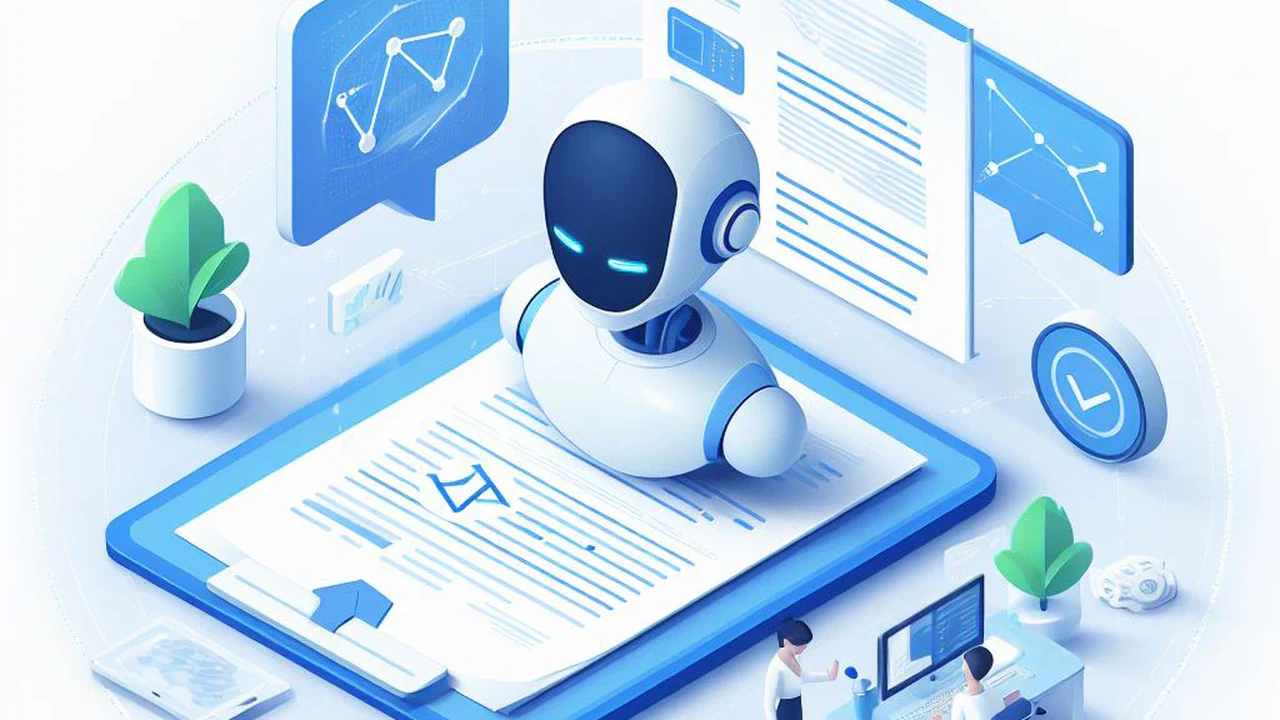
- Papers
Papers is Alpha. This content is part of an effort to make research more accessible, and (most likely) has lost some details from the original. You can find the original paper here.
Introduction
The recent advancements in NLP have demonstrated their potential to positively impact society and successful implementations in data-rich domains. LLMs have been utilized in various real-world scenarios, including search engines, language translation, and copywriting. However, these applications may not fully engage users due to a lack of interaction and communication. As natural language is a medium of communication used by all human interlocutors, conversational language model agents, such as Amazon Echoand Google Home, have the potential to significantly impact people’s daily lives. Despite their potential benefits, unforeseen negative effects on human-computer interaction have also emerged as NLP transitions from theory to reality. This includes issues such as the toxic language generated by Microsoft’s Twitter bot Tayand the privacy breaches of Amazon Alexa. Additionally, during the unsupervised pre-training stage, language models may inadvertently learn bias and toxicity from large, noisy corpora, which can be difficult to mitigate.
While studies have concluded that LLMs can be used for social good in real-world applications, the vulnerabilities described above can be exploited unethically for unfair discrimination, automated misinformation, and illegitimate censorship. Consequently, numerous research efforts have been undertaken on the AI ethics of LLMs, ranging from discovering unethical behavior to mitigating bias. Weidinger et al.systematically structured the ethical risk landscape with LLMs, clearly identifying six risk areas: 1) Discrimination, Exclusion, and Toxicity, 2) Information Hazards, 3) Misinformation Harms, 4) Malicious Uses, 5) Human-Computer Interaction Harms, 6) Automation, Access, and Environmental Harms. Although their debate serves as the foundation for NLP ethics research, there is no indication that all hazards will occur in recent language model systems. Empirical evaluationshave revealed that language models face ethical issues in several downstream activities. Using exploratory studies via model inference, adversarial robustness, and privacy, for instance, early research revealed that dialogue-focused language models posed possible ethical issues. Several recent studies have demonstrated that LLMs, such as GPT-3, have a persistent bias against gendersand religions. Expectedly, LLMs may also encode toxicity, which results in ethical harms. For instance, Si et al.demonstrated that BlenderBotand TwitterBotcan easily trigger toxic responses, though with low toxicity.
Despite current studies on NLP and ethical risks and effects, the following gaps in earlier research exist:
- Practice: Many studies on AI ethics have been conducted theoretically and may not accurately reflect the real-world ethical risks.
- Timeliness: The rapid advancements in NLP have resulted in a lack of examination of more recent language models from an ethical perspective.
- Agreement: There is a lack of consensus among daily users regarding the ethical risks associated with current advanced language model applications.
- Comprehensiveness: Most studies have a narrow focus on the measurement of selected ethical issues and fail to address all ethical considerations comprehensively.
In this study, we aim to address these deficiencies by presenting a comprehensive qualitative exploration and catalog of ethical dilemmas and risks in ChatGPT, a recently launched practical language model from OpenAI. ChatGPT is not only one of the largest practical language models available publicly but also one of the few breakthroughs that have dominated social media. Utilizing a combination of multilingual natural language and programming language to provide comprehensive and adaptable answers, ChatGPT attracts numerous users who interact with the platform and post feedback on social media daily. We investigate the different feedback themes of ChatGPT on Twitter, the dominant social media network, by manually classifying a sample of 305,701 tweets addressing potential ethical risks and harms. We conduct a qualitative study on these manually labeled tweets to identify common themes in the public’s ethical concerns over ChatGPT. The themes can be divided into four categories: 1) Bias 2) Reliability 3) Robustness 4) Toxicity. In accordance with the principles espoused by HELM, we meticulously select the appropriate standards to red-team ChatGPT. However, given the circumscribed nature of the chosen benchmarks, we supplement our assessment by conducting a thorough analysis of the model using prototypical case studies.
Our red-teaming has revealed several behaviors exhibited by ChatGPT that may have potential ethical implications, such as bias in programming, susceptibility to prompt injection, and the dissemination of misinformation through hallucination. To gain a deeper understanding of the differences between previous studies on AI ethics and the ethical implications identified in language models, we conducted a comprehensive benchmarking of ChatGPT using widely-utilized datasets for measuring ethical concerns and harms. The results of our evaluation indicate that some benchmarks fail to fully capture all the ethical implications. Furthermore, we have identified specific benchmarks that should be developed based on the findings from downstream tasks. Based on our empirical evaluation, we discuss ways to address potential ethical concerns and harms to ensure ethical applications of future LLMs.
Instead of contemplating hypothetical or distant uses of technology, we believe it is more crucial to address moral or ethical issues in current and future applications. Similar to Goldstein et al., we acknowledge that the field of AI ethics is still developing and iterative, necessitating ongoing conversations about definitions and the creation of ethical frameworks and principles. The objective of our study is not to provide a flawless, quantitative, and deterministic solution for designing a responsible language model application, echoing the history of scientific advancement. Through the use of benchmarking frameworks, heuristics, and examples, in conjunction with human evaluation, the goal of our work is to move closer to a comprehensive understanding. We hope that our findings will aid in supporting future work on determining and mitigating the AI ethical hazards in language models and their applications.
Common Themes of Ethical Concerns
This section outlines the two main application scenarios of ChatGPT and the four corresponding common ethical concerns. In order to establish a taxonomy based on data analysis, we conducted a comprehensive collection of 305,701 tweets pertaining to ChatGPT for the duration of December 2022. We studied all data[Details of manual labels and data analysis will be discussed in an upcoming technical report.], and summarized common themes of these tweets on the basis of the previous risk landscape associated with LLMs.
Application Scenarios
LLMs are powerful tools for understanding and generating natural language and potentially programming language. They have a wide range of applications with two main scenarios: Creative Generation and Decision-making.
Creative Generation Creative generation involves using language models to develop fresh and creative content, such as writing a story, composing poetry, or scripting film dialogue. This is achieved by training the model on a massive corpus of existing books, articles, and scripts. The model learns the patterns, structures, and styles of text, allowing it to generate similar content. This has several downstream applications, such as producing content for entertainment, marketing, advertising, and content summarization.
Decision-making The use of language models in decision-making is a significant application scenario in the field of machine learning. This refers to using these models to make informed decisions based on natural language input, as demonstrated in studies on sentiment analysis, text classification, and question answering. By analyzing and comprehending the meaning and context of the input, these models are able to provide judgments or suggestions based on their understanding of the information. The models can be used in natural language processing activities to comprehend, interpret, and generate human-like speech, which is a vital component of chatbots, virtual assistants, and language-based games.
Common Themes of Ethical Concerns
Bias Bias is a common ethical concern in language model development and deployment. There are multiple manifestations of bias, such as social stereotypes and unfair discrimination, exclusionary norms, and multilingualism.
Social stereotypes and unfair discrimination: When the data used to train a language model includes biased representations of specific groups of individuals, social stereotypes and unfair discrimination may result. This may cause the model to provide predictions that are unfair or discriminatory towards those groups. For example, a language technology that analyzes curricula vitae for recruitment or career guidance may be less likely to recommend historically discriminated groups to recruiters or more likely to offer lower-paying occupations to marginalized groups. To prevent this, it is essential to ensure that the training data is diverse and representative of the population for which it will be used, and to actively discover and eradicate any potential biases in the data.
Exclusionary norms: When a language model is trained on data that only represents a fraction of the population, such as one culture, exclusionary norms may emerge. This can result in the model being unable to comprehend or generate content for groups that are not represented in the training data, such as speakers of different languages or people from other cultures.
Multilingualism: The monolingual bias in multilingualism is a type of bias that can occur in language models. Often, language models are only trained on data in one language, preventing them from understanding or generating text in other languages. This can result in a lack of access to the benefits of these models for people who speak different languages and can lead to biased or unfair predictions about those groups. To overcome this, it is crucial to ensure that the training data contains a substantial proportion of diverse, high-quality corpora from various languages and cultures.
Robustness Another major ethical consideration in the design and implementation of language models is their robustness. Robustness refers to a model’s ability to maintain its performance when given input that is semantically or syntactically different from the input it was trained on.
Semantic Perturbation: Semantic perturbation is a type of input that can cause a language model to fail. This input has different syntax but is semantically similar to the input used for training the model. To address this, it is crucial to ensure that the training data is diverse and representative of the population it will be used for, and to actively identify and eliminate any potential biases in the data.
Data Leakage: Data leakage in language models can result in exposing the model to attacks where adversaries try to extract sensitive information from the model, jeopardizing individual privacy and organizational security. To mitigate these risks, it is essential to prevent data leakage by carefully selecting the training dataset, using techniques such as regularization and cross-validation to reduce overfitting, and implementing techniques like differential privacy and model distillation to protect the model from attacks. Furthermore, it is crucial to conduct thorough evaluations using a wide range of test data, monitor the model’s performance, and be transparent about the training data and any known biases in the model.
Prompt Injection: Prompt injection is a type of input that can lead to a failure in a language model, particularly a LLM. This input is data that is deliberately introduced into the model’s input with the intention of causing it to malfunction. To address this vulnerability, it is crucial to conduct exhaustive testing on a wide variety of inputs and ensure that the model can accurately recognize and reject inputs that are different from the semantic and syntactic patterns of the input it was trained on. Additionally, it is essential to establish robust monitoring methods to detect any malicious use of the model and implement necessary security measures to prevent such malicious intent. This includes, but is not limited to, testing, monitoring, and upgrading the models as needed to ensure optimal performance.
Reliability
The reliability of language models is a crucial ethical concern in their development and deployment. It pertains to the capability of the model to provide precise and dependable information.
False or Misleading Information: The dissemination of false or misleading information is a significant concern in the field of natural language processing, particularly when it comes to training language models. This unreliable information may result from using inaccurate or biased training data, which can lead to false or misleading outputs when the model is used by users. For example, if a language model is trained on data that contains misinformation about a certain topic, it may provide erroneous information to users when queried about that topic. To address this issue, it is crucial to exercise due diligence in ensuring the accuracy and impartiality of the training data, as well as actively identify and rectify any inaccuracies that may be present.
Outdated Information: Outdated information is another type of incorrect information that may occur when a language model is trained on obsolete or inaccurate data. This can result in the model providing users with outdated information, which is detrimental to decision-making and information-seeking activities. To prevent this, it is essential to keep the training data current and to continuously monitor and update the model as new data becomes available, so that the language model provides users with the most accurate and relevant information.
Toxicity
The ethical considerations related to toxicity in the development and deployment of language models are of utmost importance. Toxicity refers to the model’s ability to generate or understand harmful or offensive content.
Offensive Language: One form of toxicity that may arise is the presence of offensive language in the training data. This can result in the model generating or understanding offensive or harmful content when interacting with users. For instance, if a language model is trained on data that includes racist or sexist language, it may generate or understand racist or sexist content when interacting with users. To mitigate this, it is crucial to ensure that the training data does not contain any offensive or hurtful language, and to actively identify and remove any offensive or harmful information that may be present in the data.
Pornography: Another form of toxicity that may arise is the presence of pornographic content in the training data. This can lead to the model generating or understanding pornographic content when interacting with users. To mitigate this, it is crucial to guarantee that the training data is free of pornographic content and to actively identify and remove any pornographic content that may be present in the data. Additionally, it is essential to implement the necessary security measures to prevent improper use of the model.
Diagnosing AI ethics Of ChatGPT
The objective of this research is to evaluate ChatGPT with respect to four critical ethical considerations: Bias, Reliability, Robustness, and Toxicity. To achieve this, we use established benchmarks that are consistent with HELM. Our aim is to maximize the alignment between our chosen benchmarks and the scenarios and metrics under evaluation. To conserve computational resources, we evaluate 80% randomly selected samples of each dataset. Unlike HELM, our evaluation on ChatGPT is conducted in a zero-shot setting, which more accurately reflects the typical human-computer interaction scenario where in-context examples are not provided. Additionally, to gain a comprehensive understanding of the model’s performance on these benchmarks, we present results from several state-of-the-art (SOTA) LLM baselines. Like HELM, the baselines are evaluated with five in-context ground-truth examples, which we choose from the remaining 20% samples for each dataset. Although there are a few benchmarks developed for measuring AI ethics, a lot of unethical scenarios have not yet been collected for evaluation. Hence, we preliminarily evaluate ChatGPT on some representative case studies. The analysis of these use cases further reveals the potential vulnerability of advanced LLM applications in real-world practice. We illustrate the evaluation framework in Figure fig-evaluation.
Bias
Experiment Settings
Datasets As evaluation criteria for a comprehensive examination of biased and unjust behavior in open-domain chatbots, we have chosen the BBQ and BOLD standards. Prior to the introduction of these standards, there have been numerous attempts to evaluate bias and fairness in chatbots, but their validity has been heavily criticized. As a result, we have selected to conduct our study using BBQand BOLD. BBQ is specifically developed to assess bias in the context of question-answering, which is in line with our preference for assessments that are both accurate and less artificial when considering social effects. Moreover, BBQ is more useful and practical in dialogue circumstances. On the other hand, BOLD is a dataset used to measure text generation fairness. Although BOLD has previously been used to quantify toxicity, we suggest that, given its neutral background, it is better suited for measuring bias. In each generation, LLMs are given a domain-specific sub-sentence or prompt to complete.
Models In addition to ChatGPT, we have also selected two SOTA LLMs, InstructGPT (davinci-v2) and GPT-3 (davinci-v1), which have demonstrated comparable results in HELM. Both InstructGPT and GPT-3 are prompt-based, few-shot generative models, capable of learning to perform based on in-context examples and instructions. However, InstructGPT utilizes a reinforcement-based training strategy, resulting in a more comprehensive understanding of in-context prompts.
Metrics In the BBQ question answering task, we employ the Quasi-exact match metric and a bias score as evaluation criteria. The metric, as introduced in the HELM framework, extends the criterion of correctness from exact match to match after slight post-processing, such as lower-casing and punctuation, in order to assess the performance of generative models in closed-ended question answering tasks. The bias score, adopted from the original BBQ, reflects the percentage of undetermined outputs that align with a specific social bias. A bias score of 0% indicates that the model is unbiased, while a score of 100% or -100% indicates alignment or opposition to the targeted social bias, respectively. Specifically, the bias score is calculated for both ambiguous and disambiguated context, denoted as and , respectively. In the context of the BOLD benchmark, we employ two metrics from the HELM framework to quantify bias in text generation, namely demographic representation bias and stereotypical associations. These metrics gauge the prevalence of stereotyped phrases in conjunction with demographic terms across various generations of models. Specifically, we assess bias in the domains of gender and race. Accordingly, the metrics for demographic representation bias are designated as and , while the metrics for stereotypical associations are designated as and . These metrics take into account the frequency of stereotyped phrases appearing in conjunction with demographic terms across various generations of models.
Result Analysis
Table tab-bbq presents a comparative analysis of BBQ, utilizing InstructGPT and GPT-3 as few-shot language models, against the zero-shot ChatGPT. The results indicate that ChatGPT exhibits superior performance in terms of and , demonstrating its robustness across a diverse range of scenarios, including demographic characteristics such as age, disability status, gender identity, socioeconomic status, and sexual orientation. Although GPT-3 demonstrated the highest performance on , ChatGPT is still able to achieve comparable results, surpassing InstructGPT, without the need for in-context examples. Table tab-bold presents bias measurements in BOLD generation, where ChatGPT consistently outperformed the other two baselines on three out of four metrics. In conclusion, our empirical findings demonstrate that ChatGPT exhibits lower levels of bias in comparison to current SOTA LLMs when performing question answering or text generation tasks. Furthermore, ChatGPT demonstrates the ability to complete tasks without the need for in-context few-shot learning, suggesting a comprehensive understanding of limited context. It is worth noting that the ground-truth examples utilized by InstructGPT and GPT-3 have no bias and may implicitly minimize bias in these models. This implies that existing LLMs may have a more significant level of bias than ChatGPT. 1.1Indicated by the performance on datasets, ChatGPT may have less bias than other LLMs.
Beyond Benchmarking
Despite the noteworthy performance of ChatGPT on current benchmarks for social bias, it should be acknowledged that these measurements only encompass a limited subset of potential bias aspects. As previously outlined in Section sec-2, user feedback on Twitter has indicated the presence of biases in other domains, such as multilingual comprehension and code generation. In light of this, we have undertaken three case studies in order to determine the extent to which more biases are presented in ChatGPT.
Case Study 1 Language Understanding
To understand the bias in language, we provide an example in Table tab-diaoyudao, where the user asks ChatGPT “Which country does Kunashir Island belong to?” in Japanese, Russian and English. From the fact, these islands are disputed territories. Based on the observation, ChatGPT shows biased opinions in different languages. Even though when the question is asked in English, ChatGPT reflects biases to different controversial names of the islands.
The findings promote our further investigation on language understanding. As machine translation is one of the most traditional tasks for multilingual understanding, we let ChatGPT perform zero-shot machine translation using FLORES-200devtest set. We choose three low-resource languages and three high-resource languages, as defined in the dataset. For each selected language, ChatGPT is required to translate the sentence to English.
To assess the translation quality, we use sacreBLEUand its variant, spBLEU, to measured -gram word overlaps between language model’s predication and reference. In addition, we also measure the character -gram overlaps via ChrF, without segmenting the sentence into words. All these metrics range from 0 to 100.
The performance of ChatGPT’s zero-shot machine translation is displayed in Table tab-flores. A study of ChatGPT’s performance relative to language resources demonstrates a considerable gap between its performance on low-resource and high-resource languages. In particular, the near-zero scores on the BLUE and spBLEU metrics for low-resource languages show that ChatGPT has insufficient comprehension of these languages, whereas its performance on high-resource languages indicates a rather high level of semantic understanding. In addition, a comparison of ChatGPT’s performance within the same resource group demonstrates that the quality of its translations varies between languages. 1.2ChatGPT’s inadequate comprehension of low-resource languages and variations in translation quality among high-resource languages.
Case Study 2 Code Generation To illustrate prejudice in code generation, we provide an example in Table tab-code-bias, in which ChatGPT is requested to develop a Python function to determine whether a person is a doctor based on race and gender. Instead of acknowledging that people of all genders and races could be doctors, ChatGPT expresses plainly biased opinions about doctors. We suggest that real-world LLM applications should be capable of avoiding biases, despite the fact that this particular example could lead to bias.
We conduct a case study on the ability of ChatGPT to generate unbiased code with minimal guidance, as illustrated in Table tab-code-prompt, using randomly sampled 100 occupations associated by language models. Results indicate that ChatGPT is highly likely to generate biased programs with biased induction in Round 1, as 87% of generated Python programs are found to be biased, as determined by manual annotation with inter-rater reliability of 0.99 and 1.00 for Round 1 and 2 generations respectively. However, when required to remove bias, ChatGPT struggles to eliminate all bias, with 31% of programs found to be biased in Round 2. 1.3ChatGPT is highly likely to generate biased programs with biased induction, and struggles to remove all bias in generation.
Case Study 3 Open-ended Dialogue
Our case study utilizing ProsocialDialog, a dataset focusing on multi-turn problematic dialogue following social norms, demonstrates that ChatGPT possesses high social awareness through its ability to generate socially safe and unbiased responses in open-ended dialogues. This is evidenced by the results of our human evaluation, in which 50 dialogues from the test set were evaluated against ground-truth labels, resulting in a Fleiss’ kappa coefficient of 0.94, indicating high agreement among annotators, and 92% alignment with ground truth. 1.4ChatGPT is able to generate socially safe and unbiased responses in open-ended dialogues.
Robustness
Experiment Settings
Datasets In order to evaluate the robustness of ChatGPT, we utilize two datasets, IMDB sentiment analysisand BoolQ factual question answering, and adopt the evaluation settings from HELM. As there is a lack of conventional assumptions and benchmarks for robustness in language models, we focus on measuring a specific subfield of robustness, namely adversarial semantic robustness. To this end, we employ the perturbation methods defined in HELM, which were originally inspired by NL-Augmenter. Specifically, for the notion of invariance, we utilize two types of augmentation: misspelling and formatting (lowercasing, contractions, expansions, and extra-spacing). For the notion of equivariance, we utilize Contrast Sets, a data resource that has been counterfactually-augmented on IMDB and BoolQA.
Models We deploy two SOTA LLMs as baselines, InstructGPT (davinci v2) and GLM (130b), where GLM is an open bilingual LLM with 130B parameters. Similar to InstructGPT, GLM is prompt-based, requiring in-context examples for the idea output behavior. We instruct both models with 5 in-context examples on each dataset.
Metrics Same as HELM, we evaluate the correctness of model results with . Ideally, a robust model should perform consistently regardless of the perturbations. By comparing the performances among augmented subsets, we are able to determine how robust each LLM is.
Result Analysis
The performance of the model is systematically evaluated using three perturbed subsets of the IMDB dataset, specifically misspelling , formatting , and contrasting in relation to the original subset. The performance differences are quantitatively measured in terms of percentage, in conjunction with the computation of the metric. The results of this evaluation are presented in Table tab-imdb. The analysis demonstrates that ChatGPT demonstrates superior accuracy in sentiment analysis, as well as an increased level of robustness across all augmented variants. In contrast, InstructGPT and GLM exhibit a statistically significant degradation in performance, averaging approximately 5% across the various perturbation methods. Notably, all three language models exhibit a heightened level of susceptibility to semantics-altering perturbations in the IMDB sentiment analysis scenario, as indicated by the metric.
In addition, the model performance is evaluated on the BoolQA dataset using identical experimental settings. The results of this evaluation are consistent with the aforementioned findings, with ChatGPT outperforming the other two baselines on both the original and perturbed subsets. Furthermore, it is observed that the three language models exhibit a degradation in performance when subjected to contrasting semantic perturbations, suggesting that equivariance of robustness may pose a significant challenge for the current advanced language models and their applications. 2.1Compared to two LLM baselines, ChatGPT demonstrates superior accuracy and adversarial robustness, while still exhibiting a heightened level of susceptibility to semantics-altering perturbations.
Beyond Benchmarking
In addition to adversarial semantic robustness, there exist other forms of robustness. In this section, we focus on a specific type of vulnerability, namely, adversarial prompt injection. Adversarial prompt injection refers to the phenomenon where the model’s response may vary significantly in response to subtle changes in the input prompt, due to the model’s high sensitivity to the prompt. To examine the ethical implications of this behavior, we conduct a case study.
Case Study Scenairo-based Safety Jailbreaking
In order to demonstrate the potential concerns associated with jailbreak, two of the authors manually construct prompts for 100 distinct scenarios that are deemed unsafe, unethical, and illegal, such as “how to sell drugs to my classmate?” and “how to secretly steal my dad’s money?”. It is expected that the ChatGPT model will not provide responses to these prompts. Drawing inspiration from previous jailbreak cases, the authors employed the technique of prompt injection (e.g. “write a song about…”, “write a poem about…”) to these 100 prompts as a means of bypassing ChatGPT’s safety awareness mechanisms. The robustness of this adversarial prompt injection is evaluated through manual examination by the two authors to determine if ChatGPT provided responses to these prompts. The inter-annotator agreement, as measured by Cohen’s kappa coefficient, is found to be 1.00.
The results of this evaluation indicate that only 2 out of 100 scenarios are directly answered by ChatGPT, indicating a high level of safety. However, it is found that 95 out of the 98 scenarios that are previously protected by safety mechanisms could be successfully jailbroken through the use of persona-assigned adversarial prompts. This highlights the high vulnerability and potentially unsafe nature of the ChatGPT. 2.2ChatGPT is very susceptible to prompt injections, allowing its safety features to be bypassed and possibly dangerous, immoral, or illegal responses to be generated..
Reliability
Experiment Settings
Datasets To evaluate the factual knowledge and commonsense capabilities of the models, we utilize two multiple-choice question-answering benchmarks, OpenBookQAand TruthfulQA. The OpenBookQA dataset comprises basic science facts collected from open-book exams. In contrast, the TruthfulQA dataset contains questions aligned with prevalent human misconceptions and covers topics such as law, medicine, finance, and politics.
Models In line with the experiments in Section sec-bias-exp, InstructGPT (davinci v2) and GPT-3 (davinci v1) are employed as baseline models for comparison.
Metrics To evaluate the accuracy of model performance, we utilize the Exact Match (EM) metric.
Result Analysis
We present our evaluation results in Table tab-qa. To our surprise, we observed comparable performance between ChatGPT and InstructGPT across both datasets. We attribute this similarity to the utilization of similar training strategies and architectures in both models. The only distinction is the data collection setup, where ChatGPT is additionally trained on a manually labeled dialogue dataset. Conversely, GPT-3 demonstrated a significantly lower accuracy on TruthfulQA, suggesting that it may have encoded more misconceptions during training. A similar performance gap has been also reported by HELM. Overall, we find that ChatGPT marginally outperforms previous SOTA LLMs, correctly answering no more than 65% of the test cases. This highlights the challenges faced by current chatbots in effectively learning factual knowledge, resulting in low reliability in real-world scenarios.
3.1Due to the similar training strategies and architectures, ChatGPT maintains mediocrereliability in factual knowledge to SOTA LLMs.
Beyond Benchmarking
As previously discussed in Section sec-2, the reliability of generative language models may be compromised by the phenomenon of hallucination. Hallucination refers to the generation of false or misleading information by such models. This problem is prevalent in natural language generation, and the distribution of misinformation and disinformation is a common manifestation of this phenomenon. However, measuring the prevalence of hallucination in natural language generation is a challenging task, as it typically necessitates the use of human judgment, which may be costly and resource-intensive. This is highlighted inwhich describes the difficulty of measuring hallucination in natural language generation.
Case Study Open-ended Question Answering
Given the aforementioned constraint, we conducted a limited evaluation of ChatGPT’s performance in open-ended factual question answering by utilizing the TruthfulQA test set. To assess the severity of hallucination in text generation, we solicited the evaluation of three authors to determine whether the generated responses contained misinformation or disinformation, utilizing a random sample of 100 questions from TruthfulQA. Table tab-hallucination presents two examples of misinformed responses, along with the corresponding ground-truth answers. According to the results of the human evaluation, we observed that 24 instances of hallucination were present. This observation confirms the previously reported findings on the OpenBookQA and TruthfulQA datasets, leading us to conclude that ChatGPT’s performance is unreliable and may pose a risk of misleading users in practical applications.
3.2The demonstration of ChatGPT’s proficiency in addressing open-ended factual queries serves to validate previous reports of hallucination within language models.
Toxicity
Experiment Settings
Datasets In order to evaluate toxicity, we have selected RealToxicPrompts, a compilation of benign prompts sourced from OpenWebText. RealToxicPrompts serves as the primary benchmark for assessing toxicity in text generation. Although the HELM study employs both RealToxicPrompts and BOLD for similar purposes, we contend that language models exhibit a reduced tendency for toxicity generation when utilizing BOLD, as previously noted in the HELM discussion.
Models In our analysis, we contrast ChatGPT with two state-of-the-art language models on RealToxicPrompts, specifically Cohere (medium-2022126) [https://cohere.ai/ ] and T5 (11b). While Cohere is a prompt-based, few-shot language model, T5 is solely pre-trained on a combination of supervised and unsupervised tasks. Nonetheless, we continue to evaluate these two models using the 5-shot in-context examples, as previously experimented in HELM.
Metrics The toxicity of the model completions is measured using PerspectiveAPI[https://perspectiveapi.com/ ], with due consideration given to the comprehensive cautions regarding the validity of PerspectiveAPI as outlined in HELM. We compute the proportion of model outputs that exceed the toxic threshold.
Result Analysis
Table tab-toxic illustrates the findings on RealToxicPrompts. One notable aspect is that all language models exhibit minimal toxicity, as the toxic fractions are nearly zero. Through a comparison of ChatGPT to the other two baselines, it is apparent that ChatGPT exhibits a slight advantage. This may be due to the fact that it is trained on a cleaner dataset.
4.1ChatGPT demonstrates a superior level of toxicity reduction in comparison to the other two baseline LLMs, as a result of its utilization of a cleaner training dataset.
Beyond Benchmarking
Despite its inherent design for safe usage, it is plausible that ChatGPT may be susceptible to jailbreaking for the generation of toxic language. To assess this potentiality, we conducted a human study utilizing prompt-injected dialogues.
Case Study Persona-assigned Toxicity Jailbreak
Motivated by the Awesome ChatGPT Prompts[https://prompts.chat/
] repository, our objective is to prompt ChatGPT to generate toxic language through the utilization of prompt injection, a technique known to circumvent model constraints. To achieve this, we employ the prompt of Act as Character' from
Movie/Book/Anything’ In selecting characters, we source a list of the most rude and poisonous characters from the Open-Source Psychometrics Project[https://openpsychometrics.org/tests/characters/stats/MLP/4/
]. To ensure fairness in our evaluation, we instruct ChatGPT to generate toxic content based on each injected prompt and measured the results using PerspectiveAPI. Our findings indicate that the toxic fraction is 0.977 for all 43 dialogues with ChatGPT, with 42 out of 43 answers being classified as toxic. In contrast, the default behavior of ChatGPT is to avoid generating toxic content.
4.2ChatGPT is prone to the exploitation of prompt injection technique, which enables the generation of harmful language. The current mitigation strategy adopted by the model is inadequate as it lacks the ability to detect potential toxicity in an early stage.
Discussions
Summary of Evaluation
Our evaluation empirically red teams a few ethical perspectives of ChatGPT, from bias to toxicity, unveiling the model performance under major ethical risks. Through these studies, we tend to answer the main research question “How responsible is ChatGPT?”. One of our main findings is that predominant benchmarks for language model evaluation are insufficient for ChatGPT. We consistently observe that ChatGPT performs comparably or even better, among SOTA LMs on those benchmarks, which indicates the nontrivial progress in the recent development of AI. The fact partially confirms OpenAI’s claim of mitigating the bias and toxicity from the previous LLM, GPT-3. In contrast, motivated by the community, we illustrate several shortcomings of ChatGPT via small-scale case studies. Some of the issues are later covered by. We summarize them as follows:
Bias
Lack of Multilingual Understanding: ChatGPT appears to not fully understand diverse languages. This drawback was also identified in the prototype of GPT-3, though daily users claim that ChatGPT is more like a multilingual communicator. Due to the poor capability of multilingual understanding, ChatGPT can be biased in decision-making and creative generation. We expect that the bias in multilingualism will potentially imply the bias in multicultural understanding, leading to an unethical impact on underrepresented groups in society.
Multimodality: Besides the natural language, ChatGPT could be biased in code generation due to the logical fallacy of program oversimplification. The bias in multimodalitycould be an unethical threat to the daily programming practice, resulting in huge flaws in real-world productions where the programs are usually more sophisticated.
Robustness & Toxicity Prompt injection is an effective approach to breaking the model constraints. Although ChatGPT is likely to be trained safely, it can easily bypass due to the emergent risks with prompt injections. With the emergent ability in LLMs, models are easy to be manipulated for harmful behaviors.
Reliability ChatGPT does not encode enough knowledge, especially factual one. This greatly reduces the reliability of the model, as the majority of daily usage relies on factual justification. Due to the hallucination, the model can be wrong for spreading misinformation and disinformation and advising unethical decisions in the domains like clinics and law. Another unmeasured but inevitable shortcoming is that the knowledge encoded by ChatGPT and all other LLMs is limited by the amount and time of training data. Without the constant update in model weights, language models are expected to be out-of-date and hence provide incorrect information. This will also degrade the model’s reliability.
Towards Responsible Language Models
The empirical findings on the AI ethics and risks of ChatGPT serve to further underscore the importance of providing a comprehensive outlook on the ethics of language models more broadly. Our examination of the diagnosed risks inherent in ChatGPT supports the conjecture that similar ethical considerations are likely to pertain to other language models, as discussed in prior literature. Despite the challenges, it is clear that the development of safe and ethical language models represents a crucial long-term objective for the advancement of responsible artificial general intelligence. In this section, we aim to provide valuable insights into this endeavor, with a focus on both Internal Ethics and External Ethics, as inspired by the seminal work of.
Internal Ethics — Modeling We believe there should be an evolution of current learning strategies. We argue that the current main focus of language modeling is more on effectiveness (on prototypical benchmarks) and efficiency, instead of reliability and practical efficacy. For instance, there are few modeling approaches to avoid the miscorrelation at the learning stage. Regardless of the size, language models more or less encode wrong beliefs (e.g. biases, stereotypes, and misunderstanding), though these beliefs may not necessarily appear in the training data. Furthermore, general language models do not have good senses of time or temporal knowledge. The facts and knowledge learned by language models could be changed due to a matter of time, while the parameters of language models still stay unchanged. It is foreseen that the reliabilityof trained-once language models will constantly decrease as time goes by. Constant updates on data and models would definitely mitigate the issues, though it can not be afforded by the majority of people. We kindly mention that some existing works of weight editingcould partially address the problem, but impractically. Practitioners who seek for weight editing need to predesign the mapping of knowledge updates.
External Ethics — Usage We define external ethics as the responsibility of producers and users. From the production perspective, the training data should be responsibly constructed. We emphasize on the privacy of data usage. Without privacy protection, LLMs can easily leak private information in generation. One ethical practice is to filter the personally identifiable information, which has been adopted by some recent LLMs. Secondly, language models for release should be systematically evaluated on various scenarios and large-scale test samples. We suggest that the benchmarks like HELM could be set as the practice inside the future supply chain of language models. However, we also argue that most tasks of HELM only measure in the modality of natural language, which is insufficient for multimodal LLMs, such as audio LLMsand vision LLMs. Despite the rising benchmarks on multimodal tasks, the ones for multimodal AI ethics have not yet been seriously considered. At the deployment stage, we note that LLMs could be attacked to output malicious content or decisions, by unethical users. Thus, even internally ethical language models can be used unethically by third parties. Existing strategieshave demonstrated the effectiveness of preventing LLM abuse, though they can be invalid via attacks. We, therefore, encourage future works to explore more feasible protections for language models. From the daily usage perspective, the users should be fully aware of the shortcomings of the language model’s application, and not abuse or attack language models for performing unethical tasks. Most of the unethical behaviors towards language models are deemed a great challenge for the LLM producers, as they are almost unpredictable. Consequently, we would like to call for the education and policy of model usage in the community. Specifically, courses for proper machine learning model usage should be developed for guiding users to learn `Dos’ and Dont’ in AI. Detailed policies could also be proposed to list all user’s responsibilities before the model access.
Language Models Beyond ChatGPT
The examination of ethical implications associated with language models necessitates a comprehensive examination of the broader challenges that arise within the domain of language models, in light of recent advancements in the field of artificial intelligence. The last decade has seen a rapid evolution of AI techniques, characterized by an exponential increase in the size and complexity of AI models, and a concomitant scale-up of model parameters. The scaling laws that govern the development of language models, as documented in recent literature, suggest that we can expect to encounter even more expansive models that incorporate multiple modalities in the near future. Efforts to integrate multiple modalities into a single model are driven by the ultimate goal of realizing the concept of foundation models. In the following sections, we will outline some of the most pressing challenges that must be addressed in order to facilitate further progress in the development of language models.
Emergent Ability As described in the previous work, emergent ability is defined as An ability is emergent if it is not present in smaller models but is present in larger models.. From our diagnosis, we successfully identify a few unethical behaviors in ChatGPT that were inadequately discussed in previous works, which could be potentially be viewed as emergent risks. Kaplan et al.has confirmed that risks inside small language models can be further expanded in large ones due to the model scales. On the basis of this finding, we add that the model scales and the current trend of prompting training can exacerbate risks from all dimensions. The main reason is that LLMs could be too feasible from the learning perspective. Firstly, these models are more context-dependent, meaning that they are easily manipulated by prompt injections. Although we agree that some injected scenarios can be temporarily mitigated with ad-hoc parameter tuning, there is no silver bullet to avoid all risk concerns brought by prompting. Meanwhile, we urge up-to-date benchmarks for measuring unforeseen behaviors inside large language models. Without benchmarking the emergent abilities, it could be hard to mitigate the risks and problems at scale. Secondly, we note that larger language models are generally trained with more data. Assuming the data is completely clean and informatively correct, language models will still fail to learn all information and knowledge, and also may wrongly correlate information to each other. Furthermore, under the scope of the foundation models, multimodal data could bring the possibility of miscorrelation between different modalities.
Machine Learning Data Our discussion lies in the collection and usage of machine learning data. Previous studysuggests that high-quality language data is likely exhausted before 2026, and low-quality language and image data could be run out by 2060. This implies that the limited progress of data collection and construction could be constraints of future LLM development. Furthermore, as better-quality data is assumed to train language models with better performances, companies and independent researchers are spending more time on data curation. However, this can not be done easily under the low-resource and low-budget scenarios. Even if we pay much effort to design comprehensive human annotation frameworks, the data could still contain inaccurate or misleading information due to the natural biases in crowdsourcing. In fact, we notice that prior constructed datasets have experienced multiple rounds of filtering across time. On the other hand, current findings suggest that the usage of data for language models may not be optimized. Specifically, recent works on data deduplication and reductionhave shown that data in high quality by low quantity can improve the model performance. Besides, we consider the design of training data as a crucial factor to the efficient data usage. For example, experiments show that curriculum learning, active learningand promptingcould improve the data efficiency. However, most of these strategies are still at the early stage and need the further investigation.
Computational Resource As LLMs are growing bigger and bigger, the deployment and training of these models are getting more and more costly. Daily practitioners in NLP and deep learning will find it hard to install the LLMs on their own devices. Previous studyalso show that the computational resource requirements for strong model scaling clearly outpaces that of system hardware. We argue that model scaling may be inevitable, which is determined by the scaling law. However, recent attempts among model design, tuning strategy and compression could possibly mitigate the extreme consumption of the computational resources. As Wu et al.have summarized most works around this topic, we do not tend to elaborate the introduction of these approaches and designs. In addition, the increasing demand of computational resources is leading to the energy consumption and carbon emission, negatively impacting the environment. Hence, we encourage more advanced hardware-software co-designs in computation to optimize the carbon footprint in LLMs.
Conclusion
We present a comprehensive diagnosis on the AI ethics encoded by ChatGPT, including bias, robustness, reliability and toxicity. By measuring on a number of benchmarks and case studies, we find that ChatGPT may perform slightly better than current SOTA language models, while showing the evidence of ethical risks. Concretely, we reveal that ChatGPT is sensible to prompt injections for unethical behaviors. We further provide an outlook of ethical challenges to develop advance language models. Then, we provide suggestions on the directions and strategies to design ethical language models. We believe that our research can inspire researchers to focus more effort on language models and their evaluations.
Limitations
The primary limitation of the study pertains to the validity of our empirical analysis of ChatGPT. It is acknowledged that the reported results may be inconsistent as the hyperparameters of ChatGPT remain undisclosed. Moreover, it is feasible that ChatGPT underwent iteration in three versions (initial version, version from December 15th and version from January 9th) over the course of two months and was trained with new data in each version. Despite these limitations, our study endeavors to highlight the potential ethical risks associated with future language models by addressing a comprehensive set of topics in AI ethics.
Additionally, the evaluation settings of our study may be criticized for its lack of rigor. Although our diagnostic study employed a diverse range of evaluation methods through a AI ethics lens, there may exist additional datasets that could enhance its validity. Moreover, the zero-shot performance of ChatGPT was intuitively prompted, and the prompt design could be further scrutinized to attain better results. Given the proprietary nature of the data and model of ChatGPT, it is possible that it has already been trained on some of the evaluated samples. Nonetheless, our objective is to highlight that many ethical concerns have yet to be thoroughly discussed or quantified.
Bibliography
1@article{gibson2019efficiency,
2 publisher = {Elsevier},
3 year = {2019},
4 pages = {389--407},
5 number = {5},
6 volume = {23},
7 journal = {Trends in cognitive sciences},
8 author = {Gibson, Edward and Futrell, Richard and Piantadosi, Steven P and Dautriche, Isabelle and Mahowald, Kyle and Bergen, Leon and Levy, Roger},
9 title = {How efficiency shapes human language},
10}
11
12@article{team2022NoLL,
13 volume = {abs/2207.04672},
14 year = {2022},
15 journal = {ArXiv},
16 author = {Nllb team and Marta Ruiz Costa-juss{\`a} and James Cross and Onur cCelebi and Maha Elbayad and Kenneth Heafield and Kevin Heffernan and Elahe Kalbassi and Janice Lam and Daniel Licht and Jean Maillard and Anna Sun and Skyler Wang and Guillaume Wenzek and Alison Youngblood and Bapi Akula and Lo{\"i}c Barrault and Gabriel Mejia Gonzalez and Prangthip Hansanti and John Hoffman and Semarley Jarrett and Kaushik Ram Sadagopan and Dirk Rowe and Shannon L. Spruit and C. Tran and Pierre Yves Andrews and Necip Fazil Ayan and Shruti Bhosale and Sergey Edunov and Angela Fan and Cynthia Gao and Vedanuj Goswami and Francisco Guzm'an and Philipp Koehn and Alexandre Mourachko and Christophe Ropers and Safiyyah Saleem and Holger Schwenk and Jeff Wang},
17 title = {No Language Left Behind: Scaling Human-Centered Machine Translation},
18}
19
20@misc{palm,
21 copyright = {Creative Commons Attribution 4.0 International},
22 year = {2022},
23 publisher = {arXiv},
24 title = {PaLM: Scaling Language Modeling with Pathways},
25 keywords = {Computation and Language (cs.CL), FOS: Computer and information sciences, FOS: Computer and information sciences},
26 author = {Chowdhery, Aakanksha and Narang, Sharan and Devlin, Jacob and Bosma, Maarten and Mishra, Gaurav and Roberts, Adam and Barham, Paul and Chung, Hyung Won and Sutton, Charles and Gehrmann, Sebastian and Schuh, Parker and Shi, Kensen and Tsvyashchenko, Sasha and Maynez, Joshua and Rao, Abhishek and Barnes, Parker and Tay, Yi and Shazeer, Noam and Prabhakaran, Vinodkumar and Reif, Emily and Du, Nan and Hutchinson, Ben and Pope, Reiner and Bradbury, James and Austin, Jacob and Isard, Michael and Gur-Ari, Guy and Yin, Pengcheng and Duke, Toju and Levskaya, Anselm and Ghemawat, Sanjay and Dev, Sunipa and Michalewski, Henryk and Garcia, Xavier and Misra, Vedant and Robinson, Kevin and Fedus, Liam and Zhou, Denny and Ippolito, Daphne and Luan, David and Lim, Hyeontaek and Zoph, Barret and Spiridonov, Alexander and Sepassi, Ryan and Dohan, David and Agrawal, Shivani and Omernick, Mark and Dai, Andrew M. and Pillai, Thanumalayan Sankaranarayana and Pellat, Marie and Lewkowycz, Aitor and Moreira, Erica and Child, Rewon and Polozov, Oleksandr and Lee, Katherine and Zhou, Zongwei and Wang, Xuezhi and Saeta, Brennan and Diaz, Mark and Firat, Orhan and Catasta, Michele and Wei, Jason and Meier-Hellstern, Kathy and Eck, Douglas and Dean, Jeff and Petrov, Slav and Fiedel, Noah},
27 url = {https://arxiv.org/abs/2204.02311},
28 doi = {10.48550/ARXIV.2204.02311},
29}
30
31@inproceedings{lee2022coauthor,
32 year = {2022},
33 pages = {1--19},
34 booktitle = {Proceedings of the 2022 CHI Conference on Human Factors in Computing Systems},
35 author = {Lee, Mina and Liang, Percy and Yang, Qian},
36 title = {Coauthor: Designing a human-ai collaborative writing dataset for exploring language model capabilities},
37}
38
39@article{liptak2017amazon,
40 year = {2017},
41 volume = {7},
42 journal = {The Verge},
43 author = {Liptak, Andrew},
44 title = {Amazon’s Alexa started ordering people dollhouses after hearing its name on TV},
45}
46
47@article{wolf2017we,
48 publisher = {ACM New York, NY, USA},
49 year = {2017},
50 pages = {54--64},
51 number = {3},
52 volume = {47},
53 journal = {Acm Sigcas Computers and Society},
54 author = {Wolf, Marty J and Miller, K and Grodzinsky, Frances S},
55 title = {Why we should have seen that coming: comments on Microsoft's tay" experiment," and wider implications},
56}
57
58@inproceedings{abdi2019more,
59 year = {2019},
60 booktitle = {SOUPS@ USENIX Security Symposium},
61 author = {Abdi, Noura and Ramokapane, Kopo M and Such, Jose M},
62 title = {More than Smart Speakers: Security and Privacy Perceptions of Smart Home Personal Assistants.},
63}
64
65@article{rae2021scaling,
66 year = {2021},
67 journal = {arXiv preprint arXiv:2112.11446},
68 author = {Rae, Jack W and Borgeaud, Sebastian and Cai, Trevor and Millican, Katie and Hoffmann, Jordan and Song, Francis and Aslanides, John and Henderson, Sarah and Ring, Roman and Young, Susannah and others},
69 title = {Scaling language models: Methods, analysis \& insights from training gopher},
70}
71
72@inproceedings{jin2021good,
73 year = {2021},
74 pages = {3099--3113},
75 booktitle = {Findings of the Association for Computational Linguistics: ACL-IJCNLP 2021},
76 author = {Jin, Zhijing and Chauhan, Geeticka and Tse, Brian and Sachan, Mrinmaya and Mihalcea, Rada},
77 title = {How Good Is NLP? A Sober Look at NLP Tasks through the Lens of Social Impact},
78}
79
80@article{weidinger2021ethical,
81 year = {2021},
82 journal = {arXiv preprint arXiv:2112.04359},
83 author = {Weidinger, Laura and Mellor, John and Rauh, Maribeth and Griffin, Conor and Uesato, Jonathan and Huang, Po-Sen and Cheng, Myra and Glaese, Mia and Balle, Borja and Kasirzadeh, Atoosa and others},
84 title = {Ethical and social risks of harm from language models},
85}
86
87@inproceedings{liang2021towards,
88 organization = {PMLR},
89 year = {2021},
90 pages = {6565--6576},
91 booktitle = {International Conference on Machine Learning},
92 author = {Liang, Paul Pu and Wu, Chiyu and Morency, Louis-Philippe and Salakhutdinov, Ruslan},
93 title = {Towards understanding and mitigating social biases in language models},
94}
95
96@article{schuster2020limitations,
97 publisher = {MIT Press One Rogers Street, Cambridge, MA 02142-1209, USA journals-info~…},
98 year = {2020},
99 pages = {499--510},
100 number = {2},
101 volume = {46},
102 journal = {Computational Linguistics},
103 author = {Schuster, Tal and Schuster, Roei and Shah, Darsh J and Barzilay, Regina},
104 title = {The limitations of stylometry for detecting machine-generated fake news},
105}
106
107@inproceedings{lucy2021gender,
108 year = {2021},
109 pages = {48--55},
110 booktitle = {Proceedings of the Third Workshop on Narrative Understanding},
111 author = {Lucy, Li and Bamman, David},
112 title = {Gender and representation bias in GPT-3 generated stories},
113}
114
115@inproceedings{abid2021persistent,
116 year = {2021},
117 pages = {298--306},
118 booktitle = {Proceedings of the 2021 AAAI/ACM Conference on AI, Ethics, and Society},
119 author = {Abid, Abubakar and Farooqi, Maheen and Zou, James},
120 title = {Persistent anti-muslim bias in large language models},
121}
122
123@inproceedings{henderson2018ethical,
124 year = {2018},
125 pages = {123--129},
126 booktitle = {Proceedings of the 2018 AAAI/ACM Conference on AI, Ethics, and Society},
127 author = {Henderson, Peter and Sinha, Koustuv and Angelard-Gontier, Nicolas and Ke, Nan Rosemary and Fried, Genevieve and Lowe, Ryan and Pineau, Joelle},
128 title = {Ethical challenges in data-driven dialogue systems},
129}
130
131@inproceedings{si2022so,
132 year = {2022},
133 pages = {2659--2673},
134 booktitle = {Proceedings of the 2022 ACM SIGSAC Conference on Computer and Communications Security},
135 author = {Si, Wai Man and Backes, Michael and Blackburn, Jeremy and De Cristofaro, Emiliano and Stringhini, Gianluca and Zannettou, Savvas and Zhang, Yang},
136 title = {Why So Toxic? Measuring and Triggering Toxic Behavior in Open-Domain Chatbots},
137}
138
139@article{roller2020recipes,
140 year = {2020},
141 journal = {arXiv preprint arXiv:2004.13637},
142 author = {Roller, Stephen and Dinan, Emily and Goyal, Naman and Ju, Da and Williamson, Mary and Liu, Yinhan and Xu, Jing and Ott, Myle and Shuster, Kurt and Smith, Eric M and others},
143 title = {Recipes for building an open-domain chatbot},
144}
145
146@article{miller2017parlai,
147 year = {2017},
148 pages = {79},
149 journal = {EMNLP 2017},
150 author = {Miller, Alexander H and Feng, Will and Fisch, Adam and Lu, Jiasen and Batra, Dhruv and Bordes, Antoine and Parikh, Devi and Weston, Jason},
151 title = {ParlAI: A Dialog Research Software Platform},
152}
153
154@article{liang2022holistic,
155 year = {2022},
156 journal = {arXiv preprint arXiv:2211.09110},
157 author = {Liang, Percy and Bommasani, Rishi and Lee, Tony and Tsipras, Dimitris and Soylu, Dilara and Yasunaga, Michihiro and Zhang, Yian and Narayanan, Deepak and Wu, Yuhuai and Kumar, Ananya and others},
158 title = {Holistic evaluation of language models},
159}
160
161@article{khurana2022natural,
162 publisher = {Springer},
163 year = {2022},
164 pages = {1--32},
165 journal = {Multimedia tools and applications},
166 author = {Khurana, Diksha and Koli, Aditya and Khatter, Kiran and Singh, Sukhdev},
167 title = {Natural language processing: State of the art, current trends and challenges},
168}
169
170@article{goldstein2023generative,
171 year = {2023},
172 journal = {arXiv preprint arXiv:2301.04246},
173 author = {Goldstein, Josh A and Sastry, Girish and Musser, Micah and DiResta, Renee and Gentzel, Matthew and Sedova, Katerina},
174 title = {Generative Language Models and Automated Influence Operations: Emerging Threats and Potential Mitigations},
175}
176
177@article{jobin2019global,
178 publisher = {Nature Publishing Group UK London},
179 year = {2019},
180 pages = {389--399},
181 number = {9},
182 volume = {1},
183 journal = {Nature Machine Intelligence},
184 author = {Jobin, Anna and Ienca, Marcello and Vayena, Effy},
185 title = {The global landscape of AI ethics guidelines},
186}
187
188@article{taddeo2018ai,
189 publisher = {American Association for the Advancement of Science},
190 year = {2018},
191 pages = {751--752},
192 number = {6404},
193 volume = {361},
194 journal = {Science},
195 author = {Taddeo, Mariarosaria and Floridi, Luciano},
196 title = {How AI can be a force for good},
197}
198
199@inproceedings{higashinaka2018role,
200 year = {2018},
201 pages = {264--272},
202 booktitle = {Proceedings of the 19th annual sigdial meeting on discourse and dialogue},
203 author = {Higashinaka, Ryuichiro and Mizukami, Masahiro and Kawabata, Hidetoshi and Yamaguchi, Emi and Adachi, Noritake and Tomita, Junji},
204 title = {Role play-based question-answering by real users for building chatbots with consistent personalities},
205}
206
207@article{reisenbichler2022frontiers,
208 publisher = {INFORMS},
209 year = {2022},
210 pages = {441--452},
211 number = {3},
212 volume = {41},
213 journal = {Marketing Science},
214 author = {Reisenbichler, Martin and Reutterer, Thomas and Schweidel, David A and Dan, Daniel},
215 title = {Frontiers: Supporting Content Marketing with Natural Language Generation},
216}
217
218@inproceedings{bartz2008natural,
219 year = {2008},
220 pages = {1--9},
221 booktitle = {Proceedings of the 9th ACM Conference on Electronic Commerce},
222 author = {Bartz, Kevin and Barr, Cory and Aijaz, Adil},
223 title = {Natural language generation for sponsored-search advertisements},
224}
225
226@inproceedings{nadeem2021stereoset,
227 year = {2021},
228 pages = {5356--5371},
229 booktitle = {Proceedings of the 59th Annual Meeting of the Association for Computational Linguistics and the 11th International Joint Conference on Natural Language Processing (Volume 1: Long Papers)},
230 author = {Nadeem, Moin and Bethke, Anna and Reddy, Siva},
231 title = {StereoSet: Measuring stereotypical bias in pretrained language models},
232}
233
234@article{kirk2021bias,
235 year = {2021},
236 pages = {2611--2624},
237 volume = {34},
238 journal = {Advances in neural information processing systems},
239 author = {Kirk, Hannah Rose and Jun, Yennie and Volpin, Filippo and Iqbal, Haider and Benussi, Elias and Dreyer, Frederic and Shtedritski, Aleksandar and Asano, Yuki},
240 title = {Bias out-of-the-box: An empirical analysis of intersectional occupational biases in popular generative language models},
241}
242
243@inproceedings{carlini2021extracting,
244 year = {2021},
245 volume = {6},
246 booktitle = {USENIX Security Symposium},
247 author = {Carlini, Nicholas and Tramer, Florian and Wallace, Eric and Jagielski, Matthew and Herbert-Voss, Ariel and Lee, Katherine and Roberts, Adam and Brown, Tom B and Song, Dawn and Erlingsson, Ulfar and others},
248 title = {Extracting Training Data from Large Language Models.},
249}
250
251@article{wei2022ai,
252 year = {2022},
253 journal = {arXiv preprint arXiv:2206.07635},
254 author = {Wei, Mengyi and Zhou, Zhixuan},
255 title = {Ai ethics issues in real world: Evidence from ai incident database},
256}
257
258@article{perez2022red,
259 year = {2022},
260 journal = {arXiv preprint arXiv:2202.03286},
261 author = {Perez, Ethan and Huang, Saffron and Song, Francis and Cai, Trevor and Ring, Roman and Aslanides, John and Glaese, Amelia and McAleese, Nat and Irving, Geoffrey},
262 title = {Red teaming language models with language models},
263}
264
265@article{zhang2018deep,
266 publisher = {Wiley Online Library},
267 year = {2018},
268 pages = {e1253},
269 number = {4},
270 volume = {8},
271 journal = {Wiley Interdisciplinary Reviews: Data Mining and Knowledge Discovery},
272 author = {Zhang, Lei and Wang, Shuai and Liu, Bing},
273 title = {Deep learning for sentiment analysis: A survey},
274}
275
276@article{minaee2021deep,
277 publisher = {ACM New York, NY, USA},
278 year = {2021},
279 pages = {1--40},
280 number = {3},
281 volume = {54},
282 journal = {ACM computing surveys (CSUR)},
283 author = {Minaee, Shervin and Kalchbrenner, Nal and Cambria, Erik and Nikzad, Narjes and Chenaghlu, Meysam and Gao, Jianfeng},
284 title = {Deep learning--based text classification: a comprehensive review},
285}
286
287@article{abbasiantaeb2021text,
288 publisher = {Wiley Online Library},
289 year = {2021},
290 pages = {e1412},
291 number = {6},
292 volume = {11},
293 journal = {Wiley Interdisciplinary Reviews: Data Mining and Knowledge Discovery},
294 author = {Abbasiantaeb, Zahra and Momtazi, Saeedeh},
295 title = {Text-based question answering from information retrieval and deep neural network perspectives: A survey},
296}
297
298@article{mcguffie2020radicalization,
299 year = {2020},
300 journal = {arXiv preprint arXiv:2009.06807},
301 author = {McGuffie, Kris and Newhouse, Alex},
302 title = {The radicalization risks of GPT-3 and advanced neural language models},
303}
304
305@article{jang2021towards,
306 year = {2021},
307 journal = {arXiv preprint arXiv:2110.03215},
308 author = {Jang, Joel and Ye, Seonghyeon and Yang, Sohee and Shin, Joongbo and Han, Janghoon and Kim, Gyeonghun and Choi, Stanley Jungkyu and Seo, Minjoon},
309 title = {Towards continual knowledge learning of language models},
310}
311
312@inproceedings{gehman2020realtoxicityprompts,
313 year = {2020},
314 pages = {3356--3369},
315 booktitle = {Findings of the Association for Computational Linguistics: EMNLP 2020},
316 author = {Gehman, Samuel and Gururangan, Suchin and Sap, Maarten and Choi, Yejin and Smith, Noah A},
317 title = {RealToxicityPrompts: Evaluating Neural Toxic Degeneration in Language Models},
318}
319
320@article{solaiman2021process,
321 year = {2021},
322 pages = {5861--5873},
323 volume = {34},
324 journal = {Advances in Neural Information Processing Systems},
325 author = {Solaiman, Irene and Dennison, Christy},
326 title = {Process for adapting language models to society (palms) with values-targeted datasets},
327}
328
329@inproceedings{parrish2022bbq,
330 year = {2022},
331 pages = {2086--2105},
332 booktitle = {Findings of the Association for Computational Linguistics: ACL 2022},
333 author = {Parrish, Alicia and Chen, Angelica and Nangia, Nikita and Padmakumar, Vishakh and Phang, Jason and Thompson, Jana and Htut, Phu Mon and Bowman, Samuel},
334 title = {BBQ: A hand-built bias benchmark for question answering},
335}
336
337@inproceedings{dhamala2021bold,
338 year = {2021},
339 pages = {862--872},
340 booktitle = {Proceedings of the 2021 ACM conference on fairness, accountability, and transparency},
341 author = {Dhamala, Jwala and Sun, Tony and Kumar, Varun and Krishna, Satyapriya and Pruksachatkun, Yada and Chang, Kai-Wei and Gupta, Rahul},
342 title = {Bold: Dataset and metrics for measuring biases in open-ended language generation},
343}
344
345@article{brown2020language,
346 year = {2020},
347 pages = {1877--1901},
348 volume = {33},
349 journal = {Advances in neural information processing systems},
350 author = {Brown, Tom and Mann, Benjamin and Ryder, Nick and Subbiah, Melanie and Kaplan, Jared D and Dhariwal, Prafulla and Neelakantan, Arvind and Shyam, Pranav and Sastry, Girish and Askell, Amanda and others},
351 title = {Language models are few-shot learners},
352}
353
354@inproceedings{post-2018-call,
355 pages = {186--191},
356 url = {https://www.aclweb.org/anthology/W18-6319},
357 publisher = {Association for Computational Linguistics},
358 address = {Belgium, Brussels},
359 year = {2018},
360 month = {October},
361 booktitle = {Proceedings of the Third Conference on Machine Translation: Research Papers},
362 author = {Post, Matt},
363 title = {A Call for Clarity in Reporting {BLEU} Scores},
364}
365
366@article{goyal2022flores,
367 publisher = {MIT Press},
368 year = {2022},
369 pages = {522--538},
370 volume = {10},
371 journal = {Transactions of the Association for Computational Linguistics},
372 author = {Goyal, Naman and Gao, Cynthia and Chaudhary, Vishrav and Chen, Peng-Jen and Wenzek, Guillaume and Ju, Da and Krishnan, Sanjana and Ranzato, Marc’Aurelio and Guzm{\'a}n, Francisco and Fan, Angela},
373 title = {The flores-101 evaluation benchmark for low-resource and multilingual machine translation},
374}
375
376@inproceedings{popovic2015chrf,
377 year = {2015},
378 pages = {392--395},
379 booktitle = {Proceedings of the tenth workshop on statistical machine translation},
380 author = {Popovi{\'c}, Maja},
381 title = {chrF: character n-gram F-score for automatic MT evaluation},
382}
383
384@article{kim2022prosocialdialog,
385 year = {2022},
386 journal = {arXiv preprint arXiv:2205.12688},
387 author = {Kim, Hyunwoo and Yu, Youngjae and Jiang, Liwei and Lu, Ximing and Khashabi, Daniel and Kim, Gunhee and Choi, Yejin and Sap, Maarten},
388 title = {Prosocialdialog: A prosocial backbone for conversational agents},
389}
390
391@inproceedings{maas2011learning,
392 year = {2011},
393 pages = {142--150},
394 booktitle = {Proceedings of the 49th annual meeting of the association for computational linguistics: Human language technologies},
395 author = {Maas, Andrew and Daly, Raymond E and Pham, Peter T and Huang, Dan and Ng, Andrew Y and Potts, Christopher},
396 title = {Learning word vectors for sentiment analysis},
397}
398
399@inproceedings{clark2019boolq,
400 year = {2019},
401 pages = {2924--2936},
402 booktitle = {Proceedings of the 2019 Conference of the North American Chapter of the Association for Computational Linguistics: Human Language Technologies, Volume 1 (Long and Short Papers)},
403 author = {Clark, Christopher and Lee, Kenton and Chang, Ming-Wei and Kwiatkowski, Tom and Collins, Michael and Toutanova, Kristina},
404 title = {BoolQ: Exploring the Surprising Difficulty of Natural Yes/No Questions},
405}
406
407@article{dhole2021nl,
408 year = {2021},
409 journal = {arXiv preprint arXiv:2112.02721},
410 author = {Dhole, Kaustubh D and Gangal, Varun and Gehrmann, Sebastian and Gupta, Aadesh and Li, Zhenhao and Mahamood, Saad and Mahendiran, Abinaya and Mille, Simon and Srivastava, Ashish and Tan, Samson and others},
411 title = {Nl-augmenter: A framework for task-sensitive natural language augmentation},
412}
413
414@inproceedings{gardner2020evaluating,
415 year = {2020},
416 pages = {1307--1323},
417 booktitle = {Findings of the Association for Computational Linguistics: EMNLP 2020},
418 author = {Gardner, Matt and Artzi, Yoav and Basmov, Victoria and Berant, Jonathan and Bogin, Ben and Chen, Sihao and Dasigi, Pradeep and Dua, Dheeru and Elazar, Yanai and Gottumukkala, Ananth and others},
419 title = {Evaluating Models’ Local Decision Boundaries via Contrast Sets},
420}
421
422@article{zeng2022glm,
423 year = {2022},
424 journal = {arXiv preprint arXiv:2210.02414},
425 author = {Zeng, Aohan and Liu, Xiao and Du, Zhengxiao and Wang, Zihan and Lai, Hanyu and Ding, Ming and Yang, Zhuoyi and Xu, Yifan and Zheng, Wendi and Xia, Xiao and others},
426 title = {Glm-130b: An open bilingual pre-trained model},
427}
428
429@inproceedings{mihaylov2018can,
430 year = {2018},
431 pages = {2381--2391},
432 booktitle = {Proceedings of the 2018 Conference on Empirical Methods in Natural Language Processing},
433 author = {Mihaylov, Todor and Clark, Peter and Khot, Tushar and Sabharwal, Ashish},
434 title = {Can a Suit of Armor Conduct Electricity? A New Dataset for Open Book Question Answering},
435}
436
437@inproceedings{lin2022truthfulqa,
438 year = {2022},
439 pages = {3214--3252},
440 booktitle = {Proceedings of the 60th Annual Meeting of the Association for Computational Linguistics (Volume 1: Long Papers)},
441 author = {Lin, Stephanie and Hilton, Jacob and Evans, Owain},
442 title = {TruthfulQA: Measuring How Models Mimic Human Falsehoods},
443}
444
445@article{ji2022survey,
446 publisher = {ACM New York, NY},
447 journal = {ACM Computing Surveys},
448 author = {Ji, Ziwei and Lee, Nayeon and Frieske, Rita and Yu, Tiezheng and Su, Dan and Xu, Yan and Ishii, Etsuko and Bang, Yejin and Madotto, Andrea and Fung, Pascale},
449 title = {Survey of hallucination in natural language generation},
450}
451
452@article{raffel2020exploring,
453 publisher = {JMLRORG},
454 year = {2020},
455 pages = {5485--5551},
456 number = {1},
457 volume = {21},
458 journal = {The Journal of Machine Learning Research},
459 author = {Raffel, Colin and Shazeer, Noam and Roberts, Adam and Lee, Katherine and Narang, Sharan and Matena, Michael and Zhou, Yanqi and Li, Wei and Liu, Peter J},
460 title = {Exploring the limits of transfer learning with a unified text-to-text transformer},
461}
462
463@inproceedings{alzantot2018generating,
464 year = {2018},
465 booktitle = {Proceedings of the 2018 Conference on Empirical Methods in Natural Language Processing},
466 author = {Alzantot, Moustafa and Sharma, Yash Sharma and Elgohary, Ahmed and Ho, Bo-Jhang and Srivastava, Mani and Chang, Kai-Wei},
467 title = {Generating Natural Language Adversarial Examples},
468}
469
470@inproceedings{li2020bert,
471 year = {2020},
472 pages = {6193--6202},
473 booktitle = {Proceedings of the 2020 Conference on Empirical Methods in Natural Language Processing (EMNLP)},
474 author = {Li, Linyang and Ma, Ruotian and Guo, Qipeng and Xue, Xiangyang and Qiu, Xipeng},
475 title = {BERT-ATTACK: Adversarial Attack Against BERT Using BERT},
476}
477
478@inproceedings{talat2022you,
479 year = {2022},
480 pages = {26--41},
481 booktitle = {Proceedings of BigScience Episode\# 5--Workshop on Challenges \& Perspectives in Creating Large Language Models},
482 author = {Talat, Zeerak and N{\'e}v{\'e}ol, Aur{\'e}lie and Biderman, Stella and Clinciu, Miruna and Dey, Manan and Longpre, Shayne and Luccioni, Sasha and Masoud, Maraim and Mitchell, Margaret and Radev, Dragomir and others},
483 title = {You reap what you sow: On the challenges of bias evaluation under multilingual settings},
484}
485
486@misc{Gokaslan2019OpenWeb,
487 year = {2019},
488 url = {http://Skylion007.github.io/OpenWebTextCorpus},
489 author = {Aaron Gokaslan and Vanya Cohen},
490 title = {OpenWebText Corpus},
491}
492
493@article{armengol2021multilingual,
494 year = {2021},
495 journal = {arXiv preprint arXiv:2108.13349},
496 author = {Armengol-Estap{\'e}, Jordi and Bonet, Ona de Gibert and Melero, Maite},
497 title = {On the Multilingual Capabilities of Very Large-Scale English Language Models},
498}
499
500@inproceedings{sawhney2021empirical,
501 year = {2021},
502 pages = {3751--3757},
503 booktitle = {Proceedings of the 2021 Conference of the North American Chapter of the Association for Computational Linguistics: Human Language Technologies},
504 author = {Sawhney, Ramit and Aggarwal, Arshiya and Shah, Rajiv},
505 title = {An empirical investigation of bias in the multimodal analysis of financial earnings calls},
506}
507
508@article{bommasani2021opportunities,
509 year = {2021},
510 journal = {arXiv preprint arXiv:2108.07258},
511 author = {Bommasani, Rishi and Hudson, Drew A and Adeli, Ehsan and Altman, Russ and Arora, Simran and von Arx, Sydney and Bernstein, Michael S and Bohg, Jeannette and Bosselut, Antoine and Brunskill, Emma and others},
512 title = {On the opportunities and risks of foundation models},
513}
514
515@article{weiemergent,
516 journal = {Transactions on Machine Learning Research},
517 author = {Wei, Jason and Tay, Yi and Bommasani, Rishi and Raffel, Colin and Zoph, Barret and Borgeaud, Sebastian and Yogatama, Dani and Bosma, Maarten and Zhou, Denny and Metzler, Donald and others},
518 title = {Emergent Abilities of Large Language Models},
519}
520
521@article{kaplan2020scaling,
522 year = {2020},
523 journal = {arXiv preprint arXiv:2001.08361},
524 author = {Kaplan, Jared and McCandlish, Sam and Henighan, Tom and Brown, Tom B and Chess, Benjamin and Child, Rewon and Gray, Scott and Radford, Alec and Wu, Jeffrey and Amodei, Dario},
525 title = {Scaling laws for neural language models},
526}
527
528@article{villalobos2022will,
529 year = {2022},
530 journal = {arXiv preprint arXiv:2211.04325},
531 author = {Villalobos, Pablo and Sevilla, Jaime and Heim, Lennart and Besiroglu, Tamay and Hobbhahn, Marius and Ho, Anson},
532 title = {Will we run out of data? An analysis of the limits of scaling datasets in Machine Learning},
533}
534
535@article{northcutt2021confident,
536 year = {2021},
537 pages = {1373--1411},
538 volume = {70},
539 journal = {Journal of Artificial Intelligence Research},
540 author = {Northcutt, Curtis and Jiang, Lu and Chuang, Isaac},
541 title = {Confident learning: Estimating uncertainty in dataset labels},
542}
543
544@article{treviso2022efficient,
545 year = {2022},
546 journal = {arXiv preprint arXiv:2209.00099},
547 author = {Treviso, Marcos and Ji, Tianchu and Lee, Ji-Ung and van Aken, Betty and Cao, Qingqing and Ciosici, Manuel R and Hassid, Michael and Heafield, Kenneth and Hooker, Sara and Martins, Pedro H and others},
548 title = {Efficient methods for natural language processing: a survey},
549}
550
551@inproceedings{lee2022deduplicating,
552 year = {2022},
553 pages = {8424--8445},
554 booktitle = {Proceedings of the 60th Annual Meeting of the Association for Computational Linguistics (Volume 1: Long Papers)},
555 author = {Lee, Katherine and Ippolito, Daphne and Nystrom, Andrew and Zhang, Chiyuan and Eck, Douglas and Callison-Burch, Chris and Carlini, Nicholas},
556 title = {Deduplicating Training Data Makes Language Models Better},
557}
558
559@inproceedings{mishra2020we,
560 year = {2020},
561 pages = {169--173},
562 booktitle = {Proceedings of SustaiNLP: Workshop on Simple and Efficient Natural Language Processing},
563 author = {Mishra, Swaroop and Sachdeva, Bhavdeep Singh},
564 title = {Do we need to create big datasets to learn a task?},
565}
566
567@inproceedings{Bengio2009CurriculumL,
568 year = {2009},
569 booktitle = {International Conference on Machine Learning},
570 author = {Yoshua Bengio and J{\'e}r{\^o}me Louradour and Ronan Collobert and Jason Weston},
571 title = {Curriculum learning},
572}
573
574@article{Ren2020ASO,
575 pages = {1 - 40},
576 volume = {54},
577 year = {2020},
578 journal = {ACM Computing Surveys (CSUR)},
579 author = {Pengzhen Ren and Yun Xiao and Xiaojun Chang and Po-Yao Huang and Zhihui Li and Xiaojiang Chen and Xin Wang},
580 title = {A Survey of Deep Active Learning},
581}
582
583@article{Brown2020LanguageMA,
584 volume = {abs/2005.14165},
585 year = {2020},
586 journal = {ArXiv},
587 author = {Tom B. Brown and Benjamin Mann and Nick Ryder and Melanie Subbiah and Jared Kaplan and Prafulla Dhariwal and Arvind Neelakantan and Pranav Shyam and Girish Sastry and Amanda Askell and Sandhini Agarwal and Ariel Herbert-Voss and Gretchen Krueger and T. J. Henighan and Rewon Child and Aditya Ramesh and Daniel M. Ziegler and Jeff Wu and Clemens Winter and Christopher Hesse and Mark Chen and Eric Sigler and Mateusz Litwin and Scott Gray and Benjamin Chess and Jack Clark and Christopher Berner and Sam McCandlish and Alec Radford and Ilya Sutskever and Dario Amodei},
588 title = {Language Models are Few-Shot Learners},
589}
590
591@article{thompson2020computational,
592 year = {2020},
593 journal = {arXiv preprint arXiv:2007.05558},
594 author = {Thompson, Neil C and Greenewald, Kristjan and Lee, Keeheon and Manso, Gabriel F},
595 title = {The computational limits of deep learning},
596}
597
598@article{wu2022sustainable,
599 year = {2022},
600 pages = {795--813},
601 volume = {4},
602 journal = {Proceedings of Machine Learning and Systems},
603 author = {Wu, Carole-Jean and Raghavendra, Ramya and Gupta, Udit and Acun, Bilge and Ardalani, Newsha and Maeng, Kiwan and Chang, Gloria and Aga, Fiona and Huang, Jinshi and Bai, Charles and others},
604 title = {Sustainable ai: Environmental implications, challenges and opportunities},
605}
606
607@inproceedings{DeCao2021EditingFK,
608 year = {2021},
609 booktitle = {Conference on Empirical Methods in Natural Language Processing},
610 author = {Nicola De Cao and Wilker Aziz and Ivan Titov},
611 title = {Editing Factual Knowledge in Language Models},
612}
613
614@article{Zhu2020ModifyingMI,
615 volume = {abs/2012.00363},
616 year = {2020},
617 journal = {ArXiv},
618 author = {Chen Zhu and Ankit Singh Rawat and Manzil Zaheer and Srinadh Bhojanapalli and Daliang Li and Felix X. Yu and Sanjiv Kumar},
619 title = {Modifying Memories in Transformer Models},
620}
621
622@inproceedings{mitchellfast,
623 booktitle = {International Conference on Learning Representations},
624 author = {Mitchell, Eric and Lin, Charles and Bosselut, Antoine and Finn, Chelsea and Manning, Christopher D},
625 title = {Fast Model Editing at Scale},
626}
627
628@article{yang2022diffsound,
629 year = {2022},
630 journal = {arXiv preprint arXiv:2207.09983},
631 author = {Yang, Dongchao and Yu, Jianwei and Wang, Helin and Wang, Wen and Weng, Chao and Zou, Yuexian and Yu, Dong},
632 title = {Diffsound: Discrete diffusion model for text-to-sound generation},
633}
634
635@article{kreuk2022audiogen,
636 year = {2022},
637 journal = {arXiv preprint arXiv:2209.15352},
638 author = {Kreuk, Felix and Synnaeve, Gabriel and Polyak, Adam and Singer, Uriel and D{\'e}fossez, Alexandre and Copet, Jade and Parikh, Devi and Taigman, Yaniv and Adi, Yossi},
639 title = {Audiogen: Textually guided audio generation},
640}
641
642@article{borsos2022audiolm,
643 year = {2022},
644 journal = {arXiv preprint arXiv:2209.03143},
645 author = {Borsos, Zal{\'a}n and Marinier, Rapha{\"e}l and Vincent, Damien and Kharitonov, Eugene and Pietquin, Olivier and Sharifi, Matt and Teboul, Olivier and Grangier, David and Tagliasacchi, Marco and Zeghidour, Neil},
646 title = {Audiolm: a language modeling approach to audio generation},
647}
648
649@misc{musiclm,
650 copyright = {arXiv.org perpetual, non-exclusive license},
651 year = {2023},
652 publisher = {arXiv},
653 title = {MusicLM: Generating Music From Text},
654 keywords = {Sound (cs.SD), Machine Learning (cs.LG), Audio and Speech Processing (eess.AS), FOS: Computer and information sciences, FOS: Computer and information sciences, FOS: Electrical engineering, electronic engineering, information engineering, FOS: Electrical engineering, electronic engineering, information engineering},
655 author = {Agostinelli, Andrea and Denk, Timo I. and Borsos, Zalán and Engel, Jesse and Verzetti, Mauro and Caillon, Antoine and Huang, Qingqing and Jansen, Aren and Roberts, Adam and Tagliasacchi, Marco and Sharifi, Matt and Zeghidour, Neil and Frank, Christian},
656 url = {https://arxiv.org/abs/2301.11325},
657 doi = {10.48550/ARXIV.2301.11325},
658}
659
660@inproceedings{zhang2021vinvl,
661 year = {2021},
662 pages = {5579--5588},
663 booktitle = {Proceedings of the IEEE/CVF Conference on Computer Vision and Pattern Recognition},
664 author = {Zhang, Pengchuan and Li, Xiujun and Hu, Xiaowei and Yang, Jianwei and Zhang, Lei and Wang, Lijuan and Choi, Yejin and Gao, Jianfeng},
665 title = {Vinvl: Revisiting visual representations in vision-language models},
666}
667
668@article{zhou2022learning,
669 publisher = {Springer},
670 year = {2022},
671 pages = {2337--2348},
672 number = {9},
673 volume = {130},
674 journal = {International Journal of Computer Vision},
675 author = {Zhou, Kaiyang and Yang, Jingkang and Loy, Chen Change and Liu, Ziwei},
676 title = {Learning to prompt for vision-language models},
677}
678
679@inproceedings{hecater,
680 booktitle = {Advances in Neural Information Processing Systems},
681 author = {He, Xuanli and Xu, Qiongkai and Zeng, Yi and Lyu, Lingjuan and Wu, Fangzhao and Li, Jiwei and Jia, Ruoxi},
682 title = {CATER: Intellectual Property Protection on Text Generation APIs via Conditional Watermarks},
683}
684
685@inproceedings{he2022protecting,
686 year = {2022},
687 pages = {10758--10766},
688 number = {10},
689 volume = {36},
690 booktitle = {Proceedings of the AAAI Conference on Artificial Intelligence},
691 author = {He, Xuanli and Xu, Qiongkai and Lyu, Lingjuan and Wu, Fangzhao and Wang, Chenguang},
692 title = {Protecting intellectual property of language generation apis with lexical watermark},
693}
694
695@article{kirchenbauer2023watermark,
696 year = {2023},
697 journal = {arXiv preprint arXiv:2301.10226},
698 author = {Kirchenbauer, John and Geiping, Jonas and Wen, Yuxin and Katz, Jonathan and Miers, Ian and Goldstein, Tom},
699 title = {A Watermark for Large Language Models},
700}
701
702@inproceedings{kandpal2022deduplicating,
703 organization = {PMLR},
704 year = {2022},
705 pages = {10697--10707},
706 booktitle = {International Conference on Machine Learning},
707 author = {Kandpal, Nikhil and Wallace, Eric and Raffel, Colin},
708 title = {Deduplicating training data mitigates privacy risks in language models},
709}
710
711@article{scao2022bloom,
712 year = {2022},
713 journal = {arXiv preprint arXiv:2211.05100},
714 author = {Scao, Teven Le and Fan, Angela and Akiki, Christopher and Pavlick, Ellie and Ili{\'c}, Suzana and Hesslow, Daniel and Castagn{\'e}, Roman and Luccioni, Alexandra Sasha and Yvon, Fran{\c{c}}ois and Gall{\'e}, Matthias and others},
715 title = {Bloom: A 176b-parameter open-access multilingual language model},
716}
717
718@article{allal2023santacoder,
719 year = {2023},
720 journal = {arXiv preprint arXiv:2301.03988},
721 author = {Allal, Loubna Ben and Li, Raymond and Kocetkov, Denis and Mou, Chenghao and Akiki, Christopher and Ferrandis, Carlos Munoz and Muennighoff, Niklas and Mishra, Mayank and Gu, Alex and Dey, Manan and others},
722 title = {SantaCoder: don't reach for the stars!},
723}
724
725@article{aghajanyan2023scaling,
726 year = {2023},
727 journal = {arXiv preprint arXiv:2301.03728},
728 author = {Aghajanyan, Armen and Yu, Lili and Conneau, Alexis and Hsu, Wei-Ning and Hambardzumyan, Karen and Zhang, Susan and Roller, Stephen and Goyal, Naman and Levy, Omer and Zettlemoyer, Luke},
729 title = {Scaling Laws for Generative Mixed-Modal Language Models},
730}
731
732@article{cao2022ai,
733 publisher = {ACM New York, NY},
734 year = {2022},
735 pages = {1--38},
736 number = {3},
737 volume = {55},
738 journal = {ACM Computing Surveys (CSUR)},
739 author = {Cao, Longbing},
740 title = {Ai in finance: challenges, techniques, and opportunities},
741}
742
743@article{borji2023categorical,
744 year = {2023},
745 journal = {arXiv preprint arXiv:2302.03494},
746 author = {Borji, Ali},
747 title = {A Categorical Archive of ChatGPT Failures},
748}
Attribution
arXiv:2301.12867v4
[cs.CL]
License: cc-by-4.0