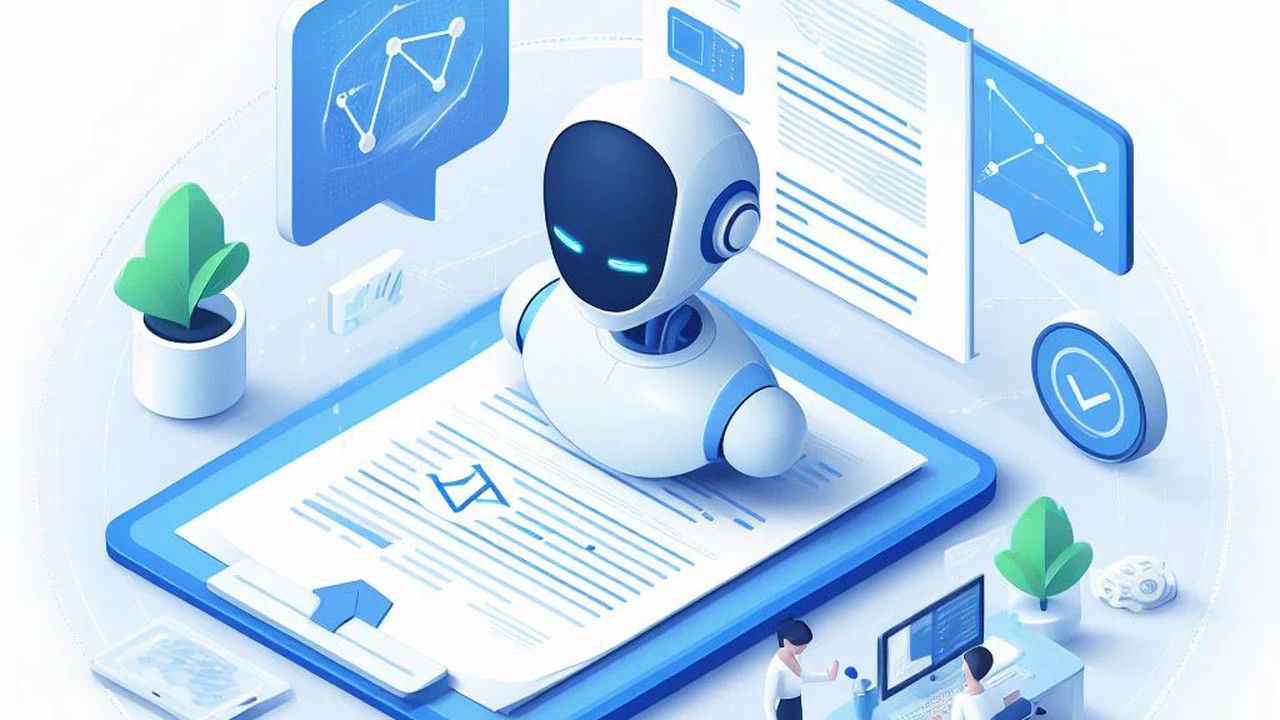
- Papers
Papers is Alpha. This content is part of an effort to make research more accessible, and (most likely) has lost some details from the original. You can find the original paper here.
Measurement is everywhere (but usually implicit)
In algorithmic[ In this paper we flexibly use “algorithmic systems” or “automated decision-making systems,” more broadlyas computational systems, AI systems, or sociotechnical systems. All such terms are meant to capture the algorithmic or computational aspects, which may be based on various machine learning models, as well as to capture the broader context of use, which may include data pipelines, system design and implementation, and organizational use.=-1 ] systems, social phenomena are constantly being operationalized, from creditworthiness, gender, and race, to employee quality, community health, product quality, and attention, user content preferences,language toxicity, relevance, image descriptions—themselves laden with cultural and social knowledge. In practice, the measurements of these phenomena are constrained by existing practices, data availability, and other problems of problem formulation. The process of creating measures of such phenomena is a version of what social scientists call measurement . But in algorithmic system development and data science, this measurement process is almost always implicit—from operationalizing the theoretical understandings of unobservable theoretical constructs (toxicity, quality, etc.) and connecting them to observable data. Here we build from the measurement framework put forward by Jacobs and Wallachto show where and how social, cultural, organizational, and political values are encoded in algorithmic systems, and furthermore how these encodings then shape the social world. Any attempt at meaningful “responsible AI” must consider our values (where and when they are encoded, implicitly or otherwise) and fairness-related harms (where and why they emerge). The lens of measurement makes such considerations possible, and moreover, reveals where governance already is playing a role in responsible AI—and where it could. =-1 =-1
The measurement process reveals where fairness-related harms emerge
Here we build directly on the measurement framework put forward by Jacobs and Wallach. Using this framework, we can understand concepts such as employee quality, language toxicity, community health, or social categories, as unobservable theoretical constructs that are reflected in observable properties of the real world. To connect these observable properties to the constructs of interest, we operationalize a theoretical understanding of the construct and, with a measurement model , show how we connect observable properties to that operationalization. In practice, this process happens constantly in the design of sociotechnical systems. Sometimes this process of assumptions, operationalization and measurement are more explicit: proxies refer to a special case of measurement models. Sometimes the distinction between constructs, operationalizations, and measurements are more obscured: consider using inferred demographic attributes, predicting clickthrough rate to infer attention, or using other system data exhaust to predict behavior. =-1
This process matters beyond academic exercise and pedantry
because fairness-related harms emerge when there is a mismatch between the thing we purport to be measuring and the thing we actually measure.
As an example,
consider a tool for hiring or promotion: we might choose to operationalize employee quality' with
past salary.’ We could then choose the measurement model of past salary' as an individual's average salary over the last three years. Even in this simple example, using this operationalization of employee quality would reinforce existing gender and racial pay disparities by systematically encoding
quality’ as salary. This is an example of one such fairness-related harm—revealed by a mismatch between the construct of employee quality' and its operationalization,
past salary.’
=-1
Measurement is governance
We have thus far established that measurement is everywhere, whether we acknowledge it or not; that fairness-related harms emerge from mismatches in the measurement process; and that being explicit about about the measurement process, whether proactively or retrospectively, can reveal where those mismatches (and potential for harms) emerge. A theme from this past work is that the assumptions underlying sociotechnical systems are crucial, and that frameworks that reveal those assumptions can mitigate and prevent harms. Furthermore, assumptions are decisions —and are decisions that reflect values—therefore frameworks that reveal those assumptions reveal implicit decisions in the creation, use, and evaluation of sociotechnical systems. So where is governance in responsible AI? =-1 Let us consider two sites. =-1
Governance in responsible AI
First, decisions about what happens within algorithmic systems are governance decisions. What data is used where, to what ends; what is being predicted or decided; what variables are named what and accessible to whom—all of these decisions shape what a system can and cannot do. Some of these decisions are explicit: for instance, gender is a binary variable; these advertisements should be served to those inferred income groups; only users that have selected one of those two binary gender variables will be eligible for an account; only users with a paid account can access this data; this functionality will be named Friend' and this one,
Like’. The impacts of such decisions, of course, can extend well beyond their immediate implementation. And the downstream consequences of the decisions—of who is included, of who gets shown what content, of how we engage with a system through an API or as a user, of how we interpret functionalities (`Like’)—need not have been explicitly engaged with.
This is measurement—of gender; of relevance of content and of socio-economic status; of a complete or verified identity; of membership; of relationships and interactions. =-1
Governance for responsible AI The second site of governance is decisions about algorithmic systems. Is the system good at what it is supposed to do? Does it work the way we expect? (And specifically, does it perform well on the training set? Does it generalize to other settings?) Is it ready for deployment, and for which contexts? Or even more directly: Is our system fair? Is it robust? Is it responsible? Taking a step back, where does “fair” or “robust” or “responsible” come from? These are themselves essentially contested constructs that are being operationalized. Measurement is governance here too. =-1
Thinking tools What of it then? This integrated perspective—measurement as governance—is a generative one. We have tools—i.e., construct validity and reliability—to unpack the measurement process in algorithmic systems and uncover sources of potential fairness-related harms—i.e., mismatches in the measurement process. Two types of validity, content and consequential, are particularly well-suited to explore governance decisions: Content validity elicits substantive understanding of the construct being operationalized, =-1 while consequential validity reveals the feedback loop of governance decisions and their impacts as a part of validity. =-1 Other types of construct validity and reliability may already be familiar to data scientists and engineers alike, including face validity (do the assumptions seem reasonable?), test–re-test reliability (this includes out of sample testing: do we get similar results if we re-run the system?), and convergent validity (do the measures correlate with others of the same phenomenon?). These types may be already familiar under different names or within different frameworks of best practices. Thus an important caveat is that naming which types of validity are being considered is not necessary to explore them. Asking the questions or interrogating assumptions, or assessing validity and reliability, can be done without the specific vocabulary. However, we can use the types of validity as inspiration for what questions we ought to ask ourselves and ask of our systems. =-1
Finally, understanding measurement as governance serves as a reminder that these assumptions will be inherently shaped by those making them. The contexts where these assumptions are made, and by whom, are not necessarily separable from the work itself. But we do better to articulate what assumptions are being made when, by whom, and to what downstream effects, when we consider this broader context (Figure fig-measurer: “when you measure, include the measurer”).=-1
Validity and governance
The tools that are available to interrogate assumptions (decisions) built in to sociotechnical systems, i.e., construct validity and reliability, can be used to interrogate governance practices. Furthermore, these tools can be used to connect with broader conversations on governance in and for AI. Here we explore several of those connections. These settings—non-exhaustive and illustrative—point to the breadth of opportunities for rigorously considering the often-implicit governance decisions built into sociotechnical systems. =-1
Content and consequential validity
Two types of validity are of particular relevance to governance in and for responsible AI: content validity and consequential validity.
Content validity Content validity captures two important places of mismatches: first, is there a coherent, agreed upon theoretical understanding of the construct of interest? And second, given a theoretical understanding of that construct, does the operationalization fully match that understanding? Many constructs of interest will face the first challenge, and also vary across contexts, time, and culture (fairness, for instance). Even if there is not an agreed upon definition, something is still being implemented: content validity then covers the substantive match between the operationalization, i.e., what is being measured, and the construct, i.e., what is intended to be measured. (Why bother with other types of validity then, if this apparently captures everything? Different types of validity, and the exploration one might pursue while trying to establish them, can reveal previously under- or mis-specified assumptions.) Content validity gets at both governance in responsible AI—e.g., have we adequately captured “creditworthiness” or “risk to society”?—and governance for responsible AI—e.g., when can we declare our system to be “fair” or “responsible”? This is a significant challenge—and even more so if that theoretical understanding is unstated or is understood differently by different stakeholders. For governance in AI, Passi and colleagues show how real-world organizations confront both theoretical mismatches and mismatches between operationalization and construct throughout the multistakeholder process of making data science systems work. For governance for AI, debates about content validity are more explicit, both in defining responsible AI systems and in operationalizing legal guidelines for algorithmic systems. =-1
Consequential validity Consequential validity considers impact. This is not obviously a part of validity, in part because its name connotes a normative assessment. The argument that Samuel Messick, a researcher at the Educational Testing Service, put forward was that how measures are defined changes how they are used—therefore defining the measure changes the world it was created for. That is, consequential validity must be a part of validity, as a measure’s operationalization changes how we understand that construct in the first place. More recently, Hand writes that “measurements both reflect structure in the natural world, and impose structure upon it”. That is, it is clear that there is a feedback loop between what we measure and how we interpret it: so to govern systems well, we must consider how the governance changes the system itself. =-1
The feedback loop inherent in measurement is recognized from different perspectives across the social sciences. One notion is as Campbell’s Law and its cousin Goodhart’s Law: “when a measure becomes a target it ceases to be a good measure”. Messick, and the broader educational community, refer to the evocatively-named washback, where incentives around testing lead to teaching to the test. In economics, these ideas appear through the Lucas critique and market devices,where models of the economy and policies then shape the economy; similar ideas appear in sociology. =-1 Philosophical and sociological understandings of performativity explore how enacting categories changes their meaningand how categories and value are socially co-constructed. This feedback loop has long been of interest in critical infrastructure studies and science and technology studies.[From Haraway: “Indeed, myth and tool mutually constitute each other.” =-1 Crucially, consequential validity formally brings systems-level feedback into our technical understanding of the measurement process. =-1
Fairness, robustness, and responsibility
Rehashing the full breadth of disagreements about how constructs such as fairness are operationalized (in algorithmic systems specifically, and governance systems more broadly) is beyond the scope of this work. Here we point to some perspectives made available through the lens of construct validity before discussing governance in practice in section subsec-govern. =-1
Fairness Fairness is an essentially contested construct with diverse, conflicting, and context-dependent understandings of the theoretical construct. Even with a given theoretical understanding of fairness, it is still nontrivial (and sometimes impossible) to operationalize the full theoretical scope of the intended definition, particularlyto implement “this thing called fairness”in meaningful applied settings (real-world organizations, legal systems). These are familiar challenges to the content validity of fairness: does the operationalization match the construct? =-1 However, content and consequential validity of fairness in algorithmic systems provide a wider view. In content and consequence, operationalizations of fairness almost always neglect notions of justice and power, and operationalizations based on static, narrow notions of fairness will perpetuate existing inequities. When encountering a “fair” system, however, different stakeholders may expect equitable or equal treatment, justice or dignity. The lens of consequential validity is key here: A system that is labeled as “fair” under a narrow or static definition may further exacerbate inequities simply by labeling inequitable outcomes as “fair.” Breaking out of this feedback loop must then be done intentionally. =-1
Robustness Robustness—asking how well a system performs, perhaps across contexts, or to do the thing it claims—may seem more neutral. Is the system good at what it is supposed to do? Does it work the way we expect? Is it ready for deployment? (And specifically, does it perform well on the training set? Does it generalize?) A growing literature has turned to the choices involved in how organizations evaluate these assessments of robustnessand, more broadly, the politics of seemingly technical decisions about model performance, into the politics of developing larger data sets and models in the name of robustness. Through the lens of consequential validity (i.e., it matters what we call things), implying that robustness only requires technical decisions obscures the hidden governance decisions at hand. =-1
Responsibility Finally, we can turn to responsibility. Without adjudicating different desirable properties of responsible algorithmic systems, we point to several threads: first, that responsibility is about harms. Centering harms foregrounds risk and power. Critical scholars have shown how pivoting to a human rights perspective, away from ethics and fairness, allows us to fundamentally attend to (mitigating) fairness-related harms. Second, naive attempts towards inclusion create opportunities for further harms. As with robustness, moving towards responsible systems through better auditing benchmarks provides an awkward path toward accountability. We now turn to ways that so-called responsible AI systems are governed more broadly, drawing from perspectives from measurement and construct validity. =-1
Governing (responsible) AI
The development and critique of governance in and for AI already uses the many of the perspectives discussed here. This is in no small part due to the framing of the challenge. If we begin from a focus on algorithmic systems in which measurement processes are mostly implicit, we can then use the perspectives given by measurement and construct validity to uncover the hidden governance decisions in algorithmic systems. However, if we begin from a focus on governance, then the relevance and use of the perspectives given by measurement and construct validity are self-evident: what do we want our system to achieve? What values or principles do we want to enact? How do we know if our system is upholding those values? What potential harms may emerge if we fail? (Of course, answering these questions remains a challenge.) Furthermore, the potential for harms arising from mismatches between the constructs intended to be operationalized and their operationalization is also more prominent: in 2016 Mittelstadt and colleagues wrote that the “gaps between the design and operation of algorithms and our understanding of their ethical implications can have severe consequences affecting individuals as well as groups and whole societies”. As such, efforts to operationalize responsible governance for responsible AI have focused on developing values, principles, and ethics statements (both widely discussed elsewhereand thoughtfully critiqued). While measurement perspectives may be more readily available, the challenges to meaningfully operationalize responsible AI are nontrivial in practice. With deep ambiguity involved in converting values into practice, governance for AI needs the social sciences, interdisciplinary and diverse teams, and broader systems perspectives.
An emphasis on measurement can unify existing work on governance. For instance, governance in the public sector relies on implicit measurement decisions that then shape policy, and governance tools like impact assessments operationalize harms and what must be done to mitigate them, i.e., impacts and assessments thereof are co-constructed. The consequences of governance decisions play out in other domains as administrative or symbolic violence—for instance when technical systems classify individuals in a way that misrepresent and exclude trans and nonbinary individualsor individuals with disabilities. Consequential validity specifically helps account for how meaning is made, encoded, and enforced (Alder: “measures are more than a creation of society, they create society”). We can then functionally connect our understanding of consequential validity to large and nearby conversations: for instance, race as and of technology, from the larger context of critical race theory. Similarly, by shifting the focus to harms—rather than, for instance, bias—we have the opportunity to reflect on what critical assumptions are being made; to reflect on what impacts are being addressed (unequal outcomes may be more or less equitable); to reflect on power (including from where decisions are being enforced, by whom, and who they impact); and to reflect on what social phenomena are being displayed—and for which the fixes may not be technical.=-1 Finally, explicitly connecting measurement and governance makes relevant lessons from past governance successes (and failures) in algorithmic systems as well as other complex social and organizational systems. =-1
Conclusion
Developing “responsible AI” is and will be a social, technical, organizational, and political activity. Angela Carter in Notes from the Front Line writes that “Language is power, life and the instrument of culture, the instrument of domination and liberation.” In algorithmic systems, it is the often-implicit measurement process where this instrumenting—where governance—happens. By understanding measurement as governance, we can bringcritical and social science perspectives more formally into our conceptions of responsible AI, while equipping researchers and practitioners with better tools and perspectives to develop responsible AI. =-1
79
#1 #1#1#1 #1 #1 #1 #1#1#1#1
[Abebe, Barocas, Kleinberg, Levy, Raghavan, and RobinsonAbebe et al2020] abebe2020roles authorpersonRediet Abebe, personSolon Barocas, personJon Kleinberg, personKaren Levy, personManish Raghavan, and personDavid G Robinson. year2020. Roles for computing in social change. In booktitleProceedings of the 2020 Conference on Fairness, Accountability, and Transparency . pages252–260.
[AlderAlder2002] alder2002measure authorpersonKen Alder. year2002. titleThe measure of all things: The seven-year odyssey and hidden error that transformed the world.
[ArnesonArneson2018] arneson2018four authorpersonRichard Arneson. year2018. Four conceptions of equal opportunity. journalThe Economic Journal volume128, number612 (year2018), pagesF152–F173.
[Bender, Gebru, McMillan-Major, and ShmitchellBender et al2021] bender2021dangers authorpersonEmily M Bender, personTimnit Gebru, personAngelina McMillan-Major, and personShmargaret Shmitchell. year2021. On the Dangers of Stochastic Parrots: Can Language Models Be Too Big?. In booktitleProceedings of the 2021 ACM Conference on Fairness, Accountability, and Transparency . pages610–623.
[BenjaminBenjamin2019] benjamin2019race authorpersonRuha Benjamin. year2019. booktitleRace after Technology: Abolitionist Tools for the New Jim Code .
[Bennett and KeyesBennett and Keyes2020] bennett2020point authorpersonCynthia Bennett and personOs Keyes. year2020. What is the point of fairness? journalInteractions volume27, number3 (year2020), pages35–39.
[BirhaneBirhane2021] birhane2021algorithmic authorpersonAbeba Birhane. year2021. Algorithmic injustice: a relational ethics approach. journalPatterns volume2, number2 (year2021), pages100205.
[Birhane and PrabhuBirhane and Prabhu2021] birhane2021large authorpersonAbeba Birhane and personVinay Uday Prabhu. year2021. Large image datasets: A pyrrhic win for computer vision?. In booktitle2021 IEEE Winter Conference on Applications of Computer Vision (WACV) . IEEE, pages1536–1546.
[Birhane and van DijkBirhane and van Dijk2020] birhane2020robot authorpersonAbeba Birhane and personJelle van Dijk. year2020. Robot Rights? Let’s Talk about Human Welfare Instead. In booktitleProceedings of the AAAI/ACM Conference on AI, Ethics, and Society . pages207–213.
[Blodgett, Barocas, Daumé III, and WallachBlodgett et al2020] blodgett2020language authorpersonSu Lin Blodgett, personSolon Barocas, personHal Daumé III, and personHanna Wallach. year2020. Language (Technology) is Power: A Critical Survey of" Bias" in NLP. journalProc. ACL (year2020).
[Bowker and StarBowker and Star2000] bowker2000sorting authorpersonGeoffrey C Bowker and personSusan Leigh Star. year2000. booktitleSorting things out: Classification and its consequences . publisherMIT Press.
[boyd and Crawfordboyd and Crawford2011] boyd2011six authorpersondanah boyd and personKate Crawford. year2011. Six provocations for big data. In booktitleA decade in internet time: Symposium on the dynamics of the internet and society .
[CampbellCampbell1979] campbell1979assessing authorpersonDonald T Campbell. year1979. Assessing the impact of planned social change. journalEvaluation and Program Planning volume2, number1 (year1979), pages67–90.
[CathCath2018] cath2018governing authorpersonCorinne Cath. year2018. Governing artificial intelligence: ethical, legal and technical opportunities and challenges. journalPhil Trans A (year2018).
[Cath, Latonero, Marda, and PakzadCath et al2020] cath2020leap authorpersonCorinne Cath, personMark Latonero, personVidushi Marda, and personRoya Pakzad. year2020. Leap of FATE: human rights as a complementary framework for AI policy and practice. In booktitleProceedings of the 2020 Conference on Fairness, Accountability, and Transparency . pages702–702.
[Chasalow and LevyChasalow and Levy2021] chasalow2021representativeness authorpersonKyla Chasalow and personKaren Levy. year2021. Representativeness in Statistics, Politics, and Machine Learning. journalProc ACM FAccT (year2021).
[Chouldechova and RothChouldechova and Roth2018] chouldechova2018frontiers authorpersonAlexandra Chouldechova and personAaron Roth. year2018. The frontiers of fairness in machine learning. journalarXiv preprint arXiv:1810.08810 (year2018).
[Delgado and StefancicDelgado and Stefancic2017] delgado2017critical authorpersonRichard Delgado and personJean Stefancic. year2017. booktitleCritical race theory: An introduction . Vol. volume20. publisherNYU Press.
[Denton, Hanna, Amironesei, Smart, Nicole, and ScheuermanDenton et al2020] denton2020bringing authorpersonEmily Denton, personAlex Hanna, personRazvan Amironesei, personAndrew Smart, personHilary Nicole, and personMorgan Klaus Scheuerman. year2020. Bringing the people back in: Contesting benchmark machine learning datasets. journalarXiv preprint arXiv:2007.07399 (year2020).
[Fish and StarkFish and Stark2020] fish2020reflexive authorpersonBenjamin Fish and personLuke Stark. year2020. Reflexive Design for Fairness and Other Human Values in Formal Models. journalarXiv preprint arXiv:2010.05084 (year2020).
[FloridiFloridi2018] floridi2018soft authorpersonLuciano Floridi. year2018. Soft ethics and the governance of the digital. journalPhilosophy & Technology volume31, number1 (year2018), pages1–8.
[FloridiFloridi2019] floridi2019establishing authorpersonLuciano Floridi. year2019. Establishing the rules for building trustworthy AI. journalNature Machine Intelligence volume1, number6 (year2019), pages261–262.
[GitelmanGitelman2013] gitelman2013raw authorpersonLisa Gitelman. year2013. booktitleRaw data is an oxymoron . publisherMIT press.
[GoldenfeinGoldenfein2019] goldenfein2018profiling authorpersonJake Goldenfein. year2019. The Profiling Potential of Computer Vision and the Challenge of Computational Empiricism. In booktitleProc.2019 ACM FAT Conference* .
[GreenGreen2021] green2021impossibility authorpersonBen Green. year2021. Impossibility of What? Formal and Substantive Equality in Algorithmic Fairness. journalPreprint, SSRN (year2021).
[Green and ChenGreen and Chen2021] green2020algorithmic authorpersonBen Green and personYiling Chen. year2021. Algorithmic risk assessments can alter human decision-making processes in high-stakes government contexts. journalProc. CSCW (year2021).
[Green and HuGreen and Hu2018] green2018myth authorpersonBen Green and personLily Hu. year2018. The myth in the methodology: Towards a recontextualization of fairness in machine learning. In booktitleProceedings of the machine learning: the debates workshop .
[Greene, Hoffmann, and StarkGreene et al2019] greene2019better authorpersonDaniel Greene, personAnna Lauren Hoffmann, and personLuke Stark. year2019. Better, nicer, clearer, fairer: A critical assessment of the movement for ethical artificial intelligence and machine learning. In booktitleProceedings of the 52nd Hawaii international conference on system sciences .
[HackingHacking1999] hacking1999social authorpersonIan Hacking. year1999. booktitleThe social construction of what? publisherHarvard university press.
[Hancox-Li and KumarHancox-Li and Kumar2021] hancox2021epistemic authorpersonLeif Hancox-Li and personI Elizabeth Kumar. year2021. Epistemic values in feature importance methods: Lessons from feminist epistemology. In booktitleProceedings of the 2021 ACM Conference on Fairness, Accountability, and Transparency . pages817–826.
[HandHand2016] hand2016measurement authorpersonDavid J Hand. year2016. booktitleMeasurement: A Very Short Introduction . publisherOxford Univ. Press.
[Hanna, Denton, Smart, and Smith-LoudHanna et al2020] hanna2020towards authorpersonAlex Hanna, personEmily Denton, personAndrew Smart, and personJamila Smith-Loud. year2020. Towards a critical race methodology in algorithmic fairness. In booktitleProceedings of the 2020 Conference on Fairness, Accountability, and Transparency . pages501–512.
[HarawayHaraway2006] haraway2006cyborg authorpersonDonna Haraway. year2006. A cyborg manifesto: Science, technology, and socialist-feminism in the late 20th century. In booktitleThe international handbook of virtual learning environments . publisherSpringer, pages117–158.
[HealyHealy2015] healy2015performativity authorpersonKieran Healy. year2015. The performativity of networks. journalEuropean Journal of Sociology/Archives Européennes de Sociologie volume56, number2 (year2015), pages175–205.
[HellmanHellman2020] hellman2020measuring authorpersonDeborah Hellman. year2020. Measuring algorithmic fairness. journalVa. L. Rev. volume106 (year2020), pages811.
[HoffmannHoffmann2019] hoffmann2019fairness authorpersonAnna Lauren Hoffmann. year2019. Where fairness fails: data, algorithms, and the limits of antidiscrimination discourse. journalInformation, Communication & Society volume22, number7 (year2019), pages900–915.
[HoffmannHoffmann2020a] hoffmannrawls authorpersonAnna Lauren Hoffmann. year2020a. Rawls, Information Technology, and the Sociotechnical Bases of Self-Respect. In booktitleThe Oxford Handbook of Philosophy of Technology .
[HoffmannHoffmann2020b] hoffmann2020terms authorpersonAnna Lauren Hoffmann. year2020b. Terms of inclusion: Data, discourse, violence. journalNew Media & Society (year2020), pages1461444820958725.
[Holstein, Vaughan, Daumé III, Dudík, and WallachHolstein et al2019] holstein2018improving authorpersonKenneth Holstein, personJennifer Wortman Vaughan, personHal Daumé III, personMiro Dudík, and personHanna Wallach. year2019. Improving fairness in machine learning systems: What do industry practitioners need?. In booktitleProc.CHI .
[HooverHoover1994] hoover1994econometrics authorpersonKevin D Hoover. year1994. Econometrics as observation: the Lucas critique and the nature of econometric inference. journalJournal of economic methodology volume1, number1 (year1994), pages65–80.
[Hutchinson and MitchellHutchinson and Mitchell2019] hutchinson2019fiftyyears authorpersonBen Hutchinson and personMargaret Mitchell. year2019. 50 Years of Test (Un) fairness: Lessons for Machine Learning. In booktitleProc. Conference on Fairness, Accountability, and Transparency (seriesFAT ‘19)* . publisherACM. 978-1-4503-6125-5
[Hutchinson, Prabhakaran, Denton, Webster, Zhong, and DenuylHutchinson et al2020] hutchinson2020unintended authorpersonBen Hutchinson, personVinodkumar Prabhakaran, personEmily Denton, personKellie Webster, personYu Zhong, and personStephen Denuyl. year2020. Unintended machine learning biases as social barriers for persons with disabilitiess. journalACM SIGACCESS Accessibility and Computing number125 (year2020), pages1–1.
[Jacobs, Blodgett, Barocas, Daumé III, and WallachJacobs et al2020] jacobs2020meaning authorpersonAbigail Z Jacobs, personSu Lin Blodgett, personSolon Barocas, personHal Daumé III, and personHanna Wallach. year2020. The meaning and measurement of bias: lessons from natural language processing. In booktitleProceedings of the 2020 Conference on Fairness, Accountability, and Transparency . pages706–706.
[Jacobs and WallachJacobs and Wallach2021] jacobs2021measurement authorpersonAbigail Z Jacobs and personHanna Wallach. year2021. Measurement and fairness. In booktitleProceedings of the 2021 ACM Conference on Fairness, Accountability, and Transparency . pages375–385.
[JafariNaimi, Nathan, and HargravesJafariNaimi et al2015] jafarinaimi2015values authorpersonNassim JafariNaimi, personLisa Nathan, and personIan Hargraves. year2015. Values as hypotheses: design, inquiry, and the service of values. journalDesign issues volume31, number4 (year2015), pages91–104.
[Kasy and AbebeKasy and Abebe2021] kasy2021fairness authorpersonMaximilian Kasy and personRediet Abebe. year2021. Fairness, equality, and power in algorithmic decision-making. In booktitleProceedings of the 2021 ACM Conference on Fairness, Accountability, and Transparency . pages576–586.
[KeyesKeyes2018] keyes2018misgendering authorpersonOs Keyes. year2018. The misgendering machines: Trans/HCI implications of automatic gender recognition. journalProceedings of the ACM on Human-Computer Interaction volume2, numberCSCW (year2018), pages1–22.
[Levy, Chasalow, and RileyLevy et al2021] levy2021algorithms authorpersonKaren Levy, personKyla E Chasalow, and personSarah Riley. year2021. Algorithms and Decision-Making in the Public Sector. journalAnnual Review of Law and Social Science volume17 (year2021).
[Lucas JrLucas Jr1976] lucas1976econometric authorpersonRobert E Lucas Jr. year1976. Econometric policy evaluation: A critique. In booktitleCarnegie-Rochester conference series on public policy , Vol. volume1. North-Holland, pages19–46.
[MacKenzieMacKenzie2008] mackenzie2008engine authorpersonDonald MacKenzie. year2008. booktitleAn engine, not a camera: How financial models shape markets . publisherMIT Press.
[Madaio, Stark, Wortman Vaughan, and WallachMadaio et al2020] madaio2020co authorpersonMichael A Madaio, personLuke Stark, personJennifer Wortman Vaughan, and personHanna Wallach. year2020. Co-designing checklists to understand organizational challenges and opportunities around fairness in AI. In booktitleProceedings of the 2020 CHI Conference on Human Factors in Computing Systems . pages1–14.
[MC HammerMC Hammer2021] hammertime authorpersonMC Hammer. year2021. booktitleTwitter . https://twitter.com/mchammer/status/1363908982289559553
“You bore us. If science is a `commitment to truth’ shall we site all the historical non-truths perpetuated by scientists ? Of course not. It’s not science vs Philosophy … It’s Science + Philosophy. Elevate your Thinking and Consciousness. When you measure include the measurer.” Tweet. February 21, 2021.
[MessickMessick1987] messick1987validity authorpersonSamuel Messick. year1987. Validity. In booktitleETS Research Report Series .
[MessickMessick1996] messick1996validity authorpersonSamuel Messick. year1996. Validity and washback in language testing. journalLanguage Testing volume13, number3 (year1996), pages241–256.
[Metcalf, Moss, Watkins, Singh, and ElishMetcalf et al2021] metcalf2021algorithmic authorpersonJacob Metcalf, personEmanuel Moss, personElizabeth Anne Watkins, personRanjit Singh, and personMadeleine Clare Elish. year2021. Algorithmic impact assessments and accountability: The co-construction of impacts. In booktitleProceedings of the 2021 ACM Conference on Fairness, Accountability, and Transparency . pages735–746.
[Mitchell, Potash, Barocas, D’Amour, and LumMitchell et al2021] mitchell2021algorithmic authorpersonShira Mitchell, personEric Potash, personSolon Barocas, personAlexander D’Amour, and personKristian Lum. year2021. Algorithmic Fairness: Choices, Assumptions, and Definitions. journalAnnual Review of Statistics and Its Application volume8 (year2021).
[MittelstadtMittelstadt2019] mittelstadt2019ai authorpersonBrent Mittelstadt. year2019. Principles alone cannot guarantee ethical AI. journalNature Machine Intelligence volume1, number11 (year2019), pages501–507.
[Mittelstadt, Allo, Taddeo, Wachter, and FloridiMittelstadt et al2016] mittelstadt2016ethics authorpersonBrent Daniel Mittelstadt, personPatrick Allo, personMariarosaria Taddeo, personSandra Wachter, and personLuciano Floridi. year2016. The ethics of algorithms: Mapping the debate. journalBig Data & Society volume3, number2 (year2016), pages2053951716679679.
[Mulligan, Kroll, Kohli, and WongMulligan et al2019] mulligan2019thing authorpersonDeirdre K Mulligan, personJoshua A Kroll, personNitin Kohli, and personRichmond Y Wong. year2019. This thing called fairness: Disciplinary confusion realizing a value in technology. journalProceedings of the ACM on Human-Computer Interaction volume3, numberCSCW (year2019), pages1–36.
[Muniesa, Millo, and CallonMuniesa et al2007] muniesa2007introduction authorpersonFabian Muniesa, personYuval Millo, and personMichel Callon. year2007. An introduction to market devices. journalThe sociological review volume55, number2_suppl (year2007), pages1–12.
[Passi and BarocasPassi and Barocas2019] passi2019problemformulation authorpersonSamir Passi and personSolon Barocas. year2019. Problem Formulation and Fairness. In booktitleProceedings of the Conference on Fairness, Accountability, and Transparency (Atlanta, GA, USA) (seriesFAT ‘19)* . publisherACM, addressNew York, NY, USA, pages39–48. 978-1-4503-6125-5 https://doi.org/10.1145/3287560.3287567
[Passi and JacksonPassi and Jackson2017] passi2017data authorpersonSamir Passi and personSteven Jackson. year2017. Data vision: Learning to see through algorithmic abstraction. In booktitleProceedings of the 2017 ACM Conference on Computer Supported Cooperative Work and Social Computing . pages2436–2447.
[Passi and SengersPassi and Sengers2020] passi2020making authorpersonSamir Passi and personPhoebe Sengers. year2020. Making data science systems work. journalBig Data & Society volume7, number2 (year2020), pages2053951720939605.
[Paullada, Raji, Bender, Denton, and HannaPaullada et al2020] paullada2020data authorpersonAmandalynne Paullada, personInioluwa Deborah Raji, personEmily M Bender, personEmily Denton, and personAlex Hanna. year2020. Data and its (dis) contents: A survey of dataset development and use in machine learning research. journalarXiv preprint arXiv:2012.05345 (year2020).
[PerryPerry2004] perry2004desi authorpersonImani Perry. year2004. Of Desi, J. Lo and color matters: Law, critical race theory the architecture of race. journalClev. St. L. Rev. volume52 (year2004), pages139.
[Raji, Gebru, Mitchell, Buolamwini, Lee, and DentonRaji et al2020a] raji2020saving authorpersonInioluwa Deborah Raji, personTimnit Gebru, personMargaret Mitchell, personJoy Buolamwini, personJoonseok Lee, and personEmily Denton. year2020a. Saving face: Investigating the ethical concerns of facial recognition auditing. In booktitleProceedings of the AAAI/ACM Conference on AI, Ethics, and Society . pages145–151.
[Raji, Smart, White, Mitchell, Gebru, Hutchinson, Smith-Loud, Theron, and BarnesRaji et al2020b] raji2020closing authorpersonInioluwa Deborah Raji, personAndrew Smart, personRebecca N White, personMargaret Mitchell, personTimnit Gebru, personBen Hutchinson, personJamila Smith-Loud, personDaniel Theron, and personParker Barnes. year2020b. Closing the AI accountability gap: defining an end-to-end framework for internal algorithmic auditing. In booktitleProceedings of the 2020 Conference on Fairness, Accountability, and Transparency . pages33–44.
[Rakova, Yang, Cramer, and ChowdhuryRakova et al2021] rakova2020responsible authorpersonBogdana Rakova, personJingying Yang, personHenriette Cramer, and personRumman Chowdhury. year2021. Where responsible AI meets reality: Practitioner perspectives on enablers for shifting organizational practices. journalProceedings of the ACM on Human-Computer Interaction volume5, numberCSCW (year2021), pages1–23.
[RidgewayRidgeway1991] ridgeway1991social authorpersonCecilia Ridgeway. year1991. The social construction of status value: Gender and other nominal characteristics. journalSocial Forces volume70, number2 (year1991), pages367–386.
[RobertsRoberts2011] roberts2011fatal authorpersonDorothy Roberts. year2011. booktitleFatal invention: How science, politics, and big business re-create race in the twenty-first century . publisherNew Press/ORIM.
[Sambasivan, Arnesen, Hutchinson, Doshi, and PrabhakaranSambasivan et al2021] sambasivan2021re authorpersonNithya Sambasivan, personErin Arnesen, personBen Hutchinson, personTulsee Doshi, and personVinodkumar Prabhakaran. year2021. Re-imagining algorithmic fairness in india and beyond. In booktitleProceedings of the 2021 ACM Conference on Fairness, Accountability, and Transparency . pages315–328.
[Sloane and MossSloane and Moss2019] sloane2019ai authorpersonMona Sloane and personEmanuel Moss. year2019. AI’s social sciences deficit. journalNature Machine Intelligence volume1, number8 (year2019), pages330–331.
[Sloane, Moss, Awomolo, and ForlanoSloane et al2020] sloane2020participation authorpersonMona Sloane, personEmanuel Moss, personOlaitan Awomolo, and personLaura Forlano. year2020. Participation is not a design fix for machine learning. journalarXiv preprint arXiv:2007.02423 (year2020).
[SpadeSpade2015] spade2015normal authorpersonDean Spade. year2015. booktitleNormal life: Administrative violence, critical trans politics, and the limits of law . publisherDuke University Press.
[StarStar1999] star1999ethnography authorpersonSusan Leigh Star. year1999. The ethnography of infrastructure. journalAmerican behavioral scientist volume43, number3 (year1999), pages377–391.
[Stark, Greene, and HoffmannStark et al2021] stark2021critical authorpersonLuke Stark, personDaniel Greene, and personAnna Lauren Hoffmann. year2021. Critical Perspectives on Governance Mechanisms for AI/ML Systems. In booktitleThe Cultural Life of Machine Learning . publisherSpringer, pages257–280.
[Stevens and KeyesStevens and Keyes2021] stevens2021seeing authorpersonNikki Stevens and personOs Keyes. year2021. Seeing infrastructure: race, facial recognition and the politics of data. journalCultural Studies (year2021), pages1–21.
[StrathernStrathern1997] strathern1997improving authorpersonMarilyn Strathern. year1997. ‘Improving ratings’: audit in the British University system. journalEuropean Review volume5, number3 (year1997), pages305–321.
[Wachter, Mittelstadt, and RussellWachter et al2021] wachter2021fairness authorpersonSandra Wachter, personBrent Mittelstadt, and personChris Russell. year2021. Why fairness cannot be automated: Bridging the gap between EU non-discrimination law and AI. journalComputer Law & Security Review volume41 (year2021), pages105567.
Attribution
arXiv:2109.05658v1
[cs.CY]
License: cc-by-4.0