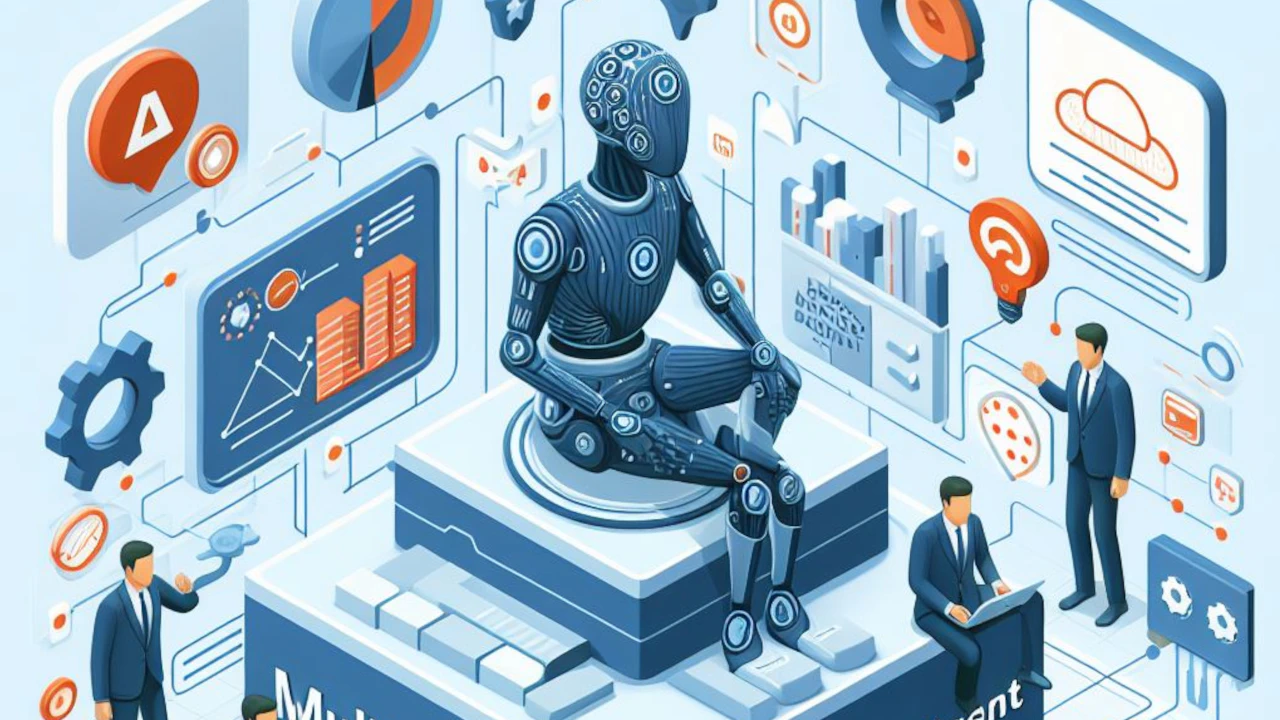
- By Justin Riddiough
- December 9, 2023
Defining Multimodality: Beyond the Unimodal World
Unlike traditional models that focus on a single modality, multimodal models analyze and process data from multiple sources, creating a unified representation of the information. This fusion of modalities allows machines to understand the world in a way that is more nuanced and comprehensive, similar to the way humans do.
“Seeing” through AI: Multimodal models unlock possibilities for accessibility, information retrieval, and artistic exploration.
Advantages of Multimodality: Enhancing AI Capabilities
By combining multiple modalities, multimodal models offer a number of advantages:
- Improved Accuracy and Performance: Multimodal models can achieve significantly better accuracy and performance on various tasks compared to unimodal models.
- Enhanced Context and Understanding: By analyzing multiple perspectives, multimodal models can gain a richer understanding of the context and meaning of information.
- Increased Robustness and Generalizability: Multimodal models are less susceptible to noise and errors in individual modalities, leading to more robust and generalizable results.
Types and Use Cases: A Multimodal Landscape
The world of multimodal models is diverse and constantly expanding. Here are some of the most common types and their exciting applications:
Text-Image Models:
- Image Captioning: Generate accurate and descriptive captions for images.
- Visual Question Answering: Answer questions about images based on their visual content.
- Image Retrieval: Find specific images based on textual descriptions.
Text-Audio Models:
- Automatic Speech Recognition: Transcribe spoken language with high accuracy.
- Sentiment Analysis: Analyze the emotional tone of spoken language.
- Speaker Recognition: Identify speakers based on their voice characteristics.
Multimodal Attention Mechanisms:
- Focus on relevant information: Models can dynamically focus on specific parts of each modality, leading to better performance.
- Capture complex relationships: Learn the complex relationships between different modalities, leading to a deeper understanding of the data.
- Improve interpretability: Make models more transparent and easier to understand, fostering trust and responsible AI practices.
Emerging Trends: A Glimpse into the Future
The field of multimodal models is rapidly evolving, with new research directions promising significant advancements:
- Self-supervised learning: Learn from the inherent structure of data, reducing the need for labeled data and making training more efficient.
- Multimodal transformers: Powerful architectures capable of processing complex multimodal data, leading to breakthroughs in performance and accuracy.
- Explainability and interpretability: Techniques to understand how multimodal models make decisions, building trust and ensuring responsible AI practices.
A Multimodal Future Awaits
Multimodal models represent a significant leap forward in AI capabilities, offering the promise of a future where machines understand the world in a way that is more human-like. By harnessing the power of multiple modalities, we can unlock new possibilities in various fields, from healthcare and education to entertainment and robotics. As research continues and ethical considerations are addressed, the future of multimodal models is bright, paving the way for a world where technology empowers us to create a better future for all.