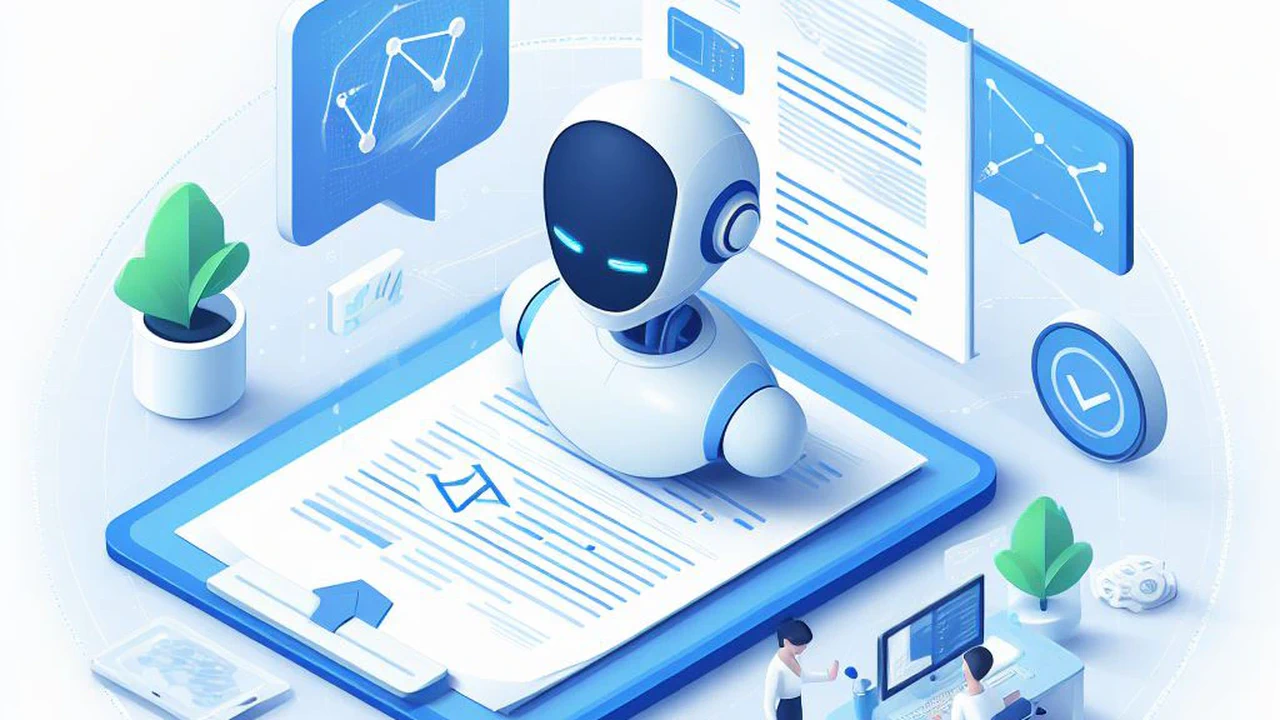
- Papers
Papers is Alpha. This content is part of an effort to make research more accessible, and (most likely) has lost some details from the original. You can find the original paper here.
Introduction
With the rapid growth of the AI industry, the need for AI and AI ethics expertise has also grown. Companies and governmental organizations are paying more attention to the impact AI can have on our society and how AI systems should be designed and deployed responsibly. From 2015 onward, a series of AI ethics principles, in-depth auditing toolkits, checklists, codebases, standards and regulationshave been proposed by many different international actors. Several communities of research and practice such as FATE (Fairness, Accountability, Transparency, and Ethics), responsible AI, AI ethics, AI safety and AI alignment have emerged. This general movement towards responsible development of AI has created new roles in the industry referred to as responsible AI practitioners in this paper. The primary mandate of these roles is understanding, analyzing, and addressing ethical and social implications of AI systems within the business context. The emergence of these roles challenges technology companies to curate these roles and teams. Leaders in AI-related organizations need to identify, recruit and train appropriate candidates for such roles. As the demand to fill such roles continue to increase, educators need effective means to train talent with the right set of skills.
Recently, scholars examined the common roles responsible AI practitioners serve, explored the challenges that they face, and criticized the problematic nature of the accountability mechanisms that relate to these roles. Moreover, others highlight the myriad practical challenges facing the development of a comprehensive training program to fill such roles. However, there is a lack of empirical research investigating the types of roles, corresponding responsibilities, and qualifications that responsible AI practitioners have in the industry. To address these gaps, we examine the following research questions:
- RQ1: What are the types of roles and responsibilities that responsible AI practitioners hold in the industry?
- RQ2: What are the skills, qualifications, and interpersonal qualities necessary for holding such roles?
We address these questions by conducting a two-part qualitative study. We examined 79 job postings from March 2020 to March 2022 and conducted expert interviews with 14 practitioners who currently hold these roles in the industry. Learning from fields of competency-based recruitment and curriculum development, we propose an ontology of different occupations and an accompanying list of competencies for those occupations.
As illustrated in Figure fig-teaser, our competency framework outlines seven occupations that responsible AI practitioners hold in the industry: researcher (of two kinds), data scientist, engineer, director/executive, manager, and policy analyst. For each occupation, the ontology includes a list of responsibilities, skills, knowledge, attitudes, and qualifications. We find that while the roles and responsibilities held by responsible AI practitioners are wide-ranging, they all have interdisciplinary backgrounds and are individuals who thrive in working with individuals from different disciplines. We discuss how educators and employers can use this competency framework to develop new curricula/programs and adequately recruit for the rapidly changing field of responsible AI development.
Background
With the increased media reporting and regulation requirements around social and ethical issues of AI-based products and services, the role of a responsible AI practitioner has emerged as a demanding position in the technology industry. In this section, we provide an overview of debates about these roles and existing educational programs that aim to train future responsible AI practitioners. We discuss how existing competency frameworks treat the role of a responsible AI practitioner and highlight the gaps we address in this work.
Emergence of the responsible AI practitioners
Considering the nascency of AI ethics as a domain, only a few scholars have characterized occupations held by responsible AI practitioners. For instance, Gambelin frames the role of an AI ethicist as “an individual with a robust knowledge of ethics” who has the responsibility and the ability to “apply such abstract concepts (i.e. ethical theories) to concrete situations” for the AI system. According to Gambelin, an AI ethicist in the industry also needs to be aware of existing policy work, have experience in business management, and possess excellent communication skills. Gambelin identifies bravery as the most important characteristic of an AI ethicist as they often need to “shoulder responsibility” for potential negative impacts of AI in the absence of regulation.
Moss and Metcalf investigated practices and challenges of responsible AI practitioners in Silicon Valley and described them as “ethics owners” who are responsible for “handling challenging ethical dilemmas with tools of tech management and translating public pressure into new corporate practices”. Echoing Moss and Metcalf’s seminal work on examining AI industry practices, a growing body of empirical work highlights that responsible AI practitioners face challenges such as misalignment of incentives, nascent organizational cultures, shortage of internal skills and capability, and the complexity of AI ethics issues when trying to do their day-to-day tasks. Furthermore, only large technology companies often have the necessary resources to hire responsible AI practitioners. Small and medium-sized companies struggle to access such expertise and rely on openly available information or hire external consultants/auditors as needed. This has given rise to AI ethics as consulting and auditing service.
While challenges in operationalizing responsible AI practices are an active area of research, there is a gap in understanding the role and necessary competencies of responsible AI practitioners in the industry.
Qualifications to be a responsible AI practitioner
The emergence of auditors in the field of responsible AI emphasizes the need for formal training and certification of such roles in the industry. This raises a few practical questions: Who is qualified to take these roles? How should these individuals be trained? Are existing computer science, engineering, and social science curricula prepare individuals for such roles?
Educators responded to this need by developing a range of educational programs and curricula. In a survey of the curricula for university courses focused on AI ethics, Garrett et al. emphasize that such topics should be formally integrated into the learning objectives of current and new courses. On the other hand, as Peterson et al. describe, discussing social and ethical issues in computer science courses remains a challenge. They propose pedagogues for fostering the emotional engagement of students in the classroom as a solution.
Recognizing the importance of interdisciplinary approaches in AI ethics, Raji et al. argue that computer science is currently valued significantly over liberal arts even in the research area of fairness of machine learning systems. Furthermore, they state that the perceived superiority culture in computer science and engineering has created a “new figure of a socio-technical expert”, titled “Ethics Unicorns” - full stack developers, who can solve challenging problems of integrating technology in society.
This overemphasis on computer science expertise and the trend toward integrating ethics content in existing technical curricula may be problematic if these efforts do not match the skills and disciplinary needs of the industry. It raises questions about whether the educational backgrounds of responsible AI practitioners today are indeed in computer science. In this work, we inform the curriculum development efforts across a diverse range of disciplinary areas by understanding these roles in the industry and outlining the attributes, qualifications, and skills necessary for holding them.
Competency frameworks in AI and AI ethics
Competency frameworks are useful tools for human resource management (i.e. recruitment, performance improvement) and educational development (i.e. new training programs and curriculum development in universities). Competency frameworks highlight different competencies required for a profession and link these competencies to skills and knowledge. According to Diana Kramer “competencies are skills, knowledge and behaviours that individuals need to possess to be successful today and in the future”. This definition frames our discussion of competency in this paper.
Competency frameworks help governmental and non-governmental organizations keep track of the type of skills their employees/general public need in the short and long term. Educators use these frameworks to update existing curricula and develop appropriate learning objectives. On the other hand, business leaders and human resource professionals use these frameworks for their recruitment practices.
Today’s existing competency frameworks do not sufficiently represent roles and competencies of a responsible AI practitioner. For example, ONET is United State’s national program for collecting and distributing information about occupations. ONET-SOC is a taxonomy that defines 923 occupations and they are linked to a list of competencies. Searching the taxonomy for “ethics”, “machine learning”, “data”, “security”, and “privacy” leads to minimal results such as “information security analysis”, “data scientist and “database architect”. The dataset do not include occupation titles such as machine learning engineer/researcher or data/AI ethics manager.
ESCO, the European skills, competencies, qualifications, and occupation is the European and multilingual equivalent of US’s O*NET. ESCO contains 3008 occupations and13890 skills. Searching for the above terms leads to more relevant results such as computer vision engineer, ICT intelligent system designer, policy manager, corporate social responsibility manager, ethics hacker, data protection officer, chief data officer, and ICT security manager. However, emerging occupations relevant to AI and AI ethics have not been well-represented in these established, Western competency frameworks.
As a response, a number of new AI competency frameworks have recently been developed. One such enabler is the series of projects funded by the Pôle montréalais d’enseignement supérieur en intelligence artificielle (PIA), a multi-institutional initiative in Montreal, Canada aimed to align educational programs with the needs of the AI industry. Six projects related to AI competency frameworks were funded – including the work presented in this paper. This resulted in an overarching AI competency for postsecondary education that includes ethical competencies, and a competency framework specific to AI ethics skills training. Bruneault et al., in particular, created a list of AI ethics competencies based on interviews of university instructors/professors already teaching courses related to AI ethics across North America.
Our work complements these collective efforts by providing a framework that represents the needs of the industry expressed in recent AI ethics-related job postings and the realities of the jobs AI ethics practitioners hold in nonprofit and for-profit corporations today.
Methodology
Practitioners and scholars of different domains typically create competencies frameworks using a process most appropriate for their needs. However, many follow a version of the process highlighted by Sanghi. The steps of the process are: 1) Define the purpose and performance objective of a position, 2) Identify the competencies and behaviors that predict and describe superior performance in the job, 3) Validate selected competencies, 4) Implement/integrate competencies and 5) Update competencies.
In this work, we focus on answering questions raised in the first two steps about the objectives of responsible AI practitioner roles and skills/qualities required to perform well in these positions. We take a two-pronged approach to understand the nature of emerging roles under the broad category of responsible AI practitioners in the industry. Firstly, we reviewed and analyzed job postings related to our working definition of responsible AI practitioner . Secondly, we interviewed individuals who are responsible AI practitioners in the industry today. We then synthesized data collected from these two sources through thematic analyses. We present our proposed competency framework in Section finding. This study was approved by the Research Ethics Board of our academic institution.
AI Ethicist Job Postings Review
We collected and analyzed 94 publicly available job postings over the period of March 2020 to March 2022. The job postings included a range of job titles, including researcher, manager, and analyst.The following sections describe the process for collecting, selecting, and analyzing these job postings that led to the development of the ontology of responsible AI practitioner roles and skills.
Collection of job postings
To collect “AI ethicist” job postings, we searched and scraped three job-finding websites, including LinkedIn, indeed.com, and SimplyHired, every two months from March 2020 to March 2022. We used the following search terms: AI ethics lead, Responsible AI lead, AI ethics researcher, data OR AI ethicist and fairness OR transparency researcher/engineer. Considering that search results only showed a few relevant job postings, we also collected job postings that came through referrals, including mailing lists such as FATML, 80000hours.org, and roboticsworldwide.
After scanning all the resulting job postings with the inclusion criteria, we gathered a total of 79 job postings for thematic analysis. We included the job postings that were published within our data collection period, were situated in the industry (including not-for-profit organizations), and outlined responsibilities with regards to implementing AI ethics practices in a given sector.[The table outlining the inclusion and exclusion criteria is in the supplemental material.]
Analysis
Using Braun and Clarke’s thematic analysis methodology, we analyzed the job postings with the coding scheme illustrated in Table tab-jp-coding.The lead author created this coding scheme after reviewing all the postings. The coding scheme was also informed by frequently used categories across competency frameworks explained earlier in section competencyframework.
The codes were generally split into four key elements: the company environment, responsibilities in the given occupation, qualifications, and skills. The codes of “company environment” and “qualifications - interdisciplinarity” are unique to this coding scheme due to their prevalence in the postings’ content.
After developing the first draft of the coding scheme, a student researcher was trained to use this scheme and coded 10% of the job postings. The student researcher’s analysis using the coding scheme was consistent with the lead researcher’s analysis of the same set of job postings. The discussion between the lead and student researcher helped clarify the description and examples for each code. However, there were no new codes that were added to the scheme. The lead author updated the coding scheme and coded the entire set of postings using the new scheme.
Expert interviews
The job postings provide a high-level analysis of the required skills and competencies expressed by recruiters; however, they may not represent the reality of these roles. Therefore, we conducted 14 interviews with experts who currently hold responsible AI practitioner positions in the industry. The focus of the interviews was on understanding the responsibilities, qualifications, and skills necessary for these roles. Considering the objective of this research project on the type of roles and skills, we did not acquire any demographic information about the participants in these roles. This also ensured that we can maintain the anonymity of these participants considering that a limited number of people hold these positions.
Recruitment
We compiled a list of potential interview candidates through (a) referrals within the authors’ professional network and (b) we used similar search terms as the ones highlighted for job postings to look for people who currently hold these positions. Moreover, we also considered people from the industry who had accepted papers at relevant conferences such as FAccT and AIES in 2020 and 2021. The suitable participants:
- worked for a minimum of three months in their role;
- held this position in the industry or worked mainly with industrial partners;
- held managerial, researcher, technical positions that are focused on implementing responsible AI practices within the industry.
We did not interview researchers or professors in academic institutes and only interviewed those holding positions at nonprofit and for-profit companies. While we only used the search terms in English to find interview participants for practical reasons, we did not limit our recruitment efforts to a geographical region given the limited number of individuals holding these roles across the industry. We recruited and conducted interviews from June 2021 - February 2022.
Interview protocol
The primary researcher conducted all fourteen interviews. All of the interviews were 45 to 60 minutes in length. The interviewer first described the project and obtained the participant’s consent. The interview was semi-structured with ten questions focused on exploring the following four topics:[The detailed interview protocol is included in the supplementary material.]
- Background and current role
- Situation your work, projects in AI ethics
- Skills, knowledge, values
- Looking into the future
Data Analysis
The interviews were audio recorded and transcribed. The primary researcher checked and corrected the transcriptions manually afterwards. The authors analyzed the interviews deductively and inductively. The lead author applied the coding scheme derived from the job postings () to the interview transcripts. Furthermore, a reflexive thematic analysiswas done by the lead author to capture the rich and nuanced details that were not represented in the coding scheme for the job postings. The results from the reflexive analysis were used to contextualize and improve the coding scheme from the job postings. However, no new codes were added to the scheme considering the focus of the research objective addressed in this paper. The lead author presented the coding scheme to the full research team, including a student researcher who had independently reviewed the data to identify general trends. The coding scheme was iteratively revised and refined based on the feedback from the research team.
Author reflexivity and limitations
We recognize that this research reflects our positionality and biases as academics in North America. Furthermore, the data we collected were all in English and they were representative of job postings and positions in companies situated in North America and Europe. We were not able to collect data on job postings and candidates representing existing efforts in Asia and the Global South. Furthermore, we recognize that the roles in this field are continually shifting. Therefore, this ontology is only a snapshot of the roles and skills that responsible AI practitioners have and are recruited for today. Further iterations on these types of frameworks will be necessary in the future as these roles evolve. Finally, this study focuses on examining responsibilities, qualifications, and skills required of today’s practitioners independent of their demographic factors (e.g., gender, age). We recognize the importance of representing a demographically diverse group of individuals and their experiences in qualitative research such as ours. Once responsible AI practitioners become a common occupation held by many, future studies should include demographic factors as part of similar investigations.
Proposed competency framework for responsible AI practitioners
From our analysis, we developed a preliminary competency framework that captures seven classes of existing occupational roles and several emerging classes of occupations. Figures postingchart and interviewchart show how each occupation type was represented in the job postings and interviews. Three of the occupations require technical expertise (researcher, data scientist, and engineer), two require policy expertise (researcher, policy analyst), and the remaining two are managerial (manager, director). In the following sections, we provide a detailed description of the responsibilities, skills, qualifications, and qualities for each of these roles.
Researcher (technical)
The most common class of occupations found in the job postings was that of a researcher focused on technical aspects of fairness, explainability, safety, alignment, privacy and auditability of AI systems (24 job postings, 2 interviews). Employers represented in this dataset were looking to hire researchers at varying levels of seniority (assistant, associate and principal). The main responsibilities of these researchers are split into four main categories: conducting research, communicating their findings, working with other teams (internally and externally), and developing novel solutions for identified problems. As expected, research directions set by these researchers need to support company-specific needs, and there is an emphasis on communication between researchers and product, legal and executive teams.
Skills The researchers in this group need to have a mix of technical skills (i.e. software engineering and programming languages such as Python), research skills (i.e. analytical thinking and synthesis of complex ideas ), and leadership skills (i.e. leading and guiding fellow researchers). The dataset from the job postings emphasized equally all these skills, and more senior positions emphasized leadership skills. A senior researcher explained that they look for “different research skills” depending on the project; however, they generally look for “some background in machine learning, statistics, computer science or something of that nature” and hire candidates that have some “interdisciplinary background” . The data from the postings and the interviews show a strong emphasis on good verbal and written communication skills. Participants highlighted the ability to publish in academic venues and some emphasized the ability to communicate with different audiences internally (i.e. product teams and executives) and externally (policy-makers and executives). A technical researcher emphasized the importance of_“convincing stakeholders”_ and creating “strategic collaborations” by communicating with practitioners with “diverse” backgrounds.
Qualifications The job postings mainly aim to attract candidates who have a PhD in computer science or a related field. Few of the job postings accept a master’s in these fields, whereas some do not highlight a specific degree and mainly focus on necessary skills and knowledge. The majority of postings have a heavy emphasis on the required experience. Interview participants also emphasized the importance of experience. A research manager expressed that they are not necessarily looking for a “PhD in computer science” . They are looking for candidates with experience in “leading and executing a research agenda” , working with different people and teams, synthesizing and “communicating challenging concepts” , and practicing software engineering. Some postings highlight experience with implementing AI ethics-related concepts. However, this was often listed as a preferred qualification rather than a required one. Similarly, researchers we interviewed, echoed the importance and value of having a publication record in “Fairness, Accountability, Transparency, and Ethics (FATE) communities” such as ACM Conference on Fairness, Accountability, and Transparency (FAccT) and AAAI/ACM Conference on AI, Ethics, and Society (AIES).
Interpersonal Qualities The most common attitude/value was the aptitude and interest to collaborate and work in an interdisciplinary environment . A researcher emphasized that the current conversations are “engineering focused” and they actively incorporate perspectives from_social science and philosophy by collaborating with experts in these areas_ . The most desired value was “curiosity to learn about [responsible AI] problems” . Many of the participants highlight other values and attitudes such as “passion” towards building safe and ethical AI systems, willingness to manage uncertainty and challenges , creativity , and resourcefulness .
Data scientist
The data scientist occupation is represented in 10 job postings in our dataset, and none in the interviews. The job postings seek to fill traditional data scientist roles with an added focus on examining responsible AI-related issues. The common responsibilities outlined for these positions are a) to collect and pre-process data, and b) to develop, analyze, and test models – these are typical of existing data science roles. However, the job postings emphasize the position’s responsibility to test machine learning models for AI ethics concerns such as fairness and transparency. Data scientists who work in the responsible AI domain have additional non-conventional roles. These roles include understanding and interpreting existing regulations, policies, and standards on the impact of AI systems and testing the systems’ capability for elements covered in these policies. They also need to work with technical and non-technical stakeholders to communicate findings, build capacity around responsible AI concepts and engage them as needed.
Skills The job postings put a heavy emphasis on advanced analytical skills and the ability to use programming languages such as R, Python and SQL for basic data mining. The ability to learn independently in a new domain and master complex code base is also listed as one of the key skills. A few of the postings list project management and organizational skills; however, this is not common. When it comes to the knowledge required, the focus shifts from the technical domain to an understanding of fields such as sociology, critical data studies, and AI regulations. Many postings highlight that potential candidates need to be familiar with concepts such as AI/ML auditing, algorithmic impact assessments, assessment of fairness in predictive models, explainability, robustness, and human-AI interaction. Technical knowledge, such as understanding transfer-based language models and logistic regression model development, is also highlighted in the posting. Lastly, the job postings outline the need for strong interpersonal, verbal, and written communication skills. However, experience publishing and presenting at academic venues is not mentioned.
Qualifications The majority of the job postings require a bachelor’s degree in quantitative fields such as data science and computer science and prefer higher degrees (master’s or Ph.D.). Companies are looking for candidates who have experience in data science, software engineering, and worked with large language models. Moreover, they are looking for experience in putting responsible AI principles into practice, evaluating the ethics of algorithms, and having basic familiarity with law and policy research. The ability and experience to translate AI ethic principles into practice are heavily emphasized throughout these job postings.
Interpersonal Qualities The job postings emphasize the ability to work with people from different backgrounds. However, these job postings do not include a comprehensive list of values. A few postings mention being a self-starter, working collaboratively to resolve conflict, and caring deeply about the data used to train ML models as key attitudes. Being flexible, innovative, curious, adaptive, and passionate about tackling real word challenges are also some of the sought-after values.
Engineer
The engineer occupation is represented in 8 of the job postings. None of our interview participants belong to this category. The key responsibility of an engineer practicing AI ethics is to help establish a safety culture and system within an organization by developing technical tools. They are tasked with developing a workflow for modeling and testing for issues such as bias, explainiability, safety, and alignment of AI systems. As part of this, engineers need to create code bases that could be used across the AI system development pipeline based on existing and evolving best practices.
Skills and Qualifications Job postings for engineers place a significant emphasis on experience-based qualifications and skills. The companies represented in this dataset are looking for skills and experience in software development, dataset production pipelines, researching fairness and safety implications of ML systems, and the development of large language models. They are also looking for experience working in a fast-paced technology company. Based on these qualifications, the main set of skills are programming and AI/ML development skills and this needs to be supported by knowledge and familiarity with foundational concepts in AI/ML, fairness, explainability, system’s safety, and safety life cycle management. Lastly, most of the job descriptions do not have a heavy emphasis on communication skills. Only a few mention excellent written and oral communication skills as a requirement.
Interpersonal Qualities In contrast to the lack of emphasis on communication skills, these postings have a particular focus on the attitude and values of ideal candidates more so than any other occupation category. These attitudes include being result-oriented, willingness to contribute as needed (even if not specified) and keen to learn new concepts. They are looking for people who value working on challenging problems and care about the societal impact of their work.
Researcher (law, philosophy and social sciences)
The second most frequent category of postings belongs to researchers that focus on topics such as policy, sociotechnical issues, and governance (14 job postings, 3 interviews). We created a separate group of positions as their responsibilities, skills, and qualities are sufficiently different from the technical researcher position. Candidates in this category need to conduct research, perform ethics or impact assessments of AI systems, act as a liaison and translator between research, product, policy, and legal teams, and lastly, advise on policy, standards, and regulations-related matters internally and externally. When conducting research, two different focus areas come up in the job postings: testing and evaluating AI system to inform policy and researching existing policies/regulations, and translating them into practice.
Skills The job postings highlight two sets of distinct skills for this group of researchers. Firstly, these researchers require a basic level of programming, advanced analytics, and data visualization skills. Few positions highlighted the need for even more advanced ML and AI skills. It is noteworthy that despite these researchers’ focus on policy, governance and sociotechnical issues, the postings still require them to have some data analytic skills. Secondly, these researchers need to have excellent facilitation, community-building, and stakeholder engagement skills. These two skills need to be complemented by strong leadership and management skills. The job postings heavily emphasize strong communication skills for this group of researchers. Besides the conventional skill of presenting and publishing papers, this group of researchers need to effectively work across different functionalities and disciplines. On a similar trend, these researchers need to have expertise in a variety of areas. They need to have a good understanding of “qualitative and quantitative research methods” , reliably know the current and emerging_“legal and regulatory frameworks and policies”_ , be “familiar with AI technology” and have a good knowledge of practices, process, design, and development of AI technology. This is a vast range of expertise and often “very difficult to recruit” for as highlighted by our expert interviewees.
Qualifications Just over half of the job postings list a Ph.D. in relevant areas as a requirement, including human-computer interaction, cognitive psychology, experimental psychology, digital anthropology, law, policy, and quantitative social sciences. Two postings require only a bachelor’s or a master’s in the listed areas. Similar to the technical researcher occupation, some positions do not specify any educational requirements and only focus on experience and skills. Our expert interviewees in this category are from a range of educational backgrounds ranging from a master’s in sociotechnical systems, a law degree combined with a background in statistics, and a master’s in cognitive systems.
Besides experience in research, companies are looking for experience in translating research into design, technology development, and policy. A researcher explained that they need to do a lot of “translational work” between the academic conversation and product teams in companies. A good candidate for this occupation would have “project management” , “change management” , “stakeholder engagement” , and “applied ethics” experience in a “fast-paced environment”. All four of these skills do not appear in all of the job postings and interview discussions. However, a permutation of them appears throughout the job posting data and participants’ responses.
Interpersonal Qualities
As emphasized strongly in both of the datasets, ideal candidates in this category need to have a “figure-it-out somehow” or “make it happen” attitude as explained by a participant. They are “driven by curiosity and passion towards” issues related to responsible AI development and are excited to engage with the product teams. Participants noted that ideal candidates in these roles are “creative problem solvers” who can work in a “fast-changing environment” .
Policy analyst
Policy analyst occupation is the least represented [1 expert interview, 4 job postings] in our data sources; however, considering the consistent list of competencies, we decided to include it within the proposed framework. The role of a policy analyst is to understand, analyze and implement a given policy within an organization. Moreover, they need to engage with policymakers and regulators and provide feedback on existing policies.
Skills and Qualifications A policy analyst needs to have proven knowledge of laws, policies, regulations, and precedents applicable to a given technology when it comes to AI ethics-related issues. Moreover, all of the job postings highlight the importance of familiarity with AI technology. According to the job postings, a good candidate would have experience in interpreting policy and developing assessments for a given application. They also need to be skilled in management, team building, and mentorship. This finding echoes remarks from expert interviews. Even though none of the job postings specify an educational degree requirement, the expert we interviewed was a lawyer with a master’s in technology law.
Interpersonal Qualities The job postings in this category heavily emphasized values and attitudes. A good analyst needs to have sound judgment and outstanding personal integrity. They should be caring and knowledgeable about the impact of technology on society. Moreover, they enjoy working on complex multifaceted problems and are passionate about improving governance of AI systems. The expert interviewee’s perspective closely matches these attributes. Participants elaborated that they needed to be “brave” and “step up to ask questions and challenge status quo consistently over a long time” . As expected communications skills are considered critical for success. The expert interviewee significantly emphasized the importance of “networking as a key factor” in succeeding in their role.
Manager
We analyzed 7 management-related job postings and 5 expert interviewees in this category. The product managers take the role of incorporating responsible AI practices in the product development process. In contrast, program managers are often leading and launching a new program on establishing AI ethics practices within the organization. These programs often involve building an organization’s capacity to manage responsible AI issues.
Skills For both streams of management, the potential candidates need to have strong business acumen and a vision for the use/development of AI technology within an organization. Some of the key management skills highlighted in the job postings include the ability to manage multiple priorities and strategically remove potential blockers to success. Another sought-after skill is the ability to effectively engage stakeholders in the process. Expert interviewees also echoed the importance of this skill as their roles often involve getting people “on board with new ways of thinking and creating” . According to the job postings, good candidates for management need to have a practical understanding of the AI life cycle and be familiar with integrating responsible AI practices into a program or a product. Our interviewees note that they continuously need to “learn and keep up with the fast-paced development of AI” .
Qualifications Not many postings have highlighted educational qualifications and instead focused on experience qualifications. However, the main educational qualification is a bachelor’s degree with a preference for higher degrees. The postings have primarily highlighted a degree in a technical field such as computer science or software engineering. Interestingly the interviews reflect a different flavor of educational backgrounds. All of the experts we interviewed had at minimum a master’s degree and the majority of them completed their studies in a non-technical field such as philosophy, media studies, and policy. However, these individuals had acquired a significant level of expertise in AI ethics through “self-studying” and “engaging with the literature” and the responsible AI “community” . For example, two of the participants trained in technical fields and had a significant level of industry experience. Similarly, they had learned about responsible AI through their own initiative.
On the other hand, the job postings heavily focus on experience , including a significant amount of technical know-how, experience focused on ML development, product and program management , and implementation of ethical and social responsibility practices within fast-paced technology companies. The interview participants had been “working in the industry for some time” before taking on these management roles. However, their range of experiences do not cover all of the required experiences outlined in the job descriptions. As expected, excellent communication skills are noted in the job descriptions and strongly echoed by the experts as well. The job postings do not necessarily elaborate on the nature of communication skills; however, the experts note that the “ability to listen” , understand , and sometimes “persuade different stakeholders” is key in such roles.
Interpersonal Qualities Few of the job postings make remarks about attitudes/values and highlight that managers need to value designing technology for social good and cooperation with other stakeholders. A good candidate for management shouldfoster a growth mindset and approach their work with agility, creativity, and passion. All of the participants expressed their passion for developing ethical technologyand indicate that they took a lot of initiative to learn and contribute to the field within their company and externally before they could take on their management roles.
Director
The job descriptions dataset has 4 postings for director positions and 2 of the expert interviewees have directorship roles. According to the job postings, director responsibilities include at least three of the following: a) lead the operationalization of AI ethics principles, b) provide strategic direction and roadmap towards enterprise-wide adoption and application of ethical principles and decision frameworks, and c) build internal capacity for AI ethics practice and governance. Depending on the nature of the organization and its need to incorporate AI ethics practices, these responsibilities vary in scope. For example, a director within a technology start-up will only be able to commit “limited amount of time to operationalizing AI ethics principles and building internal capacity” compared to a director within a larger technology company.
Skills and qualifications According to the job postings, the key skill for being a director is having the ability to build a strong relationship with a broad community that helps define and promote best practice standards of AI ethics. An ideal director can effectively pair their technical skills/know-how with their management skills and policy/standards knowledge to develop strategic plans for the company. Experience in directing and leading teams, particularly in social responsibility practices within technology companies is highly valued for such positions. Only one job posting specifies an educational (a bachelor’s related to policy development and implementation). Others only highlight experience. The two interviewees hold master’s degrees in business and information systems respectively. They also had extensive industry experience that was not directly in AI ethics. However, their experience involved “translation of policy within a technology application” .
Interpersonal Qualities As expected, according to the job postings a good candidate for directorship needs to have exceptional written and verbal communication skills, need to be able “to articulate complex ideas” to technical and non-technical audiences, “engage and influence stakeholders” and “collaborate with people from different disciplines, and cultures” . This set of skills was reflected in our expert interviews. Both interviewees emphasized how they maintain a good flow of communication with the employees and how they remain always open to having conversations on a needs basis. This allowed them to build trust within the company and pursue moving forward with their strategic plan. The job postings highlight the ability to earn trust in relationships as a sought-after value for a directorship role. A director should also be able to challenge the status quo, be passionate about good technology development, be comfortable with ambiguity , and adapt rapidly to changing environment and demands . Most importantly, a director needs to have “a strong and clear commitment to the company values” as they set the tone for others within the organization.
Emerging occupations
Besides the abovementioned classes of occupations, we found a few other positions that do not map easily to any of the existing categories. Considering the limited number of these positions, they do not justify a category of their own. However, we note these emerging roles to understand how they might shape up the responsible AI profession. These occupation titles include data ethicists (2 in job postings), AI ethics consultants (2 in interviews), dataset leads (2 in job postings), communication specialist (1 in job postings), safety specialist (1 in job posting) and UX designer (1 in job postings). The following describes the main function of these positions:
- Data ethicist: manage organizational efforts in operationalizing AI ethics practices through policy and technology development work. This role has similarities to the role of a policy analyst and data scientist.
- AI ethics consultant: apply their expertise in AI ethics to solve pain points for consulting clients.
- Dataset lead: curate datasets while accounting for fairness and bias-related issues.
- Safety specialist: use and test large language model-based systems to identify failures and errors.
- AI ethics communication specialist: write communication pieces that focus on AI ethics issues.
- UX designers: design user interfaces with ethics in mind.
Future of the responsible AI profession
Our interview participants shared a variety of responses to the question “what will the future of their job be like?”. Some participants thought that eventually, “everyone in a company will be responsible” for understanding ethical and social issues of AI as part of their job. In this scenario, everyone would need to have the appropriate knowledge and skillset to apply responsible AI practices in their work or at least know when they need to ask for advice from internal or external experts.
On the contrary, many participants expressed that “dedicated roles” need to be recruited. These participants elaborate that recruitment for these roles is and will “continue to be challenging” as it is difficult to find people with interdisciplinary backgrounds and established industry work experience. Many of the managers we interviewed have chosen “to build teams that come from different disciplinary backgrounds” and provide “professional development opportunities” on the job. However, they also described that hiring people into these roles is challenging since corporate leaders are not always willing to invest a lot of resources in AI ethics. This often can lead to “exhaustion and burn-out” for individuals who currently hold these roles - this is especially true for small and medium-sized technology companies. According to participants, this will likely change with a progressive shift in the regulatory landscape.
Discussion
Educators and employers play a pivotal role in shaping a responsible AI culture. In our efforts to create a competency framework that outlines the range of roles for responsible AI practitioners, we find that such frameworks can not only guide corporate leaders to recruit talent but also help grow their responsible AI capacity.
We find that the ability to work in an interdisciplinary environment, communicate and engage with diverse stakeholder groups, and the aptitude for curiosity and self-learning are consistently highlighted for all of the roles. This emphasizes the need to foster an environment where students and existing employees in different roles are encouraged to adopt interdisciplinary approaches/collaboration and explore responsible AI content.
In this section, we articulate how an interdisciplinary environment can be fostered, the importance of organizational support for responsible AI practitioners, and the need to proactively monitor the rapidly changing occupational demand and landscape for these roles.
Being able to work in an interdisciplinary environment is critical
Our results show that many of the responsible AI practitioners today come from non-traditional, non-linear, and interdisciplinary educational and work backgrounds to their current positions. The educational and work experiences of these participants span a multitude of fields and allowed them to develop a strong set of skills in navigating disciplinary boundaries and understanding problems from diverse perspectives. The participants often described their role as a translator and facilitator between different groups and disciplines within the organization. For instance, they remarked that a concept such as fairness, transparency, or ethically safe has completely different meanings depending on the personal and professional backgrounds of their audience. The participants often needed to translate what these concepts mean across different disciplinary boundaries (i.e. statistics and law).
Notably, while the job postings asked for a diverse array of skills and qualifications from multiple disciplines, those who hold such positions today are often specialized in one or two disciplines. However, they had been exposed to and worked across multiple disciplines in their professional career. The most important asset that our interviewees emphasized was being able to work across disciplinary boundaries. The candidates who successfully hold such positions are_not “ethical unicorn, full stack developers”_. However, they have honed the skills necessary to translate and create solutions to responsible AI issues across multiple disciplines. Building on existing proposal to improve responsible AI practicesand education, we posit that AI team leaders need to pay a special attention to hiring individuals with the capability to create, critique and communicate across multiple disciplines . Consider Furthermore, educators can get inspiration from education models in highly interdisciplinary fields such as healthcare and create curricula/spaces where students work with peers from different academic backgrounds.
Responsible AI practitioners are advocates - but they need organizational support
We find that responsible AI practitioners are often highly driven and motivated to make a positive impact. These individuals often hold a strong sense of valuing social justice and want to ensure that AI technology is developed in a way that is good for society’s well-being. One of the most consistent ideas that came through in the interviews is the attitude that the participants had toward their careers. Many of the interview participants took the time to immerse themselves in learning new topics and expressed that they were self-motivated to do so. This is especially true for the individuals who are taking some of these first positions in the industry. When looking at the career trajectory of many of the participants, we observe that they often created their own roles or came into a newly created role. Moreover, these individuals often needed to start their own projects and create relationships with others in the organization to measure their own progress and establish credibility.
Similar to any emerging profession many of the participants act as champions for ethical and safe development of AI. They are often working in an environment that questions and challenges the need for considering AI ethics principles. As some of the participants remarked, they often have to answer questions such as “why do we need to pay for ethics assessments?” ,“what is the value of considering AI ethics in a start-up?” , or “why should we put in the time? what is the value added?” . This act of advocating for AI ethics is even more challenging when existing regulations do not have proper enforcement mechanisms for responsible AI practices. Many of the participants assume the role of an advocate and often use their excellent communication skills to build relationships and capacity within their organization.
For the successful implementation of responsible AI practices, it is important that business leaders pay attention and support the advocacy efforts of these practitioners. Many of today’s responsible AI practitioners are working with limited resources, have critical responsibilities, and are experiencing burn-out. Whenever possible, leaders in AI companies need to create appropriate incentive structures, provide the necessary resources and communicate the value of establishing responsible AI practices to their employees so that these practitioners have the necessary support for the effective execution of their responsibilities. Recognizing the nature of these roles, educators can learn from existing methodsand integrate leadership training into their curricula when addressing responsible AI-related content.
Educators and employers need to monitor and plan for the rapidly changing landscape of responsible AI roles
The nature of occupations in the AI industry is continually growing and shifting. The rapid technological development, upcoming regulationsand global economic conditionsimpact how companies recruit and retain responsible AI expertise. Furthermore, there is a need for new educational efforts and programs for preparing new graduates to take on responsible AI practices. The proposed ontology provides a synthesis of roles that have emerged in responsible AI practice and it can serve as a planning tool for corporate leaders and educators.
Corporate leaders can use this ontology to build internal capacity for individuals who currently hold researcher, data scientist, engineer, policy advisor, manager, and director roles in their institutions. Depending on these companies’ responsible AI needs and resources, business executives can work towards creating interdisciplinary teams for establishing responsible AI practice by recruiting individuals with the competencies outlined for each of these roles. Besides recruiting and fostering for responsible AI competencies, these leaders need to communicate the importance of these practices and start by creating the appropriate organizational incentives and resources for adapting responsible AI practices. Government and non-governmental organizations could support such efforts, particularly small and medium-size companies, by formally recognizing such roles in their taxonomies of occupationsand providing resources.
Current computer science and engineering education focuses primarily on teaching professional ethics. There is minimal focus and resources on cultivating skills and knowledge required for cultivating the skills that focus on ethics in design. On the other hand, there is a lack of clarity of how much students in social and political sciences need to work on their technical acumen to become skilled responsible AI practitioners. Educators could use the list of competencies to develop a set of learning objectives and examine the efficacy of different teaching pedagogies in supporting these objectives. Moreover, Educators can use the competency framework as a tool for acquiring resources for further curricula and program development.
Notably, the proposed ontology primarily focuses on type of roles, responsibilities and skills without addressing other important factors in recruitment and education efforts such as diversity of individual who get to learn about responsible AI issues or take such roles in the industry. Therefore, it is critical that users of this ontology, consider factors that are not captured in the scope of this ontology. Furthermore, considering the rapidly changing conversation around responsible AI practices, the type of roles in this onotlogy will shift and expand. We invite the community of researchers , practitioners and educators to reflect on these roles and build on this ontology.
Conclusion
With the increased regulatory activities in the industry, companies have the incentive to ensure responsible AI development. In this work, we found seven different type of roles and their corresponding responsibilities, skills, qualifications, and interpersonal qualities expected in today’s responsible AI practitioner. We propose a preliminary competency framework for responsible AI practitioners and highlight the importance of creating interdisciplinary teams and providing adequate organizational support for individuals in these roles.
We thank our study participants for taking the time to share their experiences, expertise, and feedback. We also thank our anonymous reviewers for their deep engagement and valuable feedback on this paper. This work benefitted greatly from the data collection and analysis assistance from our collaborators Sandi Mak, Ivan Ivanov, Aidan Doudeau, and Nandita Jayd at Vanier College, Montreal. We are grateful for their contributions. Finally, this work was financially supported by the Natural Sciences and Engineering Research Council of Canada and Pôle montréalais d’enseignement supérieur en intelligence artificielle.
Supplementary Material
Interview protocol
Consent Process Thank you for reading and signing the Human Subjects Consent Form for this project..
Introduction Thank you for agreeing to take part in this study. My name is [interviewer] and I will be conducting this interview. I am a research assistant working with [advisor]. We have invited you to take part in this today because of your current role. The purpose of this study is to examine the experiences of professionals including ethicists, technologists and business leaders who are dealing with ethical and social implications of particular AI technology through development and implementation.
Today I am playing two roles: that of a interviewer and that of a researcher. At this time I would like to give a brief overview of the project and the consent form. [5min]
Background and current role
- Please tell us about your role at your current company.
- What is your official job title, and what are you main responsibilities?
- Could you please tell me about your background, expertise and experience that led you to take on your current role?
- Who do you work most closely with at your company? Who do you manage?Who do you report to? Who are clients? Who are your partners?
Situation your work, projects in AI ethics
- How do you situate your work within the broader field of AI ethics? What types of challenges are you working on in this field? Please feel free to share any specific examples from your projects.
- What are the main projects related to AI ethics that you are working on that you can tell us about?
- What types of resources (academic papers, academic experts, standards, guidelines) do you use in your AI ethics practice?
- Do you use the guidelines on AI ethics practice that have published in this field over the past 5 years? If so, which one and what does following the guideline look like at your company?
- What are the most important challenges to implementing ethics principles at your work?
Skills, knowledge, values
- What are the most important skillsets, knowledge base and values that you currently use at your job? What are you currently developing and will need in the future?
- If you decide to hire someone to replace you in your current role, what would you look for? What skills or background would your ideal candidate have?
Looking into the future
- From your perspective, what roles do you think are necessary in the field of AI ethics in academia, industry, governmental and non-governmental organizations? Please elaborate.
Relevant tables
Inclusion and exclusion criteria for job postings
Table Label: tab-jp-criteria
Download PDF to view tableBibliography
1@article{Abril07,
2 note = {},
3 url = {http://doi.acm.org/10.1145/1219092.1219093},
4 doi = {10.1145/1188913.1188915},
5 pages = {36--44},
6 year = {2007},
7 month = {January},
8 number = {1},
9 volume = {50},
10 journal = {Communications of the ACM},
11 title = {The patent holder's dilemma: Buy, sell, or troll?},
12 author = {Patricia S. Abril and Robert Plant},
13}
14
15@article{Cohen07,
16 acmid = {1219093},
17 url = {http://doi.acm.org/10.1145/1219092.1219093},
18 doi = {10.1145/1219092.1219093},
19 year = {2007},
20 month = {April},
21 number = {2},
22 volume = {54},
23 numpages = {50},
24 articleno = {5},
25 journal = {J. ACM},
26 title = {Deciding equivalances among conjunctive aggregate queries},
27 author = {Sarah Cohen and Werner Nutt and Yehoshua Sagic},
28}
29
30@book{Kosiur01,
31 note = {},
32 month = {},
33 series = {},
34 number = {},
35 volume = {},
36 editor = {},
37 edition = {2nd.},
38 address = {New York, NY},
39 year = {2001},
40 publisher = {Wiley},
41 title = {Understanding Policy-Based Networking},
42 author = {David Kosiur},
43}
44
45@book{Harel79,
46 note = {},
47 month = {},
48 number = {},
49 editor = {},
50 url = {http://dx.doi.org/10.1007/3-540-09237-4},
51 doi = {10.1007/3-540-09237-4},
52 publisher = {Springer-Verlag},
53 address = {New York, NY},
54 volume = {68},
55 series = {Lecture Notes in Computer Science},
56 title = {First-Order Dynamic Logic},
57 year = {1979},
58 author = {David Harel},
59}
60
61@inbook{Editor00,
62 note = {},
63 month = {},
64 type = {},
65 number = {},
66 pages = {},
67 chapter = {},
68 url = {http://dx.doi.org/10.1007/3-540-09456-9},
69 doi = {10.1007/3-540-09237-4},
70 publisher = {University of Chicago Press},
71 edition = {1st.},
72 address = {Chicago},
73 volume = {9},
74 year = {2007},
75 series = {The name of the series one},
76 subtitle = {The book subtitle},
77 title = {The title of book one},
78 editor = {Ian Editor},
79 author = {},
80}
81
82@inbook{Editor00a,
83 note = {},
84 month = {},
85 type = {},
86 number = {},
87 pages = {},
88 chapter = {100},
89 volume = {},
90 url = {http://dx.doi.org/10.1007/3-540-09456-9},
91 doi = {10.1007/3-540-09237-4},
92 publisher = {University of Chicago Press},
93 edition = {2nd.},
94 address = {Chicago},
95 year = {2008},
96 series = {The name of the series two},
97 subtitle = {The book subtitle},
98 title = {The title of book two},
99 editor = {Ian Editor},
100 author = {},
101}
102
103@incollection{Spector90,
104 note = {},
105 month = {},
106 type = {},
107 series = {},
108 number = {},
109 volume = {},
110 url = {http://doi.acm.org/10.1145/90417.90738},
111 doi = {10.1145/90417.90738},
112 pages = {19--33},
113 editor = {Sape Mullender},
114 chapter = {},
115 edition = {2nd.},
116 year = {1990},
117 address = {New York, NY},
118 publisher = {ACM Press},
119 booktitle = {Distributed Systems},
120 title = {Achieving application requirements},
121 author = {Asad Z. Spector},
122}
123
124@incollection{Douglass98,
125 note = {},
126 month = {},
127 type = {},
128 number = {},
129 edition = {},
130 url = {http://dx.doi.org/10.1007/3-540-65193-4_29},
131 doi = {10.1007/3-540-65193-4_29},
132 pages = {368--394},
133 editor = {Grzegorz Rozenberg and Frits W. Vaandrager},
134 chapter = {},
135 year = {1998},
136 volume = {1494},
137 address = {London},
138 publisher = {Springer-Verlag},
139 booktitle = {Lectures on Embedded Systems},
140 series = {Lecture Notes in Computer Science},
141 title = {Statecarts in use: structured analysis and object-orientation},
142 author = {Bruce P. Douglass and David Harel and Mark B. Trakhtenbrot},
143}
144
145@book{Knuth97,
146 note = {},
147 month = {},
148 series = {},
149 number = {},
150 volume = {},
151 editor = {},
152 edition = {},
153 address = {},
154 year = {1997},
155 publisher = {Addison Wesley Longman Publishing Co., Inc.},
156 title = {The Art of Computer Programming, Vol. 1: Fundamental Algorithms (3rd. ed.)},
157 author = {Donald E. Knuth},
158}
159
160@book{Knuth98,
161 note = {(book)},
162 month = {},
163 number = {},
164 editor = {},
165 url = {},
166 doi = {},
167 publisher = {Addison Wesley Longman Publishing Co., Inc.},
168 address = {},
169 edition = {3rd},
170 volume = {1},
171 series = {Fundamental Algorithms},
172 title = {The Art of Computer Programming},
173 year = {1998},
174 author = {Donald E. Knuth},
175}
176
177@incollection{GM05,
178 editors = {Z. Ghahramani and R. Cowell},
179 month = {January},
180 publisher = {The Society for Artificial Intelligence and Statistics},
181 booktitle = {Proceedings of Tenth International Workshop on Artificial Intelligence and Statistics, {\rm The Barbados}},
182 year = {2005},
183 title = {Structured Variational Inference Procedures and their Realizations (as incol)},
184 author = {Dan Geiger and Christopher Meek},
185}
186
187@inproceedings{Smith10,
188 note = {},
189 organization = {},
190 month = {},
191 number = {},
192 url = {http://dx.doi.org/99.0000/woot07-S422},
193 doi = {99.9999/woot07-S422},
194 pages = {422--431},
195 address = {Milan Italy},
196 publisher = {Paparazzi Press},
197 year = {2010},
198 volume = {3},
199 editor = {Reginald N. Smythe and Alexander Noble},
200 series = {LAC '10},
201 booktitle = {Proceedings of the 3rd. annual workshop on Librarians and Computers},
202 title = {An experiment in bibliographic mark-up: Parsing metadata for XML export},
203 author = {Stan W. Smith},
204}
205
206@inproceedings{VanGundy07,
207 numpages = {9},
208 articleno = {Paper 7},
209 address = {Berkley, CA},
210 publisher = {USENIX Association},
211 series = {WOOT '07},
212 booktitle = {Proceedings of the first USENIX workshop on Offensive Technologies},
213 title = {Catch me, if you can: Evading network signatures with web-based polymorphic worms},
214 year = {2007},
215 author = {Matthew Van Gundy and Davide Balzarotti and Giovanni Vigna},
216}
217
218@inproceedings{VanGundy08,
219 pages = {99-100},
220 numpages = {2},
221 articleno = {7},
222 address = {Berkley, CA},
223 publisher = {USENIX Association},
224 series = {WOOT '08},
225 booktitle = {Proceedings of the first USENIX workshop on Offensive Technologies},
226 title = {Catch me, if you can: Evading network signatures with web-based polymorphic worms},
227 year = {2008},
228 author = {Matthew Van Gundy and Davide Balzarotti and Giovanni Vigna},
229}
230
231@inproceedings{VanGundy09,
232 pages = {90--100},
233 address = {Berkley, CA},
234 publisher = {USENIX Association},
235 series = {WOOT '09},
236 booktitle = {Proceedings of the first USENIX workshop on Offensive Technologies},
237 title = {Catch me, if you can: Evading network signatures with web-based polymorphic worms},
238 year = {2009},
239 author = {Matthew Van Gundy and Davide Balzarotti and Giovanni Vigna},
240}
241
242@inproceedings{Andler79,
243 note = {},
244 organization = {},
245 month = {},
246 number = {},
247 volume = {},
248 editor = {},
249 url = {http://doi.acm.org/10.1145/567752.567774},
250 doi = {10.1145/567752.567774},
251 pages = {226--236},
252 address = {New York, NY},
253 publisher = {ACM Press},
254 year = {1979},
255 series = {POPL '79},
256 booktitle = {Proceedings of the 6th. ACM SIGACT-SIGPLAN symposium on Principles of Programming Languages},
257 title = {Predicate Path expressions},
258 author = {Sten Andler},
259}
260
261@techreport{Harel78,
262 note = {},
263 month = {},
264 address = {Cambridge, MA},
265 number = {TR-200},
266 type = {MIT Research Lab Technical Report},
267 institution = {Massachusetts Institute of Technology},
268 title = {LOGICS of Programs: AXIOMATICS and DESCRIPTIVE POWER},
269 year = {1978},
270 author = {David Harel},
271}
272
273@mastersthesis{anisi03,
274 year = {2003},
275 intitution = {FOI-R-0961-SE, Swedish Defence Research Agency (FOI)},
276 school = {Royal Institute of Technology (KTH), Stockholm, Sweden},
277 title = {Optimal Motion Control of a Ground Vehicle},
278 author = {David A. Anisi},
279}
280
281@phdthesis{Clarkson85,
282 month = {},
283 type = {},
284 note = {UMI Order Number: AAT 8506171},
285 address = {Palo Alto, CA},
286 school = {Stanford University},
287 title = {Algorithms for Closest-Point Problems (Computational Geometry)},
288 year = {1985},
289 author = {Kenneth L. Clarkson},
290}
291
292@misc{Poker06,
293 url = {http://www.poker-edge.com/stats.php},
294 lastaccessed = {June 7, 2006},
295 title = {Stats and Analysis},
296 month = {March},
297 year = {2006},
298 author = {Poker-Edge.Com},
299}
300
301@misc{Obama08,
302 note = {},
303 lastaccessed = {March 21, 2008},
304 month = {March},
305 url = {http://video.google.com/videoplay?docid=6528042696351994555},
306 day = {5},
307 howpublished = {Video},
308 title = {A more perfect union},
309 year = {2008},
310 author = {Barack Obama},
311}
312
313@misc{JoeScientist001,
314 lastaccessed = {},
315 month = {August},
316 howpublished = {},
317 url = {},
318 note = {Patent No. 12345, Filed July 1st., 2008, Issued Aug. 9th., 2009},
319 title = {The fountain of youth},
320 year = {2009},
321 author = {Joseph Scientist},
322}
323
324@inproceedings{Novak03,
325 distincturl = {1},
326 organization = {},
327 series = {},
328 number = {},
329 volume = {},
330 editor = {},
331 howpublished = {Video},
332 note = {},
333 url = {http://video.google.com/videoplay?docid=6528042696351994555},
334 doi = {99.9999/woot07-S422},
335 month = {March 21, 2008},
336 pages = {4},
337 address = {New York, NY},
338 publisher = {ACM Press},
339 year = {2003},
340 booktitle = {ACM SIGGRAPH 2003 Video Review on Animation theater Program: Part I - Vol. 145 (July 27--27, 2003)},
341 title = {Solder man},
342 author = {Dave Novak},
343}
344
345@article{Lee05,
346 note = {},
347 howpublished = {Video},
348 url = {http://doi.acm.org/10.1145/1057270.1057278},
349 doi = {10.1145/1057270.1057278},
350 month = {Jan.-March},
351 number = {1},
352 volume = {3},
353 eid = {4},
354 journal = {Comput. Entertain.},
355 title = {Interview with Bill Kinder: January 13, 2005},
356 year = {2005},
357 author = {Newton Lee},
358}
359
360@article{rous08,
361 note = {To appear},
362 howpublished = {},
363 url = {},
364 doi = {},
365 articleno = {Article~5},
366 month = {July},
367 number = {3},
368 volume = {12},
369 journal = {Digital Libraries},
370 title = {The Enabling of Digital Libraries},
371 year = {2008},
372 author = {Bernard Rous},
373}
374
375@article{384253,
376 address = {New York, NY, USA},
377 publisher = {ACM},
378 doi = {http://doi.acm.org/10.1145/351827.384253},
379 pages = {11},
380 issn = {1084-6654},
381 year = {2000},
382 volume = {5},
383 journal = {J. Exp. Algorithmics},
384 title = {(old) Finding minimum congestion spanning trees},
385 author = {Werneck,, Renato and Setubal,, Jo\~{a}o and da Conceic\~{a}o,, Arlindo},
386}
387
388@article{Werneck:2000:FMC:351827.384253,
389 address = {New York, NY, USA},
390 publisher = {ACM},
391 acmid = {384253},
392 doi = {10.1145/351827.384253},
393 url = {http://portal.acm.org/citation.cfm?id=351827.384253},
394 articleno = {11},
395 issn = {1084-6654},
396 year = {2000},
397 month = {December},
398 volume = {5},
399 journal = {J. Exp. Algorithmics},
400 title = {(new) Finding minimum congestion spanning trees},
401 author = {Werneck, Renato and Setubal, Jo\~{a}o and da Conceic\~{a}o, Arlindo},
402}
403
404@article{1555162,
405 address = {Amsterdam, The Netherlands, The Netherlands},
406 publisher = {Elsevier Science Publishers B. V.},
407 doi = {http://dx.doi.org/10.1016/j.inffus.2009.01.002},
408 pages = {342--353},
409 issn = {1566-2535},
410 year = {2009},
411 number = {4},
412 volume = {10},
413 journal = {Inf. Fusion},
414 title = {(old) Distributed data source verification in wireless sensor networks},
415 author = {Conti, Mauro and Di Pietro, Roberto and Mancini, Luigi V. and Mei, Alessandro},
416}
417
418@article{Conti:2009:DDS:1555009.1555162,
419 keywords = {Clone detection, Distributed protocol, Securing data fusion, Wireless sensor networks},
420 address = {Amsterdam, The Netherlands, The Netherlands},
421 publisher = {Elsevier Science Publishers B. V.},
422 acmid = {1555162},
423 doi = {10.1016/j.inffus.2009.01.002},
424 url = {http://portal.acm.org/citation.cfm?id=1555009.1555162},
425 numpages = {12},
426 pages = {342--353},
427 issn = {1566-2535},
428 year = {2009},
429 month = {October},
430 number = {4},
431 volume = {10},
432 journal = {Inf. Fusion},
433 title = {(new) Distributed data source verification in wireless sensor networks},
434 author = {Conti, Mauro and Di Pietro, Roberto and Mancini, Luigi V. and Mei, Alessandro},
435}
436
437@inproceedings{Li:2008:PUC:1358628.1358946,
438 keywords = {cscw, distributed knowledge acquisition, incentive design, online games, recommender systems, reputation systems, user studies, virtual community},
439 address = {New York, NY, USA},
440 publisher = {ACM},
441 acmid = {1358946},
442 doi = {10.1145/1358628.1358946},
443 url = {http://portal.acm.org/citation.cfm?id=1358628.1358946},
444 numpages = {6},
445 pages = {3873--3878},
446 location = {Florence, Italy},
447 isbn = {978-1-60558-012-X},
448 year = {2008},
449 booktitle = {CHI '08 extended abstracts on Human factors in computing systems},
450 title = {Portalis: using competitive online interactions to support aid initiatives for the homeless},
451 author = {Li, Cheng-Lun and Buyuktur, Ayse G. and Hutchful, David K. and Sant, Natasha B. and Nainwal, Satyendra K.},
452}
453
454@book{Hollis:1999:VBD:519964,
455 address = {Upper Saddle River, NJ, USA},
456 publisher = {Prentice Hall PTR},
457 edition = {1st},
458 isbn = {0130850845},
459 year = {1999},
460 title = {Visual Basic 6: Design, Specification, and Objects with Other},
461 author = {Hollis, Billy S.},
462}
463
464@book{Goossens:1999:LWC:553897,
465 address = {Boston, MA, USA},
466 publisher = {Addison-Wesley Longman Publishing Co., Inc.},
467 edition = {1st},
468 isbn = {0201433117},
469 year = {1999},
470 title = {The Latex Web Companion: Integrating TEX, HTML, and XML},
471 author = {Goossens, Michel and Rahtz, S. P. and Moore, Ross and Sutor, Robert S.},
472}
473
474@techreport{897367,
475 address = {Amherst, MA, USA},
476 publisher = {University of Massachusetts},
477 source = {http://www.ncstrl.org:8900/ncstrl/servlet/search?formname=detail\&id=oai%3Ancstrlh%3Aumass_cs%3Ancstrl.umassa_cs%2F%2FUM-CS-1987-018},
478 year = {1987},
479 title = {Vertex Types in Book-Embeddings},
480 author = {Buss, Jonathan F. and Rosenberg, Arnold L. and Knott, Judson D.},
481}
482
483@techreport{Buss:1987:VTB:897367,
484 address = {Amherst, MA, USA},
485 publisher = {University of Massachusetts},
486 source = {http://www.ncstrl.org:8900/ncstrl/servlet/search?formname=detail\&id=oai%3Ancstrlh%3Aumass_cs%3Ancstrl.umassa_cs%2F%2FUM-CS-1987-018},
487 year = {1987},
488 title = {Vertex Types in Book-Embeddings},
489 author = {Buss, Jonathan F. and Rosenberg, Arnold L. and Knott, Judson D.},
490}
491
492@proceedings{Czerwinski:2008:1358628,
493 address = {New York, NY, USA},
494 publisher = {ACM},
495 order_no = {608085},
496 location = {Florence, Italy},
497 isbn = {978-1-60558-012-X},
498 year = {2008},
499 title = {CHI '08: CHI '08 extended abstracts on Human factors in computing systems},
500 note = {General Chair-Czerwinski, Mary and General Chair-Lund, Arnie and Program Chair-Tan, Desney},
501 author = {},
502}
503
504@phdthesis{Clarkson:1985:ACP:911891,
505 address = {Stanford, CA, USA},
506 school = {Stanford University},
507 note = {AAT 8506171},
508 year = {1985},
509 title = {Algorithms for Closest-Point Problems (Computational Geometry)},
510 advisor = {Yao, Andrew C.},
511 author = {Clarkson, Kenneth Lee},
512}
513
514@article{1984:1040142,
515 address = {New York, NY, USA},
516 publisher = {ACM},
517 issue_date = {January/April 1984},
518 number = {5-1},
519 volume = {13-14},
520 issn = {0146-4833},
521 year = {1984},
522 journal = {SIGCOMM Comput. Commun. Rev.},
523 key = {{$\!\!$}},
524}
525
526@inproceedings{2004:ITE:1009386.1010128,
527 address = {Washington, DC, USA},
528 publisher = {IEEE Computer Society},
529 acmid = {1010128},
530 doi = {http://dx.doi.org/10.1109/ICWS.2004.64},
531 url = {http://dx.doi.org/10.1109/ICWS.2004.64},
532 pages = {21--22},
533 isbn = {0-7695-2167-3},
534 year = {2004},
535 series = {ICWS '04},
536 booktitle = {Proceedings of the IEEE International Conference on Web Services},
537 title = {IEEE TCSC Executive Committee},
538 key = {IEEE},
539}
540
541@book{Mullender:1993:DS:302430,
542 address = {New York, NY, USA},
543 publisher = {ACM Press/Addison-Wesley Publishing Co.},
544 isbn = {0-201-62427-3},
545 year = {1993},
546 title = {Distributed systems (2nd Ed.)},
547 editor = {Mullender, Sape},
548}
549
550@techreport{Petrie:1986:NAD:899644,
551 address = {Austin, TX, USA},
552 publisher = {University of Texas at Austin},
553 source = {http://www.ncstrl.org:8900/ncstrl/servlet/search?formname=detail\&id=oai%3Ancstrlh%3Autexas_cs%3AUTEXAS_CS%2F%2FAI86-33},
554 year = {1986},
555 title = {New Algorithms for Dependency-Directed Backtracking (Master's thesis)},
556 author = {Petrie, Charles J.},
557}
558
559@mastersthesis{Petrie:1986:NAD:12345,
560 address = {Austin, TX, USA},
561 school = {University of Texas at Austin},
562 source = {http://www.ncstrl.org:8900/ncstrl/servlet/search?formname=detail\&id=oai%3Ancstrlh%3Autexas_cs%3AUTEXAS_CS%2F%2FAI86-33},
563 year = {1986},
564 title = {New Algorithms for Dependency-Directed Backtracking (Master's thesis)},
565 author = {Petrie, Charles J.},
566}
567
568@book{book-minimal,
569 year = {1981},
570 publisher = {Addison-Wesley},
571 title = {Seminumerical Algorithms},
572 author = {Donald E. Knuth},
573}
574
575@incollection{KA:2001,
576 address = {Hershey, PA, USA},
577 publisher = {IGI Publishing},
578 acmid = {887010},
579 url = {http://portal.acm.org/citation.cfm?id=887006.887010},
580 numpages = {24},
581 pages = {51--74},
582 isbn = {1-59140-056-2},
583 year = {2001},
584 booktitle = {E-commerce and cultural values},
585 title = {The implementation of electronic commerce in SMEs in Singapore (as Incoll)},
586 author = {Kong, Wei-Chang},
587}
588
589@inbook{KAGM:2001,
590 address = {Hershey, PA, USA},
591 publisher = {IGI Publishing},
592 acmid = {887010},
593 url = {http://portal.acm.org/citation.cfm?id=887006.887010},
594 numpages = {24},
595 pages = {51--74},
596 isbn = {1-59140-056-2},
597 year = {2001},
598 title = {E-commerce and cultural values},
599 chapter = {The implementation of electronic commerce in SMEs in Singapore (Inbook-w-chap-w-type)},
600 type = {Name of Chapter:},
601 author = {Kong, Wei-Chang},
602}
603
604@article{Brundage2020e,
605 year = {2020},
606 url = {http://arxiv.org/abs/2004.07213},
607 title = {{Toward Trustworthy AI Development: Mechanisms for Supporting Verifiable Claims}},
608 mendeley-groups = {PhD/AI competency project},
609 file = {:C$\backslash$:/Users/shala/AppData/Local/Mendeley Ltd./Mendeley Desktop/Downloaded/Brundage et al. - 2020 - Toward Trustworthy AI Development Mechanisms for Supporting Verifiable Claims.pdf:pdf},
610 eprint = {2004.07213},
611 author = {Brundage, Miles and Avin, Shahar and Wang, Jasmine and Belfield, Haydn and Krueger, Gretchen and Hadfield, Gillian and Khlaaf, Heidy and Yang, Jingying and Toner, Helen and Fong, Ruth and Maharaj, Tegan and Koh, Pang Wei and Hooker, Sara and Leung, Jade and Trask, Andrew and Bluemke, Emma and Lebensold, Jonathan and O'Keefe, Cullen and Koren, Mark and Ryffel, Th{\'{e}}o and Rubinovitz, JB and Besiroglu, Tamay and Carugati, Federica and Clark, Jack and Eckersley, Peter and de Haas, Sarah and Johnson, Maritza and Laurie, Ben and Ingerman, Alex and Krawczuk, Igor and Askell, Amanda and Cammarota, Rosario and Lohn, Andrew and Krueger, David and Stix, Charlotte and Henderson, Peter and Graham, Logan and Prunkl, Carina and Martin, Bianca and Seger, Elizabeth and Zilberman, Noa and H{\'{E}}igeartaigh, Se{\'{a}}n {\'{O}} and Kroeger, Frens and Sastry, Girish and Kagan, Rebecca and Weller, Adrian and Tse, Brian and Barnes, Elizabeth and Dafoe, Allan and Scharre, Paul and Herbert-Voss, Ariel and Rasser, Martijn and Sodhani, Shagun and Flynn, Carrick and Gilbert, Thomas Krendl and Dyer, Lisa and Khan, Saif and Bengio, Yoshua and Anderljung, Markus},
612 arxivid = {2004.07213},
613 archiveprefix = {arXiv},
614 abstract = {With the recent wave of progress in artificial intelligence (AI) has come a growing awareness of the large-scale impacts of AI systems, and recognition that existing regulations and norms in industry and academia are insufficient to ensure responsible AI development. In order for AI developers to earn trust from system users, customers, civil society, governments, and other stakeholders that they are building AI responsibly, they will need to make verifiable claims to which they can be held accountable. Those outside of a given organization also need effective means of scrutinizing such claims. This report suggests various steps that different stakeholders can take to improve the verifiability of claims made about AI systems and their associated development processes, with a focus on providing evidence about the safety, security, fairness, and privacy protection of AI systems. We analyze ten mechanisms for this purpose--spanning institutions, software, and hardware--and make recommendations aimed at implementing, exploring, or improving those mechanisms.},
615}
616
617@inproceedings{Holstein2019,
618 year = {2019},
619 title = {{Improving fairness in machine learning systems: What do industry practitioners need?}},
620 mendeley-groups = {PhD/AI competency project},
621 keywords = {Algorithmic bias,Empirical study,Fair machine learning,Need-finding,Product teams,UX of machine learning},
622 isbn = {9781450359702},
623 file = {:G$\backslash$:/My Drive/PhD/AI Competency Project/Literature Review/improving fairness in ML systems - what do industry practitioners need.pdf:pdf},
624 eprint = {1812.05239},
625 doi = {10.1145/3290605.3300830},
626 booktitle = {Conf. Hum. Factors Comput. Syst. - Proc.},
627 author = {Holstein, Kenneth and Vaughan, Jennifer Wortman and Daum{\'{e}}, Hal and Dud{\'{i}}k, Miroslav and Wallach, Hanna},
628 arxivid = {1812.05239},
629 archiveprefix = {arXiv},
630 abstract = {The potential for machine learning (ML) systems to amplify social inequities and unfairness is receiving increasing popular and academic attention. A surge of recent work has focused on the development of algorithmic tools to assess and mitigate such unfairness. If these tools are to have a positive impact on industry practice, however, it is crucial that their design be informed by an understanding of real-world needs. Through 35 semi-structured interviews and an anonymous survey of 267 ML practitioners, we conduct the first systematic investigation of commercial product teams' challenges and needs for support in developing fairer ML systems. We identify areas of alignment and disconnect between the challenges faced by teams in practice and the solutions proposed in the fair ML research literature. Based on these findings, we highlight directions for future ML and HCI research that will better address practitioners' needs.},
631}
632
633@inproceedings{Belfield2020,
634 year = {2020},
635 title = {{Activism by the AI Community}},
636 pages = {15--21},
637 mendeley-groups = {PhD/AI competency project},
638 isbn = {9781450371100},
639 file = {:G$\backslash$:/My Drive/PhD/AI Competency Project/Literature Review/activitist by AI ethics community.pdf:pdf},
640 doi = {10.1145/3375627.3375814},
641 booktitle = {AIES 2019 - Proc. 2019 AAAI/ACM Conf. AI, Ethics, Soc.},
642 author = {Belfield, Haydn},
643 abstract = {The artificial intelligence (AI) community has recently engaged in activism in relation to their employers, other members of the community, and their governments in order to shape the societal and ethical implications of AI. It has achieved some notable successes, but prospects for further political organising and activism are uncertain. We survey activism by the AI community over the last six years; apply two analytical frameworks drawing upon the literature on epistemic communities, and worker organising and bargaining; and explore what they imply for the future prospects of the AI community. Success thus far has hinged on a coherent shared culture, and high bargaining power due to the high demand for a limited supply of AI 'talent'. Both are crucial to the future of AI activism and worthy of sustained attention.},
644}
645
646@techreport{Bruneault2022,
647 year = {2022},
648 title = {{AI Ethics Training in Higher Education : Competency Framework}},
649 number = {February},
650 mendeley-groups = {PhD/AI competency project},
651 file = {:G$\backslash$:/My Drive/PhD/AI Competency Project/Literature Review/22949-Cegep-Andre-Laurendeau-Referentiel-de-competence-V4-FINAL-Interactif (1).pdf:pdf},
652 author = {Bruneault, Fr{\'{e}}d{\'{e}}rick and Andr{\'{e}}-laurendeau, C{\'{e}}gep and Laflamme, Andr{\'{e}}ane Sabourin and Fillion, Gabrielle and Abtroun, Neila and Freeman, Andrew},
653}
654
655@techreport{YuriDemchenkoAdamBelloum2017,
656 year = {2017},
657 url = {https://edison-project.eu/sites/edison-project.eu/files/filefield{\_}paths/edison{\_}cf-ds-release2-v08{\_}0.pdf},
658 title = {{EDISON Data Science Framework: Part1. Data Science Competence Framework Release 2}},
659 pages = {1--59},
660 number = {July},
661 mendeley-groups = {PhD/AI competency project},
662 file = {:G$\backslash$:/My Drive/PhD/AI Competency Project/Literature Review/edison{\_}cf-ds-release2-v08{\_}0.pdf:pdf},
663 author = {{Yuri Demchenko, Adam Belloum}, Tomasz Wiktorski},
664}
665
666@techreport{Blok2021,
667 year = {2021},
668 title = {{Artificial Intelligence Competency Framework Table of Contents}},
669 number = {September},
670 mendeley-groups = {PhD/AI competency project},
671 file = {:G$\backslash$:/My Drive/PhD/AI Competency Project/Literature Review/Corrected-FINAL{\_}PIA{\_}ConcordiaDawson{\_}AICompetencyFramework.pdf:pdf},
672 author = {Blok, Sherry and Trudeau, Joel and Cassidy, Robert},
673}
674
675@article{Rakova2021c,
676 year = {2021},
677 volume = {5},
678 title = {{Where Responsible AI meets Reality: Practitioner Perspectives on Enablers for Shifting Organizational Practices}},
679 pages = {1--23},
680 number = {CSCW1},
681 mendeley-groups = {PhD/AI competency project},
682 keywords = {industry practice,organizational structure,responsible ai},
683 journal = {Proc. ACM Human-Computer Interact.},
684 issn = {25730142},
685 file = {:G$\backslash$:/My Drive/PhD/AI Competency Project/Literature Review/Where Responsible AI meets Reality - Practitioner's Perspective.pdf:pdf},
686 eprint = {2006.12358},
687 doi = {10.1145/3449081},
688 author = {Rakova, Bogdana and Yang, Jingying and Cramer, Henriette and Chowdhury, Rumman},
689 arxivid = {2006.12358},
690 archiveprefix = {arXiv},
691 abstract = {Large and ever-evolving technology companies continue to invest more time and resources to incorporate responsible Artificial Intelligence (AI) into production-ready systems to increase algorithmic accountability. This paper examines and seeks to offer a framework for analyzing how organizational culture and structure impact the effectiveness of responsible AI initiatives in practice. We present the results of semi-structured qualitative interviews with practitioners working in industry, investigating common challenges, ethical tensions, and effective enablers for responsible AI initiatives. Focusing on major companies developing or utilizing AI, we have mapped what organizational structures currently support or hinder responsible AI initiatives, what aspirational future processes and structures would best enable effective initiatives, and what key elements comprise the transition from current work practices to the aspirational future.},
692}
693
694@article{Gambelin2021,
695 year = {2020},
696 volume = {1},
697 url = {https://doi.org/10.1007/s43681-020-00020-5},
698 title = {{Brave: what it means to be an AI Ethicist}},
699 publisher = {Springer International Publishing},
700 pages = {87--91},
701 number = {1},
702 mendeley-groups = {PhD/AI competency project},
703 keywords = {AI Ethics,Artificial intelligence,Bravery,Ethical decision making,ai ethics,artificial intelligence,bravery,ethical decision making},
704 journal = {AI Ethics},
705 issn = {2730-5953},
706 isbn = {0123456789},
707 file = {:G$\backslash$:/My Drive/PhD/AI Competency Project/Literature Review/Gambelin2020{\_}Article{\_}BraveWhatItMeansToBeAnAIEthici.pdf:pdf},
708 doi = {10.1007/s43681-020-00020-5},
709 author = {Gambelin, Olivia},
710 abstract = {Despite there being a strong call for responsible technology, the path towards putting ethics into action is still yet to be fully understood. To help guide the implementation of ethics, we have seen the rise of a new professional title; the AI Ethicist. However, it is still unclear what the role and skill set of this new profession must include. The purpose of this piece is to offer a preliminary definition of what it means to be an AI Ethicist by first examining the concept of an ethicist in the context of artificial intelligence, followed by exploring what responsibilities are added to the role in industry specifically, and ending on the fundamental characteristic that underlies it all: bravery.},
711}
712
713@inproceedings{Raji2021,
714 year = {2021},
715 title = {{"you can't sit with us": Exclusionary pedagogy in AI ethics education}},
716 pages = {515--525},
717 mendeley-groups = {PhD/AI competency project},
718 isbn = {9781450383097},
719 file = {:G$\backslash$:/My Drive/PhD/AI Competency Project/Literature Review/Raji-pedagogy2021.pdf:pdf},
720 doi = {10.1145/3442188.3445914},
721 booktitle = {FAccT 2021 - Proc. 2021 ACM Conf. Fairness, Accountability, Transpar.},
722 author = {Raji, Inioluwa Deborah and Scheuerman, Morgan Klaus and Amironesei, Razvan},
723 abstract = {Given a growing concern about the lack of ethical consideration in the Artificial Intelligence (AI) field, many have begun to question how dominant approaches to the disciplinary education of computer science (CS) - -and its implications for AI - -has led to the current "ethics crisis". However, we claim that the current AI ethics education space relies on a form of "exclusionary pedagogy,"where ethics is distilled for computational approaches, but there is no deeper epistemological engagement with other ways of knowing that would benefit ethical thinking or an acknowledgement of the limitations of uni-vocal computational thinking. This results in indifference, devaluation, and a lack of mutual support between CS and humanistic social science (HSS), elevating the myth of technologists as "ethical unicorns"that can do it all, though their disciplinary tools are ultimately limited. Through an analysis of computer science education literature and a review of college-level course syllabi in AI ethics, we discuss the limitations of the epistemological assumptions and hierarchies of knowledge which dictate current attempts at including ethics education in CS training and explore evidence for the practical mechanisms through which this exclusion occurs. We then propose a shift towards a substantively collaborative, holistic, and ethically generative pedagogy in AI education.},
724}
725
726@misc{orcaa,
727 language = {en},
728 note = {Accessed: 2023-3-15},
729 howpublished = {\url{https://orcaarisk.com/}},
730 year = {2023},
731 author = {ORCAA Consulting},
732 title = {ORCAA},
733}
734
735@misc{Lab2019-ur,
736 language = {en},
737 note = {Accessed: 2023-3-15},
738 howpublished = {\url{https://aiethicslab.com/}},
739 year = {2019},
740 month = {December},
741 author = {AI Ethics Lab},
742 title = {AI Ethics Lab},
743}
744
745@misc{ethical-advisory,
746 language = {en},
747 note = {Accessed: 2023-3-15},
748 howpublished = {\url{https://www.ethicalai.ai/}},
749 year = {2023},
750 author = {Ethical AI Advisory},
751 title = {Ethical AI Advisory},
752}
753
754@article{Fjeld2020-rb,
755 year = {2020},
756 month = {January},
757 abstract = {The rapid spread of artificial intelligence (AI) systems has
758precipitated a rise in ethical and human rights-based frameworks
759intended to guide the development and use of these technologies.
760Despite the proliferation of these ``AI principles,'' there has
761been little scholarly focus on understanding these efforts either
762individually or as contextualized within an expanding universe of
763principles with discernible trends.To that end, this white paper
764and its associated data visualization compare the contents of
765thirty-six prominent AI principles documents side-by-side. This
766effort uncovered a growing consensus around eight key thematic
767trends: privacy, accountability, safety and security,
768transparency and explainability, fairness and non-discrimination,
769human control of technology, professional responsibility, and
770promotion of human values. Underlying this ``normative core,''
771our analysis examined the forty-seven individual principles that
772make up the themes, detailing notable similarities and
773differences in interpretation found across the documents. In
774sharing these observations, it is our hope that policymakers,
775advocates, scholars, and others working to maximize the benefits
776and minimize the harms of AI will be better positioned to build
777on existing efforts and to push the fractured, global
778conversation on the future of AI toward consensus.},
779 author = {Fjeld, Jessica and Achten, Nele and Hilligoss, Hannah and Nagy,
780Adam and Srikumar, Madhulika},
781 title = {Principled Artificial Intelligence: Mapping Consensus in Ethical
782and Rights-Based Approaches to Principles for AI},
783}
784
785@article{Jobin2019-kt,
786 language = {en},
787 year = {2019},
788 month = {September},
789 pages = {389--399},
790 number = {9},
791 volume = {1},
792 publisher = {Nature Publishing Group},
793 journal = {Nature Machine Intelligence},
794 abstract = {In the past five years, private companies, research institutions
795and public sector organizations have issued principles and
796guidelines for ethical artificial intelligence (AI). However,
797despite an apparent agreement that AI should be `ethical', there
798is debate about both what constitutes `ethical AI' and which
799ethical requirements, technical standards and best practices are
800needed for its realization. To investigate whether a global
801agreement on these questions is emerging, we mapped and analysed
802the current corpus of principles and guidelines on ethical AI.
803Our results reveal a global convergence emerging around five
804ethical principles (transparency, justice and fairness,
805non-maleficence, responsibility and privacy), with substantive
806divergence in relation to how these principles are interpreted,
807why they are deemed important, what issue, domain or actors they
808pertain to, and how they should be implemented. Our findings
809highlight the importance of integrating guideline-development
810efforts with substantive ethical analysis and adequate
811implementation strategies. As AI technology develops rapidly, it
812is widely recognized that ethical guidelines are required for
813safe and fair implementation in society. But is it possible to
814agree on what is `ethical AI'? A detailed analysis of 84 AI
815ethics reports around the world, from national and international
816organizations, companies and institutes, explores this question,
817finding a convergence around core principles but substantial
818divergence on practical implementation.},
819 author = {Jobin, Anna and Ienca, Marcello and Vayena, Effy},
820 title = {The global landscape of AI ethics guidelines},
821}
822
823@inproceedings{Rismani2023-im,
824 location = { Hamburg, Germany},
825 year = {2023},
826 month = {April},
827 volume = {1},
828 publisher = {Association for Computing Machinery},
829 author = {Rismani, Shalaleh and Shelby, Renee and Smart, Andrew and
830Jatho, Edgar and Kroll, Josh A and Moon, Ajung and
831Rostamzadeh, Negar},
832 booktitle = {Proceedings of the 2023 {CHI} Conference on Human Factors
833in Computing Systems ({CHI} '23)},
834 title = {From Plane Crashes to Algorithmic Harm: Applicability of
835Safety Engineering Frameworks for Responsible {ML}},
836}
837
838@article{Shelby2022-oi,
839 eprint = {2210.05791},
840 primaryclass = {cs.HC},
841 archiveprefix = {arXiv},
842 year = {2022},
843 month = {October},
844 abstract = {Understanding the landscape of potential harms from
845algorithmic systems enables practitioners to better
846anticipate consequences of the systems they build. It also
847supports the prospect of incorporating controls to help
848minimize harms that emerge from the interplay of
849technologies and social and cultural dynamics. A growing
850body of scholarship has identified a wide range of harms
851across different algorithmic technologies. However,
852computing research and practitioners lack a high level and
853synthesized overview of harms from algorithmic systems
854arising at the micro, meso-, and macro-levels of society. We
855present an applied taxonomy of sociotechnical harms to
856support more systematic surfacing of potential harms in
857algorithmic systems. Based on a scoping review of computing
858research $(n=172)$, we identified five major themes related
859to sociotechnical harms - representational, allocative,
860quality-of-service, interpersonal harms, and social
861system/societal harms - and sub-themes. We describe these
862categories and conclude with a discussion of challenges and
863opportunities for future research.},
864 author = {Shelby, Renee and Rismani, Shalaleh and Henne, Kathryn and
865Moon, Ajung and Rostamzadeh, Negar and Nicholas, Paul and
866Yilla, N'mah and Gallegos, Jess and Smart, Andrew and
867Garcia, Emilio and Virk, Gurleen},
868 title = {Identifying Sociotechnical Harms of Algorithmic Systems:
869Scoping a Taxonomy for Harm Reduction},
870}
871
872@inproceedings{Weidinger2022-ni,
873 location = {Seoul, Republic of Korea},
874 keywords = {responsible AI, language models, risk assessment, responsible
875innovation, technology risks},
876 address = {New York, NY, USA},
877 year = {2022},
878 month = {June},
879 series = {FAccT '22},
880 pages = {214--229},
881 publisher = {Association for Computing Machinery},
882 abstract = {Responsible innovation on large-scale Language Models (LMs)
883requires foresight into and in-depth understanding of the risks
884these models may pose. This paper develops a comprehensive
885taxonomy of ethical and social risks associated with LMs. We
886identify twenty-one risks, drawing on expertise and literature
887from computer science, linguistics, and the social sciences. We
888situate these risks in our taxonomy of six risk areas: I.
889Discrimination, Hate speech and Exclusion, II. Information
890Hazards, III. Misinformation Harms, IV. Malicious Uses, V.
891Human-Computer Interaction Harms, and VI. Environmental and
892Socioeconomic harms. For risks that have already been observed
893in LMs, the causal mechanism leading to harm, evidence of the
894risk, and approaches to risk mitigation are discussed. We
895further describe and analyse risks that have not yet been
896observed but are anticipated based on assessments of other
897language technologies, and situate these in the same taxonomy.
898We underscore that it is the responsibility of organizations to
899engage with the mitigations we discuss throughout the paper. We
900close by highlighting challenges and directions for further
901research on risk evaluation and mitigation with the goal of
902ensuring that language models are developed responsibly.},
903 author = {Weidinger, Laura and Uesato, Jonathan and Rauh, Maribeth and
904Griffin, Conor and Huang, Po-Sen and Mellor, John and Glaese,
905Amelia and Cheng, Myra and Balle, Borja and Kasirzadeh, Atoosa
906and Biles, Courtney and Brown, Sasha and Kenton, Zac and
907Hawkins, Will and Stepleton, Tom and Birhane, Abeba and
908Hendricks, Lisa Anne and Rimell, Laura and Isaac, William and
909Haas, Julia and Legassick, Sean and Irving, Geoffrey and
910Gabriel, Iason},
911 booktitle = {2022 {ACM} Conference on Fairness, Accountability, and
912Transparency},
913 title = {Taxonomy of Risks posed by Language Models},
914}
915
916@article{Stuurman2022-kb,
917 keywords = {AI regulation; Labels; Certification; Self-regulation; Soft law},
918 year = {2022},
919 month = {April},
920 pages = {105657},
921 volume = {44},
922 journal = {Computer Law \& Security Review},
923 abstract = {AI regulation is emerging in the EU. The European authorities,
924NGOs and academics have already issued a series of proposals to
925accommodate the `development and uptake of AI' with an
926`appropriate ethical and legal framework' and promote what the
927European Commission has called an `ecosystem of trust'. In the
928spring of 2020, the European Commission submitted a legislative
929proposal for public consultation including four options ranging
930from ``soft law only'' to a broad scope of mandatory requirements
931and combinations thereof, for addressing the risks linked to the
932development and use of certain AI applications. One year later,
933the Commission unveiled on 21 April 2021 the EU Act on Artificial
934Intelligence.11Regulation of the European Parliament and of the
935Council laying down harmonised rules on artificial intelligence
936(artificial intelligence act) and amending certain union
937legislative acts, Brussels, 21.4.2021 COM (2021) 206 final. The
938proposal primarily focusses on regulating 'high-risk' systems
939through mandatory requirements and prohibition measures. This
940approach leaves a wide range of AI-systems, with potentially
941serious impact on fundamental rights, merely unregulated as
942regards specifically AI related risks. This paper explores the
943boundaries of the impact of the Act for primarily non-high-risk
944systems and discuss the options for introducing a voluntary
945labelling scheme for enhancing protection against the risks of
946medium and low risk AI systems.},
947 author = {Stuurman, Kees and Lachaud, Eric},
948 title = {Regulating {AI}. A label to complete the proposed Act on
949Artificial Intelligence},
950}
951
952@misc{aida-euai,
953 language = {en},
954 note = {Accessed: 2023-2-15},
955 howpublished = {\url{https://www.fasken.com/en/knowledge/2022/10/18-the-regulation-of-artificial-intelligence-in-canada-and-abroad}},
956 year = {2022},
957 month = {October},
958 publisher = {Fasken},
959 title = {The Regulation of Artificial Intelligence in Canada and
960Abroad: Comparing the Proposed {AIDA} and {EU} {AI} Act},
961}
962
963@article{Heikkila2022-ld,
964 language = {en},
965 year = {2022},
966 month = {October},
967 journal = {MIT Technology Review},
968 abstract = {Companies say they want ethical AI. But those working in the
969field say that ambition comes at their expense.},
970 author = {Heikkil{\"a}, Melissa},
971 title = {Responsible {AI} has a burnout problem},
972}
973
974@article{Farr2009-ab,
975 year = {2009},
976 month = {March},
977 pages = {3--8},
978 number = {1},
979 volume = {21},
980 publisher = {Taylor \& Francis},
981 journal = {Engineering Management Journal},
982 abstract = {Abstract:Leadership must be a key element advancing for the
983engineering profession to remain relevant and connected in an
984era of heightened outsourcing and global competition. Companies
985intent on maintaining a competitive edge are calling upon
986educators to produce engineers capable of leading
987multidisciplinary teams, combine technical ingenuity with
988business acumen, and produce graduates who have a passion for
989lifelong learning. Industry is also challenging universities to
990broaden curricula beyond the intellectual endeavors of design
991and scientific inquiry to the greater domain of professional
992leadership and entrepreneurship. Managers in industry are
993similarly challenged to cultivate key leadership attributes in
994junior engineers. This article explores the changing nature of
995engineering in a globally competitive environment and addresses
996why leadership must become a key issue in the career progression
997of engineers. We will present a literature review of leadership
998models along with some proposed solutions for cultivating
999leadership skills as part of the career development process.
1000Lastly, we will present specific recommendations on how to
1001cultivate leadership attributes throughout an engineering
1002career.},
1003 author = {Farr, John V and Brazil, Donna M},
1004 title = {Leadership Skills Development for Engineers},
1005}
1006
1007@article{Farr2009-ab,
1008 year = {2009},
1009 month = {March},
1010 pages = {3--8},
1011 number = {1},
1012 volume = {21},
1013 publisher = {Taylor \& Francis},
1014 journal = {Engineering Management Journal},
1015 abstract = {Abstract:Leadership must be a key element advancing for the
1016engineering profession to remain relevant and connected in an
1017era of heightened outsourcing and global competition. Companies
1018intent on maintaining a competitive edge are calling upon
1019educators to produce engineers capable of leading
1020multidisciplinary teams, combine technical ingenuity with
1021business acumen, and produce graduates who have a passion for
1022lifelong learning. Industry is also challenging universities to
1023broaden curricula beyond the intellectual endeavors of design
1024and scientific inquiry to the greater domain of professional
1025leadership and entrepreneurship. Managers in industry are
1026similarly challenged to cultivate key leadership attributes in
1027junior engineers. This article explores the changing nature of
1028engineering in a globally competitive environment and addresses
1029why leadership must become a key issue in the career progression
1030of engineers. We will present a literature review of leadership
1031models along with some proposed solutions for cultivating
1032leadership skills as part of the career development process.
1033Lastly, we will present specific recommendations on how to
1034cultivate leadership attributes throughout an engineering
1035career.},
1036 author = {Farr, John V and Brazil, Donna M},
1037 title = {Leadership Skills Development for Engineers},
1038}
1039
1040@article{Knight2022-ym,
1041 language = {en},
1042 year = {2022},
1043 month = {November},
1044 journal = {Wired},
1045 abstract = {As part of a wave of layoffs, the new CEO disbanded a group
1046working to make Twitter's algorithms more transparent and fair.},
1047 author = {Knight, Will},
1048 title = {Elon Musk Has Fired Twitter's `Ethical {AI'} Team},
1049}
1050
1051@inproceedings{Bessen2022-gy,
1052 location = {Oxford, United Kingdom},
1053 keywords = {scale barriers, ethics, startups, data, AI},
1054 address = {New York, NY, USA},
1055 year = {2022},
1056 month = {July},
1057 series = {AIES '22},
1058 pages = {92--106},
1059 publisher = {Association for Computing Machinery},
1060 abstract = {Artificial Intelligence startups use training data as direct
1061inputs in product development. These firms must balance numerous
1062tradeoffs between ethical issues and data access without
1063substantive guidance from regulators or existing judicial
1064precedence. We survey these startups to determine what actions
1065they have taken to address these ethical issues and the
1066consequences of those actions. We find that 58\% of these
1067startups have established a set of AI principles. Startups with
1068data-sharing relationships with high-technology firms or that
1069have prior experience with privacy regulations are more likely
1070to establish ethical AI principles and are more likely to take
1071costly steps, like dropping training data or turning down
1072business, to adhere to their ethical AI policies. Moreover,
1073startups with ethical AI policies are more likely to invest in
1074unconscious bias training, hire ethnic minorities and female
1075programmers, seek expert advice, and search for more diverse
1076training data. Potential costs associated with data-sharing
1077relationships and the adherence to ethical policies may create
1078tradeoffs between increased AI product competition and more
1079ethical AI production.},
1080 author = {Bessen, James and Impink, Stephen Michael and Seamans, Robert},
1081 booktitle = {Proceedings of the 2022 {AAAI/ACM} Conference on {AI}, Ethics,
1082and Society},
1083 title = {The Cost of Ethical {AI} Development for {AI} Startups},
1084}
1085
1086@article{mit_2021-dg,
1087 language = {en},
1088 year = {2021},
1089 month = {May},
1090 journal = {MIT Technology Review},
1091 abstract = {Artificial intelligence is changing every industry---from
1092manufacturing to retail. It's also changing the culture at
1093companies as they strive to keep up with accelerating digital
1094technologies.},
1095 title = {Embracing the rapid pace of {AI}},
1096}
1097
1098@inproceedings{Meek2016-wz,
1099 keywords = {Artificial intelligence;Ethics;Technology
1100management;Technological innovation;History;Computers;Process
1101control},
1102 year = {2016},
1103 month = {September},
1104 pages = {682--693},
1105 publisher = {ieeexplore.ieee.org},
1106 abstract = {The development of emergent technologies carries with it ethical
1107issues and risks. We review ways to better manage the ethical
1108issues and risks of one emerging technology: Artificial
1109Intelligence (AI). Depending on how AI's development is managed,
1110it may have beneficial and/or deleterious effects. The
1111processing capacity of Tianhe-2, the world's fastest
1112supercomputer, by some measures, exceeds the processing capacity
1113of a single human brain. but at a prohibitive processing/power
1114consumption ratio and physical size. Given the current pace of
1115AI R\&D activities, some estimates in the literature suggest
1116that the technology could become capable of self-determination
1117and super intelligence in only a few decades. This demands a
1118serious analysis of the ethical implications of AI's development
1119and the risks it might pose, in addition to technology
1120management recommendations. We review the state of AI
1121development, the timeline and scope of its possible future
1122development, and potential ethical risks in its implementation.
1123Further, we briefly review ethics and risk management practices
1124as they relate to technology. Finally, we make technology
1125management recommendations, which may help to address the
1126ethical implications and to mitigate existential risks to
1127humanity-with the development and dissemination of AI-by guiding
1128its proper management.},
1129 author = {Meek, Taylor and Barham, Husam and Beltaif, Nader and Kaadoor,
1130Amani and Akhter, Tanzila},
1131 booktitle = {2016 Portland International Conference on Management of
1132Engineering and Technology ({PICMET})},
1133 title = {Managing the ethical and risk implications of rapid advances in
1134artificial intelligence: A literature review},
1135}
1136
1137@misc{Goldman2022-ip,
1138 note = {Accessed: 2023-3-12},
1139 howpublished = {\url{https://venturebeat.com/ai/why-meta-and-twitters-ai-and-ml-layoffs-matter-the-ai-beat/}},
1140 year = {2022},
1141 month = {November},
1142 author = {Goldman, Sharon},
1143 title = {Why Meta and Twitter's {AI} and {ML} layoffs matter},
1144}
1145
1146@inproceedings{Carter2011-np,
1147 location = {Dallas, TX, USA},
1148 keywords = {soft skills, service learning in computer science, communication},
1149 address = {New York, NY, USA},
1150 year = {2011},
1151 month = {March},
1152 series = {SIGCSE '11},
1153 pages = {517--522},
1154 publisher = {Association for Computing Machinery},
1155 abstract = {Soft skills such as communication, teamwork, and organization
1156are important to students' future success in the working world.
1157Faculty members know it, students know it, and employers are
1158explicitly asking for these skills. Are computer science
1159departments responsible to teach these skills? If so, where in
1160the curriculum should they be covered? This paper explores the
1161soft skills that employers want, and possible places to include
1162the teaching of those skills in the curriculum. It then shows
1163how an extensive set of soft skills were incorporated into a
1164service learning course for the students in the Mathematical,
1165Information and Computer Sciences department at Point Loma
1166Nazarene University. Finally, it makes suggestions as to how
1167other service learning or capstone courses could be altered to
1168afford more opportunity for soft skill education.},
1169 author = {Carter, Lori},
1170 booktitle = {Proceedings of the 42nd {ACM} technical symposium on Computer
1171science education},
1172 title = {Ideas for adding soft skills education to service learning and
1173capstone courses for computer science students},
1174}
1175
1176@article{Hall2001-rg,
1177 language = {en},
1178 year = {2001},
1179 month = {September},
1180 pages = {867--875},
1181 number = {9},
1182 volume = {35},
1183 publisher = {Wiley Online Library},
1184 journal = {Med. Educ.},
1185 abstract = {PURPOSE: This article examines literature on interdisciplinary
1186education and teamwork in health care, to discover the major
1187issues and best practices. METHODS: A literature review of
1188mainly North American articles using search terms such as
1189interdisciplinary, interprofessional, multidisciplinary with
1190medical education. MAIN FINDINGS: Two issues are emerging in
1191health care as clinicians face the complexities of current
1192patient care: the need for specialized health professionals, and
1193the need for these professionals to collaborate.
1194Interdisciplinary health care teams with members from many
1195professions answer the call by working together, collaborating
1196and communicating closely to optimize patient care. Education on
1197how to function within a team is essential if the endeavour is
1198to succeed. Two main categories of issues emerged: those related
1199to the medical education system and those related to the content
1200of the education. CONCLUSIONS: Much of the literature pertained
1201to programme evaluations of academic activities, and did not
1202compare interdisciplinary education with traditional methods.
1203Many questions about when to educate, who to educate and how to
1204educate remain unanswered and open to future research.},
1205 author = {Hall, P and Weaver, L},
1206 title = {Interdisciplinary education and teamwork: a long and winding
1207road},
1208}
1209
1210@article{Dyer2003-bd,
1211 year = {2003},
1212 pages = {186},
1213 number = {4},
1214 volume = {24},
1215 publisher = {journals.lww.com},
1216 journal = {Nurs. Educ. Perspect.},
1217 abstract = {making an informed decision about integrated curriculum
1218development and course implementation, multidisciplinary,
1219interdisciplinary, and transdisciplinary educational teams are
1220defined. Examples are offered that reflect these three
1221integrated educational team models. Finally, the benefits and
1222potential problem areas that result from team initiatives are
1223briefly reviewed....},
1224 author = {Dyer, Jean A},
1225 title = {Multidisciplinary, Interdisciplinary, and
1226{TransdisciplinaryEducational} Models and Nursing Education},
1227}
1228
1229@article{Klaassen2018-tk,
1230 year = {2018},
1231 month = {November},
1232 pages = {842--859},
1233 number = {6},
1234 volume = {43},
1235 publisher = {Taylor \& Francis},
1236 journal = {Eur. J. Eng. Educ.},
1237 abstract = {ABSTRACTToday, interdisciplinary education is a hot topic.
1238Gaining an insight into the nature of interdisciplinary
1239education may help when making design decisions for
1240interdisciplinary education. In this study, we argue that,
1241derived from interdisciplinary research, the choice of problem,
1242the level of interaction between different disciplines and
1243constructive alignment are variables to consider when designing
1244interdisciplinary education. Several models of analysis have
1245been used in two descriptive case studies to gain insight into
1246the design parameters for interdisciplinary education. In this
1247study, we AIM to describe (a) the level and nature of
1248integration, (b) the problem definitions as a guiding principle
1249for constructive alignment for (c) the design and execution of
1250interdisciplinary/transdisciplinary education.},
1251 author = {Klaassen, Renate G},
1252 title = {Interdisciplinary education: a case study},
1253}
1254
1255@article{Braun2006-rj,
1256 year = {2006},
1257 month = {January},
1258 pages = {77--101},
1259 number = {2},
1260 volume = {3},
1261 publisher = {Routledge},
1262 journal = {Qual. Res. Psychol.},
1263 abstract = {Thematic analysis is a poorly demarcated, rarely acknowledged,
1264yet widely used qualitative analytic method within psychology.
1265In this paper, we argue that it offers an accessible and
1266theoretically flexible approach to analysing qualitative data.
1267We outline what thematic analysis is, locating it in relation to
1268other qualitative analytic methods that search for themes or
1269patterns, and in relation to different epistemological and
1270ontological positions. We then provide clear guidelines to those
1271wanting to start thematic analysis, or conduct it in a more
1272deliberate and rigorous way, and consider potential pitfalls in
1273conducting thematic analysis. Finally, we outline the
1274disadvantages and advantages of thematic analysis. We conclude
1275by advocating thematic analysis as a useful and flexible method
1276for qualitative research in and beyond psychology.},
1277 author = {Braun, Virginia and Clarke, Victoria},
1278 title = {Using thematic analysis in psychology},
1279}
1280
1281@article{Mokander2022-ae,
1282 language = {en},
1283 keywords = {Artificial Intelligence; Auditing; Conformity assessment;
1284European Union; Governance; Regulation; Technology},
1285 year = {2022},
1286 pages = {241--268},
1287 number = {2},
1288 volume = {32},
1289 journal = {Minds Mach.},
1290 abstract = {The proposed European Artificial Intelligence Act (AIA) is the
1291first attempt to elaborate a general legal framework for AI
1292carried out by any major global economy. As such, the AIA is
1293likely to become a point of reference in the larger discourse on
1294how AI systems can (and should) be regulated. In this article, we
1295describe and discuss the two primary enforcement mechanisms
1296proposed in the AIA: the conformity assessments that providers of
1297high-risk AI systems are expected to conduct, and the post-market
1298monitoring plans that providers must establish to document the
1299performance of high-risk AI systems throughout their lifetimes.
1300We argue that the AIA can be interpreted as a proposal to
1301establish a Europe-wide ecosystem for conducting AI auditing,
1302albeit in other words. Our analysis offers two main
1303contributions. First, by describing the enforcement mechanisms
1304included in the AIA in terminology borrowed from existing
1305literature on AI auditing, we help providers of AI systems
1306understand how they can prove adherence to the requirements set
1307out in the AIA in practice. Second, by examining the AIA from an
1308auditing perspective, we seek to provide transferable lessons
1309from previous research about how to refine further the regulatory
1310approach outlined in the AIA. We conclude by highlighting seven
1311aspects of the AIA where amendments (or simply clarifications)
1312would be helpful. These include, above all, the need to translate
1313vague concepts into verifiable criteria and to strengthen the
1314institutional safeguards concerning conformity assessments based
1315on internal checks.},
1316 author = {M{\"o}kander, Jakob and Axente, Maria and Casolari, Federico and
1317Floridi, Luciano},
1318 title = {Conformity Assessments and Post-market Monitoring: A Guide to the
1319Role of Auditing in the Proposed European {AI} Regulation},
1320}
1321
1322@techreport{Moss2020,
1323 year = {2020},
1324 title = {{Ethics Owners: A new model of organizational responsibility in data-driven technology companies}},
1325 pages = {1--71},
1326 mendeley-groups = {PhD/AI competency project},
1327 file = {:G$\backslash$:/My Drive/PhD/AI Competency Project/Literature Review/Ethics-Owners{\_}20200923-DataSociety.pdf:pdf},
1328 booktitle = {Data Soc.},
1329 author = {Moss, Emanuel and Metcalf, Jacob},
1330}
1331
1332@inproceedings{Fiesler2020,
1333 year = {2020},
1334 url = {https://doi.org/10.1145/3328778.3366825},
1335 title = {{What Do We Teach When We Teach Tech Ethics? A Syllabi Analysis}},
1336 mendeley-groups = {PhD,PhD/AI competency project},
1337 keywords = {curriculum,ethics,professional responsibility,syllabi},
1338 isbn = {9781450367936},
1339 file = {:C$\backslash$:/Users/shala/AppData/Local/Mendeley Ltd./Mendeley Desktop/Downloaded/Fiesler, Garrett, Beard - 2020 - What Do We Teach When We Teach Tech Ethics A Syllabi Analysis.pdf:pdf},
1340 doi = {10.1145/3328778.3366825},
1341 booktitle = {SIGCSE '20},
1342 author = {Fiesler, Casey and Garrett, Natalie and Beard, Nathan},
1343 abstract = {As issues of technology ethics become more pervasive in the media and public discussions, there is increasing interest in what role ethics should play in computing education. Not only are there more standalone ethics classes being offered at universities, but calls for greater integration of ethics across computer science curriculum mean that a growing number of CS instructors may be including ethics as part of their courses. To both describe current trends in computing ethics coursework and to provide guidance for further ethics inclusion in computing, we present an in-depth qualitative analysis of 115 syllabi from university technology ethics courses. Our analysis contributes a snapshot of the content and goals of tech ethics classes, and recommendations for how these might be integrated across a computing curriculum. CCS CONCEPTS • Social and professional topics → Computing education.},
1344}
1345
1346@article{Borenstein2021,
1347 year = {2020},
1348 volume = {1},
1349 url = {https://doi.org/10.1007/s43681-020-00002-7},
1350 title = {{Emerging challenges in AI and the need for AI ethics education}},
1351 publisher = {Springer International Publishing},
1352 pages = {61--65},
1353 number = {1},
1354 mendeley-groups = {PhD/AI competency project},
1355 keywords = {AI ethics,Artificial intelligence,Design ethics,Ethics education,Professional responsibility,ai ethics,artificial intelligence,design ethics,ethics education,professional responsibility},
1356 journal = {AI Ethics},
1357 issn = {2730-5953},
1358 isbn = {0123456789},
1359 file = {:G$\backslash$:/My Drive/PhD/AI Competency Project/Literature Review/Borenstein-Howard2021{\_}Article{\_}EmergingChallengesInAIAndTheNe.pdf:pdf},
1360 doi = {10.1007/s43681-020-00002-7},
1361 author = {Borenstein, Jason and Howard, Ayanna},
1362 abstract = {Artificial Intelligence (AI) is reshaping the world in profound ways; some of its impacts are certainly beneficial but widespread and lasting harms can result from the technology as well. The integration of AI into various aspects of human life is underway, and the complex ethical concerns emerging from the design, deployment, and use of the technology serves as a reminder that it is time to revisit what future developers and designers, along with professionals, are learning when it comes to AI. It is of paramount importance to train future members of the AI community, and other stakeholders as well, to reflect on the ways in which AI might impact people's lives and to embrace their responsibilities to enhance its benefits while mitigating its potential harms. This could occur in part through the fuller and more systematic inclusion of AI ethics into the curriculum. In this paper, we briefly describe different approaches to AI ethics and offer a set of recommendations related to AI ethics pedagogy.},
1363}
1364
1365@inproceedings{Madaio2020,
1366 year = {2020},
1367 title = {{Co-Designing Checklists to Understand Organizational Challenges and Opportunities around Fairness in AI}},
1368 pages = {1--14},
1369 mendeley-groups = {PhD/AI competency project},
1370 keywords = {"AI,ML,checklists",co-design,ethics,fairness},
1371 isbn = {9781450367080},
1372 file = {:C$\backslash$:/Users/shala/AppData/Local/Mendeley Ltd./Mendeley Desktop/Downloaded/Madaio et al. - 2020 - Co-Designing Checklists to Understand Organizational Challenges and Opportunities around Fairness in AI.pdf:pdf},
1373 doi = {10.1145/3313831.3376445},
1374 booktitle = {Comput. Hum. Interact.},
1375 author = {Madaio, Michael A. and Stark, Luke and {Wortman Vaughan}, Jennifer and Wallach, Hanna},
1376 abstract = {Many organizations have published principles intended to guide the ethical development and deployment of AI systems; however, their abstract nature makes them difficult to oper-ationalize. Some organizations have therefore produced AI ethics checklists, as well as checklists for more specific concepts , such as fairness, as applied to AI systems. But unless checklists are grounded in practitioners' needs, they may be misused. To understand the role of checklists in AI ethics, we conducted an iterative co-design process with 48 practitioners, focusing on fairness. We co-designed an AI fairness checklist and identified desiderata and concerns for AI fairness checklists in general. We found that AI fairness checklists could provide organizational infrastructure for formalizing ad-hoc processes and empowering individual advocates. We discuss aspects of organizational culture that may impact the efficacy of such checklists, and highlight future research directions.},
1377}
1378
1379@article{Raji2020a,
1380 year = {2020},
1381 title = {{Closing the AI accountability gap: Defining an end-to-end framework for internal algorithmic auditing}},
1382 pages = {33--44},
1383 mendeley-groups = {PhD/AI Ethics Metrics Paper/Acct/Resp,PhD/AI competency project},
1384 keywords = {Accountability,Algorithmic audits,Machine learning,Responsible innovation},
1385 journal = {FAT* 2020 - Proc. 2020 Conf. Fairness, Accountability, Transpar.},
1386 isbn = {9781450369367},
1387 file = {:C$\backslash$:/Users/shala/AppData/Local/Mendeley Ltd./Mendeley Desktop/Downloaded/Raji et al. - 2020 - Closing the AI accountability gap Defining an end-to-end framework for internal algorithmic auditing.pdf:pdf},
1388 eprint = {2001.00973},
1389 doi = {10.1145/3351095.3372873},
1390 author = {Raji, Inioluwa Deborah and Smart, Andrew and White, Rebecca N. and Mitchell, Margaret and Gebru, Timnit and Hutchinson, Ben and Smith-Loud, Jamila and Theron, Daniel and Barnes, Parker},
1391 arxivid = {2001.00973},
1392 archiveprefix = {arXiv},
1393 abstract = {Rising concern for the societal implications of artificial intelligence systems has inspired a wave of academic and journalistic literature in which deployed systems are audited for harm by investigators from outside the organizations deploying the algorithms. However, it remains challenging for practitioners to identify the harmful repercussions of their own systems prior to deployment, and, once deployed, emergent issues can become difficult or impossible to trace back to their source. In this paper, we introduce a framework for algorithmic auditing that supports artificial intelligence system development end-to-end, to be applied throughout the internal organization development lifecycle. Each stage of the audit yields a set of documents that together form an overall audit report, drawing on an organization's values or principles to assess the fit of decisions made throughout the process. The proposed auditing framework is intended to contribute to closing the accountability gap in the development and deployment of large-scale artificial intelligence systems by embedding a robust process to ensure audit integrity.},
1394}
1395
1396@article{Jobin2019,
1397 year = {2019},
1398 volume = {1},
1399 url = {http://dx.doi.org/10.1038/s42256-019-0088-2},
1400 title = {{The global landscape of AI ethics guidelines}},
1401 publisher = {Springer US},
1402 pages = {389--399},
1403 number = {9},
1404 mendeley-groups = {PhD,ECSE 681,PhD/AI competency project},
1405 journal = {Nat. Mach. Intell.},
1406 issn = {2522-5839},
1407 isbn = {4225601900},
1408 file = {:C$\backslash$:/Users/shala/AppData/Local/Mendeley Ltd./Mendeley Desktop/Downloaded/Jobin, Ienca, Vayena - 2019 - The global landscape of AI ethics guidelines.pdf:pdf},
1409 doi = {10.1038/s42256-019-0088-2},
1410 author = {Jobin, Anna and Ienca, Marcello and Vayena, Effy},
1411 abstract = {In the past five years, private companies, research institutions and public sector organizations have issued principles and guidelines for ethical artificial intelligence (AI). However, despite an apparent agreement that AI should be ‘ethical', there is debate about both what constitutes ‘ethical AI' and which ethical requirements, technical standards and best practices are needed for its realization. To investigate whether a global agreement on these questions is emerging, we mapped and analysed the current corpus of principles and guidelines on ethical AI. Our results reveal a global convergence emerging around five ethical principles (transparency, justice and fairness, non-maleficence, responsibility and privacy), with substantive divergence in relation to how these principles are interpreted, why they are deemed important, what issue, domain or actors they pertain to, and how they should be implemented. Our findings highlight the importance of integrating guideline-development efforts with substantive ethical analysis and adequate implementation strategies. As AI technology develops rapidly, it is widely recognized that ethical guidelines are required for safe and fair implementation in society. But is it possible to agree on what is ‘ethical AI'? A detailed analysis of 84 AI ethics reports around the world, from national and international organizations, companies and institutes, explores this question, finding a convergence around core principles but substantial divergence on practical implementation.},
1412}
1413
1414@misc{Moon2019,
1415 year = {2019},
1416 title = {{Foresight into AI Ethics}},
1417 publisher = {Open Roboethics Institute},
1418 number = {October},
1419 mendeley-groups = {PhD/AI competency project},
1420 file = {:G$\backslash$:/My Drive/PhD/AI Competency Project/Literature Review/ORI-Foresight-into-Artificial-Intelligence-Ethics-Launch-V1.pdf:pdf},
1421 author = {Moon, AJung and Rismani, Shalaleh and Millar, Jason and Forsyth, Terralynn and Eshpeter, Jordan and Jaffar, Muhammad and Phan, Anh},
1422}
1423
1424@misc{Andersona,
1425 year = {2020},
1426 url = {https://ethicstoolkit.ai/},
1427 title = {{Ethics and algorithms toolkit}},
1428 mendeley-groups = {PhD/AI competency project},
1429 author = {Anderson, David and Bonaguro, Joy and McKinney, Miriam and Nicklin, Andrew and Wiseman, Jane},
1430}
1431
1432@misc{cipd,
1433 year = {2021},
1434 url = {https://www.cipd.co.uk/knowledge/fundamentals/people/performance/competency-factsheet},
1435 title = {{Competence and competency frameworks - factsheets - CIPD}},
1436 mendeley-groups = {PhD/AI competency project},
1437 booktitle = {CIPD},
1438}
1439
1440@misc{ieee,
1441 year = {2022},
1442 title = {{IEEE Ethics in Action in Autonomous and Intelligent Systems}},
1443 mendeley-groups = {PhD/AI competency project},
1444 booktitle = {IEEE},
1445}
1446
1447@article{eu,
1448 year = {2021},
1449 url = {https://digital-strategy.ec.europa.eu/en/policies/european-approach-artificial-intelligence},
1450 title = {{A European approach to artificial intelligence - shaping Europe's digital future}},
1451 mendeley-groups = {PhD/AI competency project},
1452 booktitle = {Eur. Comm.},
1453}
1454
1455@article{microsoft,
1456 year = {2022},
1457 url = {https://www.microsoft.com/en-us/ai/responsible-ai-resources},
1458 title = {{Responsible AI resources}},
1459 mendeley-groups = {PhD/AI competency project},
1460 booktitle = {Microsoft AI},
1461}
1462
1463@article{ibm,
1464 year = {2022},
1465 url = {https://www.ibm.com/artificial-intelligence/ethics},
1466 title = {{AI Ethics Toolkit}},
1467 mendeley-groups = {PhD/AI competency project},
1468 booktitle = {IBM},
1469}
1470
1471@misc{canada,
1472 year = {2022},
1473 url = {https://www.canada.ca/en/government/system/digital-government/digital-government-innovations/responsible-use-ai/algorithmic-impact-assessment.html},
1474 title = {{Algorithmic Impact Assessment}},
1475 mendeley-groups = {PhD/AI competency project},
1476 booktitle = {Gov. Canada},
1477}
1478
1479@book{Sanghi2016,
1480 year = {2016},
1481 title = {{The handbook of competency mapping}},
1482 publisher = {SAGE},
1483 mendeley-groups = {PhD/AI competency project},
1484 edition = {3e},
1485 author = {Sanghi, Seema},
1486}
1487
1488@book{Spencer1993,
1489 year = {1993},
1490 title = {{Competence at Work - Models for Superior Performance}},
1491 publisher = {John Wiley {\&} Sons, Inc.},
1492 mendeley-groups = {PhD/AI competency project},
1493 author = {Spencer, L.M. and Spencer, S.M.},
1494 address = {New York},
1495}
1496
1497@misc{EuropeanCommission2022,
1498 year = {2022},
1499 url = {https://esco.ec.europa.eu/en/classification},
1500 title = {{The ESCO Classification}},
1501 mendeley-groups = {PhD/AI competency project},
1502 author = {{European Commission}},
1503}
1504
1505@misc{Administration,
1506 year = {2022},
1507 url = {https://www.onetcenter.org/content.html},
1508 title = {{O*NET database Content Model}},
1509 mendeley-groups = {PhD/AI competency project},
1510 author = {Administration, Employment {\&} Training},
1511}
1512
1513@article{Heger2022-eo,
1514 keywords = {responsible AI, documentation, machine learning, datasets},
1515 address = {New York, NY, USA},
1516 year = {2022},
1517 month = {November},
1518 pages = {1--29},
1519 number = {CSCW2},
1520 volume = {6},
1521 publisher = {Association for Computing Machinery},
1522 journal = {Proc. ACM Hum.-Comput. Interact.},
1523 abstract = {Data is central to the development and evaluation of machine
1524learning (ML) models. However, the use of problematic or
1525inappropriate datasets can result in harms when the resulting
1526models are deployed. To encourage responsible AI practice
1527through more deliberate reflection on datasets and transparency
1528around the processes by which they are created, researchers and
1529practitioners have begun to advocate for increased data
1530documentation and have proposed several data documentation
1531frameworks. However, there is little research on whether these
1532data documentation frameworks meet the needs of ML
1533practitioners, who both create and consume datasets. To address
1534this gap, we set out to understand ML practitioners' data
1535documentation perceptions, needs, challenges, and desiderata,
1536with the ultimate goal of deriving design requirements that can
1537inform future data documentation frameworks. We conducted a
1538series of semi-structured interviews with 14 ML practitioners at
1539a single large, international technology company. We had them
1540answer a list of questions taken from datasheets for
1541datasets~\textbackslashcitegebru2018datasheets. Our findings
1542show that current approaches to data documentation are largely
1543ad hoc and myopic in nature. Participants expressed needs for
1544data documentation frameworks to be adaptable to their contexts,
1545integrated into their existing tools and workflows, and
1546automated wherever possible. Despite the fact that data
1547documentation frameworks are often motivated from the
1548perspective of responsible AI, participants did not make the
1549connection between the questions that they were asked to answer
1550and their responsible AI implications. In addition, participants
1551often had difficulties prioritizing the needs of dataset
1552consumers and providing information that someone unfamiliar with
1553their datasets might need to know. Based on these findings, we
1554derive seven design requirements for future data documentation
1555frameworks such as more actionable guidance on how the
1556characteristics of datasets might result in harms and how these
1557harms might be mitigated, more explicit prompts for reflection,
1558automated adaptation to different contexts, and integration into
1559ML practitioners' existing tools and workflows.},
1560 author = {Heger, Amy K and Marquis, Liz B and Vorvoreanu, Mihaela and
1561Wallach, Hanna and Wortman Vaughan, Jennifer},
1562 title = {Understanding Machine Learning Practitioners' Data Documentation
1563Perceptions, Needs, Challenges, and Desiderata},
1564}
1565
1566@article{Mantymaki2022-im,
1567 eprint = {2206.00335},
1568 primaryclass = {cs.AI},
1569 archiveprefix = {arXiv},
1570 year = {2022},
1571 month = {June},
1572 abstract = {The organizational use of artificial intelligence (AI) has
1573rapidly spread across various sectors. Alongside the
1574awareness of the benefits brought by AI, there is a growing
1575consensus on the necessity of tackling the risks and
1576potential harms, such as bias and discrimination, brought
1577about by advanced AI technologies. A multitude of AI ethics
1578principles have been proposed to tackle these risks, but the
1579outlines of organizational processes and practices for
1580ensuring socially responsible AI development are in a
1581nascent state. To address the paucity of comprehensive
1582governance models, we present an AI governance framework,
1583the hourglass model of organizational AI governance, which
1584targets organizations that develop and use AI systems. The
1585framework is designed to help organizations deploying AI
1586systems translate ethical AI principles into practice and
1587align their AI systems and processes with the forthcoming
1588European AI Act. The hourglass framework includes governance
1589requirements at the environmental, organizational, and AI
1590system levels. At the AI system level, we connect governance
1591requirements to AI system life cycles to ensure governance
1592throughout the system's life span. The governance model
1593highlights the systemic nature of AI governance and opens
1594new research avenues into its practical implementation, the
1595mechanisms that connect different AI governance layers, and
1596the dynamics between the AI governance actors. The model
1597also offers a starting point for organizational
1598decision-makers to consider the governance components needed
1599to ensure social acceptability, mitigate risks, and realize
1600the potential of AI.},
1601 author = {M{\"a}ntym{\"a}ki, Matti and Minkkinen, Matti and Birkstedt,
1602Teemu and Viljanen, Mika},
1603 title = {Putting {AI} Ethics into Practice: The Hourglass Model of
1604Organizational {AI} Governance},
1605}
1606
1607@article{Atkins2021-ei,
1608 year = {2021},
1609 publisher = {researchgate.net},
1610 journal = {Behav. Res. Methods Instrum. Comput.},
1611 abstract = {… frameworks, we evaluated four commercial chatbots against four
1612responsible AI frameworks. We found that the ethical frameworks
1613produced quite di erent assessment scores. Many ethi…},
1614 author = {Atkins, Suzanne and Badrie, Ishwarradj and van Otterloo,
1615Sieuwert},
1616 title = {Applying Ethical {AI} Frameworks in practice: Evaluating
1617conversational {AI} chatbot solutions},
1618}
1619
1620@article{Wang2023-js,
1621 year = {2023},
1622 publisher = {research.google},
1623 abstract = {… ized in practice across sectors and organizations [61]. We use
1624the term `` responsible AI '' by its … primarily opted to defne
1625responsible AI in terms of the practices they used to enact it,
1626rather …},
1627 author = {Wang, Qiaosi and Madaio, Michael Adam and Kapania, Shivani and
1628Kane, Shaun and Terry, Michael and Wilcox, Lauren},
1629 title = {Designing responsible {AI}: Adaptations of {UX} practice to meet
1630responsible {AI} challenges},
1631}
1632
1633@techreport{Pak-Hang_Wong2020-jv,
1634 year = {2020},
1635 month = {February},
1636 author = {Pak-Hang Wong, Judith Simon},
1637 title = {Thinking About `Ethics' in the Ethics of {AI}},
1638}
1639
1640@article{Widder2023-lh,
1641 year = {2023},
1642 month = {January},
1643 pages = {20539517231177620},
1644 number = {1},
1645 volume = {10},
1646 publisher = {SAGE Publications Ltd},
1647 journal = {Big Data \& Society},
1648 abstract = {Responsible artificial intelligence guidelines ask engineers to
1649consider how their systems might harm. However, contemporary
1650artificial intelligence systems are built by composing many
1651preexisting software modules that pass through many hands before
1652becoming a finished product or service. How does this shape
1653responsible artificial intelligence practice? In interviews with
165427 artificial intelligence engineers across industry, open
1655source, and academia, our participants often did not see the
1656questions posed in responsible artificial intelligence
1657guidelines to be within their agency, capability, or
1658responsibility to address. We use Suchman's ?located
1659accountability? to show how responsible artificial intelligence
1660labor is currently organized and to explore how it could be done
1661differently. We identify cross-cutting social logics, like
1662modularizability, scale, reputation, and customer orientation,
1663that organize which responsible artificial intelligence actions
1664do take place and which are relegated to low status staff or
1665believed to be the work of the next or previous person in the
1666imagined ?supply chain.? We argue that current responsible
1667artificial intelligence interventions, like ethics checklists
1668and guidelines that assume panoptical knowledge and control over
1669systems, could be improved by taking a located accountability
1670approach, recognizing where relations and obligations might
1671intertwine inside and outside of this supply chain.},
1672 author = {Widder, David Gray and Nafus, Dawn},
1673 title = {Dislocated accountabilities in the {``AI} supply chain'':
1674Modularity and developers' notions of responsibility},
1675}
1676
1677@article{Figueras2022-dm,
1678 year = {2022},
1679 pages = {6},
1680 number = {2},
1681 volume = {34},
1682 publisher = {aisel.aisnet.org},
1683 journal = {Scandinavian Journal of Information Systems},
1684 abstract = {The increasing use of Artificial Intelligence (AI) systems has
1685sparked discussions regarding developing ethically responsible
1686technology. Consequently, various organizations have released
1687high-level AI ethics frameworks to assist in AI design. However,
1688we still know too little about how AI ethics principles are
1689perceived and work in practice, especially in public
1690organizations. This study examines how AI practitioners perceive
1691ethical issues in their work concerning AI design and how they
1692interpret and put them into practice. We conducted an empirical
1693study consisting of semi-structured qualitative interviews with
1694AI practitioners working in or for public organizations. Taking
1695the lens provided by the In-Action Ethics framework and previous
1696studies on ethical tensions, we analyzed practitioners'
1697interpretations of AI ethics principles and their application in
1698practice. We found tensions between practitioners'
1699interpretation of ethical principles in their work and ethos
1700tensions. In this vein, we argue that understanding the
1701different tensions that can occur in practice and how they are
1702tackled is key to studying ethics in practice. Understanding how
1703AI practitioners perceive and apply ethical principles is
1704necessary for practical ethics to contribute toward an
1705empirically grounded, Responsible AI.},
1706 author = {Figueras, Cl{\`a}udia and Verhagen, Harko and Pargman, Teresa
1707Cerratto},
1708 title = {Exploring tensions in Responsible {AI} in practice. An interview
1709study on {AI} practices in and for Swedish public organizations},
1710}
1711
1712@article{Nabavi2023-ce,
1713 language = {en},
1714 year = {2023},
1715 month = {March},
1716 pages = {1--9},
1717 number = {1},
1718 volume = {10},
1719 publisher = {Palgrave},
1720 journal = {Humanities and Social Sciences Communications},
1721 abstract = {There is a growing debate amongst academics and practitioners on
1722whether interventions made, thus far, towards Responsible AI
1723have been enough to engage with the root causes of AI problems.
1724Failure to effect meaningful changes in this system could see
1725these initiatives not reach their potential and lead to the
1726concept becoming another buzzword for companies to use in their
1727marketing campaigns. Systems thinking is often touted as a
1728methodology to manage and effect change; however, there is
1729little practical advice available for decision-makers to include
1730systems thinking insights to work towards Responsible AI. Using
1731the notion of `leverage zones' adapted from the systems thinking
1732literature, we suggest a novel approach to plan for and
1733experiment with potential initiatives and interventions. This
1734paper presents a conceptual framework called the Five Ps to help
1735practitioners construct and identify holistic interventions that
1736may work towards Responsible AI, from lower-order interventions
1737such as short-term fixes, tweaking algorithms and updating
1738parameters, through to higher-order interventions such as
1739redefining the system's foundational structures that govern
1740those parameters, or challenging the underlying purpose upon
1741which those structures are built and developed in the first
1742place. Finally, we reflect on the framework as a scaffold for
1743transdisciplinary question-asking to improve outcomes towards
1744Responsible AI.},
1745 author = {Nabavi, Ehsan and Browne, Chris},
1746 title = {Leverage zones in Responsible {AI}: towards a systems thinking
1747conceptualization},
1748}
1749
1750@article{Schiff2020-mq,
1751 eprint = {2006.04707},
1752 primaryclass = {cs.CY},
1753 archiveprefix = {arXiv},
1754 year = {2020},
1755 month = {June},
1756 abstract = {Companies have considered adoption of various high-level
1757artificial intelligence (AI) principles for responsible AI,
1758but there is less clarity on how to implement these
1759principles as organizational practices. This paper reviews
1760the principles-to-practices gap. We outline five
1761explanations for this gap ranging from a disciplinary divide
1762to an overabundance of tools. In turn, we argue that an
1763impact assessment framework which is broad,
1764operationalizable, flexible, iterative, guided, and
1765participatory is a promising approach to close the
1766principles-to-practices gap. Finally, to help practitioners
1767with applying these recommendations, we review a case study
1768of AI's use in forest ecosystem restoration, demonstrating
1769how an impact assessment framework can translate into
1770effective and responsible AI practices.},
1771 author = {Schiff, Daniel and Rakova, Bogdana and Ayesh, Aladdin and
1772Fanti, Anat and Lennon, Michael},
1773 title = {Principles to Practices for Responsible {AI}: Closing the
1774Gap},
1775}
1776
1777@inproceedings{Deshpande2022-rv,
1778 location = {Oxford, United Kingdom},
1779 keywords = {ISO 26000:2010 guidance on social responsibility, AI system
1780builders, responsible AI systems, AI ethics, corporate social
1781responsibility, stakeholder identification},
1782 address = {New York, NY, USA},
1783 year = {2022},
1784 month = {July},
1785 series = {AIES '22},
1786 pages = {227--236},
1787 publisher = {Association for Computing Machinery},
1788 abstract = {As of 2021, there were more than 170 guidelines on AI ethics and
1789responsible, trustworthy AI in circulation according to the AI
1790Ethics Guidelines Global Inventory maintained by AlgorithmWatch,
1791an organisation which tracks the effects of increased
1792digitalisation on everyday lives. However, from the perspective
1793of day-to-day work, for those engaged in designing, developing,
1794and maintaining AI systems identifying relevant guidelines and
1795translating them into practice presents a challenge.The aim of
1796this paper is to help anyone engaged in building a responsible
1797AI system by identifying an indicative long-list of potential
1798stakeholders. This list of impacted stakeholders is intended to
1799enable such AI system builders to decide which guidelines are
1800most suited to their practice. The paper draws on a literature
1801review of articles short-listed based on searches conducted in
1802the ACM Digital Library and Google Scholar. The findings are
1803based on content analysis of the short-listed literature guided
1804by probes which draw on the ISO 26000:2010 Guidance on social
1805responsibility.The paper identifies three levels of potentially
1806relevant stakeholders when responsible AI systems are
1807considered: individual stakeholders (including users,
1808developers, and researchers), organisational stakeholders, and
1809national / international stakeholders engaged in making laws,
1810rules, and regulations. The main intended audience for this
1811paper is software, requirements, and product engineers engaged
1812in building AI systems. In addition, business executives, policy
1813makers, legal/regulatory experts, AI researchers, public,
1814private, and third sector organisations developing responsible
1815AI guidelines, and anyone interested in seeing functional
1816responsible AI systems are the other intended audience for this
1817paper.},
1818 author = {Deshpande, Advait and Sharp, Helen},
1819 booktitle = {Proceedings of the 2022 {AAAI/ACM} Conference on {AI}, Ethics,
1820and Society},
1821 title = {Responsible {AI} Systems: Who are the Stakeholders?},
1822}
1823
1824@misc{Heger_undated-ke,
1825 note = {Accessed: 2023-3-11},
1826 howpublished = {\url{https://ai-cultures.github.io/papers/all_the_tools_none_of_the_moti.pdf}},
1827 abstract = {As applications of artificial intelligence (AI) have
1828proliferated so too have ethical concerns regarding their
1829potential to cause harm to society. As a result, many
1830organizations that build or use AI systems have developed
1831frameworks or codes of conduct specifying ethical or
1832responsible AI principles they strive to follow (e.g.},
1833 author = {Heger, Amy and Passi, Samir and Vorvoreanu, Mihaela},
1834 title = {All the tools, none of the motivation: Organizational culture
1835and barriers to responsible {AI} work},
1836}
1837
1838@article{Schiff2021-et,
1839 keywords = {Economics;Social factors;Education;Medical services;Artificial
1840intelligence;Sustainable development;Autonomous vehicles},
1841 year = {2021},
1842 month = {June},
1843 pages = {81--94},
1844 number = {2},
1845 volume = {40},
1846 publisher = {ieeexplore.ieee.org},
1847 journal = {IEEE Technol. Soc. Mag.},
1848 abstract = {As artificial intelligence (AI) permeates across social and
1849economic life, its ethical and governance implications have come
1850to the forefront. Active debates surround AI's role in labor
1851displacement, autonomous vehicles, military, misinformation,
1852healthcare, education, and more. As societies collectively
1853grapple with these challenges, new opportunities for AI to
1854proactively contribute to social good (AI4SG) and equity (AI4Eq)
1855have also been proposed [1], [2], such as Microsoft's AI for
1856Earth program. These efforts highlight the potential of AI to
1857address global challenges and help achieve targets like the
1858United Nation's sustainable development goals (SDGs) [3]. Yet,
1859whether AI efforts are directed explicitly at social good and
1860equity or not, there are many barriers that stand between
1861aspirations to be responsible and the translation of these
1862aspirations into concrete practicalities.},
1863 author = {Schiff, Daniel and Rakova, Bogdana and Ayesh, Aladdin and Fanti,
1864Anat and Lennon, Michael},
1865 title = {Explaining the Principles to Practices Gap in {AI}},
1866}
1867
1868@article{Rakova2021-dg,
1869 keywords = {responsible ai, organizational structure, industry practice},
1870 address = {New York, NY, USA},
1871 year = {2021},
1872 month = {April},
1873 pages = {1--23},
1874 number = {CSCW1},
1875 volume = {5},
1876 publisher = {Association for Computing Machinery},
1877 journal = {Proc. ACM Hum.-Comput. Interact.},
1878 abstract = {Large and ever-evolving technology companies continue to invest
1879more time and resources to incorporate responsible Artificial
1880Intelligence (AI) into production-ready systems to increase
1881algorithmic accountability. This paper examines and seeks to
1882offer a framework for analyzing how organizational culture and
1883structure impact the effectiveness of responsible AI initiatives
1884in practice. We present the results of semi-structured
1885qualitative interviews with practitioners working in industry,
1886investigating common challenges, ethical tensions, and effective
1887enablers for responsible AI initiatives. Focusing on major
1888companies developing or utilizing AI, we have mapped what
1889organizational structures currently support or hinder
1890responsible AI initiatives, what aspirational future processes
1891and structures would best enable effective initiatives, and what
1892key elements comprise the transition from current work practices
1893to the aspirational future.},
1894 author = {Rakova, Bogdana and Yang, Jingying and Cramer, Henriette and
1895Chowdhury, Rumman},
1896 title = {Where Responsible {AI} meets Reality: Practitioner Perspectives
1897on Enablers for Shifting Organizational Practices},
1898}
1899
1900@article{Dignum2021-xt,
1901 language = {en},
1902 year = {2021},
1903 month = {January},
1904 number = {1},
1905 volume = {19},
1906 publisher = {UCL Press},
1907 journal = {Lond. Rev. Educ.},
1908 abstract = {Artificial intelligence (AI) is impacting education in many
1909different ways. From virtual assistants for personalized
1910education, to student or teacher tracking systems, the potential
1911benefits of AI for education often come with a discussion of its
1912impact on privacy and well-being. At the same time, the social
1913transformation brought about by AI requires reform of
1914traditional education systems. This article discusses what a
1915responsible, trustworthy vision for AI is and how this relates
1916to and affects education.},
1917 author = {Dignum, Virginia},
1918 title = {The role and challenges of education for responsible {AI}},
1919}
1920
1921@article{Gambelin2021-rh,
1922 year = {2021},
1923 month = {February},
1924 pages = {87--91},
1925 number = {1},
1926 volume = {1},
1927 journal = {AI and Ethics},
1928 abstract = {Despite there being a strong call for responsible technology, the
1929path towards putting ethics into action is still yet to be fully
1930understood. To help guide the implementation of ethics, we have
1931seen the rise of a new professional title; the AI Ethicist.
1932However, it is still unclear what the role and skill set of this
1933new profession must include. The purpose of this piece is to
1934offer a preliminary definition of what it means to be an AI
1935Ethicist by first examining the concept of an ethicist in the
1936context of artificial intelligence, followed by exploring what
1937responsibilities are added to the role in industry specifically,
1938and ending on the fundamental characteristic that underlies it
1939all: bravery.},
1940 author = {Gambelin, Olivia},
1941 title = {Brave: what it means to be an {AI} Ethicist},
1942}
1943
1944@article{Peterson2023-xa,
1945 keywords = {Ethics;Computer
1946science;Writing;Faces;Cognition;Codes;Philosophical
1947considerations;power;abstraction;responsibility;social
1948impact;emotional engagement;ethics},
1949 year = {2023},
1950 pages = {1--1},
1951 publisher = {ieeexplore.ieee.org},
1952 journal = {IEEE Transactions on Technology and Society},
1953 abstract = {As computing becomes more powerful and extends the reach of
1954those who wield it, the imperative grows for computing
1955professionals to make ethical decisions regarding the use of
1956that power. We propose the concept of abstracted power to help
1957computer science students understand how technology may distance
1958them perceptually from consequences of their actions.
1959Specifically, we identify technological intermediation and
1960computational thinking as two factors in computer science that
1961contribute to this distancing. To counter the abstraction of
1962power, we argue for increased emotional engagement in computer
1963science ethics education, to encourage students to feel as well
1964as think regarding the potential impacts of their power on
1965others. We suggest four concrete pedagogical approaches to
1966enable this emotional engagement in computer science ethics
1967curriculum, and we share highlights of student reactions to the
1968material.},
1969 author = {Peterson, Tina L and Ferreira, Rodrigo and Vardi, Moshe Y},
1970 title = {Abstracted Power and Responsibility in Computer Science Ethics
1971Education},
1972}
1973
1974@article{Ryan2022-ej,
1975 language = {en},
1976 copyright = {https://creativecommons.org/licenses/by/4.0},
1977 year = {2022},
1978 month = {March},
1979 publisher = {Springer Science and Business Media LLC},
1980 journal = {AI Soc.},
1981 abstract = {AbstractArtificial intelligence ethics requires a united
1982approach from policymakers, AI companies, and individuals, in
1983the development, deployment, and use of these technologies.
1984However, sometimes discussions can become fragmented because of
1985the different levels of governance (Schmitt in AI Ethics 1--12,
19862021) or because of different values, stakeholders, and actors
1987involved (Ryan and Stahl in J Inf Commun Ethics Soc 19:61--86,
19882021). Recently, these conflicts became very visible, with such
1989examples as the dismissal of AI ethics researcher Dr. Timnit
1990Gebru from Google and the resignation of whistle-blower Frances
1991Haugen from Facebook. Underpinning each debacle was a conflict
1992between the organisation's economic and business interests and
1993the morals of their employees. This paper will examine tensions
1994between the ethics of AI organisations and the values of their
1995employees, by providing an exploration of the AI ethics
1996literature in this area, and a qualitative analysis of three
1997workshops with AI developers and practitioners. Common ethical
1998and social tensions (such as power asymmetries, mistrust,
1999societal risks, harms, and lack of transparency) will be
2000discussed, along with proposals on how to avoid or reduce these
2001conflicts in practice (e.g., building trust, fair allocation of
2002responsibility, protecting employees' autonomy, and encouraging
2003ethical training and practice). Altogether, we suggest the
2004following steps to help reduce ethical issues within AI
2005organisations: improved and diverse ethics education and
2006training within businesses; internal and external ethics
2007auditing; the establishment of AI ethics ombudsmen, AI ethics
2008review committees and an AI ethics watchdog; as well as access
2009to trustworthy AI ethics whistle-blower organisations.},
2010 author = {Ryan, Mark and Christodoulou, Eleni and Antoniou, Josephina and
2011Iordanou, Kalypso},
2012 title = {An {AI} ethics `David and Goliath': value conflicts between
2013large tech companies and their employees},
2014}
2015
2016@inproceedings{Gorur2020-vu,
2017 year = {2020},
2018 institution = {IEEE},
2019 pages = {945--947},
2020 publisher = {ieeexplore.ieee.org},
2021 abstract = {… the key ethical requirements for AI development and … in AI
2022environments are well aware of potential consequences of the
2023technologies they develop and deploy, sensitive to the ethical
2024…},
2025 author = {Gorur, Radhika and Hoon, Leonard and Kowal, Emma},
2026 booktitle = {2020 {IEEE} International Conference on Teaching, Assessment,
2027and Learning for Engineering ({TALE})},
2028 title = {Computer Science Ethics Education in {Australia--A} Work in
2029Progress},
2030}
2031
2032@article{Williams2020-ar,
2033 language = {en},
2034 year = {2020},
2035 month = {April},
2036 pages = {13428--13435},
2037 number = {09},
2038 volume = {34},
2039 publisher = {ojs.aaai.org},
2040 journal = {AAAI},
2041 abstract = {We propose an experimental ethics-based curricular module for an
2042undergraduate course on Robot Ethics. The proposed module aims
2043to teach students how human subjects research methods can be
2044used to investigate potential ethical concerns arising in
2045human-robot interaction, by engaging those students in real
2046experimental ethics research. In this paper we describe the
2047proposed curricular module, describe our implementation of that
2048module within a Robot Ethics course offered at a medium-sized
2049engineering university, and statistically evaluate the
2050effectiveness of the proposed curricular module in achieving
2051desired learning objectives. While our results do not provide
2052clear evidence of a quantifiable benefit to undergraduate
2053achievement of the described learning objectives, we note that
2054the module did provide additional learning opportunities for
2055graduate students in the course, as they helped to supervise,
2056analyze, and write up the results of this
2057undergraduate-performed research experiment.},
2058 author = {Williams, Tom and Zhu, Qin and Grollman, Daniel},
2059 title = {An Experimental Ethics Approach to Robot Ethics Education},
2060}
2061
2062@inproceedings{Williams2021-sd,
2063 location = {Virtual Event, USA},
2064 keywords = {middle school, cs education, machine learning, ai literacy},
2065 address = {New York, NY, USA},
2066 year = {2021},
2067 month = {March},
2068 series = {SIGCSE '21},
2069 pages = {1382},
2070 publisher = {Association for Computing Machinery},
2071 abstract = {We developed the How to Train Your Robot curriculum to empower
2072middle school students to become conscientious users and
2073creators of Artificial Intelligence (AI). As AI becomes more
2074embedded in our daily lives, all members of society should have
2075the opportunity to become AI literate. Today, most deployed work
2076in K-12 AI education takes place at strong STEM schools or
2077during extracurricular clubs. But, to promote equity in the
2078field of AI, we must also design curricula for classroom use at
2079schools with limited resources. How to Train Your Robot
2080leverages a low-cost (\$40) robot, a block-based programming
2081platform, novice-friendly model creation tools, and hands-on
2082activities to introduce students to machine learning. During the
2083summer of 2020, we trained in-service teachers, primarily from
2084Title 1 public schools, to deliver a five-day, online version of
2085the curriculum to their students. In this work, we describe how
2086students' self-directed final projects demonstrate their
2087understanding of technical and ethical AI concepts. Students
2088successfully selected project ideas, taking the strengths and
2089weaknesses of machine learning into account, and implemented an
2090array of projects about everything from entertainment to
2091science. We saw that students had the most difficulty designing
2092mechanisms to respond to user feedback after deployment. We hope
2093this work inspires future AI curricula that can be used in
2094middle school classrooms.},
2095 author = {Williams, Randi},
2096 booktitle = {Proceedings of the 52nd {ACM} Technical Symposium on Computer
2097Science Education},
2098 title = {How to Train Your Robot: {Project-Based} {AI} and Ethics
2099Education for Middle School Classrooms},
2100}
2101
2102@article{Quinn2021-jj,
2103 eprint = {2109.02866},
2104 primaryclass = {cs.AI},
2105 archiveprefix = {arXiv},
2106 year = {2021},
2107 month = {September},
2108 abstract = {Medical students will almost inevitably encounter powerful
2109medical AI systems early in their careers. Yet, contemporary
2110medical education does not adequately equip students with
2111the basic clinical proficiency in medical AI needed to use
2112these tools safely and effectively. Education reform is
2113urgently needed, but not easily implemented, largely due to
2114an already jam-packed medical curricula. In this article, we
2115propose an education reform framework as an effective and
2116efficient solution, which we call the Embedded AI Ethics
2117Education Framework. Unlike other calls for education reform
2118to accommodate AI teaching that are more radical in scope,
2119our framework is modest and incremental. It leverages
2120existing bioethics or medical ethics curricula to develop
2121and deliver content on the ethical issues associated with
2122medical AI, especially the harms of technology misuse,
2123disuse, and abuse that affect the risk-benefit analyses at
2124the heart of healthcare. In doing so, the framework provides
2125a simple tool for going beyond the ``What?'' and the
2126``Why?'' of medical AI ethics education, to answer the
2127``How?'', giving universities, course directors, and/or
2128professors a broad road-map for equipping their students
2129with the necessary clinical proficiency in medical AI.},
2130 author = {Quinn, Thomas P and Coghlan, Simon},
2131 title = {Readying Medical Students for Medical {AI}: The Need to
2132Embed {AI} Ethics Education},
2133}
2134
2135@inproceedings{Garrett2020-dw,
2136 location = {New York NY USA},
2137 conference = {AIES '20: AAAI/ACM Conference on AI, Ethics, and Society},
2138 address = {New York, NY, USA},
2139 year = {2020},
2140 month = {February},
2141 publisher = {ACM},
2142 abstract = {… Therefore, to explore one component of the current state of
2143AI ethics education , we examine a sample of these classes for
2144patterns in what topics are currently being taught. …},
2145 author = {Garrett, Natalie and Beard, Nathan and Fiesler, Casey},
2146 booktitle = {Proceedings of the {AAAI/ACM} Conference on {AI}, Ethics, and
2147Society},
2148 title = {More than ``if time allows'': The Role of Ethics in {AI}
2149Education},
2150}
2151
2152@inproceedings{Raji2021-ih,
2153 location = {Virtual Event, Canada},
2154 address = {New York, NY, USA},
2155 year = {2021},
2156 month = {March},
2157 series = {FAccT '21},
2158 pages = {515--525},
2159 publisher = {Association for Computing Machinery},
2160 abstract = {Given a growing concern about the lack of ethical consideration
2161in the Artificial Intelligence (AI) field, many have begun to
2162question how dominant approaches to the disciplinary education
2163of computer science (CS)---and its implications for AI---has led
2164to the current ``ethics crisis''. However, we claim that the
2165current AI ethics education space relies on a form of
2166``exclusionary pedagogy,'' where ethics is distilled for
2167computational approaches, but there is no deeper epistemological
2168engagement with other ways of knowing that would benefit ethical
2169thinking or an acknowledgement of the limitations of uni-vocal
2170computational thinking. This results in indifference,
2171devaluation, and a lack of mutual support between CS and
2172humanistic social science (HSS), elevating the myth of
2173technologists as ``ethical unicorns'' that can do it all, though
2174their disciplinary tools are ultimately limited. Through an
2175analysis of computer science education literature and a review
2176of college-level course syllabi in AI ethics, we discuss the
2177limitations of the epistemological assumptions and hierarchies
2178of knowledge which dictate current attempts at including ethics
2179education in CS training and explore evidence for the practical
2180mechanisms through which this exclusion occurs. We then propose
2181a shift towards a substantively collaborative, holistic, and
2182ethically generative pedagogy in AI education.},
2183 author = {Raji, Inioluwa Deborah and Scheuerman, Morgan Klaus and
2184Amironesei, Razvan},
2185 booktitle = {Proceedings of the 2021 {ACM} Conference on Fairness,
2186Accountability, and Transparency},
2187 title = {You Can't Sit With Us: Exclusionary Pedagogy in {AI} Ethics
2188Education},
2189}
2190
2191@article{Furey2019-xz,
2192 address = {New York, NY, USA},
2193 year = {2019},
2194 month = {January},
2195 pages = {13--15},
2196 number = {4},
2197 volume = {4},
2198 publisher = {Association for Computing Machinery},
2199 journal = {AI Matters},
2200 abstract = {In this column, we introduce our Model AI Assignment, A Module
2201on Ethical Thinking about Autonomous Vehicles in an AI Course,
2202and more broadly introduce a conversation on ethics education in
2203AI education.},
2204 author = {Furey, Heidi and Martin, Fred},
2205 title = {{AI} education matters: a modular approach to {AI} ethics
2206education},
2207}
2208
2209@article{Borenstein2021-kf,
2210 language = {en},
2211 year = {2021},
2212 month = {February},
2213 pages = {61--65},
2214 number = {1},
2215 volume = {1},
2216 publisher = {Springer Science and Business Media LLC},
2217 journal = {AI Ethics},
2218 abstract = {… approaches to AI ethics and offer a set of recommendations
2219related to AI ethics pedagogy. … Within this realm, we highlight
2220recent approaches to AI ethics education , especially as they …},
2221 author = {Borenstein, Jason and Howard, Ayanna},
2222 title = {Emerging challenges in {AI} and the need for {AI} ethics
2223education},
2224}
2225
2226@inproceedings{Costanza-Chock2022-ch,
2227 location = {Seoul, Republic of Korea},
2228 keywords = {algorithm audit, ethical AI, AI bias, AI audit, audit,
2229algorithmic accountability, AI policy, AI harm},
2230 address = {New York, NY, USA},
2231 year = {2022},
2232 month = {June},
2233 series = {FAccT '22},
2234 pages = {1571--1583},
2235 publisher = {Association for Computing Machinery},
2236 abstract = {Algorithmic audits (or `AI audits') are an increasingly popular
2237mechanism for algorithmic accountability; however, they remain
2238poorly defined. Without a clear understanding of audit
2239practices, let alone widely used standards or regulatory
2240guidance, claims that an AI product or system has been audited,
2241whether by first-, second-, or third-party auditors, are
2242difficult to verify and may potentially exacerbate, rather than
2243mitigate, bias and harm. To address this knowledge gap, we
2244provide the first comprehensive field scan of the AI audit
2245ecosystem. We share a catalog of individuals (N=438) and
2246organizations (N=189) who engage in algorithmic audits or whose
2247work is directly relevant to algorithmic audits; conduct an
2248anonymous survey of the group (N=152); and interview industry
2249leaders (N=10). We identify emerging best practices as well as
2250methods and tools that are becoming commonplace, and enumerate
2251common barriers to leveraging algorithmic audits as effective
2252accountability mechanisms. We outline policy recommendations to
2253improve the quality and impact of these audits, and highlight
2254proposals with wide support from algorithmic auditors as well as
2255areas of debate. Our recommendations have implications for
2256lawmakers, regulators, internal company policymakers, and
2257standards-setting bodies, as well as for auditors. They are: 1)
2258require the owners and operators of AI systems to engage in
2259independent algorithmic audits against clearly defined
2260standards; 2) notify individuals when they are subject to
2261algorithmic decision-making systems; 3) mandate disclosure of
2262key components of audit findings for peer review; 4) consider
2263real-world harm in the audit process, including through
2264standardized harm incident reporting and response mechanisms; 5)
2265directly involve the stakeholders most likely to be harmed by AI
2266systems in the algorithmic audit process; and 6) formalize
2267evaluation and, potentially, accreditation of algorithmic
2268auditors.},
2269 author = {Costanza-Chock, Sasha and Raji, Inioluwa Deborah and Buolamwini,
2270Joy},
2271 booktitle = {2022 {ACM} Conference on Fairness, Accountability, and
2272Transparency},
2273 title = {Who Audits the Auditors? Recommendations from a field scan of
2274the algorithmic auditing ecosystem},
2275}
2276
2277@inproceedings{Sloane2022-ag,
2278 location = {Seoul, Republic of Korea},
2279 keywords = {regulation, start-ups, fairness, social practice, transparency,
2280innovation, AI ethics, organizations, socio-cultural history,
2281accountability},
2282 address = {New York, NY, USA},
2283 year = {2022},
2284 month = {June},
2285 series = {FAccT '22},
2286 pages = {935--947},
2287 publisher = {Association for Computing Machinery},
2288 abstract = {The current AI ethics discourse focuses on developing
2289computational interpretations of ethical concerns, normative
2290frameworks, and concepts for socio-technical innovation. There
2291is less emphasis on understanding how AI practitioners
2292themselves understand ethics and socially organize to
2293operationalize ethical concerns. This is particularly true for
2294AI start-ups, despite their significance as a conduit for the
2295cultural production of innovation and progress, especially in
2296the US and European context. This gap in empirical research
2297intensifies the risk of a disconnect between scholarly research,
2298innovation and application. This risk materializes acutely as
2299mounting pressures to identify and mitigate the potential harms
2300of AI systems have created an urgent need to rapidly assess and
2301implement socio-technical innovation focused on fairness,
2302accountability, and transparency. In this paper, we address this
2303need. Building on social practice theory, we propose a framework
2304that allows AI researchers, practitioners, and regulators to
2305systematically analyze existing cultural understandings,
2306histories, and social practices of ``ethical AI'' to define
2307appropriate strategies for effectively implementing
2308socio-technical innovations. We argue that this approach is
2309needed because socio-technical innovation ``sticks'' better if
2310it sustains the cultural meaning of socially shared (ethical) AI
2311practices, rather than breaking them. By doing so, it creates
2312pathways for technical and socio-technical innovations to be
2313integrated into already existing routines. Against that
2314backdrop, our contributions are threefold: (1) we introduce a
2315practice-based approach for understanding ``ethical AI''; (2) we
2316present empirical findings from our study on the
2317operationalization of ``ethics'' in German AI start-ups to
2318underline that AI ethics and social practices must be understood
2319in their specific cultural and historical contexts; and (3)
2320based on our empirical findings, suggest that ``ethical AI''
2321practices can be broken down into principles, needs, narratives,
2322materializations, and cultural genealogies to form a useful
2323backdrop for considering socio-technical innovations. We
2324conclude with critical reflections and practical implications of
2325our work, as well as recommendations for future research.},
2326 author = {Sloane, Mona and Zakrzewski, Janina},
2327 booktitle = {2022 {ACM} Conference on Fairness, Accountability, and
2328Transparency},
2329 title = {German {AI} {Start-Ups} and {``AI} Ethics'': Using A Social
2330Practice Lens for Assessing and Implementing {Socio-Technical}
2331Innovation},
2332}
Attribution
arXiv:2205.03946v2
[cs.CY]
License: cc-by-4.0