend that without such an explicit commitment, VSD lacks a methodology for distinguishing genuine moral values from mere stakeholders-preferences and runs the risk of attending to a set of values that is unprincipled or unbounded. We argue that VSD practitioners need to complement it with an ethical theory. We argue in favour of a mid-level ethical theory to fulfil this role.},
284 doi = {10.1007/s10676-018-9467-3},
285 url = {https://doi.org/10.1007/s10676-018-9467-3},
286 issn = {1572-8439},
287 title = {Why value sensitive design needs ethical commitments},
288}
289
290@article{manders-huits_what_2011,
291 file = {Springer Full Text PDF:C\:\\Users\\dssch\\Zotero\\storage\\UJHN2QYT\\Manders-Huits - 2011 - What Values in Design The Challenge of Incorporat.pdf:application/pdf},
292 pages = {271--287},
293 year = {2011},
294 month = {June},
295 author = {Manders-Huits, Noëmi},
296 journal = {Science and Engineering Ethics},
297 urldate = {2020-03-02},
298 number = {2},
299 language = {en},
300 abstract = {Recently, there is increased attention to the integration of moral values into the conception, design, and development of emerging IT. The most reviewed approach for this purpose in ethics and technology so far is Value-Sensitive Design (VSD). This article considers VSD as the prime candidate for implementing normative considerations into design. Its methodology is considered from a conceptual, analytical, normative perspective. The focus here is on the suitability of VSD for integrating moral values into the design of technologies in a way that joins in with an analytical perspective on ethics of technology. Despite its promising character, it turns out that VSD falls short in several respects: (1) VSD does not have a clear methodology for identifying stakeholders, (2) the integration of empirical methods with conceptual research within the methodology of VSD is obscure, (3) VSD runs the risk of committing the naturalistic fallacy when using empirical knowledge for implementing values in design, (4) the concept of values, as well as their realization, is left undetermined and (5) VSD lacks a complimentary or explicit ethical theory for dealing with value trade-offs. For the normative evaluation of a technology, I claim that an explicit and justified ethical starting point or principle is required. Moreover, explicit attention should be given to the value aims and assumptions of a particular design. The criteria of adequacy for such an approach or methodology follow from the evaluation of VSD as the prime candidate for implementing moral values in design.},
301 doi = {10.1007/s11948-010-9198-2},
302 url = {https://doi.org/10.1007/s11948-010-9198-2},
303 shorttitle = {What {Values} in {Design}?},
304 issn = {1471-5546},
305 volume = {17},
306 title = {What {Values} in {Design}? {The} {Challenge} of {Incorporating} {Moral} {Values} into {Design}},
307}
308
309@article{zhao_improving_2018,
310 file = {Full Text:C\:\\Users\\dssch\\Zotero\\storage\\EU6ZEIJD\\Zhao - 2018 - Improving Social Responsibility of Artificial Inte.pdf:application/pdf},
311 pages = {012049},
312 year = {2018},
313 month = {October},
314 author = {Zhao, Wei-Wei},
315 journal = {IOP Conference Series: Materials Science and Engineering},
316 urldate = {2020-03-02},
317 doi = {10.1088/1757-899X/428/1/012049},
318 url = {http://stacks.iop.org/1757-899X/428/i=1/a=012049?key=crossref.0473e278f03c3198e25b68ed24c46b8e},
319 issn = {1757-899X},
320 volume = {428},
321 title = {Improving {Social} {Responsibility} of {Artificial} {Intelligence} by {Using} {ISO} 26000},
322}
323
324@article{oetzel_systematic_2014,
325 file = {Full Text PDF:C\:\\Users\\dssch\\Zotero\\storage\\2JZ5TWME\\Oetzel and Spiekermann - 2014 - A systematic methodology for privacy impact assess.pdf:application/pdf;Snapshot:C\:\\Users\\dssch\\Zotero\\storage\\R4SDNFPP\\ejis.2013.html:text/html},
326 pages = {126--150},
327 keywords = {design science, privacy impact assessment, privacy-by-design, security risk assessment},
328 note = {Publisher: Taylor \& Francis
329\_eprint: https://doi.org/10.1057/ejis.2013.18},
330 year = {2014},
331 month = {March},
332 author = {Oetzel, Marie Caroline and Spiekermann, Sarah},
333 journal = {European Journal of Information Systems},
334 urldate = {2020-03-02},
335 number = {2},
336 abstract = {For companies that develop and operate IT applications that process the personal data of customers and employees, a major problem is protecting these data and preventing privacy breaches. Failure to adequately address this problem can result in considerable damage to the company's reputation and finances, as well as negative effects for customers or employees (data subjects). To address this problem, we propose a methodology that systematically considers privacy issues by using a step-by-step privacy impact assessment (PIA). Existing PIA approaches cannot be applied easily because they are improperly structured or imprecise and lengthy. We argue that companies that employ our PIA can achieve ‘privacy-by-design’, which is widely heralded by data protection authorities. In fact, the German Federal Office for Information Security (BSI) ratified the approach we present in this article for the technical field of RFID and published it as a guideline in November 2011. The contribution of the artefacts we created is twofold: First, we provide a formal problem representation structure for the analysis of privacy requirements. Second, we reduce the complexity of the privacy regulation landscape for practitioners who need to make privacy management decisions for their IT applications.},
337 doi = {10.1057/ejis.2013.18},
338 url = {https://doi.org/10.1057/ejis.2013.18},
339 shorttitle = {A systematic methodology for privacy impact assessments},
340 issn = {0960-085X},
341 volume = {23},
342 title = {A systematic methodology for privacy impact assessments: a design science approach},
343}
344
345@article{guidotti_survey_2018,
346 file = {Full Text PDF:C\:\\Users\\dssch\\Zotero\\storage\\WMV4XUJF\\Guidotti et al. - 2018 - A Survey of Methods for Explaining Black Box Model.pdf:application/pdf},
347 pages = {93:1--93:42},
348 keywords = {explanations, interpretability, Open the black box, transparent models},
349 year = {2018},
350 month = {August},
351 author = {Guidotti, Riccardo and Monreale, Anna and Ruggieri, Salvatore and Turini, Franco and Giannotti, Fosca and Pedreschi, Dino},
352 journal = {ACM Computing Surveys},
353 urldate = {2020-03-02},
354 number = {5},
355 abstract = {In recent years, many accurate decision support systems have been constructed as black boxes, that is as systems that hide their internal logic to the user. This lack of explanation constitutes both a practical and an ethical issue. The literature reports many approaches aimed at overcoming this crucial weakness, sometimes at the cost of sacrificing accuracy for interpretability. The applications in which black box decision systems can be used are various, and each approach is typically developed to provide a solution for a specific problem and, as a consequence, it explicitly or implicitly delineates its own definition of interpretability and explanation. The aim of this article is to provide a classification of the main problems addressed in the literature with respect to the notion of explanation and the type of black box system. Given a problem definition, a black box type, and a desired explanation, this survey should help the researcher to find the proposals more useful for his own work. The proposed classification of approaches to open black box models should also be useful for putting the many research open questions in perspective.},
356 doi = {10.1145/3236009},
357 url = {https://doi.org/10.1145/3236009},
358 issn = {0360-0300},
359 volume = {51},
360 title = {A {Survey} of {Methods} for {Explaining} {Black} {Box} {Models}},
361}
362
363@article{friedman_survey_2017,
364 file = {Full Text PDF:C\:\\Users\\dssch\\Zotero\\storage\\QCPG7ULX\\Friedman et al. - 2017 - A Survey of Value Sensitive Design Methods.pdf:application/pdf;Snapshot:C\:\\Users\\dssch\\Zotero\\storage\\I3RCPDHM\\HCI-015.html:text/html},
365 pages = {63--125},
366 note = {Publisher: Now Publishers, Inc.},
367 year = {2017},
368 month = {November},
369 author = {Friedman, Batya and Hendry, David G. and Borning, Alan},
370 journal = {Foundations and Trends® in Human–Computer Interaction},
371 urldate = {2020-03-02},
372 number = {2},
373 language = {English},
374 abstract = {A Survey of Value Sensitive Design Methods},
375 doi = {10.1561/1100000015},
376 url = {https://www.nowpublishers.com/article/Details/HCI-015},
377 issn = {1551-3955, 1551-3963},
378 volume = {11},
379 title = {A {Survey} of {Value} {Sensitive} {Design} {Methods}},
380}
381
382@incollection{bolukbasi_man_2016,
383 file = {NIPS Snapshot:C\:\\Users\\dssch\\Zotero\\storage\\UWYEI5GM\\6228-man-is-to-computer-programmer-as-woman-is-to-homemaker-d.html:text/html},
384 pages = {4349--4357},
385 year = {2016},
386 editor = {Lee, D. D. and Sugiyama, M. and Luxburg, U. V. and Guyon, I. and Garnett, R.},
387 author = {Bolukbasi, Tolga and Chang, Kai-Wei and Zou, James Y and Saligrama, Venkatesh and Kalai, Adam T},
388 publisher = {Curran Associates, Inc.},
389 booktitle = {Advances in {Neural} {Information} {Processing} {Systems} 29},
390 urldate = {2020-03-02},
391 url = {http://papers.nips.cc/paper/6228-man-is-to-computer-programmer-as-woman-is-to-homemaker-debiasing-word-embeddings.pdf},
392 shorttitle = {Man is to {Computer} {Programmer} as {Woman} is to {Homemaker}?},
393 title = {Man is to {Computer} {Programmer} as {Woman} is to {Homemaker}? {Debiasing} {Word} {Embeddings}},
394}
395
396@article{vakkuri_ethically_2019,
397 file = {arXiv Fulltext PDF:C\:\\Users\\dssch\\Zotero\\storage\\GHG4XRY4\\Vakkuri et al. - 2019 - Ethically Aligned Design of Autonomous Systems In.pdf:application/pdf;arXiv.org Snapshot:C\:\\Users\\dssch\\Zotero\\storage\\HN7CFK3Z\\1906.html:text/html},
398 keywords = {Computer Science - Computers and Society},
399 note = {arXiv: 1906.07946},
400 year = {2019},
401 month = {June},
402 author = {Vakkuri, Ville and Kemell, Kai-Kristian and Kultanen, Joni and Siponen, Mikko and Abrahamsson, Pekka},
403 journal = {arXiv:1906.07946 [cs]},
404 urldate = {2020-03-02},
405 abstract = {Progress in the field of artificial intelligence has been accelerating rapidly in the past two decades. Various autonomous systems from purely digital ones to autonomous vehicles are being developed and deployed out on the field. As these systems exert a growing impact on society, ethics in relation to artificial intelligence and autonomous systems have recently seen growing attention among the academia. However, the current literature on the topic has focused almost exclusively on theory and more specifically on conceptualization in the area. To widen the body of knowledge in the area, we conduct an empirical study on the current state of practice in artificial intelligence ethics. We do so by means of a multiple case study of five case companies, the results of which indicate a gap between research and practice in the area. Based on our findings we propose ways to tackle the gap.},
406 url = {http://arxiv.org/abs/1906.07946},
407 shorttitle = {Ethically {Aligned} {Design} of {Autonomous} {Systems}},
408 title = {Ethically {Aligned} {Design} of {Autonomous} {Systems}: {Industry} viewpoint and an empirical study},
409}
410
411@article{chouldechova_fair_2017,
412 file = {arXiv Fulltext PDF:C\:\\Users\\dssch\\Zotero\\storage\\295JCW84\\Chouldechova - 2017 - Fair prediction with disparate impact A study of .pdf:application/pdf;arXiv.org Snapshot:C\:\\Users\\dssch\\Zotero\\storage\\373MRD7L\\1703.html:text/html},
413 keywords = {Computer Science - Computers and Society, Statistics - Machine Learning, Statistics - Applications},
414 note = {arXiv: 1703.00056},
415 year = {2017},
416 month = {February},
417 author = {Chouldechova, Alexandra},
418 journal = {arXiv:1703.00056 [cs, stat]},
419 urldate = {2019-12-12},
420 abstract = {Recidivism prediction instruments (RPI's) provide decision makers with an assessment of the likelihood that a criminal defendant will reoffend at a future point in time. While such instruments are gaining increasing popularity across the country, their use is attracting tremendous controversy. Much of the controversy concerns potential discriminatory bias in the risk assessments that are produced. This paper discusses several fairness criteria that have recently been applied to assess the fairness of recidivism prediction instruments. We demonstrate that the criteria cannot all be simultaneously satisfied when recidivism prevalence differs across groups. We then show how disparate impact can arise when a recidivism prediction instrument fails to satisfy the criterion of error rate balance.},
421 url = {http://arxiv.org/abs/1703.00056},
422 shorttitle = {Fair prediction with disparate impact},
423 title = {Fair prediction with disparate impact: {A} study of bias in recidivism prediction instruments},
424}
425
426@inproceedings{leben_normative_2020,
427 file = {Full Text PDF:C\:\\Users\\dssch\\Zotero\\storage\\DCWR7SCF\\Leben - 2020 - Normative Principles for Evaluating Fairness in Ma.pdf:application/pdf},
428 pages = {86--92},
429 keywords = {machine learning, discrimination, algorithmic decision-making, fairness, political philosophy},
430 year = {2020},
431 month = {February},
432 author = {Leben, Derek},
433 publisher = {Association for Computing Machinery},
434 booktitle = {Proceedings of the {AAAI}/{ACM} {Conference} on {AI}, {Ethics}, and {Society}},
435 urldate = {2020-03-02},
436 abstract = {There are many incompatible ways to measure fair outcomes for machine learning algorithms. The goal of this paper is to characterize rates of success and error across protected groups (race, gender, sexual orientation) as a distribution problem, and describe the possible solutions to this problem according to different normative principles from moral and political philosophy. These normative principles are based on various competing attributes within a distribution problem: intentions, compensation, desert, consent, and consequences. Each principle will be applied to a sample risk-assessment classifier to demonstrate the philosophical arguments underlying different sets of fairness metrics.},
437 doi = {10.1145/3375627.3375808},
438 url = {https://doi.org/10.1145/3375627.3375808},
439 isbn = {978-1-4503-7110-0},
440 title = {Normative {Principles} for {Evaluating} {Fairness} in {Machine} {Learning}},
441 series = {{AIES} '20},
442 address = {New York, NY, USA},
443}
444
445@article{mitchell_model_2019,
446 file = {arXiv Fulltext PDF:C\:\\Users\\dssch\\Zotero\\storage\\4B2EEVGY\\Mitchell et al. - 2019 - Model Cards for Model Reporting.pdf:application/pdf;arXiv.org Snapshot:C\:\\Users\\dssch\\Zotero\\storage\\WHXQTTZH\\1810.html:text/html},
447 pages = {220--229},
448 keywords = {Computer Science - Artificial Intelligence, Computer Science - Machine Learning},
449 note = {arXiv: 1810.03993},
450 year = {2019},
451 author = {Mitchell, Margaret and Wu, Simone and Zaldivar, Andrew and Barnes, Parker and Vasserman, Lucy and Hutchinson, Ben and Spitzer, Elena and Raji, Inioluwa Deborah and Gebru, Timnit},
452 journal = {Proceedings of the Conference on Fairness, Accountability, and Transparency - FAT* '19},
453 urldate = {2020-03-02},
454 abstract = {Trained machine learning models are increasingly used to perform high-impact tasks in areas such as law enforcement, medicine, education, and employment. In order to clarify the intended use cases of machine learning models and minimize their usage in contexts for which they are not well suited, we recommend that released models be accompanied by documentation detailing their performance characteristics. In this paper, we propose a framework that we call model cards, to encourage such transparent model reporting. Model cards are short documents accompanying trained machine learning models that provide benchmarked evaluation in a variety of conditions, such as across different cultural, demographic, or phenotypic groups (e.g., race, geographic location, sex, Fitzpatrick skin type) and intersectional groups (e.g., age and race, or sex and Fitzpatrick skin type) that are relevant to the intended application domains. Model cards also disclose the context in which models are intended to be used, details of the performance evaluation procedures, and other relevant information. While we focus primarily on human-centered machine learning models in the application fields of computer vision and natural language processing, this framework can be used to document any trained machine learning model. To solidify the concept, we provide cards for two supervised models: One trained to detect smiling faces in images, and one trained to detect toxic comments in text. We propose model cards as a step towards the responsible democratization of machine learning and related AI technology, increasing transparency into how well AI technology works. We hope this work encourages those releasing trained machine learning models to accompany model releases with similar detailed evaluation numbers and other relevant documentation.},
455 doi = {10.1145/3287560.3287596},
456 url = {http://arxiv.org/abs/1810.03993},
457 title = {Model {Cards} for {Model} {Reporting}},
458}
459
460@article{gebru_datasheets_2020,
461 file = {arXiv Fulltext PDF:C\:\\Users\\dssch\\Zotero\\storage\\CLQL6CUT\\Gebru et al. - 2020 - Datasheets for Datasets.pdf:application/pdf;arXiv.org Snapshot:C\:\\Users\\dssch\\Zotero\\storage\\DPX4HIFE\\1803.html:text/html},
462 keywords = {Computer Science - Artificial Intelligence, Computer Science - Machine Learning, Computer Science - Databases},
463 note = {arXiv: 1803.09010},
464 year = {2020},
465 month = {January},
466 author = {Gebru, Timnit and Morgenstern, Jamie and Vecchione, Briana and Vaughan, Jennifer Wortman and Wallach, Hanna and Daumé III, Hal and Crawford, Kate},
467 journal = {arXiv:1803.09010 [cs]},
468 urldate = {2020-03-02},
469 abstract = {Currently there is no standard way to identify how a dataset was created, and what characteristics, motivations, and potential skews it represents. To begin to address this issue, we propose the concept of a datasheet for datasets, a short document to accompany public datasets, commercial APIs, and pretrained models. The goal of this proposal is to enable better communication between dataset creators and users, and help the AI community move toward greater transparency and accountability. By analogy, in computer hardware, it has become industry standard to accompany everything from the simplest components (e.g., resistors), to the most complex microprocessor chips, with datasheets detailing standard operating characteristics, test results, recommended usage, and other information. We outline some of the questions a datasheet for datasets should answer. These questions focus on when, where, and how the training data was gathered, its recommended use cases, and, in the case of human-centric datasets, information regarding the subjects' demographics and consent as applicable. We develop prototypes of datasheets for two well-known datasets: Labeled Faces in The Wild and the Pang {\textbackslash}\& Lee Polarity Dataset.},
470 url = {http://arxiv.org/abs/1803.09010},
471 title = {Datasheets for {Datasets}},
472}
473
474@article{faulkner_nuts_2007,
475 file = {SAGE PDF Full Text:C\:\\Users\\dssch\\Zotero\\storage\\46JMHXS5\\Faulkner - 2007 - `Nuts and Bolts and People' Gender-Troubled Engin.pdf:application/pdf},
476 pages = {331--356},
477 keywords = {gender, engineer identities, heterogeneity, technical/social dualism},
478 note = {Publisher: SAGE Publications Ltd},
479 year = {2007},
480 month = {June},
481 author = {Faulkner, Wendy},
482 journal = {Social Studies of Science},
483 urldate = {2020-03-03},
484 number = {3},
485 language = {en},
486 abstract = {Engineers have two types of stories about what constitutes `real' engineering. In sociological terms, one is technicist, the other heterogeneous. How and where boundaries are drawn between `the technical' and `the social' in engineering identities and practices is a central concern for feminist technology studies, given the strong marking of sociality as feminine and technology as masculine. I explore these themes, drawing on ethnographic observations of building design engineering. This is a profoundly heterogeneous and networked engineering practice, which entails troubled boundary drawing and identities for the individuals involved — evident in interactions between engineers and architects, and among engineers, especially around management and design. Many engineers cleave to a technicist engineering identity, and even those who embrace the heterogeneous reality of their actual work oscillate between or straddle, not always comfortably, the two identities. There are complex gender tensions, as well as professional tensions, at work here — associated with distinct versions of hegemonic masculinity, with the technical/social dualism, and with what I call `gender in/authenticity' issues. I conclude that technicist engineering identities persist in part because they converge with (and perform) available masculinities, and that women's (perceived and felt) membership as `real' engineers is likely to be more fragile than men's. Engineering as a profession must foreground and celebrate the heterogeneity of engineering work. Improving the representation of women in engineering requires promoting more heterogeneous versions of gender as well as engineering.},
487 doi = {10.1177/0306312706072175},
488 url = {https://doi.org/10.1177/0306312706072175},
489 shorttitle = {`{Nuts} and {Bolts} and {People}'},
490 issn = {0306-3127},
491 volume = {37},
492 title = {`{Nuts} and {Bolts} and {People}': {Gender}-{Troubled} {Engineering} {Identities}},
493}
494
495@article{floridi_distributed_2013,
496 pages = {727--743},
497 note = {Publisher: Springer Verlag},
498 year = {2013},
499 author = {Floridi, Luciano},
500 journal = {Science and Engineering Ethics},
501 number = {3},
502 doi = {10.1007/s11948-012-9413-4},
503 volume = {19},
504 title = {Distributed {Morality} in an {Information} {Society}},
505}
506
507@article{bellamy_ai_2018,
508 file = {arXiv Fulltext PDF:C\:\\Users\\dssch\\Zotero\\storage\\PPVGNHX5\\Bellamy et al. - 2018 - AI Fairness 360 An Extensible Toolkit for Detecti.pdf:application/pdf;arXiv.org Snapshot:C\:\\Users\\dssch\\Zotero\\storage\\3KZQ9AN2\\1810.html:text/html},
509 keywords = {Computer Science - Artificial Intelligence},
510 note = {arXiv: 1810.01943},
511 year = {2018},
512 month = {October},
513 author = {Bellamy, Rachel K. E. and Dey, Kuntal and Hind, Michael and Hoffman, Samuel C. and Houde, Stephanie and Kannan, Kalapriya and Lohia, Pranay and Martino, Jacquelyn and Mehta, Sameep and Mojsilovic, Aleksandra and Nagar, Seema and Ramamurthy, Karthikeyan Natesan and Richards, John and Saha, Diptikalyan and Sattigeri, Prasanna and Singh, Moninder and Varshney, Kush R. and Zhang, Yunfeng},
514 journal = {arXiv:1810.01943 [cs]},
515 urldate = {2020-03-04},
516 abstract = {Fairness is an increasingly important concern as machine learning models are used to support decision making in high-stakes applications such as mortgage lending, hiring, and prison sentencing. This paper introduces a new open source Python toolkit for algorithmic fairness, AI Fairness 360 (AIF360), released under an Apache v2.0 license \{\vphantom{\}}https://github.com/ibm/aif360). The main objectives of this toolkit are to help facilitate the transition of fairness research algorithms to use in an industrial setting and to provide a common framework for fairness researchers to share and evaluate algorithms. The package includes a comprehensive set of fairness metrics for datasets and models, explanations for these metrics, and algorithms to mitigate bias in datasets and models. It also includes an interactive Web experience (https://aif360.mybluemix.net) that provides a gentle introduction to the concepts and capabilities for line-of-business users, as well as extensive documentation, usage guidance, and industry-specific tutorials to enable data scientists and practitioners to incorporate the most appropriate tool for their problem into their work products. The architecture of the package has been engineered to conform to a standard paradigm used in data science, thereby further improving usability for practitioners. Such architectural design and abstractions enable researchers and developers to extend the toolkit with their new algorithms and improvements, and to use it for performance benchmarking. A built-in testing infrastructure maintains code quality.},
517 url = {http://arxiv.org/abs/1810.01943},
518 shorttitle = {{AI} {Fairness} 360},
519 title = {{AI} {Fairness} 360: {An} {Extensible} {Toolkit} for {Detecting}, {Understanding}, and {Mitigating} {Unwanted} {Algorithmic} {Bias}},
520}
521
522@article{adadi_peeking_2018,
523 file = {IEEE Xplore Abstract Record:C\:\\Users\\dssch\\Zotero\\storage\\WN6LP8XN\\8466590.html:text/html;IEEE Xplore Full Text PDF:C\:\\Users\\dssch\\Zotero\\storage\\URY75FGV\\Adadi and Berrada - 2018 - Peeking Inside the Black-Box A Survey on Explaina.pdf:application/pdf},
524 pages = {52138--52160},
525 keywords = {artificial intelligence, fourth industrial revolution, Machine learning, interpretable machine learning, AI-based systems, Biological system modeling, black-box models, black-box nature, Conferences, explainable AI, explainable artificial intelligence, Explainable artificial intelligence, Machine learning algorithms, Market research, Prediction algorithms, XAI},
526 note = {Conference Name: IEEE Access},
527 year = {2018},
528 author = {Adadi, Amina and Berrada, Mohammed},
529 journal = {IEEE Access},
530 abstract = {At the dawn of the fourth industrial revolution, we are witnessing a fast and widespread adoption of artificial intelligence (AI) in our daily life, which contributes to accelerating the shift towards a more algorithmic society. However, even with such unprecedented advancements, a key impediment to the use of AI-based systems is that they often lack transparency. Indeed, the black-box nature of these systems allows powerful predictions, but it cannot be directly explained. This issue has triggered a new debate on explainable AI (XAI). A research field holds substantial promise for improving trust and transparency of AI-based systems. It is recognized as the sine qua non for AI to continue making steady progress without disruption. This survey provides an entry point for interested researchers and practitioners to learn key aspects of the young and rapidly growing body of research related to XAI. Through the lens of the literature, we review the existing approaches regarding the topic, discuss trends surrounding its sphere, and present major research trajectories.},
531 doi = {10.1109/ACCESS.2018.2870052},
532 shorttitle = {Peeking {Inside} the {Black}-{Box}},
533 issn = {2169-3536},
534 volume = {6},
535 title = {Peeking {Inside} the {Black}-{Box}: {A} {Survey} on {Explainable} {Artificial} {Intelligence} ({XAI})},
536}
537
538@article{bagloee_autonomous_2016,
539 file = {Springer Full Text PDF:C\:\\Users\\dssch\\Zotero\\storage\\GT73JTX5\\Bagloee et al. - 2016 - Autonomous vehicles challenges, opportunities, an.pdf:application/pdf},
540 pages = {284--303},
541 year = {2016},
542 month = {December},
543 author = {Bagloee, Saeed Asadi and Tavana, Madjid and Asadi, Mohsen and Oliver, Tracey},
544 journal = {Journal of Modern Transportation},
545 urldate = {2020-03-04},
546 number = {4},
547 language = {en},
548 abstract = {This study investigates the challenges and opportunities pertaining to transportation policies that may arise as a result of emerging autonomous vehicle (AV) technologies. AV technologies can decrease the transportation cost and increase accessibility to low-income households and persons with mobility issues. This emerging technology also has far-reaching applications and implications beyond all current expectations. This paper provides a comprehensive review of the relevant literature and explores a broad spectrum of issues from safety to machine ethics. An indispensable part of a prospective AV development is communication over cars and infrastructure (connected vehicles). A major knowledge gap exists in AV technology with respect to routing behaviors. Connected-vehicle technology provides a great opportunity to implement an efficient and intelligent routing system. To this end, we propose a conceptual navigation model based on a fleet of AVs that are centrally dispatched over a network seeking system optimization. This study contributes to the literature on two fronts: (i) it attempts to shed light on future opportunities as well as possible hurdles associated with AV technology; and (ii) it conceptualizes a navigation model for the AV which leads to highly efficient traffic circulations.},
549 doi = {10.1007/s40534-016-0117-3},
550 url = {https://doi.org/10.1007/s40534-016-0117-3},
551 shorttitle = {Autonomous vehicles},
552 issn = {2196-0577},
553 volume = {24},
554 title = {Autonomous vehicles: challenges, opportunities, and future implications for transportation policies},
555}
556
557@article{difranzo_filter_2017,
558 file = {Full Text PDF:C\:\\Users\\dssch\\Zotero\\storage\\N55A3QB2\\DiFranzo and Gloria-Garcia - 2017 - Filter bubbles and fake news.pdf:application/pdf},
559 pages = {32--35},
560 year = {2017},
561 month = {April},
562 author = {DiFranzo, Dominic and Gloria-Garcia, Kristine},
563 journal = {XRDS: Crossroads, The ACM Magazine for Students},
564 urldate = {2020-03-04},
565 number = {3},
566 abstract = {The results of the 2016 Brexit referendum in the U.K. and presidential election in the U.S. surprised pollsters and traditional media alike, and social media is now being blamed in part for creating echo chambers that encouraged the spread of fake news that influenced voters.},
567 doi = {10.1145/3055153},
568 url = {https://doi.org/10.1145/3055153},
569 issn = {1528-4972},
570 volume = {23},
571 title = {Filter bubbles and fake news},
572}
573
574@inproceedings{garrett_more_2020,
575 file = {Garrett et al. - 2020 - More Than If Time Allows The Role of Ethics in .pdf:C\:\\Users\\dssch\\Zotero\\storage\\3AEQA2K3\\Garrett et al. - 2020 - More Than If Time Allows The Role of Ethics in .pdf:application/pdf},
576 pages = {272--278},
577 year = {2020},
578 month = {February},
579 author = {Garrett, Natalie and Beard, Nathan and Fiesler, Casey},
580 publisher = {ACM},
581 booktitle = {Proceedings of the {AAAI}/{ACM} {Conference} on {AI}, {Ethics}, and {Society}},
582 urldate = {2020-03-04},
583 language = {en},
584 abstract = {Even as public pressure mounts for technology companies to consider societal impacts of products, industries and governments in the AI race are demanding technical talent. To meet this demand, universities clamor to add technical artificial intelligence (AI) and machine learning (ML) courses into computing curriculum—but how are societal and ethical considerations part of this landscape? We explore two pathways for ethics content in AI education: (1) standalone AI ethics courses, and (2) integrating ethics into technical AI courses. For both pathways, we ask: What is being taught? As we train computer scientists who will build and deploy AI tools, how are we training them to consider the consequences of their work? In this exploratory work, we qualitatively analyzed 31 standalone AI ethics classes from 22 U.S. universities and 20 AI/ML technical courses from 12 U.S. universities to understand which ethics-related topics instructors include in courses. We identify and categorize topics in AI ethics education, share notable practices, and note omissions. Our analysis will help AI educators identify what topics should be taught and create scaffolding for developing future AI ethics education.},
585 doi = {10.1145/3375627.3375868},
586 url = {http://dl.acm.org/doi/10.1145/3375627.3375868},
587 shorttitle = {More {Than} "{If} {Time} {Allows}"},
588 isbn = {978-1-4503-7110-0},
589 title = {More {Than} "{If} {Time} {Allows}": {The} {Role} of {Ethics} in {AI} {Education}},
590 address = {New York NY USA},
591}
592
593@article{saltz_integrating_2019,
594 pages = {32:1--32:26},
595 keywords = {education, Machine learning, ethics},
596 year = {2019},
597 month = {August},
598 author = {Saltz, Jeffrey and Skirpan, Michael and Fiesler, Casey and Gorelick, Micha and Yeh, Tom and Heckman, Robert and Dewar, Neil and Beard, Nathan},
599 journal = {ACM Transactions on Computing Education},
600 urldate = {2020-03-04},
601 number = {4},
602 abstract = {This article establishes and addresses opportunities for ethics integration into Machine-learning (ML) courses. Following a survey of the history of computing ethics and the current need for ethical consideration within ML, we consider the current state of ML ethics education via an exploratory analysis of course syllabi in computing programs. The results reveal that though ethics is part of the overall educational landscape in these programs, it is not frequently a part of core technical ML courses. To help address this gap, we offer a preliminary framework, developed via a systematic literature review, of relevant ethics questions that should be addressed within an ML project. A pilot study with 85 students confirms that this framework helped them identify and articulate key ethical considerations within their ML projects. Building from this work, we also provide three example ML course modules that bring ethical thinking directly into learning core ML content. Collectively, this research demonstrates: (1) the need for ethics to be taught as integrated within ML coursework, (2) a structured set of questions useful for identifying and addressing potential issues within an ML project, and (3) novel course models that provide examples for how to practically teach ML ethics without sacrificing core course content. An additional by-product of this research is the collection and integration of recent publications in the emerging field of ML ethics education.},
603 doi = {10.1145/3341164},
604 url = {https://doi.org/10.1145/3341164},
605 volume = {19},
606 title = {Integrating {Ethics} within {Machine} {Learning} {Courses}},
607}
608
609@article{dignum_ethics_2018,
610 file = {Springer Full Text PDF:C\:\\Users\\dssch\\Zotero\\storage\\SV3VZ7GZ\\Dignum - 2018 - Ethics in artificial intelligence introduction to.pdf:application/pdf},
611 pages = {1--3},
612 year = {2018},
613 month = {March},
614 author = {Dignum, Virginia},
615 journal = {Ethics and Information Technology},
616 urldate = {2020-03-04},
617 number = {1},
618 language = {en},
619 doi = {10.1007/s10676-018-9450-z},
620 url = {https://doi.org/10.1007/s10676-018-9450-z},
621 shorttitle = {Ethics in artificial intelligence},
622 issn = {1572-8439},
623 volume = {20},
624 title = {Ethics in artificial intelligence: introduction to the special issue},
625}
626
627@article{shane_business_2018,
628 keywords = {Artificial Intelligence, Drones (Pilotless Planes), Defense Contracts, Google Inc, Pichai, Sundar, United States Defense and Military Forces},
629 year = {2018},
630 month = {April},
631 author = {Shane, Scott and Wakabayashi, Daisuke},
632 journal = {The New York Times},
633 urldate = {2020-03-09},
634 language = {en-US},
635 abstract = {Thousands of employees have signed a letter calling on their C.E.O. to pull out of a project that could be used to improve drone strike targeting.},
636 url = {https://www.nytimes.com/2018/04/04/technology/google-letter-ceo-pentagon-project.html},
637 shorttitle = {‘{The} {Business} of {War}’},
638 issn = {0362-4331},
639 title = {‘{The} {Business} of {War}’: {Google} {Employees} {Protest} {Work} for the {Pentagon}},
640 chapter = {Technology},
641}
642
643@article{oswald_algorithmic_2018,
644 file = {Full Text PDF:C\:\\Users\\dssch\\Zotero\\storage\\2WJEK4RN\\Oswald et al. - 2018 - Algorithmic risk assessment policing models lesso.pdf:application/pdf;Snapshot:C\:\\Users\\dssch\\Zotero\\storage\\T7S2YHNI\\13600834.2018.html:text/html},
645 pages = {223--250},
646 keywords = {predictions, Algorithms, law, criminal justice, proportionality, risk assessment},
647 note = {Publisher: Routledge
648\_eprint: https://doi.org/10.1080/13600834.2018.1458455},
649 year = {2018},
650 month = {May},
651 author = {Oswald, Marion and Grace, Jamie and Urwin, Sheena and Barnes, Geoffrey C.},
652 journal = {Information \& Communications Technology Law},
653 urldate = {2020-03-10},
654 number = {2},
655 abstract = {As is common across the public sector, the UK police service is under pressure to do more with less, to target resources more efficiently and take steps to identify threats proactively; for example under risk-assessment schemes such as ‘Clare’s Law’ and ‘Sarah’s Law’. Algorithmic tools promise to improve a police force’s decision-making and prediction abilities by making better use of data (including intelligence), both from inside and outside the force. This article uses Durham Constabulary’s Harm Assessment Risk Tool (HART) as a case-study. HART is one of the first algorithmic models to be deployed by a UK police force in an operational capacity. Our article comments upon the potential benefits of such tools, explains the concept and method of HART and considers the results of the first validation of the model’s use and accuracy. The article then critiques the use of algorithmic tools within policing from a societal and legal perspective, focusing in particular upon substantive common law grounds for judicial review. It considers a concept of ‘experimental’ proportionality to permit the use of unproven algorithms in the public sector in a controlled and time-limited way, and as part of a combination of approaches to combat algorithmic opacity, proposes ‘ALGO-CARE’, a guidance framework of some of the key legal and practical concerns that should be considered in relation to the use of algorithmic risk assessment tools by the police. The article concludes that for the use of algorithmic tools in a policing context to result in a ‘better’ outcome, that is to say, a more efficient use of police resources in a landscape of more consistent, evidence-based decision-making, then an ‘experimental’ proportionality approach should be developed to ensure that new solutions from ‘big data’ can be found for criminal justice problems traditionally arising from clouded, non-augmented decision-making. Finally, this article notes that there is a sub-set of decisions around which there is too great an impact upon society and upon the welfare of individuals for them to be influenced by an emerging technology; to an extent, in fact, that they should be removed from the influence of algorithmic decision-making altogether.},
656 doi = {10.1080/13600834.2018.1458455},
657 url = {https://doi.org/10.1080/13600834.2018.1458455},
658 shorttitle = {Algorithmic risk assessment policing models},
659 issn = {1360-0834},
660 volume = {27},
661 title = {Algorithmic risk assessment policing models: lessons from the {Durham} {HART} model and ‘{Experimental}’ proportionality},
662}
663
664@techreport{reisman_algorithmic_2018,
665 pages = {22},
666 year = {2018},
667 month = {April},
668 author = {Reisman, Dillon and Schultz, Jason and Crawford, Kate and Whittaker, Meredith},
669 institution = {AI Now},
670 url = {https://ainowinstitute.org/aiareport2018.pdf},
671 title = {Algorithmic {Impact} {Assessments}: {A} {Practical} {Framework} for {Public} {Agency} {Accountability}},
672 address = {New York, NY},
673}
674
675@techreport{women_leading_in_ai_women_2019,
676 pages = {28},
677 year = {2019},
678 month = {January},
679 author = {{Women Leading in AI}},
680 institution = {Women Leading in AI},
681 url = {https://womenleadinginai.org/wp-content/uploads/2019/02/WLiAI-Report-2019.pdf},
682 title = {Women {Leading} in {AI}: 10 {Principles} of {Responsible} {AI}},
683}
684
685@techreport{latonero_governing_2018,
686 pages = {38},
687 year = {2018},
688 month = {October},
689 author = {Latonero, Mark},
690 institution = {Data\&Society},
691 url = {https://datasociety.net/pubs/governing_ai.pdf},
692 title = {Governing {Artificial} {Intelligence}: {Upholding} {Human} {Rights} \& {Dignity}},
693 address = {New York, NY},
694}
695
696@inproceedings{bietti_ethics_2020,
697 file = {Full Text PDF:C\:\\Users\\dssch\\Zotero\\storage\\F7UGRDLW\\Bietti - 2020 - From ethics washing to ethics bashing a view on t.pdf:application/pdf},
698 pages = {210--219},
699 keywords = {AI, ethics, regulation, moral philosophy, self-regulation, technology ethics, technology law},
700 year = {2020},
701 month = {January},
702 author = {Bietti, Elettra},
703 publisher = {Association for Computing Machinery},
704 booktitle = {Proceedings of the 2020 {Conference} on {Fairness}, {Accountability}, and {Transparency}},
705 urldate = {2020-03-10},
706 abstract = {The word 'ethics' is under siege in technology policy circles. Weaponized in support of deregulation, self-regulation or handsoff governance, "ethics" is increasingly identified with technology companies' self-regulatory efforts and with shallow appearances of ethical behavior. So-called "ethics washing" by tech companies is on the rise, prompting criticism and scrutiny from scholars and the tech community at large. In parallel to the growth of ethics washing, its condemnation has led to a tendency to engage in "ethics bashing." This consists in the trivialization of ethics and moral philosophy now understood as discrete tools or pre-formed social structures such as ethics boards, self-governance schemes or stakeholder groups. The misunderstandings underlying ethics bashing are at least threefold: (a) philosophy and "ethics" are seen as a communications strategy and as a form of instrumentalized cover-up or façade for unethical behavior, (b) philosophy is understood in opposition and as alternative to political representation and social organizing and (c) the role and importance of moral philosophy is downplayed and portrayed as mere "ivory tower" intellectualization of complex problems that need to be dealt with in practice. This paper argues that the rhetoric of ethics and morality should not be reductively instrumentalized, either by the industry in the form of "ethics washing," or by scholars and policy-makers in the form of "ethics bashing." Grappling with the role of philosophy and ethics requires moving beyond both tendencies and seeing ethics as a mode of inquiry that facilitates the evaluation of competing tech policy strategies. In other words, we must resist narrow reductivism of moral philosophy as instrumentalized performance and renew our faith in its intrinsic moral value as a mode of knowledgeseeking and inquiry. Far from mandating a self-regulatory scheme or a given governance structure, moral philosophy in fact facilitates the questioning and reconsideration of any given practice, situating it within a complex web of legal, political and economic institutions. Moral philosophy indeed can shed new light on human practices by adding needed perspective, explaining the relationship between technology and other worthy goals, situating technology within the human, the social, the political. It has become urgent to start considering technology ethics also from within and not only from outside of ethics.},
707 doi = {10.1145/3351095.3372860},
708 url = {https://doi.org/10.1145/3351095.3372860},
709 shorttitle = {From ethics washing to ethics bashing},
710 isbn = {978-1-4503-6936-7},
711 title = {From ethics washing to ethics bashing: a view on tech ethics from within moral philosophy},
712 series = {{FAT}* '20},
713 address = {Barcelona, Spain},
714}
715
716@inproceedings{raji_closing_2020,
717 file = {Full Text PDF:C\:\\Users\\dssch\\Zotero\\storage\\3ECGJJTV\\Raji et al. - 2020 - Closing the AI accountability gap defining an end.pdf:application/pdf},
718 pages = {33--44},
719 keywords = {machine learning, responsible innovation, accountability, algorithmic audits},
720 year = {2020},
721 month = {January},
722 author = {Raji, Inioluwa Deborah and Smart, Andrew and White, Rebecca N. and Mitchell, Margaret and Gebru, Timnit and Hutchinson, Ben and Smith-Loud, Jamila and Theron, Daniel and Barnes, Parker},
723 publisher = {Association for Computing Machinery},
724 booktitle = {Proceedings of the 2020 {Conference} on {Fairness}, {Accountability}, and {Transparency}},
725 urldate = {2020-03-10},
726 abstract = {Rising concern for the societal implications of artificial intelligence systems has inspired a wave of academic and journalistic literature in which deployed systems are audited for harm by investigators from outside the organizations deploying the algorithms. However, it remains challenging for practitioners to identify the harmful repercussions of their own systems prior to deployment, and, once deployed, emergent issues can become difficult or impossible to trace back to their source. In this paper, we introduce a framework for algorithmic auditing that supports artificial intelligence system development end-to-end, to be applied throughout the internal organization development life-cycle. Each stage of the audit yields a set of documents that together form an overall audit report, drawing on an organization's values or principles to assess the fit of decisions made throughout the process. The proposed auditing framework is intended to contribute to closing the accountability gap in the development and deployment of large-scale artificial intelligence systems by embedding a robust process to ensure audit integrity.},
727 doi = {10.1145/3351095.3372873},
728 url = {https://doi.org/10.1145/3351095.3372873},
729 shorttitle = {Closing the {AI} accountability gap},
730 isbn = {978-1-4503-6936-7},
731 title = {Closing the {AI} accountability gap: defining an end-to-end framework for internal algorithmic auditing},
732 series = {{FAT}* '20},
733 address = {Barcelona, Spain},
734}
735
736@inproceedings{madaio_co-designing_2020,
737 file = {Full Text PDF:C\:\\Users\\dssch\\Zotero\\storage\\U4QUDK9J\\Madaio and Vaughan - 2020 - Co-Designing Checklists to Understand Organization.pdf:application/pdf},
738 year = {2020},
739 author = {Madaio, Michael A. and Vaughan, Jennifer Wortman},
740 abstract = {Many organizations have published principles intended to guide the ethical development and deployment of AI systems; however, their abstract nature makes them difficult to operationalize. Some organizations have therefore produced AI ethics checklists, as well as checklists for more specific concepts, such as fairness, as applied to AI systems. But unless checklists are grounded in practitioners’ needs, they may be misused. To understand the role of checklists in AI ethics, we conducted an iterative co-design process with 48 practitioners, focusing on fairness. We co-designed an AI fairness checklist and identified desiderata and concerns for AI fairness checklists in general. We found that AI fairness checklists could provide organizational infrastructure for formalizing ad-hoc processes and empowering individual advocates. We discuss aspects of organizational culture that may impact the efficacy of such checklists, and highlight future research directions.},
741 title = {Co-{Designing} {Checklists} to {Understand} {Organizational} {Challenges} and {Opportunities} around {Fairness} in {AI}},
742}
743
744@article{metcalf_owning_2019,
745 file = {Full Text PDF:C\:\\Users\\dssch\\Zotero\\storage\\K6ZPH856\\Metcalf et al. - 2019 - Owning Ethics Corporate Logics, Silicon Valley, a.pdf:application/pdf},
746 pages = {449--476},
747 note = {Publisher: Johns Hopkins University Press},
748 year = {2019},
749 month = {August},
750 author = {Metcalf, Jacob and Moss, Emanuel and Boyd, Danah},
751 journal = {Social Research: An International Quarterly},
752 urldate = {2020-03-13},
753 number = {2},
754 language = {en},
755 url = {https://muse.jhu.edu/article/732185},
756 shorttitle = {Owning {Ethics}},
757 issn = {1944-768X},
758 volume = {86},
759 title = {Owning {Ethics}: {Corporate} {Logics}, {Silicon} {Valley}, and the {Institutionalization} of {Ethics}},
760}
761
762@article{calvo_advancing_2020,
763 file = {Snapshot:C\:\\Users\\dssch\\Zotero\\storage\\IHDA9G5B\\s42256-020-0151-z.html:text/html},
764 pages = {89--91},
765 note = {Number: 2
766Publisher: Nature Publishing Group},
767 year = {2020},
768 month = {February},
769 author = {Calvo, Rafael A. and Peters, Dorian and Cave, Stephen},
770 journal = {Nature Machine Intelligence},
771 urldate = {2020-03-13},
772 number = {2},
773 language = {en},
774 abstract = {With the rise of AI technologies in society, we need a human impact assessment for technology.},
775 doi = {10.1038/s42256-020-0151-z},
776 url = {https://www.nature.com/articles/s42256-020-0151-z},
777 issn = {2522-5839},
778 copyright = {2020 Springer Nature Limited},
779 volume = {2},
780 title = {Advancing impact assessment for intelligent systems},
781}
782
783@article{nanni_give_2020,
784 file = {arXiv Fulltext PDF:C\:\\Users\\dssch\\Zotero\\storage\\SXCHUB93\\Nanni et al. - 2020 - Give more data, awareness and control to individua.pdf:application/pdf;arXiv.org Snapshot:C\:\\Users\\dssch\\Zotero\\storage\\ZYQPKQKP\\2004.html:text/html},
785 keywords = {Computer Science - Computers and Society, Computer Science - Social and Information Networks},
786 note = {arXiv: 2004.05222
787version: 1},
788 year = {2020},
789 month = {April},
790 author = {Nanni, Mirco and Andrienko, Gennady and Boldrini, Chiara and Bonchi, Francesco and Cattuto, Ciro and Chiaromonte, Francesca and Comandé, Giovanni and Conti, Marco and Coté, Mark and Dignum, Frank and Dignum, Virginia and Domingo-Ferrer, Josep and Giannotti, Fosca and Guidotti, Riccardo and Helbing, Dirk and Kertesz, Janos and Lehmann, Sune and Lepri, Bruno and Lukowicz, Paul and Monreale, Anna and Morik, Katharina and Oliver, Nuria and Passarella, Andrea and Passerini, Andrea and Pedreschi, Dino and Pentland, Alex and Pratesi, Francesca and Rinzivillo, Salvatore and Ruggieri, Salvatore and Siebes, Arno and Trasarti, Roberto and Hoven, Jeroen van den and Vespignani, Alessandro},
791 journal = {arXiv:2004.05222 [cs]},
792 urldate = {2020-04-17},
793 abstract = {The rapid dynamics of COVID-19 calls for quick and effective tracking of virus transmission chains and early detection of outbreaks, especially in the phase 2 of the pandemic, when lockdown and other restriction measures are progressively withdrawn, in order to avoid or minimize contagion resurgence. For this purpose, contact-tracing apps are being proposed for large scale adoption by many countries. A centralized approach, where data sensed by the app are all sent to a nation-wide server, raises concerns about citizens' privacy and needlessly strong digital surveillance, thus alerting us to the need to minimize personal data collection and avoiding location tracking. We advocate the conceptual advantage of a decentralized approach, where both contact and location data are collected exclusively in individual citizens' "personal data stores", to be shared separately and selectively, voluntarily, only when the citizen has tested positive for COVID-19, and with a privacy preserving level of granularity. This approach better protects the personal sphere of citizens and affords multiple benefits: it allows for detailed information gathering for infected people in a privacy-preserving fashion; and, in turn this enables both contact tracing, and, the early detection of outbreak hotspots on more finely-granulated geographic scale. Our recommendation is two-fold. First to extend existing decentralized architectures with a light touch, in order to manage the collection of location data locally on the device, and allow the user to share spatio-temporal aggregates - if and when they want, for specific aims - with health authorities, for instance. Second, we favour a longer-term pursuit of realizing a Personal Data Store vision, giving users the opportunity to contribute to collective good in the measure they want, enhancing self-awareness, and cultivating collective efforts for rebuilding society.},
794 url = {http://arxiv.org/abs/2004.05222},
795 title = {Give more data, awareness and control to individual citizens, and they will help {COVID}-19 containment},
796}
797
798@article{brundage_toward_2020,
799 file = {arXiv Fulltext PDF:C\:\\Users\\dssch\\Zotero\\storage\\KIHU4F64\\Brundage et al. - 2020 - Toward Trustworthy AI Development Mechanisms for .pdf:application/pdf;arXiv.org Snapshot:C\:\\Users\\dssch\\Zotero\\storage\\KX7IPUS8\\2004.html:text/html},
800 keywords = {Computer Science - Computers and Society},
801 note = {arXiv: 2004.07213},
802 year = {2020},
803 month = {April},
804 author = {Brundage, Miles and Avin, Shahar and Wang, Jasmine and Belfield, Haydn and Krueger, Gretchen and Hadfield, Gillian and Khlaaf, Heidy and Yang, Jingying and Toner, Helen and Fong, Ruth and Maharaj, Tegan and Koh, Pang Wei and Hooker, Sara and Leung, Jade and Trask, Andrew and Bluemke, Emma and Lebensold, Jonathan and O'Keefe, Cullen and Koren, Mark and Ryffel, Théo and Rubinovitz, J. B. and Besiroglu, Tamay and Carugati, Federica and Clark, Jack and Eckersley, Peter and de Haas, Sarah and Johnson, Maritza and Laurie, Ben and Ingerman, Alex and Krawczuk, Igor and Askell, Amanda and Cammarota, Rosario and Lohn, Andrew and Krueger, David and Stix, Charlotte and Henderson, Peter and Graham, Logan and Prunkl, Carina and Martin, Bianca and Seger, Elizabeth and Zilberman, Noa and hÉigeartaigh, Seán Ó and Kroeger, Frens and Sastry, Girish and Kagan, Rebecca and Weller, Adrian and Tse, Brian and Barnes, Elizabeth and Dafoe, Allan and Scharre, Paul and Herbert-Voss, Ariel and Rasser, Martijn and Sodhani, Shagun and Flynn, Carrick and Gilbert, Thomas Krendl and Dyer, Lisa and Khan, Saif and Bengio, Yoshua and Anderljung, Markus},
805 journal = {arXiv:2004.07213 [cs]},
806 urldate = {2020-04-26},
807 abstract = {With the recent wave of progress in artificial intelligence (AI) has come a growing awareness of the large-scale impacts of AI systems, and recognition that existing regulations and norms in industry and academia are insufficient to ensure responsible AI development. In order for AI developers to earn trust from system users, customers, civil society, governments, and other stakeholders that they are building AI responsibly, they will need to make verifiable claims to which they can be held accountable. Those outside of a given organization also need effective means of scrutinizing such claims. This report suggests various steps that different stakeholders can take to improve the verifiability of claims made about AI systems and their associated development processes, with a focus on providing evidence about the safety, security, fairness, and privacy protection of AI systems. We analyze ten mechanisms for this purpose--spanning institutions, software, and hardware--and make recommendations aimed at implementing, exploring, or improving those mechanisms.},
808 url = {http://arxiv.org/abs/2004.07213},
809 shorttitle = {Toward {Trustworthy} {AI} {Development}},
810 title = {Toward {Trustworthy} {AI} {Development}: {Mechanisms} for {Supporting} {Verifiable} {Claims}},
811}
812
813@article{thomas_reliance_nodate,
814 file = {Thomas and Uminsky - Reliance on Metrics is a Fundamental Challenge for.pdf:C\:\\Users\\dssch\\Zotero\\storage\\BJ84SRIB\\Thomas and Uminsky - Reliance on Metrics is a Fundamental Challenge for.pdf:application/pdf},
815 pages = {7},
816 author = {Thomas, Rachel and Uminsky, David},
817 language = {en},
818 abstract = {Optimizing a given metric is a central aspect of most current AI approaches, yet overemphasizing metrics leads to manipulation, gaming, a myopic focus on short-term goals, and other unexpected negative consequences. This poses a fundamental challenge in the use and development of AI. We first review how metrics can go wrong in practice and aspects of how our online environment and current business practices are exacerbating these failures. We put forward here an evidence based framework that takes steps toward mitigating the harms caused by overemphasis of metrics within AI by: (1) using a slate of metrics to get a fuller and more nuanced picture, (2) combining metrics with qualitative accounts, and (3) involving a range of stakeholders, including those who will be most impacted.},
819 title = {Reliance on {Metrics} is a {Fundamental} {Challenge} for {AI}},
820}
821
822@article{katell_algorithmic_2019,
823 file = {arXiv Fulltext PDF:C\:\\Users\\dssch\\Zotero\\storage\\33G3MH3Q\\Katell et al. - 2019 - An Algorithmic Equity Toolkit for Technology Audit.pdf:application/pdf;arXiv.org Snapshot:C\:\\Users\\dssch\\Zotero\\storage\\JLPZAW6M\\1912.html:text/html},
824 keywords = {Computer Science - Computers and Society, Computer Science - Artificial Intelligence, Computer Science - Machine Learning, Computer Science - Social and Information Networks, Computer Science - Human-Computer Interaction},
825 note = {arXiv: 1912.02943},
826 year = {2019},
827 month = {December},
828 author = {Katell, Michael and Young, Meg and Herman, Bernease and Dailey, Dharma and Tam, Aaron and Guetler, Vivian and Binz, Corinne and Raz, Daniella and Krafft, P. M.},
829 journal = {arXiv:1912.02943 [cs]},
830 urldate = {2020-04-26},
831 abstract = {A wave of recent scholarship documenting the discriminatory harms of algorithmic systems has spurred widespread interest in algorithmic accountability and regulation. Yet effective accountability and regulation is stymied by a persistent lack of resources supporting public understanding of algorithms and artificial intelligence. Through interactions with a US-based civil rights organization and their coalition of community organizations, we identify a need for (i) heuristics that aid stakeholders in distinguishing between types of analytic and information systems in lay language, and (ii) risk assessment tools for such systems that begin by making algorithms more legible. The present work delivers a toolkit to achieve these aims. This paper both presents the Algorithmic Equity Toolkit (AEKit) Equity as an artifact, and details how our participatory process shaped its design. Our work fits within human-computer interaction scholarship as a demonstration of the value of HCI methods and approaches to problems in the area of algorithmic transparency and accountability.},
832 url = {http://arxiv.org/abs/1912.02943},
833 title = {An {Algorithmic} {Equity} {Toolkit} for {Technology} {Audits} by {Community} {Advocates} and {Activists}},
834}
835
836@article{wearn_responsible_2019,
837 file = {Snapshot:C\:\\Users\\dssch\\Zotero\\storage\\H5DYWZY4\\s42256-019-0022-7.html:text/html;Submitted Version:C\:\\Users\\dssch\\Zotero\\storage\\8XGRAHDP\\Wearn et al. - 2019 - Responsible AI for conservation.pdf:application/pdf},
838 pages = {72--73},
839 note = {Number: 2
840Publisher: Nature Publishing Group},
841 year = {2019},
842 month = {February},
843 author = {Wearn, Oliver R. and Freeman, Robin and Jacoby, David M. P.},
844 journal = {Nature Machine Intelligence},
845 urldate = {2020-04-27},
846 number = {2},
847 language = {en},
848 abstract = {Artificial intelligence (AI) promises to be an invaluable tool for nature conservation, but its misuse could have severe real-world consequences for people and wildlife. Conservation scientists discuss how improved metrics and ethical oversight can mitigate these risks.},
849 doi = {10.1038/s42256-019-0022-7},
850 url = {https://www.nature.com/articles/s42256-019-0022-7},
851 issn = {2522-5839},
852 copyright = {2019 Springer Nature Limited},
853 volume = {1},
854 title = {Responsible {AI} for conservation},
855}
856
857@techreport{cowls_designing_2019,
858 file = {Snapshot:C\:\\Users\\dssch\\Zotero\\storage\\N5ASE3JK\\papers.html:text/html;Submitted Version:C\:\\Users\\dssch\\Zotero\\storage\\KX3TZ2LQ\\Cowls et al. - 2019 - Designing AI for Social Good Seven Essential Fact.pdf:application/pdf},
859 keywords = {AI4SG, Artificial Intelligence, Ethics, Privacy, Safety, Social Good, Sustainable Development Goals},
860 doi = {10.2139/ssrn.3388669},
861 year = {2019},
862 month = {May},
863 author = {Cowls, Josh and King, Thomas and Taddeo, Mariarosaria and Floridi, Luciano},
864 institution = {Social Science Research Network},
865 urldate = {2020-04-27},
866 number = {ID 3388669},
867 language = {en},
868 abstract = {The idea of Artificial Intelligence for Social Good (henceforth AI4SG) is gaining traction within information societies in general and the AI community in particular. It has the potential to address social problems effectively through the development of AI-based solutions. Yet, to date, there is only limited understanding of what makes AI socially good in theory, what counts as AI4SG in practice, and how to reproduce its initial successes in terms of policies (Cath et al. 2018). This article addresses this gap by extrapolating seven ethical factors that are essential for future AI4SG initiatives from the analysis of 27 case studies of AI4SG projects. Some of these factors are almost entirely novel to AI, while the significance of other factors is heightened by the use of AI. From each of these factors, corresponding best practices are formulated which, subject to context and balance, may serve as preliminary guidelines to ensure that well-designed AI is more likely to serve the social good.},
869 url = {https://papers.ssrn.com/abstract=3388669},
870 shorttitle = {Designing {AI} for {Social} {Good}},
871 title = {Designing {AI} for {Social} {Good}: {Seven} {Essential} {Factors}},
872 type = {{SSRN} {Scholarly} {Paper}},
873 address = {Rochester, NY},
874}
875
876@article{joppa_case_2017,
877 file = {Full Text:C\:\\Users\\dssch\\Zotero\\storage\\ASWQJ9W2\\Joppa - 2017 - The case for technology investments in the environ.pdf:application/pdf;Snapshot:C\:\\Users\\dssch\\Zotero\\storage\\6NVBDZ99\\d41586-017-08675-7.html:text/html},
878 pages = {325--328},
879 note = {Number: 7685
880Publisher: Nature Publishing Group},
881 year = {2017},
882 month = {December},
883 author = {Joppa, Lucas N.},
884 journal = {Nature},
885 urldate = {2020-04-27},
886 number = {7685},
887 language = {en},
888 abstract = {Create an artificial-intelligence platform for the planet, urges Lucas N. Joppa.},
889 doi = {10.1038/d41586-017-08675-7},
890 url = {https://www.nature.com/articles/d41586-017-08675-7},
891 copyright = {2020 Nature},
892 volume = {552},
893 title = {The case for technology investments in the environment},
894}
895
896@inproceedings{abebe_roles_2020,
897 collection = {FAT* ’20},
898 pages = {252–260},
899 month = {Jan},
900 year = {2020},
901 author = {Abebe, Rediet and Barocas, Solon and Kleinberg, Jon and Levy, Karen and Raghavan, Manish and Robinson, David G.},
902 publisher = {Association for Computing Machinery},
903 booktitle = {Proceedings of the 2020 Conference on Fairness, Accountability, and Transparency},
904 abstractnote = {A recent normative turn in computer science has brought concerns about fairness, bias, and accountability to the core of the field. Yet recent scholarship has warned that much of this technical work treats problematic features of the status quo as fixed, and fails to address deeper patterns of injustice and inequality. While acknowledging these critiques, we posit that computational research has valuable roles to play in addressing social problems --- roles whose value can be recognized even from a perspective that aspires toward fundamental social change. In this paper, we articulate four such roles, through an analysis that considers the opportunities as well as the significant risks inherent in such work. Computing research can serve as a diagnostic, helping us to understand and measure social problems with precision and clarity. As a formalizer, computing shapes how social problems are explicitly defined --- changing how those problems, and possible responses to them, are understood. Computing serves as rebuttal when it illuminates the boundaries of what is possible through technical means. And computing acts as synecdoche when it makes long-standing social problems newly salient in the public eye. We offer these paths forward as modalities that leverage the particular strengths of computational work in the service of social change, without overclaiming computing’s capacity to solve social problems on its own.},
905 doi = {10.1145/3351095.3372871},
906 url = {https://doi.org/10.1145/3351095.3372871},
907 isbn = {978-1-4503-6936-7},
908 title = {Roles for computing in social change},
909 series = {FAT* ’20},
910 place = {Barcelona, Spain},
911}
912
913@article{winner_artifacts_1980,
914 pages = {121–136},
915 year = {1980},
916 author = {Winner, Langdon},
917 journal = {Daedalus},
918 title = {Do artifacts have politics?},
919}
920
921@misc{canada_2019,
922 month = {May},
923 year = {2019},
924 author = {Government of Canada},
925 note = {Last Modified: 2019-05-31},
926 url = {https://www.canada.ca/en/government/system/digital-government/modern-emerging-technologies/responsible-use-ai/algorithmic-impact-assessment.html},
927 title = {Algorithmic Impact Assessment (AIA)},
928}
929
930@book{Schuler_Namioka_1993,
931 month = {Mar},
932 year = {1993},
933 author = {Schuler, Douglas and Namioka, Aki},
934 publisher = {CRC Press},
935 note = {Google-Books-ID: pWOEk6Sk4YkC},
936 abstractnote = {The voices in this collection are primarily those of researchers and developers concerned with bringing knowledge of technological possibilities to bear on informed and effective system design. Their efforts are distinguished from many previous writings on system development by their central and abiding reliance on direct and continuous interaction with those who are the ultimate arbiters of system adequacy; namely, those who will use the technology in their everyday lives and work. A key issue throughout is the question of who does what to whom: whose interests are at stake, who initiates action and for what reason, who defines the problem and who decides that there is one. The papers presented follow in the footsteps of a small but growing international community of scholars and practitioners of participatory systems design. Many of the original European perspectives are represented here as well as some new and distinctively American approaches. The collection is characterized by a rich and diverse set of perspectives and experiences that, despite their differences, share a distinctive spirit and direction -- a more humane, creative, and effective relationship between those involved in technology’s design and use, and between technology and the human activities that motivate the technology.},
937 isbn = {978-0-8058-0951-0},
938 title = {Participatory Design: Principles and Practices},
939}
940
941@inbook{Chatila_Havens_2019,
942 collection = {Intelligent Systems, Control and Automation: Science and Engineering},
943 pages = {11–16},
944 year = {2019},
945 editor = {Aldinhas Ferreira, Maria Isabel and Silva Sequeira, João and Singh Virk, Gurvinder and Tokhi, Mohammad Osman and E. Kadar, Endre},
946 author = {Chatila, Raja and Havens, John C.},
947 publisher = {Springer International Publishing},
948 booktitle = {Robotics and Well-Being},
949 doi = {10.1007/978-3-030-12524-0_2},
950 url = {https://doi.org/10.1007/978-3-030-12524-0_2},
951 isbn = {978-3-030-12524-0},
952 title = {The IEEE Global Initiative on Ethics of Autonomous and Intelligent Systems},
953 series = {Intelligent Systems, Control and Automation: Science and Engineering},
954 place = {Cham},
955}
956
957@misc{OBrien_Sweetman_Crampton_Veeraraghavan_2020,
958 month = {Jan},
959 year = {2020},
960 author = {O’Brien, Tim and Sweetman, Steve and Crampton, Natasha and Veeraraghavan, Venky},
961 journal = {World Economic Forum},
962 abstractnote = {What global tech companies can learn from Microsoft’s Responsible AI Champs.},
963 url = {https://www.weforum.org/agenda/2020/01/tech-companies-ethics-responsible-ai-microsoft/},
964 title = {A model for ethical artificial intelligence},
965}
966
967@article{Radaelli_2009,
968 pages = {1145–1164},
969 month = {Dec},
970 year = {2009},
971 author = {Radaelli, Claudio M.},
972 publisher = {Routledge},
973 journal = {Journal of European Public Policy},
974 number = {8},
975 abstractnote = {Do analytic approaches to policy appraisal, specifically regulatory impact assessment (RIA), enable complex organizations to learn? To answer this question, this article distinguishes between types of learning (instrumental, legitimacy-seeking emulation, and political), spells out their micro-foundations, and formulates expectations about evidence drawing on the literature on knowledge utilization. Findings from Denmark, the Netherlands, Sweden, the UK and the EU corroborate emulation and to some extent political learning rather than instrumental learning. The conclusions explain why some types of learning prevail over others.},
976 doi = {10.1080/13501760903332647},
977 issn = {1350-1763},
978 volume = {16},
979 title = {Measuring policy learning: regulatory impact assessment in Europe},
980}
981
982@article{rolnick2019tackling,
983 pages = {33},
984 year = {2019},
985 journal = {arXiv preprint arXiv:1906.05433},
986 author = {Rolnick, David and Donti, Priya L and Kaack, Lynn H and Kochanski, Kelly and Lacoste, Alexandre and Sankaran, Kris and Ross, Andrew Slavin and Milojevic-Dupont, Nikola and Jaques, Natasha and Waldman-Brown, Anna and others},
987 title = {Tackling climate change with machine learning},
988}
989
990@article{rodriguez2017quantifying,
991 publisher = {Springer},
992 year = {2017},
993 pages = {1--18},
994 number = {1},
995 volume = {3},
996 journal = {Current Forestry Reports},
997 author = {Rodriguez-Veiga, Pedro and Wheeler, James and Louis, Valentin and Tansey, Kevin and Balzter, Heiko},
998 title = {Quantifying forest biomass carbon stocks from space},
999}
1000
1001@misc{tara,
1002 year = {2019},
1003 howpublished = {\url{https:
1004//www.planet.com/pulse/developing-the-worlds-first-indicator-of-forestcarbon-stocks-emissions/}},
1005 title = {Developing the world’s first indicator of forest carbon stocks & emissions},
1006 author = {O’Shea, Tara},
1007}
1008
1009@misc{mushegian,
1010 year = {2018},
1011 howpublished = {\url{http://ai4good.org/carbon-mitigation/}},
1012 title = {Modelling Carbon Sequestration Rates in Recovering and Secondary Forests Worldwide},
1013 author = {Mushegian, N., Zhu, A., Santini, G., Uliana, P., & Balingit, K.},
1014}
1015
1016@misc{BioCarbon,
1017 month = {5},
1018 year = {2019},
1019 title = {Drones planting trees: An interview with BioCarbon engineering},
1020 author = {BioCarbon Engineering},
1021}
1022
1023@article{hethcoat2019machine,
1024 publisher = {Elsevier},
1025 year = {2019},
1026 pages = {569--582},
1027 volume = {221},
1028 journal = {Remote sensing of environment},
1029 author = {Hethcoat, Matthew G and Edwards, David P and Carreiras, Joao MB and Bryant, Robert G and Franca, Filipe M and Quegan, Shaun},
1030 title = {A machine learning approach to map tropical selective logging},
1031}
1032
1033@article{lippitt2008mapping,
1034 publisher = {American Society for Photogrammetry and Remote Sensing},
1035 year = {2008},
1036 pages = {1201--1211},
1037 number = {10},
1038 volume = {74},
1039 journal = {Photogrammetric Engineering \& Remote Sensing},
1040 author = {Lippitt, Christopher D and Rogan, John and Li, Zhe and Eastman, J Ronald and Jones, Trevor G},
1041 title = {Mapping selective logging in mixed deciduous forest},
1042}
1043
1044@book{Karachalios_Stern_Havens_2019,
1045 pages = {17},
1046 year = {2019},
1047 author = {Karachalios, Konstantinos and Stern, Nick and Havens, John C.},
1048 institution = {IEEE Standards Association},
1049 url = {https://standards.ieee.org/content/dam/ieee-standards/standards/web/documents/other/ec-measuring-what-matters.pdf},
1050 title = {Measuring What Matters in the Era of Global Warming and the Age of Algorithmic Promises},
1051 place = {Piscataway, New Jersey},
1052}
1053
1054@book{European_Commission_2019,
1055 pages = {41},
1056 month = {Apr},
1057 year = {2019},
1058 author = {European Commission, High-Level Expert Group on Artificial Intelligence (AI HLEG)},
1059 institution = {European Commission, High-Level Expert Group on Artificial Intelligence (AI HLEG)},
1060 url = {https://ec.europa.eu/newsroom/dae/document.cfm?doc_id=58477},
1061 title = {European Commission: Ethical Guidelines for Trustworthy AI},
1062 place = {Brussels, Belgium},
1063}
1064
1065@inproceedings{Schiff_Ayesh_Musikansi_2020,
1066 year = {2020},
1067 author = {Schiff, Daniel and Ayesh, Aladdin and Musikansi, Laura},
1068 booktitle = {Paper under review},
1069 title = {IEEE 7010: A New Standard for Assessing the Well-being Implications of Artificial Intelligence},
1070}
1071
1072@book{Fjeld_Achten_Hilligoss_Nagy_Srikumar_2020,
1073 month = {Jan},
1074 year = {2020},
1075 author = {Fjeld, Jessica and Achten, Nele and Hilligoss, Hannah and Nagy, Adam and Srikumar, Madhulika},
1076 institution = {Berkman Klein Center for Internet & Society},
1077 number = {ID 3518482},
1078 abstractnote = {The rapid spread of artificial intelligence (AI) systems has precipitated a rise in ethical and human rights-based frameworks intended to guide the development and use of these technologies. Despite the proliferation of these “AI principles,” there has been little scholarly focus on understanding these efforts either individually or as contextualized within an expanding universe of principles with discernible trends.To that end, this white paper and its associated data visualization compare the contents of thirty-six prominent AI principles documents side-by-side. This effort uncovered a growing consensus around eight key thematic trends: privacy, accountability, safety and security, transparency and explainability, fairness and non-discrimination, human control of technology, professional responsibility, and promotion of human values. Underlying this “normative core,” our analysis examined the forty-seven individual principles that make up the themes, detailing notable similarities and differences in interpretation found across the documents. In sharing these observations, it is our hope that policymakers, advocates, scholars, and others working to maximize the benefits and minimize the harms of AI will be better positioned to build on existing efforts and to push the fractured, global conversation on the future of AI toward consensus.},
1079 url = {https://papers.ssrn.com/abstract=3518482},
1080 title = {Principled Artificial Intelligence: Mapping Consensus in Ethical and Rights-Based Approaches to Principles for AI},
1081 place = {Rochester, NY},
1082}
1083
1084@article{Nilsson_Schopfhauser_1995,
1085 pages = {267–293},
1086 month = {Jul},
1087 year = {1995},
1088 author = {Nilsson, Sten and Schopfhauser, Wolfgang},
1089 journal = {Climatic Change},
1090 number = {3},
1091 abstractnote = {We analyzed the changes in the carbon cycle that could be achieved with a global, largescale afforestation program that is economically, politically, and technically feasible. We estimated that of the areas regarded as suitable for large-scale plantations, only about 345 million ha would actually be available for plantations and agroforestry for the sole purpose of sequestering carbon. The maximum annual rate of carbon fixation (1.48 Gt/yr) would only be achieved 60 years after the establishment of the plantations - 1.14 Gt by above-ground biomass and 0.34 Gt by below-ground biomass. Over the period from 1995 to 2095, a total of 104 Gt of carbon would be sequestered. This is substantially lower than the amount of carbon required to offset current carbon emissions (3.8 Gt/yr) in order to stabilize the carbon content of the atmosphere.},
1092 doi = {10.1007/BF01091928},
1093 issn = {1573-1480},
1094 volume = {30},
1095 title = {The carbon-sequestration potential of a global afforestation program},
1096}
1097
1098@article{Musikanski_Rakova_Bradbury_Phillips_Manson_2020,
1099 pages = {39–55},
1100 month = {Mar},
1101 year = {2020},
1102 author = {Musikanski, Laura and Rakova, Bogdana and Bradbury, James and Phillips, Rhonda and Manson, Margaret},
1103 journal = {International Journal of Community Well-Being},
1104 number = {1},
1105 abstractnote = {We are calling for a new area of research on the nexus of community well-being and artificial intelligence (AI). Three components of this research we propose are (1) the development and use of well-being metrics to measure the impacts of AI; (2) the use of community-based approaches in the development of AI; and (3) development of AI interventions to safeguard or improve community well-being. After providing definitions of community, well-being, and community well-being, we suggest a definition of AI for use by community well-being researchers, with brief explanations of types and uses of AI within this context. A brief summary of threats and opportunities facing community well-being for which AI could potentially present solutions or exacerbate problems is provided. The three components we propose are then discussed, followed by our call for cross-sector, interdisciplinary, transdisciplinary and systems-based approaches for the formation of this proposed area of research.},
1106 doi = {10.1007/s42413-019-00054-6},
1107 issn = {2524-5295, 2524-5309},
1108 volume = {3},
1109 title = {Artificial Intelligence and Community Well-being: A Proposal for an Emerging Area of Research},
1110}
1111
1112@article{Vinuesa_2020,
1113 pages = {1–10},
1114 month = {Jan},
1115 year = {2020},
1116 author = {Vinuesa, Ricardo and Azizpour, Hossein and Leite, Iolanda and Balaam, Madeline and Dignum, Virginia and Domisch, Sami and Felländer, Anna and Langhans, Simone Daniela and Tegmark, Max and Fuso Nerini, Francesco},
1117 publisher = {Nature Publishing Group},
1118 journal = {Nature Communications},
1119 number = {11},
1120 abstractnote = {Artificial intelligence (AI) is becoming more and more common in people’s lives. Here, the authors use an expert elicitation method to understand how AI may affect the achievement of the Sustainable Development Goals.},
1121 doi = {10.1038/s41467-019-14108-y},
1122 issn = {2041-1723},
1123 volume = {11},
1124 title = {The role of artificial intelligence in achieving the Sustainable Development Goals},
1125}
1126
1127@article{Elkington_1998,
1128 pages = {37–51},
1129 year = {1998},
1130 author = {Elkington, John},
1131 journal = {Environmental Quality Management},
1132 number = {1},
1133 abstractnote = {Editor’s Note: John Elkington’s new book, Cannibals with Forks: The Triple Bottom Line of 21st-Century Business, has been hailed as “practical, compassionate and deeply informed, a brilliant synthesis of his genius for cutting through the thicket of tough issues–in the world of business and sustainability–and producing elegant solutions that can be applied today” (Paul Hawken). We are pleased to have the opportunity to publish a selection from this award-winning book. In this discussion of partnerships, Elkington explores how effective, long-term partnerships will be crucial for companies making the transition to sustainability and offers approaches and examples of keen interest. Special thanks to Capstone Publishers, U.K., for their gracious cooperation.},
1134 doi = {10.1002/tqem.3310080106},
1135 issn = {1520-6483},
1136 volume = {8},
1137 title = {Partnerships from cannibals with forks: The triple bottom line of 21st-century business},
1138}
1139
1140@article{Kursuncu_Gaur_Sheth_2020,
1141 month = {Feb},
1142 year = {2020},
1143 author = {Kursuncu, Ugur and Gaur, Manas and Sheth, Amit},
1144 journal = {arXiv:1912.00512 [cs]},
1145 note = {arXiv: 1912.00512},
1146 abstractnote = {Learning the underlying patterns in data goes beyond instance-based generalization to external knowledge represented in structured graphs or networks. Deep learning that primarily constitutes neural computing stream in AI has shown significant advances in probabilistically learning latent patterns using a multi-layered network of computational nodes (i.e., neurons/hidden units). Structured knowledge that underlies symbolic computing approaches and often supports reasoning, has also seen significant growth in recent years, in the form of broad-based (e.g., DBPedia, Yago) and domain, industry or application specific knowledge graphs. A common substrate with careful integration of the two will raise opportunities to develop neuro-symbolic learning approaches for AI, where conceptual and probabilistic representations are combined. As the incorporation of external knowledge will aid in supervising the learning of features for the model, deep infusion of representational knowledge from knowledge graphs within hidden layers will further enhance the learning process. Although much work remains, we believe that knowledge graphs will play an increasing role in developing hybrid neuro-symbolic intelligent systems (bottom-up deep learning with top-down symbolic computing) as well as in building explainable AI systems for which knowledge graphs will provide scaffolding for punctuating neural computing. In this position paper, we describe our motivation for such a neuro-symbolic approach and framework that combines knowledge graph and neural networks.},
1147 url = {http://arxiv.org/abs/1912.00512},
1148 title = {Knowledge Infused Learning (K-IL): Towards Deep Incorporation of Knowledge in Deep Learning},
1149}
1150
1151@inbook{Nadeau_Bengio_2000,
1152 pages = {307–313},
1153 year = {2000},
1154 editor = {Solla, S. A. and Leen, T. K. and Müller, K.},
1155 author = {Nadeau, Claude and Bengio, Yoshua},
1156 publisher = {MIT Press},
1157 booktitle = {Advances in Neural Information Processing Systems 12},
1158 url = {http://papers.nips.cc/paper/1661-inference-for-the-generalization-error.pdf},
1159 title = {Inference for the Generalization Error},
1160}
1161
1162@article{Gardner_2019,
1163 pages = {163–177},
1164 month = {Sep},
1165 year = {2019},
1166 author = {Gardner, T. A. and Benzie, M. and Börner, J. and Dawkins, E. and Fick, S. and Garrett, R. and Godar, J. and Grimard, A. and Lake, S. and Larsen, R. K. and et al.},
1167 journal = {World Development},
1168 abstractnote = {Over the last few decades rapid advances in processes to collect, monitor, disclose, and disseminate information have contributed towards the development of entirely new modes of sustainability governance for global commodity supply chains. However, there has been very little critical appraisal of the contribution made by different transparency initiatives to sustainability and the ways in which they can (and cannot) influence new governance arrangements. Here we seek to strengthen the theoretical underpinning of research and action on supply chain transparency by addressing four questions: (1) What is meant by supply chain transparency? (2) What is the relevance of supply chain transparency to supply chain sustainability governance? (3) What is the current status of supply chain transparency, and what are the strengths and weaknesses of existing initiatives? and (4) What propositions can be advanced for how transparency can have a positive transformative effect on the governance interventions that seek to strengthen sustainability outcomes? We use examples from agricultural supply chains and the zero-deforestation agenda as a focus of our analysis but draw insights that are relevant to the transparency and sustainability of supply chains in general. We propose a typology to distinguish among types of supply chain information that are needed to support improvements in sustainability governance, and illustrate a number of major shortfalls and systematic biases in existing information systems. We also propose a set of ten propositions that, taken together, serve to expose some of the potential pitfalls and undesirable outcomes that may result from (inevitably) limited or poorly designed transparency systems, whilst offering guidance on some of the ways in which greater transparency can make a more effective, lasting and positive contribution to sustainability.},
1169 doi = {10.1016/j.worlddev.2018.05.025},
1170 issn = {0305-750X},
1171 volume = {121},
1172 title = {Transparency and sustainability in global commodity supply chains},
1173}
1174
1175@book{Cussins_Newman_2020,
1176 collection = {CLTC White Paper Series},
1177 pages = {58},
1178 month = {May},
1179 year = {2020},
1180 author = {Cussins Newman, Jessica},
1181 institution = {UC Berkeley Center for Long-Term Cybersecurity},
1182 url = {https://cltc.berkeley.edu/wp-content/uploads/2020/05/Decision_Points_AI_Governance.pdf},
1183 title = {Decision Points in AI Governance: Three Case Studies Explore Efforts to Operationalize AI Principles},
1184 series = {CLTC White Paper Series},
1185 place = {Berkeley, CA},
1186}
1187
1188@misc{Project_Drawdown_2020a,
1189 month = {Feb},
1190 year = {2020},
1191 author = {Project Drawdown},
1192 journal = {Project Drawdown},
1193 abstractnote = {Tropical forests have suffered extensive clearing, fragmentation, degradation, and depletion of biodiversity. Restoring these forests also restores their function as carbon sinks.},
1194 url = {https://www.drawdown.org/solutions/tropical-forest-restoration},
1195 title = {Tropical Forest Restoration},
1196}
1197
1198@misc{Project_Drawdown_2020b,
1199 month = {Feb},
1200 year = {2020},
1201 author = {Project Drawdown},
1202 journal = {Project Drawdown},
1203 abstractnote = {Degraded lands present potential locations for tree plantations. Managed well, they can restore soil, sequester carbon, and produce wood resources in a more sustainable way.},
1204 url = {https://www.drawdown.org/solutions/tree-plantations-on-degraded-land},
1205 title = {Tree Plantations (on Degraded Land)},
1206}
1207
1208@misc{United_Nations_2020,
1209 year = {2020},
1210 author = {United Nations},
1211 url = {https://sustainabledevelopment.un.org/topics/forests},
1212 title = {Forests},
1213}
1214
1215@article{Bucknum_1997,
1216 pages = {305–344},
1217 year = {1997},
1218 author = {Bucknum, Susan},
1219 journal = {Duke Environmental Law & Policy Forum},
1220 number = {2},
1221 volume = {8},
1222 title = {The U.S. Commitment to Agenda 21: Chapter 11 Combating Deforestation-The Ecosystem Management Approach Note},
1223}
1224
1225@book{Carolee_Heather_2014,
1226 month = {Nov},
1227 year = {2014},
1228 author = {Carolee, Buckler and Heather, Creech},
1229 publisher = {UNESCO},
1230 note = {Google-Books-ID: ImZuBgAAQBAJ},
1231 isbn = {978-92-3-100053-9},
1232 title = {Shaping the future we want: UN Decade of Education for Sustainable Development; final report},
1233}
1234
1235@article{Sitarz_1993,
1236 month = {Jan},
1237 year = {1993},
1238 author = {Sitarz, D.},
1239 publisher = {Boulder, CO (United States); EarthPress},
1240 abstractnote = {The U.S. Department of Energy’s Office of Scientific and Technical Information},
1241 url = {https://www.osti.gov/biblio/6289330},
1242 title = {Agenda 21: The Earth summit strategy to save our planet},
1243}
1244
1245@inbook{Schmoldt_2001,
1246 collection = {Managing Forest Ecosystems},
1247 pages = {49–74},
1248 year = {2001},
1249 editor = {von Gadow, Klaus},
1250 author = {Schmoldt, Daniel L.},
1251 publisher = {Springer Netherlands},
1252 booktitle = {Risk Analysis in Forest Management},
1253 abstractnote = {Forest ecosystems are subject to a variety of natural and anthropogenic disturbances that extract a penalty from human population values. Such value losses (undesirable effects) combined with their likelihoods of occurrence constitute risk. Assessment or prediction of risk for various events is an important aid to forest management. Artificial intelligence (AI) techniques have been applied to risk analysis owing to their ability to deal with uncertainty, vagueness, incomplete and inexact specifications, intuition, and qualitative information. This paper examines knowledge-based systems, fuzzy logic, artificial neural networks, and Bayesian belief networks and their application to risk analysis in the context of forested ecosystems. Disturbances covered are: fire, insects/diseases, meteorological, and anthropogenic. Insect/disease applications use knowledge-based system methods exclusively, whereas meteorological applications use only artificial neural networks. Bayesian belief network applications are almost nonexistent, even though they possess many theoretical and practical advantages. Embedded systems -that use AI alongside traditional methods-are, not unexpectedly, quite common.},
1254 doi = {10.1007/978-94-017-2905-5_3},
1255 url = {https://doi.org/10.1007/978-94-017-2905-5_3},
1256 isbn = {978-94-017-2905-5},
1257 title = {Application of Artificial Intelligence to Risk Analysis for Forested Ecosystems},
1258 series = {Managing Forest Ecosystems},
1259 place = {Dordrecht},
1260}
Attribution
arXiv:2006.04707v1
[cs.CY]
License: cc-by-4.0