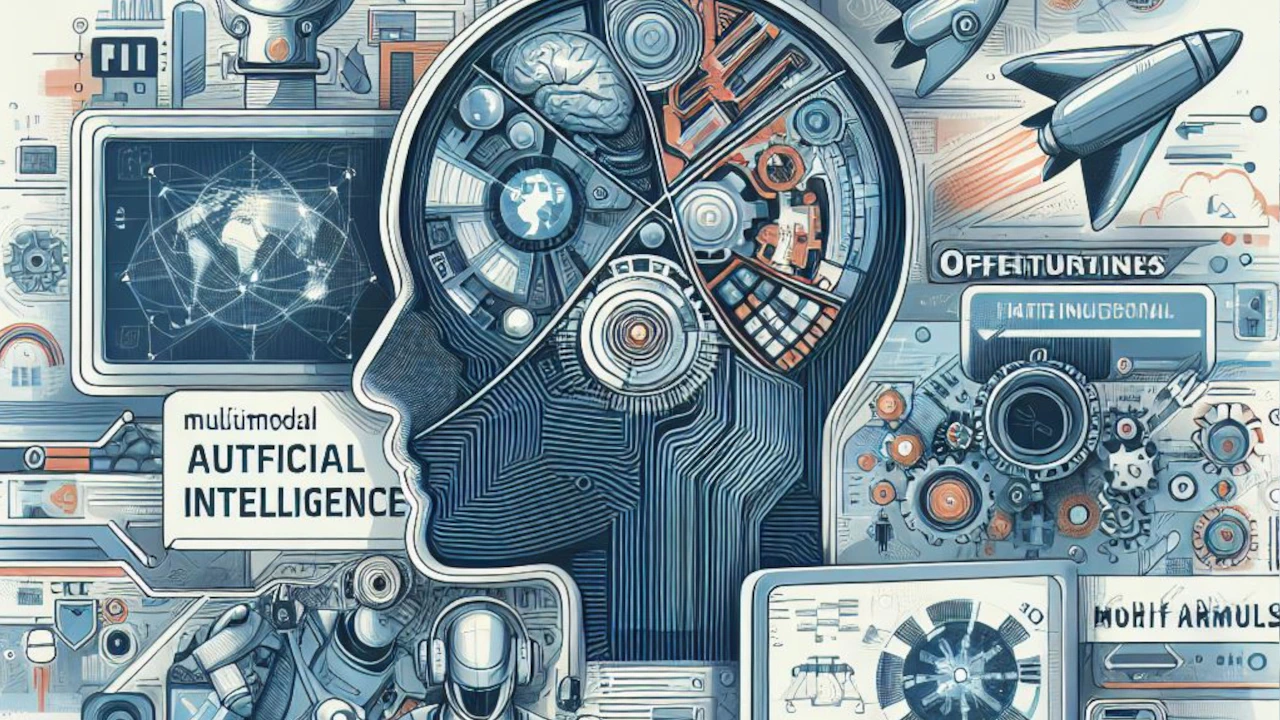
- By Justin Riddiough
- December 9, 2023
As the field of multimodal models continues to evolve, exciting new developments are on the horizon. Let’s explore some of the emerging trends and potential breakthroughs that promise to shape the future of this technology:
Emerging Trends in Multimodal Research
- Multimodal Transformer Architectures: These powerful architectures are revolutionizing how we process and understand multimodal data, leading to significant improvements in performance across various tasks.
- Self-Supervised Learning: By utilizing the inherent structure and relationships within data, self-supervised learning techniques can significantly reduce the need for labeled data, making multimodal model training more efficient and scalable.
- Multimodal Explainability and Interpretability: Understanding how multimodal models make decisions is crucial for building trust and ensuring responsible AI. Research efforts are focusing on developing techniques to explain and interpret the inner workings of these complex models.
The future of multimodal models is bright, with new research directions promising advancements in performance, efficiency, and interpretability.
Ethical Considerations: Navigating the Moral Maze of Multimodal AI
While the potential of multimodal models is immense, ethical considerations remain critical. Here are some key concerns to be addressed:
- Bias: Multimodal models can inherit and amplify biases present in the data they are trained on. This can lead to unfair and discriminatory outcomes.
- Privacy: Multimodal data can be highly sensitive and reveal personal information. Protecting user privacy and ensuring responsible data collection and usage are paramount.
- Transparency and Explainability: Lack of transparency and explainability can make it difficult to understand how models arrive at their decisions, hindering trust and accountability.
Responsible AI practices are essential for ensuring the ethical development and application of multimodal models.
Building a Future of Responsible Multimodal AI
To ensure the ethical and beneficial development of multimodal models, it’s crucial to:
- Develop diverse and representative datasets: This helps mitigate bias and ensure models are fair and inclusive.
- Implement robust privacy-preserving techniques: Mechanisms like data anonymization and differential privacy can protect user privacy.
- Invest in research on explainability and interpretability: This allows us to understand how models work and build trust in their decisions.
By prioritizing ethical considerations and engaging in open dialogue, we can ensure that multimodal models are used for good, empowering us to build a future where technology serves humanity in a responsible and beneficial manner.
A Bright Future Awaits
Multimodal models hold immense potential to revolutionize various fields, from healthcare and education to entertainment and robotics. As we navigate the exciting future of this technology, it’s crucial to remain vigilant about ethical concerns and prioritize responsible development and application. By ensuring fairness, inclusivity, and transparency, we can build a future where multimodal AI empowers us to create a better world for all.