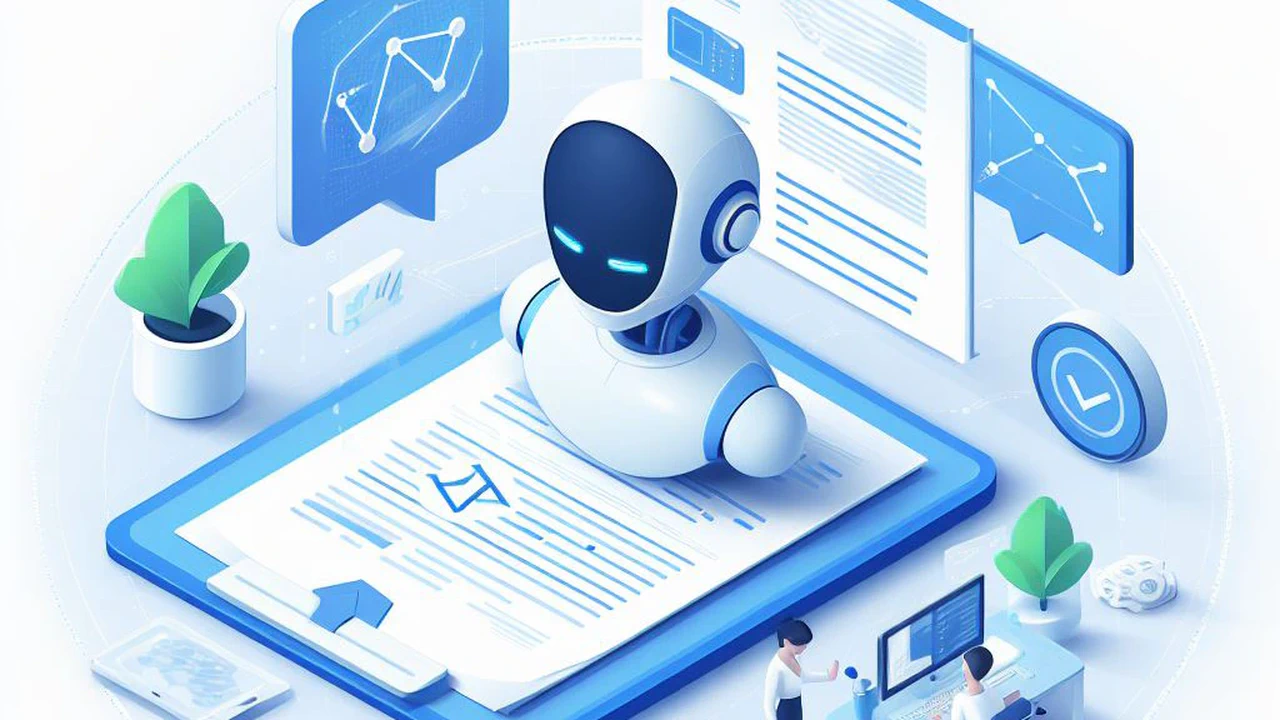
- Papers
Papers is Alpha. This content is part of an effort to make research more accessible, and (most likely) has lost some details from the original. You can find the original paper here.
Introduction
Ensuring the responsible development and use of AI is becoming a main direction in AI research and practice. Governments, corporations and international organisations alike are coming forward with proposals and declarations of their commitment to an accountable, responsible, transparent approach to AI, where human values and ethical principles are leading.
Currently, there are over 600 AI-related policy recommendations, guidelines or strategy reports, which have been released by prominent intergovernmental organisations, professional bodies, national-level committees and other public organisations, non-governmental, and private for-profit companies. A recent study of the global landscape of AI ethics guidelines shows that there is a global convergence around five ethical principles: Transparency, Justice and Fairness, Non-Maleficence, Responsibility, and Privacy. These are much-needed efforts, but still much work is needed to ensure that all AI is developed and used in responsible ways that contribute to trust and well-being. Nevertheless, even though organisations agree on the need to consider ethical, legal and societal principles, how these are interpreted and applied in practice, varies significantly across the different recommendation documents.
At the same time, the growing hype around `AI’ is blurring its definition and shoving into the same heap concepts and applications of many different sorts. A hard needed first step in the responsible development and use of AI is to ensure a proper AI narrative, one that demystifies its capabilities, minimises both overselling and underselling of AI-driven solutions, and that enables wide and inclusive participation in the discussion on the role of AI in society. Understanding the capabilities and addressing the risks of AI, requires that all of us, from developers to policy-makers, from provides to end-users and bystanders, have a clear understanding of what AI is, how it is applied and what are the opportunities and risks involved.
What AI is not: data, algorithms, magic
Without a proper understanding of what AI is and what it can, and cannot, do, all the efforts towards governance, regulation and the responsible development and use of AI have the risk to become void. Current AI narratives bring forward benefits and risks and describe AI in many different ways, from the obvious next step in digitisation to some kind of magic. If the business as usual' narrative is detrimental of innovation and contributes to the maintenance current power structures, the
magic’ narrative, well fed by science fiction and the popular press, often supports a feeling that nothing can be done against such an all-knowing entity that rules over us in possibly unexpected ways, either solving all our problems, or destroying the world in the process. In both cases, the danger is that the message is that little can be done against the risks and challenges of AI.
Currently, AI is mostly associated with data-driven techniques that use statistical methods to enable computers to perceive some characteristics of their environment. Such techniques are particularly efficient in perceiving images, written or spoken text, as well as the many applications of structured data. These techniques are extremely successful at pattern matching: By analysing many thousands of examples (typically a few million), the system is able to identify commonalities in these examples, which then enable it to interpret data that it has never seen before, which is often referred to as prediction. These results, however impressive and useful, are still far from any thing that we would consider as `intelligent’. Moreover, data-driven approaches to AI have been proven to be problematic in terms of bias, explanation, inclusion and transparency. Algorithms are too complex for human inspection, and a over-reliance on data, condemns the future to repeat the past. Indeed, data is always about the past, and decisions on which, how, when, why collect and maintain data fundamentally influence the availability and quality of data. Those that have the power to decide on data, have the power to determine how AI system will be design, deployed and used.
AI is based on algorithms, but then so is any computer program and most of the technologies around us. Nevertheless, the concept of `algorithm’ is achieving magical proportions, used right and left to signify many things, de facto seen as a synonym to AI. The easiest way to understand an algorithm is as a recipe, a set of precise rules to achieve a certain result. Every time you multiply two numbers, you are using an algorithm, as well as you are when you are baking an apple pie. However, by itself, the recipe has never turned into an apple pie; and, the end result of your pie has as much to do with your baking skills and your choice of ingredients, as with the choice for a specific recipe. The same applies to AI algorithms: for a large part the behaviour and results of the system depends on its input data, and on the choices made by those that developed, trained and selected the algorithm. In the same way as we have the choice to use organic apples to make our pie, in AI we also must consider our choices on which models, data to use, who to include in the design and considerations about impact, and how these choices respect and ensure fairness, privacy, transparency and all other values we hold dear.
AI is a socio-technical ecosystem: datification, power and costs
If AI is not intelligent, nor magic, nor business as usual, nor an algorithm, how best can we describe AI in order to take into account not only its capabilities but also its societal implications? AI is first and foremost technology that can automatise (simple, lesser) tasks and decision making processes. At the present, AI systems are largely incapable of understanding meaning and the context of their operation and results. At the same time, considering its societal impact and need for human contribution, AI is much more than an automation technique. When considering effects and the governance thereof, the technology, or the artefact that embeds that technology, cannot be separated from the ecosystem of which it is a component. In this sense, AI can best be understood as a socio-technical ecosystem, recognising the interaction between people and technology, and how complex infrastructures affect and are affected by society and by human behaviour. AI is not just about the automation of decisions and actions, the adaptability to learn from the changes affected in the environment, and the interactivity required to be sensitive to the actions and aims of other agents in that environment, and decide when to cooperate or to compete. It is mostly about the structures of power, participation and access to technology that determine who can influence which decisions or actions are being automated, which data, knowledge and resources are used to learn from, and how interactions between those that decide and those that are impacted are defined and maintained.
Much has been said about the dangers of biased data, and discriminating applications. Minimising or eliminating discriminatory bias or unfair outcomes is more than excluding the use of low-quality data. The design of any artefact, such as an AI system, is in itself an accumulation of choices and choices are biased by nature as they involve selecting an option over another. Most importantly, it starts with the current reliance on data as a measure of what can be done. Increasingly, we are seeing that the availability of that (or the possibility to access data) is taken as a guiding criteria to solving societal issues. If there is data, it is a problem we can address, but if there is no data, there is no problem. This is intrinsically related to power and to power structures. Those that can decide on which problems are worth address, are shaping not only how AI is being developed and used, which technologies to use and what values to prioritise. Those in power are shaping the way we live with AI and how our future societies will look like.
Nevertheless, attention for the societal, environmental and climate costs of AI systems is increasing. All these must be included in any effort to ensure the responsible development and use of AI. A responsible, ethical, approach to AI will ensure transparency about how adaptation is done, responsibility for the level of automation on which the system is able to reason, and accountability for the results and the principles that guide its interactions with others, most importantly with people. In addition, and above all, a responsible approach to AI makes clear that AI systems are artefacts manufactured by people for some purpose, and that those which make these have the power to decide on the use of AI. It is time to discuss how power structures determine AI and how AI establishes and maintains power structures, and on the balance between, those who benefit from, and those who are harmed by the use of AI.
Responsible AI - The question zero
Responsible AI (or Ethical AI, or Trustworthy AI) is not, as some may claim, a way to give machines some kind of `responsibility’ for their actions and decisions, and in the process discharge people and organisations of their responsibility. On the contrary, responsible development and use of AI requires more responsibility and more accountability from the people and organisations involved: for the decisions and actions of the AI applications, and for their own decision of using AI in a given application context. When considering effects and the governance thereof, the technology, or the artefact that embeds that technology, cannot be separated from the socio-technical ecosystem of which it is a component. Guidelines, principles and strategies to ensure trust and responsibility in AI, must be directed towards the socio-technical ecosystem in which AI is developed and used. It is not the AI artefact or application that needs to be ethical, trustworthy, or responsible. Rather, it is the social component of this ecosystem that can and should take responsibility and act in consideration of an ethical framework such that the overall system can be trusted by the society. Having said this, governance can be achieved by several means, softer or harder. Currently several directions are being explored, the main ones are highlighted in the remainder of this section. Future research and experience will identify which approaches are the most suitable, but given the complexity of the problem, it is very likely that a combination of approaches will be needed.
Responsible AI is more than the ticking of some ethical `boxes’ or the development of some add-on features in AI systems. Nevertheless, developers and users can benefit from support and concrete steps to understand the relevant legal and ethical standards and considerations when making decisions on the use of AI applications. Impact assessment tools provide a step-by-step evaluation of the impact of systems, methods or tools on aspects such as privacy, transparency, explanation, bias, or liability.
Inclusion and diversity are a broader societal challenge central to AI development. It is therefore important that as broad a group of people as possible have a basic knowledge of AI, what can (and can’t) be done with AI, and how AI impacts individual decisions and shapes society. In parallel, research and development of AI systems must be informed by diversity, in all the meanings of diversity, and obviously including gender, cultural background, and ethnicity. Moreover, AI is not any longer an engineering discipline and at the same time there is growing evidence that cognitive diversity contributes to better decision making. Therefore, it is important to diversify the discipline background and expertise of those working on AI to include AI professionals with knowledge of, amongst others, philosophy, social science, law and economy.
Design for Responsibility
A multidisciplinary stance supporting understanding and critiquing the intended and unforeseen, positive and negative, and the socio-political consequences of AI for society, is core to the responsible design of AI systems. This multidisciplinary approach is fundamental to understand governance, not only in terms of competences and responsibilities, but also in terms of power, trust and accountability; to analyse the societal, legal and economic functioning of socio-technical systems, providing value-based design approaches and ethical frameworks for inclusion and diversity in design, and how such strategies may inform processes and results.
Achieving trustworthy AI systems is a multifaceted complex process, which requires both technical and socio-legal initiatives and solutions to ensure that we always align an intelligent system’s goals with human values. Core values, as well as the processes used for value elicitation, must be made explicit and that all stakeholders are involved in this process. Furthermore, the methods used for the elicitation processes and the decisions of who is involved in the value identification process must be clearly identified and documented.
Where it concerns the design process itself, responsibility includes the need to elicit and represent stakeholders, their values and expectations, as well as ensuring transparency about how such values are interpreted and prioritised in the concrete functionalities of the AI system. Design for Values methodologiesare often used for this end, providing a structured way for translation from abstract values into concrete norms comprehensive enough so that fulfilling the norm will be considered as adhering to the value. Following a Design for Values approach, the shift from abstract to concrete necessarily involves careful consideration of the context. Design for Values approach means that the process needs to include activities for (i) the identification of societal values, (ii) deciding on a moral deliberation approach (e.g. through algorithms, user control or regulation), and (3) linking values to formal system requirementsand concrete functionalities.
My research group is developing the Glass Box frameworkthat is both an approach to software development, a verification method and a source of high-level transparency for intelligent systems. It provides a modular approach integrating verification with value-based design.
Conclusions
Increasingly, AI systems will be taking decisions that affect our lives, in smaller or larger ways. In all areas of application, AI must be able to take into account societal values, moral and ethical considerations, weigh the respective priorities of values held by different stakeholders and in multicultural contexts, explain its reasoning, and guarantee transparency. As the capabilities for autonomous decision-making grow, perhaps the most important issue to consider is the need to rethink responsibility. Being fundamentally tools, AI systems are fully under the control and responsibility of their owners or users. However, their potential autonomy and capability to learn, require that design considers accountability, responsibility and transparency principles in an explicit and systematic manner. The development of AI algorithms has so far been led by the goal of improving performance, leading to opaque black boxes. Putting human values at the core of AI systems calls for a mind-shift of researchers and developers towards the goal of improving transparency rather than performance, which will lead to novel and exciting techniques and applications.
Finally, it is crucial to understand responsibility, regulation and ethics as stepping-stone for innovation, rather than the often referred hinder to innovation. True innovation is moving technology forward, not use existing technology ‘as is’. Taking responsibility and regulation as beacons pointing the direction to move, will not only lead to better technology but also ensure trust and public acceptance, serve as a drive for transformation and for business differentiation. Efforts in fundamental research are part of this. Currently, much AI `innovation’ is based on brute force: when the main data analytics paradigm is correlation, better accuracy is achieved by increased amount of data and computational power. The effort/accuracy ratio is huge. However, human intelligence is not based on correlation, and includes causality and abstraction. Responsibility in AI is not just about ethics, bias, and trolley problems. It is also about responsible innovation: ensuring the best tools for the job, minimize side effects. Responsible innovation in AI requires “a shift from a perspective in which learning is more or less the only first-class citizen to one in which learning is a central member of a broader coalition that is more welcoming to variables, prior knowledge, reasoning, and rich cognitive models.".
*
Acknowledgements This work was partially supported by the Wallenberg AI, Autonomous Systems and Software Program (WASP), funded by the Knut and Alice Wallenberg Foundation and by the European Commission’s Horizon2020 project HumaneAI-Net (grant 952026).
Bibliography
1@book{cihon2019a,
2 language = {en},
3 year = {2019},
4 publisher = {Future of Humanity Institute. University of Oxford},
5 title = {Standards for AI governance: international standards to enable global coordination in ai research \& development},
6 author = {Cihon, Peter},
7 citation-number = {1},
8}
9
10@book{colman2015a,
11 language = {en},
12 year = {2015},
13 publisher = {Oxford quick reference},
14 title = {A dictionary of psychology},
15 author = {Colman, Andrew M.},
16 citation-number = {2},
17}
18
19@book{crawford2021a,
20 language = {en},
21 year = {2021},
22 publisher = {Yale University Press},
23 title = {The Atlas of AI},
24 author = {Crawford, Kate},
25 citation-number = {3},
26}
27
28@article{dignum2017a,
29 number = {11},
30 journal = {Communications of the ACM},
31 language = {en},
32 pages = {32–34},
33 year = {2017},
34 volume = {60},
35 title = {Social agents: bridging simulation and engineering},
36 author = {Dignum, Virginia},
37 citation-number = {5},
38}
39
40@article{dignum2020a,
41 number = {215},
42 journal = {The Oxford Handbook of Ethics of AI},
43 language = {en},
44 year = {2020},
45 volume = {4698},
46 title = {Responsibility and artificial intelligence},
47 author = {Dignum, Virginia},
48 citation-number = {6},
49}
50
51@article{dignum2021a,
52 journal = {Nature},
53 language = {en},
54 year = {2021},
55 volume = {593:499–500},
56 title = {{AI} - the people and places that make, use and manage it},
57 author = {Dignum, Virginia},
58 citation-number = {7},
59}
60
61@article{dignum2010a,
62 number = {3},
63 journal = {IJAOSE},
64 language = {en},
65 pages = {224–243},
66 year = {2010},
67 volume = {4},
68 title = {Designing agent systems: state of the practice},
69 author = {Dignum, Virginia and Dignum, Frank},
70 citation-number = {8},
71}
72
73@article{durham1990a,
74 number = {1},
75 journal = {Annual Review of Anthropology},
76 language = {en},
77 pages = {187–210},
78 year = {1990},
79 volume = {19},
80 title = {Advances in evolutionary culture theory},
81 author = {Durham, William H.},
82 citation-number = {9},
83}
84
85@book{eglash1999a,
86 language = {en},
87 year = {1999},
88 publisher = {Rutgers University Press},
89 title = {African Fractals: Modern Computing and Indigenous Design},
90 author = {Eglash, R.},
91 citation-number = {10},
92}
93
94@article{ewuoso2019a,
95 number = {2},
96 journal = {South African Journal of Bioethics and Law},
97 language = {en},
98 pages = {93–103},
99 year = {2019},
100 volume = {12},
101 title = {Core aspects of Ubuntu: A systematic review},
102 author = {Ewuoso, C. and Hall, S.},
103 citation-number = {11},
104}
105
106@misc{gardner2011a,
107 address = {Hachette, UK},
108 language = {en},
109 year = {2011},
110 title = {Frames of mind: The theory of multiple intelligences},
111 author = {Gardner, Howard E.},
112 citation-number = {12},
113}
114
115@article{gould1997a,
116 number = {1},
117 journal = {Proceedings of the American Philosophical Society},
118 language = {en},
119 pages = {30–54},
120 year = {1997},
121 volume = {141},
122 title = {Redrafting the tree of life},
123 author = {Gould, Stephen Jay},
124 citation-number = {13},
125}
126
127@article{jobin2019a,
128 number = {9},
129 journal = {Nature Machine Intelligence},
130 language = {en},
131 pages = {389–399},
132 year = {2019},
133 volume = {1},
134 title = {The global landscape of {AI} ethics guidelines},
135 author = {Jobin, Anna and Ienca, Marcello and Vayena, Effy},
136 citation-number = {14},
137}
138
139@incollection{lindenberg2001a,
140 booktitle = {Handbook of sociological theory},
141 language = {en},
142 year = {2001},
143 publisher = {Springer},
144 pages = {635–668},
145 title = {Social rationality versus rational egoism},
146 author = {Lindenberg, S.},
147 citation-number = {15},
148}
149
150@article{lutz2009a,
151 number = {3},
152 journal = {Journal of Business Ethics},
153 language = {en},
154 pages = {313–328},
155 year = {2009},
156 volume = {84},
157 title = {African Ubuntu philosophy and global management},
158 author = {Lutz, David W.},
159 citation-number = {16},
160}
161
162@article{metz2011a,
163 number = {2},
164 journal = {African Human Rights Law Journal},
165 language = {en},
166 pages = {532–559},
167 year = {2011},
168 volume = {11},
169 title = {Ubuntu as a moral theory and human rights in South Africa},
170 author = {Metz, Thaddeus},
171 citation-number = {17},
172}
173
174@article{mugumbate2013a,
175 number = {1},
176 journal = {African Journal of Social Work},
177 language = {en},
178 year = {2013},
179 pages = {100,},
180 volume = {3},
181 title = {Exploring African philosophy: The value of Ubuntu in social work},
182 author = {Mugumbate, Jacob and Nyanguru, Andrew},
183 citation-number = {18},
184}
185
186@article{osei-hwedie2007a,
187 number = {2},
188 journal = {Social work/Maatskaplike werk},
189 language = {nl},
190 year = {2007},
191 volume = {43},
192 title = {Afro-centrism: The challenge of social development},
193 author = {Osei-Hwedie, Kwaku},
194 citation-number = {19},
195}
196
197@article{piaget1964a,
198 number = {3},
199 journal = {Journal of research in science teaching},
200 language = {en},
201 pages = {176–186},
202 year = {1964},
203 volume = {2},
204 title = {Part I: Cognitive development in children: Piaget development and learning},
205 author = {Piaget, Jean},
206 citation-number = {20},
207}
208
209@book{russell2010a,
210 language = {it},
211 year = {2010},
212 publisher = {Prentice Hall},
213 title = {Artificial intelligence: a modern approach},
214 author = {Russell, Stuart and Norvig, Peter},
215 citation-number = {21},
216}
217
218@article{sternberg1984a,
219 number = {2},
220 journal = {Behavioral and Brain Sciences},
221 language = {en},
222 pages = {269–287},
223 year = {1984},
224 volume = {7},
225 title = {Toward a triarchic theory of human intelligence},
226 author = {Sternberg, Robert J.},
227 citation-number = {22},
228}
229
230@article{taddeo2018a,
231 number = {6404},
232 journal = {Science},
233 language = {en},
234 pages = {751–752},
235 year = {2018},
236 volume = {361},
237 title = {How {AI} can be a force for good},
238 author = {Taddeo, Mariarosaria and Floridi, Luciano},
239 citation-number = {23},
240}
241
242@article{theodorou2020a,
243 number = {1},
244 journal = {Nature Machine Intelligence},
245 language = {en},
246 pages = {10–12},
247 year = {2020},
248 volume = {2},
249 title = {Towards ethical and socio-legal governance in AI},
250 author = {Theodorou, Andreas and Dignum, Virginia},
251 citation-number = {24},
252}
253
254@article{breda2019a,
255 number = {439},
256 journal = {Social Work},
257 language = {en},
258 year = {2019},
259 pages = {– 450, 00},
260 volume = {55},
261 title = {Developing the notion of Ubuntu as African theory for social work practice},
262 author = {Breda, Adrian D.},
263 citation-number = {25},
264}
265
266@article{vinuesa2020a,
267 number = {1},
268 journal = {Nature communications},
269 language = {en},
270 pages = {1–10},
271 year = {2020},
272 volume = {11},
273 title = {The role of artificial intelligence in achieving the sustainable development goals},
274 author = {Vinuesa, Ricardo and Azizpour, Hossein and Leite, Iolanda and Balaam, Madeline and Dignum, Virginia and Domisch, Sami and Felländer, Anna and Langhans, Simone Daniela and Tegmark, Max and Nerini, Francesco Fuso},
275 citation-number = {26},
276}
277
278@book{Larson2021,
279 title = {The Myth of Artificial Intelligence},
280 author = {Erik J. Larson},
281 publisher = {Harvard University Press},
282 month = {April},
283 year = {2021},
284 url = {https://doi.org/10.4159/9780674259935},
285 doi = {10.4159/9780674259935},
286}
287
288@book{raibook2019,
289 abstract = {In this book, the author examines the ethical implications of Artificial Intelligence systems as they integrate and replace traditional social structures in new sociocognitive-technological environments. She discusses issues related to the integrity of researchers, technologists, and manufacturers as they design, construct, use, and manage artificially intelligent systems; formalisms for reasoning about moral decisions as part of the behavior of artificial autonomous systems such as agents and robots; and design methodologies for social agents based on societal, moral, and legal values. Throughout the book the author discusses related work, conscious of both classical, philosophical treatments of ethical issues and the implications in modern, algorithmic systems, and she combines regular references and footnotes with suggestions for further reading. This short overview is suitable for undergraduate students, in both technical and non-technical courses, and for interested and concerned researchers, practitioners, and citizens.},
290 publisher = {Springer},
291 year = {2019},
292 title = {Responsible Artificial Intelligence: How to Develop and Use AI in a Responsible Way},
293 author = {Dignum, Virginia},
294}
295
296@incollection{chatila2019ieee,
297 publisher = {Springer},
298 year = {2019},
299 pages = {11--16},
300 booktitle = {Robotics and well-being},
301 author = {Chatila, Raja and Havens, John C},
302 title = {The {IEEE} global initiative on ethics of autonomous and intelligent systems},
303}
304
305@book{p7000,
306 publisher = {IEEE},
307 year = {2021},
308 author = {{Systems and Software Engineering Standards Committee} et al.},
309 title = {7000-2021-{IEEE} {S}tandard {M}odel {P}rocess for {A}ddressing {E}thical {C}oncerns during {S}ystem {D}esign},
310}
311
312@article{simon1991bounded,
313 publisher = {INFORMS},
314 year = {1991},
315 pages = {125--134},
316 number = {1},
317 volume = {2},
318 journal = {Organization science},
319 author = {Simon, Herbert A},
320 title = {Bounded rationality and organizational learning},
321}
322
323@article{birhane2021algorithmic,
324 publisher = {Elsevier},
325 year = {2021},
326 pages = {100205},
327 number = {2},
328 volume = {2},
329 journal = {Patterns},
330 author = {Birhane, Abeba},
331 title = {Algorithmic injustice: a relational ethics approach},
332}
333
334@article{adam1995artificial,
335 organization = {Elsevier},
336 year = {1995},
337 pages = {407--415},
338 number = {4},
339 volume = {18},
340 booktitle = {Women's Studies International Forum},
341 author = {Adam, Alison},
342 title = {Artificial intelligence and women's knowledge: What can feminist epistemologies tell us?},
343}
344
345@article{dignazio2015would,
346 year = {2015},
347 volume = {20},
348 journal = {MIT Center for Civic Media},
349 author = {D’Ignazio, Catherine},
350 title = {What would feminist data visualization look like},
351}
352
353@misc{dignum22,
354 copyright = {Creative Commons Attribution 4.0 International},
355 year = {2022},
356 publisher = {arXiv},
357 title = {Relational {A}rtificial {I}ntelligence},
358 author = {Dignum, Virginia},
359 url = {https://arxiv.org/abs/2202.07446},
360 doi = {10.48550/ARXIV.2202.07446},
361}
362
363@misc{marcus20,
364 copyright = {arXiv.org perpetual, non-exclusive license},
365 year = {2020},
366 publisher = {arXiv},
367 title = {The Next Decade in AI: Four Steps Towards Robust Artificial Intelligence},
368 keywords = {Artificial Intelligence (cs.AI), Machine Learning (cs.LG), FOS: Computer and information sciences, FOS: Computer and information sciences, I.2; I.2.6},
369 author = {Marcus, Gary},
370 url = {https://arxiv.org/abs/2002.06177},
371 doi = {10.48550/ARXIV.2002.06177},
372}
373
374@inproceedings{aler2019glass,
375 organization = {CEUR-WS},
376 year = {2019},
377 booktitle = {AISafety 2019, Macao, China, August 11-12, 2019},
378 author = {Aler Tubella, Andrea and Dignum, Virginia},
379 title = {The glass box approach: Verifying contextual adherence to values},
380}
381
382@article{Hoven05,
383 pages = {4--7},
384 year = {2005},
385 number = {2},
386 volume = {7},
387 journal = {Information Age +, Journal of the Australian Computer Society},
388 title = {Design for values and values for design},
389 author = {van den Hoven, Jeroen},
390}
391
392@article{friedman2006,
393 pages = {348 - 372},
394 volume = {6},
395 year = {2006},
396 publisher = {M.E. Sharpe},
397 journal = {Advances in Management Information Systems},
398 title = {Value Sensitive Design and Information Systems},
399 author = {Batya Friedman and Peter Kahn and Alan Borning},
400}
Attribution
arXiv:2205.10785v1
[cs.CY]
License: cc-by-4.0