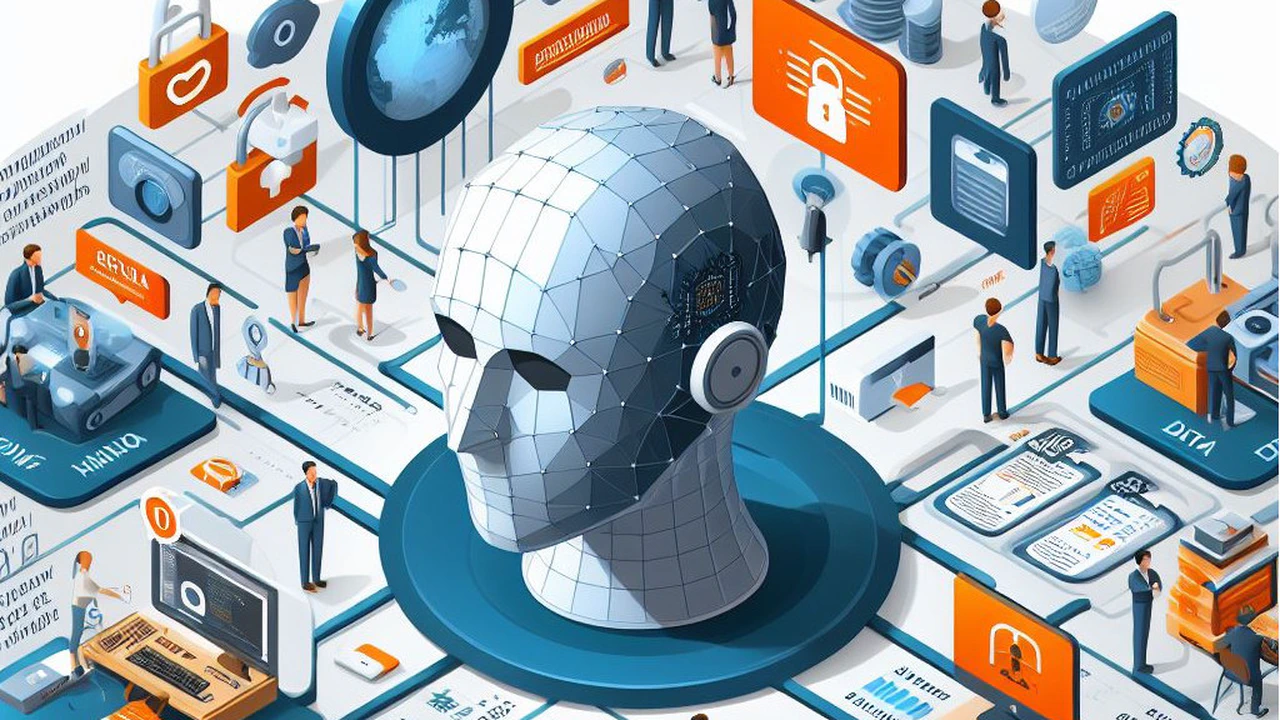
- By Justin Riddiough
- December 10, 2023
In the realm of AI governance, prioritizing the safety and resilience of AI systems is a non-negotiable responsibility. For those at the helm of implementation, ensuring reliability, safety, security, and robustness are key objectives. Let’s delve into actionable strategies and best practices to achieve these goals.
Ensuring Reliability and Harm Prevention
Reliability is the linchpin of AI systems. It’s imperative to ensure that the AI system is trustworthy and will not cause harm. Here’s a roadmap to achieve this:
- Impact/Risk Assessment: Conduct a comprehensive impact and risk assessment to identify potential hazards and vulnerabilities.
Practical Example: In an autonomous vehicle system, a risk assessment may involve evaluating the impact of sensor malfunctions and implementing fail-safes to prevent accidents.
- Known Risks Identification/Mitigation: Systematically identify and mitigate known risks through proactive measures.
Best Practice: Maintain an up-to-date register of known risks and corresponding mitigation strategies. Regularly review and update as needed.
Safety: Conducting Impact/Risk Assessment
Safety in AI governance entails conducting thorough impact and risk assessments. This proactive approach is instrumental in identifying potential hazards and vulnerabilities. Consider the following best practices:
- Collaborative Assessments: Involve cross-functional teams, including AI experts, domain specialists, and risk management professionals, in the assessment process.
Real-world Application: In a healthcare AI system, collaboration between data scientists and medical professionals ensures a holistic understanding of potential risks in diagnostic decision-making.
- Continuous Monitoring: Implement continuous monitoring mechanisms to detect and address emerging risks over the AI system’s lifecycle.
Practical Example: A financial institution regularly monitors its AI-driven fraud detection system to adapt to evolving fraud patterns.
Security: Protecting AI Systems
Security is paramount in the AI landscape. Protecting AI systems from unauthorized access, disclosure, modification, destruction, or disruption is critical. Adopt these security best practices:
- Access Controls: Implement stringent access controls to restrict system access to authorized personnel only.
Best Practice: Regularly audit and update access permissions to align with organizational changes.
- Encryption: Employ robust encryption mechanisms to safeguard data in transit and at rest.
Real-world Application: In an AI-driven customer service application, encryption ensures the protection of sensitive customer data during interactions.
Robustness: Ensuring Functionality Despite Challenges
Robustness is the measure of an AI system’s ability to function despite unexpected inputs or challenges. Consider the following practices to enhance robustness:
- Error Handling Mechanisms: Implement robust error-handling mechanisms to gracefully manage unexpected situations.
Practical Example: In an AI chatbot, effective error handling ensures a seamless transition to a human operator if the bot encounters a query it can’t handle.
- Diversity in Training Data: Ensure diversity in training data to enhance the AI system’s adaptability to a wide range of inputs.
Best Practice: Regularly update and expand the training dataset to improve the system’s response to new scenarios.
Navigating the Path to AI Safety and Resilience
In the dynamic landscape of AI governance, prioritizing safety and resilience is paramount. By integrating impact assessments, robust security measures, and strategies for system robustness, organizations can navigate the complexities of AI with confidence.
Remember, safety is an ongoing commitment. Regular assessments, collaboration between experts, and staying informed about emerging risks are essential for maintaining a secure and resilient AI ecosystem.