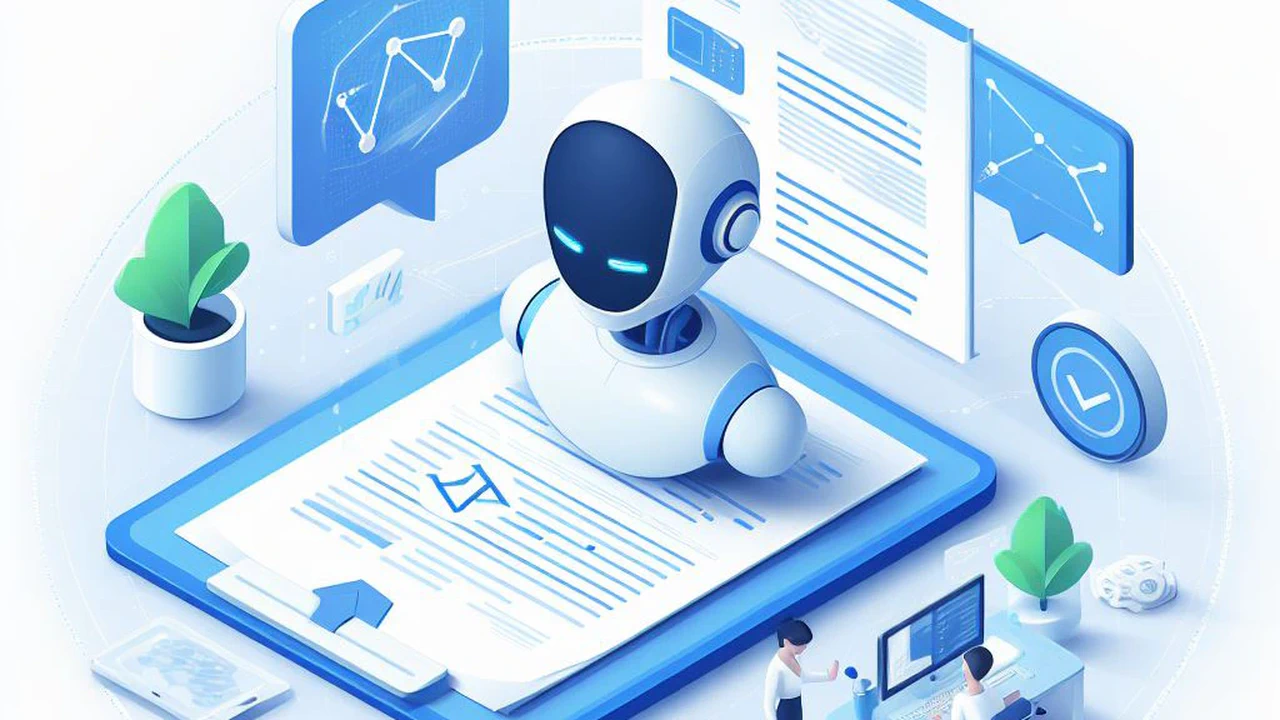
- Papers
Papers is Alpha. This content is part of an effort to make research more accessible, and (most likely) has lost some details from the original. You can find the original paper here.
Introduction
Artificial Intelligence (AI) comes with both promises and perils. AI significantly improves countless aspects of day-to-day life by performing human-like tasks with high efficiency and precision. It also brings potential risks for oppression and calamity because how AI works has not been fully understood and regulations surround its use are still lacking. Many striking stories in media (e.g., Stanford’s COVID-19 vaccine distribution algorithm) have brought Socially Responsible AI (SRAI) into the spotlight.
Substantial risks can arise when AI systems are trained to improve accuracy without knowing the underlying data generating process (DGP). First, the societal patterns hidden in the data are inevitably injected into AI algorithms. The resulting socially indifferent behaviors of AI can be further exacerbated by data heterogeneity and sparsity. Second, lacking knowledge of the DGP can cause researchers and practitioners to unconsciously make some frame of reference commitment to the formalization of AI algorithms, spanning from data and label formalization to the formalization of evaluation metrics. Third, DGP is also central to identifying the cause-effect connections and the causal relations between variables, which are two indispensable ingredients to achieve SRAI. We gain in-depth understanding of AI by intervening, interrogating, altering its environment, and finally answering “what-if” questions.
Causal inference is the key to uncovering the real-world DGPs. In the era of big data, especially, it is possible to learn causality by leveraging both causal knowledge and the copious real-world data, i.e., causal learning (CL). There have been growing interests seeking to improve AI’s social responsibility from a CL perspective, e.g., causal interpretabilityand causal-based machine learning fairness. In this survey, therefore, we first examine the seven tools in CL that are inherently related to SRAI. We then review existing efforts of connecting four of these tools to emerging tasks in SRAI, including bias mitigation, fairness, transparency , and generalizability/invariance . We conclude with open problems and challenges of leveraging CL to enhance both the functionality and social responsibility of AI.
Causal Tools for Socially Responsible AI
Based on the available causal information, we can describe CL in a three-layer hierarchy: association, intervention, and counterfactual. At the first layer, association seeks statistical relations between variables, i.e., $p(y|x)$ . By contrast, intervention and counterfactual demand causal information. An intervention is a change to the DGP. With $do$ -calculus, the interventional distribution $p(y|do(x))$ describes the distribution of $Y$ if we force $X$ to take the value $x$ while keeping the rest in the process same. This corresponds to removing all the inbound arrows to $X$ in the causal graph. At the top layer is counterfactual, denoted as $p(y_x|x',y')$ . It stands for the probability of $Y=y$ had $X$ been $x$ given what we observed were $X=x'$ and $Y=y'$ .
In the rest of this section, we review the seven tools of CL introduced inand briefly discuss how it naturally steers a course towards a SRAI-future.
- Causal Assumptions make an AI system more transparent and testable. Encoding causal assumptions explicitly allows us to discern whether these assumptions are plausible and compatible with available data. It also improves our understandings of how the system works and gives a precise framework to debate.
- Do-calculus enables the system to exclude spurious correlations by eliminating confounding through back-door criterion. Confounding is a major cause of many socially indifferent behaviors of an AI system.
- Counterfactual analysis involves a “what would have happened if” question. It is the building block of scientific thinking, thus, a key ingredient to warrant SRAI.
- Mediation Analysis decomposes the effect of an intervention into direct and indirect effects. Its identification helps understand how and why a cause-effect arises. Therefore, mediation analysis is essential for generating explanations.
- Adaptability is a model’s capability to generalize to different environments. AI algorithms typically cannot perform well when the environment changes. CL can uniquely identify the underlying mechanism responsible for the changes.
- Missing data is a common problem in AI tasks. It reduces statistical power, data representativeness, and can cause biased estimations. CL can help recover causal and statistical relationships from incomplete data via causal graphs.
- Causal discovery learns causal directionality between variables from observational data. As causal graphs are mostly unknown and arbitrarily complex in real-world applications, causal discovery offers a ladder to true causal graphs. We propose the taxonomy of CL for SRAI in Fig. fig-taxonomy and review how these CL tools – particularly, the Causal Assumptions, Do-calculus, Counterfactual Analysis, and Adaptability – help SRAI in terms of bias mitigation, fairness, transparency, and generalizability/invariance below.
Bias Mitigation
AI systems can be biased due to hidden or neglected biases in the data, algorithms, and user interaction.introduced 23 different types of bias including selection, measurement biases, and so on. There are various ways to de-bias AI systems such as adversarial training, reinforcement learning, and causal inference. Due to its interpretable nature, causal inference offers high confidence in making decisions and can show the relation between data attributes and AI system’s outcomes. Here, we review two popular causality-based methods for bias mitigation – propensity score and counterfactual data augmentation (CDA).
Propensity Score
Propensity score is used to eliminate treatment selection bias and ensure the treatment and control groups are comparable. It is the “conditional probability of assignment to a particular treatment given a vector of observed covariates”. Due to its effectiveness and simplicity, propensity score has been used to reduce unobserved biases in various domains, e.g., NLP and recommender systems. Here, we focus our discussions on recommender systems.
Inverse Propensity Scoring (IPS) is used to alleviate the selection and position biases commonly present in recommender systems. Selection bias appears when users selectively rate or click items, rendering observed ratings not representative of the true ratings, i.e., ratings obtained when users randomly rate items. Given a user-item pair $(u,i)$ and $O_{u,i}\{0,1\}$ denoting whether $u$ observed $i$ , we define propensity score as $P_{u,i}=P(O_{u,i}=1)$ , i.e., the marginal probability of observing a rating. During the model training phase, IPS-based unbiased estimator is defined using following empirical risk function:
\[ \small \argmin_{\theta} \sum_{O_{u,i}=1}\frac{\hat{\sigma}_{u,i}(r,\hat{r}(\theta))}{P_{u,i}}+Reg(\theta), \]where $\hat{\sigma}_{u,i}(r,\hat{r}(\theta))$ denotes an evaluation function and $Reg(\theta)$ the regularization for model complexity. IPS is also used to mitigate selection bias during evaluation, see, e.g.,.
Position bias occurs in a ranking system as users tend to interact with items with higher ranking positions. To remedy position bias, previous methods used IPS to weigh each data instance with a position-aware value. The loss function of such models is defined as follows: $L(M, q) = \sum_{x\in \pi_q} \Delta(x, y | \pi_q),$ where $M$ indicates the ranking model, $q \in Q$ denotes a query from a set of all queries to the model, $\pi_q$ is the ranked list by $M$ , and $\Delta(x,y|\pi_q)$ denotes the individual loss on each item $x$ with relevant label $y$ . In another method,proposed to estimate propensity scores using ranking randomization. First, the ranking results of the system are randomized. Then the propensity score is calculated based on user clicks on different positions. Although this method is shown to be effective, it can significantly degrade user experience as the highly ranked items may not be users’ favorites. Therefore,proposed a method in an offline setting where randomized experiments are not available. They specifically considered multiple types of user feedback and applied IPS to learn an unbiased ranking model.
Counterfactual Data Augmentation (CDA)
CDA is a technique to augment training data with their counterfactually-revised counterparts via causal interventions that seek to eliminate spurious correlations. It enables AI algorithms to be trained on unseen data, therefore, reducing undesired biases and improving model generalizability. Here, we focus on CDA for bias mitigation and will discuss model generalizability in Sec. 6.3.
One of the domains using CDA to reduce biases hidden in the data is NLP. Particularly, one begins by sampling a subset of original data that contains attributes of interest, e.g., gender or sentiment. Then expert annotators or inference models are asked to generate the counterfactual counterparts. The augmented datasets are later fed into the downstream NLP tasks, e.g., sentiment analysis. For example, CDA can be used to reduce gender bias by generating a dataset that encourages training algorithms not to capture gender -related information. One such method generates a gender-free list of sentences using a series of sentence templates to replace every occurrence of gendered word pairs (e.g., he:she, her:him/his). It formally defined CDA as:
Given input instances $S = \{(x_1, y_1), (x_2, y_2), ..., $ $ (x_N, y_N)\}$ and intervention $c$ , a $c$ -augmented dataset $S'$ is $S \cup \{(c(x), y)\}_{(x,y)\in S}$ .
The underlying assumption is that an unbiased model should not distinguish between matched pairs and should produce the same outcome for different genders. For sentiment analysis,generated counterfactual scenarios with help of human annotators who were provided with positive movie reviews and were asked to perform minimal changes to make them negative.
Causal Graphs
Causal graph is a powerful approach for counterfactual reasoning as it allows us to study the effects of various sensitive attributes on the outcome. It is a probabilistic graphical model to encode assumptions and widely used to detect and mitigate bias in AI systems. In the NLP domain, for example, to mitigate gender bias in word embeddings, a recent work byproposed a causal graph characterizing relation between gender-definition and gender-biased non-gender-definition word vectors. In the domain of recommender systems, causal graph can mitigate bias that affects decisions toward popular items (i.e., popularity bias) or gender-specific recommendations. The underlying assumption is that, a user click on biased recommendation results from two independent causes: user interest and item popularity. Or formally, $P_{\text{click}} = P_{\text{user interest}} + P_{\text{item popularity}},$ where $P$ indicates matching probability for a user and an item. A corresponding causal graph that disentangles user interest and item popularity is shown in Fig. fig-causal-graph-zheng. They further created two different embeddings to capture users’ real-interest in items and pseudo-interest caused by popularity. Finally, the user interest embedding is used to create a de-biased recommender where the popularity bias has been disentangled.
Discussions
Bias is a primary reason that AI systems fail to make fair decisions. Using propensity-score-based approaches needs to specify the correct forms of propensity scores in real-world applications. The alternate randomization experiments – which may be inapplicable due to ethical and financial considerations – might decrease the utility performance of the AI systems. One challenge of using CDA is to design a process to generate a modified dataset using the considered interventions. While causal graphs appear promising, one has to make assumptions that may be impractical to reflect real-world biases. Beyond bias mitigation, measuring bias via experimentation can help understand causal connections between attributes of interest and algorithmic performance, therefore, mitigating biases.
Fairness
Biases in AI systems can lead to many undesired consequences such as model overfitting and other societal issues. One of the most frequently discussed issues in AI is fairness, the property of a model that produces results independent of given variables, especially those considered sensitive, e.g., gender. Here, we briefly review another line of research that aims to train a fair AI system apart from the de-biasing perspective discussed above. A comprehensive survey on causal fairness can be referred to.
Fairness via Causal Modeling
From the causal perspective, fairness can be formulated as estimating causal effects of sensitive attributes such as gender on the outcome of an AI system. Such causal effects are evaluated using counterfactual interventions over a causal graph with features, sensitive attributes, and other variables. Underpinning this approach is the concept of counterfactual fairness (CF). CF implies that a decision is considered fair if it is the same in both “the actual world” and “a counterfactual world” where, e.g., for an individual belongs to a different demographic group. Formally, considering $Y$ , $A$ , and $X$ as the observed outcome, sensitive attributes, and features, respectively, CF is defined as follows:
Given $x\in X$ and $a \in A$ , predictor $\hat{Y}$ is counterfactually fair if
\[ \small \begin{aligned} &P(\hat{Y}_{A\leftarrow a}(U)=y|X=x, A=a) \\&=P(\hat{Y}_{A\leftarrow a'}(U)=y|X=x, A=a) \end{aligned} \]holds for all $y$ and any $a' \in A$ .
$U$ refers to a set of latent background variables in a causal graph. This definition states that if the two outcome probabilities $P(y_{a}|x, a)$ and $P(y_{a'}|x, a)$ are equal for an individual, then s/he is treated fairly as if s/he had been from another sensitive group. Or, $A$ should not be the cause of $\hat{Y}$ . CF assumed that fairness can be uniquely quantified from observational data, which is not valid in certain situations due to the unidentifiability of the counterfactual quantity.then introduced a graphical criterion determining the identifiability of counterfactual quantities. With the derived bounds, CF is achieved by constraining the classifier’s training process on the amount of unfairness in the predictor.
Another stepping stone toward creating fair AI systems using CL is the intervention-based fairness.first proposed to improve fairness by expressing direct and indirect discrimination through the different paths connecting the sensitive attributes (i.e., the treatment) and the outcome of an AI system. Similarly,modeled discrimination based on the effect of a sensitive attribute on an outcome along certain disallowed causal paths. These methods generally formulate and quantify fairness as the average causal effect of the sensitive attribute on the decision attribute, which is then evaluated by the intervention through the post-interventional distributions.
Discussions
CF considers more general situations than intervention-based fairness where the set of profile attributes is empty, therefore, it is more challenging. One limitation of CF is that these sensitive attributes may not admit counterfactual manipulation. What does it mean to suppose a different version of an individual with the counterfactually manipulated sensitive attributes such as race? In order to use a sensitive attribute appropriately, it is necessary to specify what the categories are and what perception of an attribute to be used. The validity of counterfactuals is also challenging to assess. What is the metric used to measure the similarity between the actual and imaginary worlds? CF-based models may fail when the identifiable assumption is violated due to the unidentifiable counterfactual quantity. Although this might be solved via a relaxed assumption, the idea of using social categories to achieve fairness can be problematic as they may not admit counterfactual manipulation. Lastly, parallel to algorithmic fairness, algorithmic recourse offers explanations and recommendations to individuals unfavourably treated. Future research can consider causal algorithmic recourse and its relation to CF and other fairness criteria.
Transparency
Conventional AI algorithms often lack transparency , typically presented in the concept of interpretability/explanability . When AI algorithms do not provide explanations for how and why they make a decision, users’ trust on these algorithms can be eroded. Hence, there is a need for AI systems to produce interpretable results. Causal Interpretability helps generate human friendly explanations by answering questions such as “Why does a model makes such decisions?”. In this section, we describe two approaches associated with causal interventional interpretability and counterfactual interpretability, respectively. Please refer tofor more details.
Model-based Interpretations
CL for model-based interpretations seeks to estimate the causal effect of a particular input neuron on a certain output neuron in the network. Drawing from causal inference theories, causally interpretable models first map a neural network structure into a Structural Causal Model (SCM)and then estimate the effect of each model component on the output based on the data and a learned function (i.e., a neural network) using $do$ -calculus. Particularly, every $l$ -layer neural network $N(l_1,l_2,...,l_n)$ has a corresponding SCM $M([l_1,...,l_n],U,[f_1,...,f_n],P_U)$ where $f_i$ refers to the set of causal functions for neurons in layer $l_i$ . $U$ denotes a group of exogenous random variables that act as causal factors for input layer $l_1$ . $P_u$ defines the probability distribution of $U$ . $M$ can be further reduced to a SCM with only input layer $l_1$ and output layer $l_n$ : $M'([l_1,l_n],U,f',P_U)$ , by marginalizing out the hidden neurons. Finally, we can estimate the average causal effect (ACE) of a feature $x_{i} \in l_1$ with value $\alpha$ on output $y\in l_n$ by
\[ ACE_{do\left(x_{i}=\alpha\right)}^{y}=\mathbb{E}\left[y \mid do\left(x_{i}=\alpha\right)\right]-baseline_{x_{i}}, \]where $baseline_{x_{i}} = \mathbb{E}_{x_{i}}\left[\mathbb { E } _ { y } \left[y \mid do\left(x_{i}=\right.\right.\right. \alpha)]]$ . Similar method was applied to CNN architectures trained on image data to reason over deep learning models.leveraged partial dependence plotand Individual Conditional Expectationto extract causal information (e.g., relations between input and output variables) from black-box models.proposed an approach for explaining the predictions of a visual model with the causal relations between the latent factors which they leveraged to build a Counterfactual Image Generator.
Counterfactual Explanations
Different from model-based interpretation which deals with model parameters to determine the vital components of the model, counterfactual explanations typically describe scenarios such as “If X had not occurred, Y would not have occurred”. Specifically, the predicted outcome is considered as the event $Y$ and the features fed to the model are the causes $X$ . A counterfactual explanation can be defined as a causal situation of the form where an output $Y$ , which occurs given the feature input $X$ , can be changed to a predefined output $Y'$ by minimally changing the feature vector $X$ to $X'$ .
To generate counterfactual explanations, a common approach is a generative counterfactual frameworkthat leverages generative models along with attribute editing mechanisms. The objective function is defined as
\[ \small \begin{array}{l} \underset{x_{c f}}{\arg \min } \max _{\lambda} L\left(x, x_{c f}, y, y_{c f}\right) \\ L\left(x, x_{c f}, y, y_{c f}\right)=\lambda \cdot\left(\hat{f}\left(x_{c f}\right)-y_{c f}\right)^{2}+d\left(x, x_{c f}\right), \end{array} \]where $x$ /$x_{cf}$ denotes the observed/counterfactual features and $y$ /$y_{cf}$ the observed/counterfactual outcome. The first term indicates the distance between the model’s prediction for the counterfactual input $x_{cf}$ and the desired counterfactual output. The second term describes the distance between the observed features $x$ and counterfactual features $x_{cf}$ . $\lambda$ is the hyperparameter balancing the importance of the two distances. Another category of approach relies on adversarial examples to provide counterfactual explanations. Rather than explaining why a model predicts an output with a given input, it finds an alternative version of the input that receives different classification results. One can also lay constraints on the features so that only the desired features are subject to changes. The third category of approach uses class prototypes for the counterfactual search process. These prototypes – refer to the mean encoding of the instances that belong to the class – are integrated into the objective function so that the perturbations can produce interpretable counterfactuals. For a more detailed understanding readers could refer to.
Discussions
There are still limitations in existing models for causal interepretability. For instance, evaluating such models is difficult due to the lack of ground-truth causal relations between the components of the model or causal effect of one component on another. While using counterfactual explanations for interpretability may seem feasible, it has been shown that there exist unspecified contextual presumptions and choices while generating counterfactual scenarios that may not stand well in the social world. For instance, social categories such as race and gender need to be first defined in order to generate counterfactual scenarios around these variables. Even with ground truth and well-defined social categories, existing works may still fail because causal assumptions in these works are not explicitly explained. It is critical to clearly define causal assumptions.
Invariance/Generalizability
Due to societal biases hidden in data and the shortcut learning, AI algorithms can easily overfit to training data, i.e., learning spurious correlations . Common approaches for avoiding overfitting rely on the assumption that samples of the entire population are i.i.d., which is rarely satisfied in practice. Violating the i.i.d. assumption leads to poor generalizability of an AI algorithm. Because whether a training and a testing DGP (or environment) differ is unknown, we have recourse to data heterogeneity and a model that is robust to distributional shifts among heterogeneous environments, or the invariance property. Causal relations are, by their nature, invariant. Environment is defined by intervention, therefore, in a causal graph, only direct causal relations remain invariant when an intervention changes the environment. We first examine two popular approaches that incorporate the invariance property into predictions.
Invariant Causal Prediction (ICP)
Built upon SCM, ICPaims to discover the causal parents (direct causes) of a given variable directly pointing to the target variable without constructing the entire causal graph. We consider the setting where multiple environments $e\in \mathcal{E}$ exist and in each environment $e$ , there is a predictor variable $X^e\in\mathbb{R}^p$ and a target variable $Y^e\in\mathbb{R}$ . Given a set $S\subseteq\{1,...,p\}$ , a vector $X_{S}$ containing all variables $X_k, k\in S$ , ICP assumes Invariant Prediction: [Invariant Prediction.] There is a vector of coefficients $\gamma^*=(\gamma_1^*,...,\gamma_p^*)^\intercal$ with support $S^*:=\{k:\gamma_k^*\neq0\}\subseteq\{1,...,p\}$ such that for all $e\in\mathcal{E}$ and $X^e$ with an arbitrary distribution:
\[ \small Y^e=\mu+X^e\gamma^*+\epsilon^e, \quad \epsilon^e\sim F_{\epsilon}, \epsilon^e\indep X_{S^*}^e, \]where $\mu$ is the intercept and $\epsilon^e$ denotes the random noise with the same distribution $F_{\epsilon}$ across all $e\in \mathcal{E}$ .
With multiple environments, ICP then fits a linear (Gaussian) regression in each environment. The goal is to find a set of features that results in invariant predictions between environments. In particular, ICP iterates over subsets of features combinatorially and looks for features in a model that are invariant across environments, i.e., invariant coefficients or residuals. The intersection of these sets of features is then a subset of the true direct causes. ICP also relies on the unconfoundedness assumption: no unobserved confounders exist between input features and the target. In practice, it is common to choose an observed variable to be the environment variable (e.g., background color of an image), when it could plausibly be so. Limited to using linear models and discrete variable for environment separation,extended ICP to a non-linear setting.
Invariant Risk Minimization (IRM)
Causal graphs are, in many cases, inaccessible, e.g., the causal relations between pixels and a target predict. Without the need to retrieve direct causes of a target variable in a causal graph, IRMelevates invariance by focusing on out-of-distribution (OOD) generalization – the performance of a predictive model when evaluated in a new environment. IRM seeks to learn a data representation $\phi$ that achieves two goals: predicting accurately and eliciting an invariant predictor across environments. This can be formulated as the constrained optimization problem:
\[ \small \min_{\phi:\mathcal{X}\rightarrow\mathcal{Y}}\sum_{e\in\mathcal{E}}R^e(\phi)+\lambda\cdot\|\bigtriangledown_{w|w=1.0}R^e(w\cdot\phi)\|^2, \]where $\phi$ is the invariant predictor in a latent causal system generating observed features, $w=1.0$ is a fixed “dummy” classifier. $R^e(\cdot)$ denotes the risk under $e$ such as prediction errors. $\lambda$ controls the balance between prediction accuracy in each $e$ and the invariance of the predictor $1\cdot\phi(x)$ . In practice, the prediction performance in the defined environments is almost certainly reduced due to the exclusion of some spurious correlations. IRM cannot guarantee to remove all spurious correlations as it also depends on the provided environments. There are a number of follow-up works such asrelying on a stronger assumption of invariance of $p(y|\phi(x))$ than that of $\mathbb{E}[y|\phi(x)]$ in IRM.
CDA for Invariance/Generalizability
Another causality-inspired approach for improving model invariance is to augment original data with counterfactuals that can expose the model to OOD scenarios. CDA prevents models from learning spurious patterns present in the training data, thus, improving model invariance. A general pipeline describing CDA for model invariance can be seen in Fig. fig-my-label. In computer vision, CDA is used to generate counterfactual images close to training samples yet may not belong to existing training categories. For example, to detect unknown classes, generative adversarial networks were used to generate perturbed examples from the known class and labeled as unknown categories. Drawing on independent mechanisms (IMs),proposed to decompose the image generation process into different mechanisms related to its shape, texture, and background. With known causal structure and learned IMs, a counterfactual generative network generated counterfactual images regarding each mechanism. Similar concept was used in visual question answeringto improve the generalizability of various multimodal and unimodal vision and language tasks.
Inherently related to causal inference, Reinforcement Learning (RL) uses CDA to learn more generalizable policies. Counterfactual data in RL introduces various scenarios that an RL agent generally does not experience during training. In dynamic processes, for instance,proposed to decompose the dynamics of different subprocesses into local IMs which can be used to generate counterfactual experiences. To choose the optimal treatment for a given patient,proposed a data-efficient RL algorithm that used SCM to generate counterfactual-based data.
Discussions
Current applications of IRM have been focused on computer vision, nevertheless, an environment needs not to be scenery in an image. Some promising applications include health care, robotics, NLP, recommender systems, and so on. IRM also highly relates to fairness. When applying IRM, one may pay attention to the non-linear settings where formal results for latent-variable models are lacking and risks are under-explored. Another caveat of existing works in invariant prediction is the reliance on the stringent unconfoundedness assumption, which is typically impractical. ICP is more interpretable than IRM in terms of discovering causal features. For CDA, the counterfactual samples generation strategy usually relieves the conditional independence assumption of training data, which helps improve model generalizability. When generating counterfactual data is not feasible, one can use minimally-different examples in existing datasets with different labels to improve model generalizability.
Summary and Open Problems
We review recent advances in SRAI from CL perspective. Purely reliant on statistical relationships, current AI algorithms achieve prominent performance meanwhile its potential risks raise great concerns. To achieve SRAI, we argue that CL is an effective means for it seeks to uncover the DGPs. Our survey begins by introducing the seven CL tools and their connections to SRAI. We then discuss how four of these tools are used in developing SRAI. In the following, we briefly describe promising future research directions of SRAI.
Privacy-preserving. Privacy is a crucial tenet of SRAI. Many research has shown that AI systems can learn and remember users’ private attributes. However, how to use CL to enhance privacy has been barely studied in literature. Similar to de-biasing methods, we can use CL to remove sensitive information and create privacy-preserving data representations.
Making explicit causal assumptions. Explicitly making assumptions ensures more valid, testable, and transparent causal models. Given causal assumptions might be disputed or uncertain, we need sensitivity analysis to measure the model performance with assumption violations. Critically, assumptions should be made with humility and researchers are responsible to protect against unethical assumptions.
Causal discovery. While causal discovery has been extensively studied, its connection to SRAI is not well understood. Discovering causal relations helps determine if assumptions are properly made and interventions are correctly applied. Given that causal graph is key to many CL approaches in SRAI, causal discovery is an important future research.
Mediation analysis. Causal mediation analysis improves model transparency. For example, in CF, sensitive attributes such as gender and race are assumed to solely have direct influence on the classification. Is the effect of race on loan granting mediated by the job type? Similarly, mediation analysis could be used in explainable AI, e.g., neurons directly or indirectly influence algorithmic decisions.
Missing data. CL is a missing data problem: inferring the potential outcomes of the same units with different treatment assignments. We might apply CL to a more general setting of missing data. For example, graphical model based procedures can be used to provide performance guarantees when data are Missing Not At Random.
Long-term impact. The majority of works in SRAI overlooks its long-term commitment to be fulfilled. This hinders both the efficiency and efficacy of existing works to achieve SRAI. For instance, static fairness criterion used in bank loan granting may cost credibility scores of the minorities in the long run.
Social good. Essentially, SRAI is designed to protect, inform users, and prevent/mitigate the harms of AI. With the burgeoning AI-for-social-good movement, CL is becoming the core component of AI systems to tackle societal issues.
Causal tools and libraries for SRAI. SRAI research can also benefit from using existing CL libraries such as Causal ML[https://github.com/uber/causalml], DoWhy[https://microsoft.github.io/dowhy], and Causal Discovery Toolbox[https://fentechsolutions.github.io/CausalDiscoveryToolbox/html]. It is possible to integrate CL models for SRAI into these tools.
Acknowledgements
This material is based upon work supported by, or in part by, the U.S. Army Research Laboratory and the U.S. Army Research Office under contract/grant number W911NF2110030 and W911NF2020124 as well as by the National Science Foundation (NSF) grant 1909555.
Bibliography
1@article{hawkins2004problem,
2 publisher = {ACS Publications},
3 year = {2004},
4 pages = {1--12},
5 number = {1},
6 volume = {44},
7 journal = {J Chem Inform Comput Sci},
8 author = {Hawkins, Douglas M},
9 title = {The problem of overfitting},
10}
11
12@misc{cloudera2020causality,
13 note = {Accessed: 2021-02-14},
14 howpublished = {\url{https://ff13.fastforwardlabs.com/}},
15 title = {Causality for Machine Learning},
16 year = {2020},
17 author = {Cloudera},
18}
19
20@inproceedings{swinger2019biases,
21 year = {2019},
22 pages = {305--311},
23 booktitle = {AIES},
24 author = {Swinger, Nathaniel and De-Arteaga, Maria and Heffernan IV, Neil Thomas and Leiserson, Mark DM and Kalai, Adam Tauman},
25 title = {What are the biases in my word embedding?},
26}
27
28@article{makhlouf2020survey,
29 year = {2020},
30 journal = {arXiv preprint arXiv:2010.09553},
31 author = {Makhlouf, Karima and Zhioua, Sami and Palamidessi, Catuscia},
32 title = {Survey on Causal-based Machine Learning Fairness Notions},
33}
34
35@inproceedings{balakrishnan2020towards,
36 organization = {Springer},
37 year = {2020},
38 pages = {547--563},
39 booktitle = {ECCV},
40 author = {Balakrishnan, Guha and Xiong, Yuanjun and Xia, Wei and Perona, Pietro},
41 title = {Towards causal benchmarking of bias in face analysis algorithms},
42}
43
44@inproceedings{beigi2020privacy,
45 year = {2020},
46 pages = {34--42},
47 booktitle = {WSDM},
48 author = {Beigi, Ghazaleh and Mosallanezhad, Ahmadreza and Guo, Ruocheng and Alvari, Hamidreza and Nou, Alexander and Liu, Huan},
49 title = {Privacy-aware recommendation with private-attribute protection using adversarial learning},
50}
51
52@inproceedings{agarwal2019general,
53 year = {2019},
54 booktitle = {SIGIR},
55 author = {Agarwal, Aman and Takatsu, Kenta and Zaitsev, Ivan and Joachims, Thorsten},
56 title = {A general framework for counterfactual learning-to-rank},
57}
58
59@incollection{lu2020gender,
60 publisher = {Springer},
61 year = {2020},
62 pages = {189--202},
63 booktitle = {Logic, Language, and Security},
64 author = {Lu, Kaiji and Mardziel, Piotr and Wu, Fangjing and Amancharla, Preetam and Datta, Anupam},
65 title = {Gender bias in neural natural language processing},
66}
67
68@inproceedings{ai2018unbiased,
69 year = {2018},
70 pages = {385--394},
71 booktitle = {SIGIR},
72 author = {Ai, Qingyao and Bi, Keping and Luo, Cheng and Guo, Jiafeng and Croft, W Bruce},
73 title = {Unbiased learning to rank with unbiased propensity estimation},
74}
75
76@article{hajian2012methodology,
77 publisher = {IEEE},
78 year = {2012},
79 pages = {1445--1459},
80 number = {7},
81 volume = {25},
82 journal = {TKDE},
83 author = {Hajian, Sara and Domingo-Ferrer, Josep},
84 title = {A methodology for direct and indirect discrimination prevention in data mining},
85}
86
87@inproceedings{zmigrod2019counterfactual,
88 year = {2019},
89 booktitle = {ACL},
90 author = {Zmigrod, Ran and Mielke, Sabrina J and Wallach, Hanna and Cotterell, Ryan},
91 title = {Counterfactual data augmentation for mitigating gender stereotypes in languages with rich morphology},
92}
93
94@inproceedings{guo2020debiasing,
95 year = {2020},
96 booktitle = {KDD},
97 author = {Guo, Ruocheng and Zhao, Xiaoting and Henderson, Adam and Hong, Liangjie and Liu, Huan},
98 title = {Debiasing grid-based product search in e-commerce},
99}
100
101@inproceedings{yang2018unbiased,
102 year = {2018},
103 booktitle = {RecSys},
104 author = {Yang, Longqi and Cui, Yin and Xuan, Yuan and Wang, Chenyang and Belongie, Serge and Estrin, Deborah},
105 title = {Unbiased offline recommender evaluation for missing-not-at-random implicit feedback},
106}
107
108@inproceedings{joachims2017unbiased,
109 year = {2017},
110 pages = {781--789},
111 booktitle = {WSDM},
112 author = {Joachims, Thorsten and Swaminathan, Adith and Schnabel, Tobias},
113 title = {Unbiased learning-to-rank with biased feedback},
114}
115
116@inproceedings{hofmann2013reusing,
117 year = {2013},
118 pages = {183--192},
119 booktitle = {WSDM},
120 author = {Hofmann, Katja and Schuth, Anne and Whiteson, Shimon and De Rijke, Maarten},
121 title = {Reusing historical interaction data for faster online learning to rank for IR},
122}
123
124@article{glass2013causal,
125 publisher = {Annual Reviews},
126 year = {2013},
127 pages = {61--75},
128 volume = {34},
129 journal = {Annual review of public health},
130 author = {Glass, Thomas A and Goodman, Steven N and Hern{\'a}n, Miguel A and Samet, Jonathan M},
131 title = {Causal inference in public health},
132}
133
134@article{olteanu2019social,
135 publisher = {Frontiers},
136 year = {2019},
137 pages = {13},
138 volume = {2},
139 journal = {Frontiers in Big Data},
140 author = {Olteanu, Alexandra and Castillo, Carlos and Diaz, Fernando and K{\i}c{\i}man, Emre},
141 title = {Social data: Biases, methodological pitfalls, and ethical boundaries},
142}
143
144@inproceedings{huang2019reducing,
145 year = {2020},
146 booktitle = {EMNLP},
147 author = {Huang, Po-Sen and Zhang, Huan and Jiang, Ray and Stanforth, Robert and Welbl, Johannes and Rae, Jack and Maini, Vishal and Yogatama, Dani and Kohli, Pushmeet},
148 title = {Reducing sentiment bias in language models via counterfactual evaluation},
149}
150
151@article{qureshi2019causal,
152 publisher = {Springer},
153 year = {2019},
154 pages = {1--13},
155 journal = {JIIS},
156 author = {Qureshi, Bilal and Kamiran, Faisal and Karim, Asim and Ruggieri, Salvatore and Pedreschi, Dino},
157 title = {Causal inference for social discrimination reasoning},
158}
159
160@article{von2020fairness,
161 year = {2020},
162 journal = {arXiv preprint arXiv:2010.06529},
163 author = {von K{\"u}gelgen, Julius and Bhatt, Umang and Karimi, Amir-Hossein and Valera, Isabel and Weller, Adrian and Sch{\"o}lkopf, Bernhard},
164 title = {On the fairness of causal algorithmic recourse},
165}
166
167@inproceedings{peters2018deep,
168 year = {2018},
169 booktitle = {NAACL},
170 author = {Peters, Matthew E and Neumann, Mark and Iyyer, Mohit and Gardner, Matt and Clark, Christopher and Lee, Kenton and Zettlemoyer, Luke},
171 title = {Deep contextualized word representations},
172}
173
174@inproceedings{wang2020mitigating,
175 year = {2020},
176 pages = {9322--9331},
177 booktitle = {CVPR},
178 author = {Wang, Mei and Deng, Weihong},
179 title = {Mitigating bias in face recognition using skewness-aware reinforcement learning},
180}
181
182@inproceedings{zhang2018mitigating,
183 year = {2018},
184 pages = {335--340},
185 booktitle = {AIES},
186 author = {Zhang, Brian Hu and Lemoine, Blake and Mitchell, Margaret},
187 title = {Mitigating unwanted biases with adversarial learning},
188}
189
190@book{imbens2015causal,
191 publisher = {Cambridge University Press},
192 year = {2015},
193 author = {Imbens, Guido W and Rubin, Donald B},
194 title = {Causal inference in statistics, social, and biomedical sciences},
195}
196
197@article{mehrabi2019survey,
198 year = {2019},
199 journal = {arXiv preprint arXiv:1908.09635},
200 author = {Mehrabi, Ninareh and Morstatter, Fred and Saxena, Nripsuta and Lerman, Kristina and Galstyan, Aram},
201 title = {A survey on bias and fairness in machine learning},
202}
203
204@inproceedings{corbett2017algorithmic,
205 year = {2017},
206 pages = {797--806},
207 booktitle = {KDD},
208 author = {Corbett-Davies, Sam and Pierson, Emma and Feller, Avi and Goel, Sharad and Huq, Aziz},
209 title = {Algorithmic decision making and the cost of fairness},
210}
211
212@book{o2016weapons,
213 publisher = {Crown},
214 year = {2016},
215 author = {O'neil, Cathy},
216 title = {Weapons of math destruction: How big data increases inequality and threatens democracy},
217}
218
219@inproceedings{mosallanezhad2019deep,
220 year = {2019},
221 pages = {2360--2369},
222 booktitle = {EMNLP-IJCNLP},
223 author = {Mosallanezhad, Ahmadreza and Beigi, Ghazaleh and Liu, Huan},
224 title = {Deep reinforcement learning-based text anonymization against private-attribute inference},
225}
226
227@article{mu2018survey,
228 publisher = {IEEE},
229 year = {2018},
230 pages = {69009--69022},
231 volume = {6},
232 journal = {Ieee Access},
233 author = {Mu, Ruihui},
234 title = {A survey of recommender systems based on deep learning},
235}
236
237@article{bareinboim2016causal,
238 publisher = {National Acad Sciences},
239 year = {2016},
240 pages = {7345--7352},
241 number = {27},
242 volume = {113},
243 journal = {PNAS},
244 author = {Bareinboim, Elias and Pearl, Judea},
245 title = {Causal inference and the data-fusion problem},
246}
247
248@article{shiffrin2016drawing,
249 publisher = {National Acad Sciences},
250 year = {2016},
251 pages = {7308--7309},
252 number = {27},
253 volume = {113},
254 journal = {PNAS},
255 author = {Shiffrin, Richard M},
256 title = {Drawing causal inference from big data},
257}
258
259@inproceedings{mosallanezhad2019deep,
260 year = {2019},
261 pages = {2360--2369},
262 booktitle = {EMNLP-IJCNLP},
263 author = {Mosallanezhad, Ahmadreza and Beigi, Ghazaleh and Liu, Huan},
264 title = {Deep reinforcement learning-based text anonymization against private-attribute inference},
265}
266
267@article{zheng2020disentangling,
268 year = {2021},
269 journal = {WWW},
270 author = {Zheng, Yu and Gao, Chen and Li, Xiang and He, Xiangnan and Jin, Depeng and Li, Yong},
271 title = {Disentangling User Interest and Conformity for Recommendation with Causal Embedding},
272}
273
274@inproceedings{kasirzadeh2021use,
275 year = {2021},
276 booktitle = {FAccT},
277 author = {Kasirzadeh, Atoosa and Smart, Andrew},
278 title = {The Use and Misuse of Counterfactuals in Ethical Machine Learning},
279}
280
281@inproceedings{yang2020causal,
282 year = {2020},
283 pages = {9434--9441},
284 booktitle = {AAAI},
285 author = {Yang, Zekun and Feng, Juan},
286 title = {A causal inference method for reducing gender bias in word embedding relations},
287}
288
289@inproceedings{zhao2019gender,
290 year = {2019},
291 booktitle = {NAACL},
292 author = {Zhao, Jieyu and Wang, Tianlu and Yatskar, Mark and Cotterell, Ryan and Ordonez, Vicente and Chang, Kai-Wei},
293 title = {Gender bias in contextualized word embeddings},
294}
295
296@misc{neuberg2003causality,
297 publisher = {JSTOR},
298 year = {2003},
299 author = {Neuberg, Leland Gerson},
300 title = {Causality: Models, Reasoning, and Inference},
301}
302
303@article{kusner2017counterfactual,
304 year = {2017},
305 journal = {NeurIPS},
306 author = {Kusner, Matt J and Loftus, Joshua R and Russell, Chris and Silva, Ricardo},
307 title = {Counterfactual fairness},
308}
309
310@inproceedings{zhang2018fairness,
311 year = {2018},
312 number = {1},
313 volume = {32},
314 booktitle = {AAAI},
315 author = {Zhang, Junzhe and Bareinboim, Elias},
316 title = {Fairness in decision-making—the causal explanation formula},
317}
318
319@inproceedings{wu2019counterfactual,
320 year = {2019},
321 booktitle = {IJCAI},
322 author = {Wu, Yongkai and Zhang, Lu and Wu, Xintao},
323 title = {Counterfactual fairness: Unidentification, bound and algorithm},
324}
325
326@inproceedings{nabi2018fair,
327 year = {2018},
328 number = {1},
329 volume = {32},
330 booktitle = {AAAI},
331 author = {Nabi, Razieh and Shpitser, Ilya},
332 title = {Fair inference on outcomes},
333}
334
335@inproceedings{choe2020empirical,
336 year = {2020},
337 booktitle = {ICML UDL},
338 author = {Choe, Yo Joong and Ham, Jiyeon and Park, Kyubyong},
339 title = {An empirical study of invariant risk minimization},
340}
341
342@article{wang2018deconfounded,
343 year = {2018},
344 journal = {arXiv preprint arXiv:1808.06581},
345 author = {Wang, Yixin and Liang, Dawen and Charlin, Laurent and Blei, David M},
346 title = {The deconfounded recommender: A causal inference approach to recommendation},
347}
348
349@article{giusti2015machine,
350 publisher = {IEEE},
351 year = {2015},
352 pages = {661--667},
353 number = {2},
354 volume = {1},
355 journal = {IEEE Robotics and Automation Letters},
356 author = {Giusti, Alessandro and Guzzi, J{\'e}r{\^o}me and Cire{\c{s}}an, Dan C and He, Fang-Lin and Rodr{\'\i}guez, Juan P and Fontana, Flavio and Faessler, Matthias and Forster, Christian and Schmidhuber, J{\"u}rgen and Di Caro, Gianni and others},
357 title = {A machine learning approach to visual perception of forest trails for mobile robots},
358}
359
360@article{krueger2020out,
361 year = {2020},
362 journal = {arXiv preprint arXiv:2003.00688},
363 author = {Krueger, David and Caballero, Ethan and Jacobsen, Joern-Henrik and Zhang, Amy and Binas, Jonathan and Priol, Remi Le and Courville, Aaron},
364 title = {Out-of-distribution generalization via risk extrapolation (rex)},
365}
366
367@article{pearl2019seven,
368 publisher = {ACM New York, NY, USA},
369 year = {2019},
370 pages = {54--60},
371 number = {3},
372 volume = {62},
373 journal = {Communications of the ACM},
374 author = {Pearl, Judea},
375 title = {The seven tools of causal inference, with reflections on machine learning},
376}
377
378@article{mohan2021graphical,
379 publisher = {Taylor \& Francis},
380 year = {2021},
381 pages = {1--42},
382 journal = {JASA},
383 author = {Mohan, Karthika and Pearl, Judea},
384 title = {Graphical models for processing missing data},
385}
386
387@article{guo2020survey,
388 publisher = {ACM New York, NY, USA},
389 year = {2020},
390 pages = {1--37},
391 number = {4},
392 volume = {53},
393 journal = {CSUR},
394 author = {Guo, Ruocheng and Cheng, Lu and Li, Jundong and Hahn, P Richard and Liu, Huan},
395 title = {A survey of learning causality with data: Problems and methods},
396}
397
398@article{moraffah2020causal,
399 publisher = {ACM New York, NY, USA},
400 year = {2020},
401 pages = {18--33},
402 number = {1},
403 volume = {22},
404 journal = {ACM SIGKDD Explorations Newsletter},
405 author = {Moraffah, Raha and Karami, Mansooreh and Guo, Ruocheng and Raglin, Adrienne and Liu, Huan},
406 title = {Causal interpretability for machine learning-problems, methods and evaluation},
407}
408
409@inproceedings{getoor2019responsible,
410 organization = {IEEE},
411 year = {2019},
412 pages = {1--1},
413 booktitle = {Big Data},
414 author = {Getoor, Lise},
415 title = {Responsible Data Science},
416}
417
418@article{cheng2021socially,
419 year = {2021},
420 journal = {arXiv preprint arXiv:2101.02032},
421 author = {Cheng, Lu and Varshney, Kush R and Liu, Huan},
422 title = {Socially Responsible AI Algorithms: Issues, Purposes, and Challenges},
423}
424
425@article{imai2010general,
426 publisher = {American Psychological Association},
427 year = {2010},
428 pages = {309},
429 number = {4},
430 volume = {15},
431 journal = {Psychological methods},
432 author = {Imai, Kosuke and Keele, Luke and Tingley, Dustin},
433 title = {A general approach to causal mediation analysis.},
434}
435
436@article{kouw2018introduction,
437 year = {2018},
438 journal = {arXiv preprint arXiv:1812.11806},
439 author = {Kouw, Wouter M and Loog, Marco},
440 title = {An introduction to domain adaptation and transfer learning},
441}
442
443@article{jin2020domain,
444 year = {2020},
445 journal = {arXiv preprint arXiv:2006.03908},
446 author = {Jin, Wengong and Barzilay, Regina and Jaakkola, Tommi},
447 title = {Domain extrapolation via regret minimization},
448}
449
450@article{peters2016causal,
451 publisher = {JSTOR},
452 year = {2016},
453 pages = {947--1012},
454 journal = {J R Stat Soc Series B Stat Methodol},
455 author = {Peters, Jonas and B{\"u}hlmann, Peter and Meinshausen, Nicolai},
456 title = {Causal inference by using invariant prediction: identification and confidence intervals},
457}
458
459@inproceedings{zmigrod2019counterfactual,
460 year = {2019},
461 booktitle = {ACL},
462 author = {Zmigrod, Ran and Mielke, Sabrina J and Wallach, Hanna and Cotterell, Ryan},
463 title = {Counterfactual data augmentation for mitigating gender stereotypes in languages with rich morphology},
464}
465
466@article{plappert2018multi,
467 year = {2018},
468 journal = {arXiv preprint arXiv:1802.09464},
469 author = {Plappert, Matthias and Andrychowicz, Marcin and Ray, Alex and McGrew, Bob and Baker, Bowen and Powell, Glenn and Schneider, Jonas and Tobin, Josh and Chociej, Maciek and Welinder, Peter and others},
470 title = {Multi-goal reinforcement learning: Challenging robotics environments and request for research},
471}
472
473@inproceedings{zhao2018gender,
474 year = {2018},
475 booktitle = {NAACL},
476 author = {Zhao, Jieyu and Wang, Tianlu and Yatskar, Mark and Ordonez, Vicente and Chang, Kai-Wei},
477 title = {Gender bias in coreference resolution: Evaluation and debiasing methods},
478}
479
480@book{spirtes2000causation,
481 publisher = {MIT press},
482 year = {2000},
483 author = {Spirtes, Peter and Glymour, Clark N and Scheines, Richard and Heckerman, David},
484 title = {Causation, prediction, and search},
485}
486
487@inproceedings{de2019bias,
488 year = {2019},
489 pages = {120--128},
490 booktitle = {ACM FAccT},
491 author = {De-Arteaga, Maria and Romanov, Alexey and Wallach, Hanna and Chayes, Jennifer and Borgs, Christian and Chouldechova, Alexandra and Geyik, Sahin and Kenthapadi, Krishnaram and Kalai, Adam Tauman},
492 title = {Bias in bios: A case study of semantic representation bias in a high-stakes setting},
493}
494
495@article{blodgett2020language,
496 year = {2020},
497 journal = {arXiv preprint arXiv:2005.14050},
498 author = {Blodgett, Su Lin and Barocas, Solon and Daum{\'e} III, Hal and Wallach, Hanna},
499 title = {Language (technology) is power: A critical survey of" bias" in nlp},
500}
501
502@inproceedings{lu2020sample,
503 year = {2020},
504 booktitle = {NeurIPS Offline RL},
505 author = {Lu, Chaochao and Huang, Biwei and Wang, Ke and Hern{\'a}ndez-Lobato, Jos{\'e} Miguel and Zhang, Kun and Sch{\"o}lkopf, Bernhard},
506 title = {Sample-Efficient Reinforcement Learning via Counterfactual-Based Data Augmentation},
507}
508
509@article{kaushik2020learning,
510 year = {2020},
511 journal = {ICLR},
512 author = {Kaushik, Divyansh and Hovy, Eduard and Lipton, Zachary C},
513 title = {Learning the difference that makes a difference with counterfactually-augmented data},
514}
515
516@inproceedings{pitis2020counterfactual,
517 year = {2020},
518 booktitle = {NeurIPS},
519 author = {Pitis, Silviu and Creager, Elliot and Garg, Animesh},
520 title = {Counterfactual Data Augmentation using Locally Factored Dynamics},
521}
522
523@article{sauer2021counterfactual,
524 year = {2021},
525 booktitle = {ICLR},
526 author = {Sauer, Axel and Geiger, Andreas},
527 title = {Counterfactual Generative Networks},
528}
529
530@inproceedings{neal2018open,
531 year = {2018},
532 booktitle = {ECCV},
533 author = {Neal, Lawrence and Olson, Matthew and Fern, Xiaoli and Wong, Weng-Keen and Li, Fuxin},
534 title = {Open set learning with counterfactual images},
535}
536
537@book{peters2017elements,
538 publisher = {The MIT Press},
539 year = {2017},
540 author = {Peters, Jonas and Janzing, Dominik and Sch{\"o}lkopf, Bernhard},
541 title = {Elements of causal inference: foundations and learning algorithms},
542}
543
544@inproceedings{abbasnejad2020counterfactual,
545 year = {2020},
546 pages = {10044--10054},
547 booktitle = {CVPR},
548 author = {Abbasnejad, Ehsan and Teney, Damien and Parvaneh, Amin and Shi, Javen and Hengel, Anton van den},
549 title = {Counterfactual vision and language learning},
550}
551
552@inproceedings{teney2020learning,
553 year = {2020},
554 booktitle = {ECCV},
555 author = {Teney, Damien and Abbasnedjad, Ehsan and Hengel, Anton van den},
556 title = {Learning what makes a difference from counterfactual examples and gradient supervision},
557}
558
559@article{rosenfeld2020risks,
560 year = {2020},
561 journal = {arXiv preprint arXiv:2010.05761},
562 author = {Rosenfeld, Elan and Ravikumar, Pradeep and Risteski, Andrej},
563 title = {The Risks of Invariant Risk Minimization},
564}
565
566@article{heinze2018invariant,
567 publisher = {De Gruyter},
568 year = {2018},
569 number = {2},
570 volume = {6},
571 journal = {JCI},
572 author = {Heinze-Deml, Christina and Peters, Jonas and Meinshausen, Nicolai},
573 title = {Invariant causal prediction for nonlinear models},
574}
575
576@inproceedings{schnabel2016recommendations,
577 year = {2016},
578 booktitle = {ICML},
579 author = {Schnabel, Tobias and Swaminathan, Adith and Singh, Ashudeep and Chandak, Navin and Joachims, Thorsten},
580 title = {Recommendations as treatments: Debiasing learning and evaluation},
581}
582
583@article{rosenbaum1983central,
584 publisher = {Oxford University Press},
585 year = {1983},
586 pages = {41--55},
587 number = {1},
588 volume = {70},
589 journal = {Biometrika},
590 author = {Rosenbaum, Paul R and Rubin, Donald B},
591 title = {The central role of the propensity score in observational studies for causal effects},
592}
593
594@article{arjovsky2019invariant,
595 year = {2019},
596 journal = {arXiv preprint arXiv:1907.02893},
597 author = {Arjovsky, Martin and Bottou, L{\'e}on and Gulrajani, Ishaan and Lopez-Paz, David},
598 title = {Invariant risk minimization},
599}
600
601@book{pearl2009causality,
602 year = {2009},
603 author = {Pearl, Judea},
604 title = {Causality},
605}
606
607@inproceedings{pearl2011transportability,
608 year = {2011},
609 number = {1},
610 volume = {25},
611 booktitle = {AAAI},
612 author = {Pearl, Judea and Bareinboim, Elias},
613 title = {Transportability of causal and statistical relations: A formal approach},
614}
615
616@inproceedings{beery2018recognition,
617 year = {2018},
618 pages = {456--473},
619 booktitle = {ECCV},
620 author = {Beery, Sara and Van Horn, Grant and Perona, Pietro},
621 title = {Recognition in terra incognita},
622}
623
624@inproceedings{schwab2019cxplain,
625 year = {2019},
626 booktitle = {NeurIPS},
627 author = {Schwab, Patrick and Karlen, Walter},
628 title = {Cxplain: Causal explanations for model interpretation under uncertainty},
629}
630
631@article{angwin2016machine,
632 year = {2016},
633 volume = {23},
634 journal = {ProPublica, May},
635 author = {Angwin, Julia and Larson, Jeff and Mattu, Surya and Kirchner, Lauren},
636 title = {Machine bias risk assessments in criminal sentencing},
637}
638
639@article{narendra2018explaining,
640 year = {2018},
641 journal = {arXiv preprint arXiv:1811.04376},
642 author = {Narendra, Tanmayee and Sankaran, Anush and Vijaykeerthy, Deepak and Mani, Senthil},
643 title = {Explaining deep learning models using causal inference},
644}
645
646@article{zhao2021causal,
647 publisher = {Taylor \& Francis},
648 year = {2021},
649 pages = {272--281},
650 number = {1},
651 volume = {39},
652 journal = {JBES},
653 author = {Zhao, Qingyuan and Hastie, Trevor},
654 title = {Causal interpretations of black-box models},
655}
656
657@article{geirhos2020shortcut,
658 publisher = {Nature Publishing Group},
659 year = {2020},
660 pages = {665--673},
661 number = {11},
662 volume = {2},
663 journal = {Nature Machine Intelligence},
664 author = {Geirhos, Robert and Jacobsen, J{\"o}rn-Henrik and Michaelis, Claudio and Zemel, Richard and Brendel, Wieland and Bethge, Matthias and Wichmann, Felix A},
665 title = {Shortcut learning in deep neural networks},
666}
667
668@inproceedings{martinez2019explaining,
669 organization = {IEEE},
670 year = {2019},
671 pages = {4167--4175},
672 booktitle = {ICCVW},
673 author = {Mart{\'\i}nez, {\'A}lvaro Parafita and Marca, Jordi Vitri{\`a}},
674 title = {Explaining Visual Models by Causal Attribution},
675}
676
677@article{friedman2001greedy,
678 publisher = {JSTOR},
679 year = {2001},
680 pages = {1189--1232},
681 journal = {Annals of statistics},
682 author = {Friedman, Jerome H},
683 title = {Greedy function approximation: a gradient boosting machine},
684}
685
686@article{goldstein2015peeking,
687 publisher = {Taylor \& Francis},
688 year = {2015},
689 pages = {44--65},
690 number = {1},
691 volume = {24},
692 journal = {JCGS},
693 author = {Goldstein, Alex and Kapelner, Adam and Bleich, Justin and Pitkin, Emil},
694 title = {Peeking inside the black box: Visualizing statistical learning with plots of individual conditional expectation},
695}
696
697@inproceedings{chattopadhyay2019neural,
698 organization = {PMLR},
699 year = {2019},
700 pages = {981--990},
701 booktitle = {ICML},
702 author = {Chattopadhyay, Aditya and Manupriya, Piyushi and Sarkar, Anirban and Balasubramanian, Vineeth N},
703 title = {Neural network attributions: A causal perspective},
704}
705
706@article{liu2019generative,
707 year = {2019},
708 journal = {arXiv preprint arXiv:1907.03077},
709 author = {Liu, Shusen and Kailkhura, Bhavya and Loveland, Donald and Han, Yong},
710 title = {Generative counterfactual introspection for explainable deep learning},
711}
712
713@inproceedings{moore2019explaining,
714 organization = {Springer},
715 year = {2019},
716 pages = {43--56},
717 booktitle = {PRICAI},
718 author = {Moore, Jonathan and Hammerla, Nils and Watkins, Chris},
719 title = {Explaining deep learning models with constrained adversarial examples},
720}
721
722@article{van2019interpretable,
723 year = {2019},
724 journal = {arXiv preprint arXiv:1907.02584},
725 author = {Van Looveren, Arnaud and Klaise, Janis},
726 title = {Interpretable counterfactual explanations guided by prototypes},
727}
728
729@article{xu2020causality,
730 year = {2020},
731 journal = {arXiv preprint arXiv:2006.16789},
732 author = {Xu, Guandong and Duong, Tri Dung and Li, Qian and Liu, Shaowu and Wang, Xianzhi},
733 title = {Causality Learning: A New Perspective for Interpretable Machine Learning},
734}
735
736@inproceedings{liu2018delayed,
737 year = {2018},
738 pages = {3150--3158},
739 booktitle = {ICML},
740 author = {Liu, Lydia T and Dean, Sarah and Rolf, Esther and Simchowitz, Max and Hardt, Moritz},
741 title = {Delayed impact of fair machine learning},
742}
743
744@article{dowhypaper,
745 year = {2020},
746 journal = {arXiv preprint arXiv:2011.04216},
747 author = {Sharma, Amit and Kiciman, Emre},
748 title = {DoWhy: An End-to-End Library for Causal Inference},
749}
750
751@misc{econml,
752 year = {2019},
753 note = {Version 0.x},
754 howpublished = {https://github.com/microsoft/EconML},
755 title = {{EconML}: {A Python Package for ML-Based Heterogeneous Treatment Effects Estimation}},
756 author = {Microsoft Research},
757}
Attribution
arXiv:2104.12278v2
[cs.AI]
License: cc-by-4.0