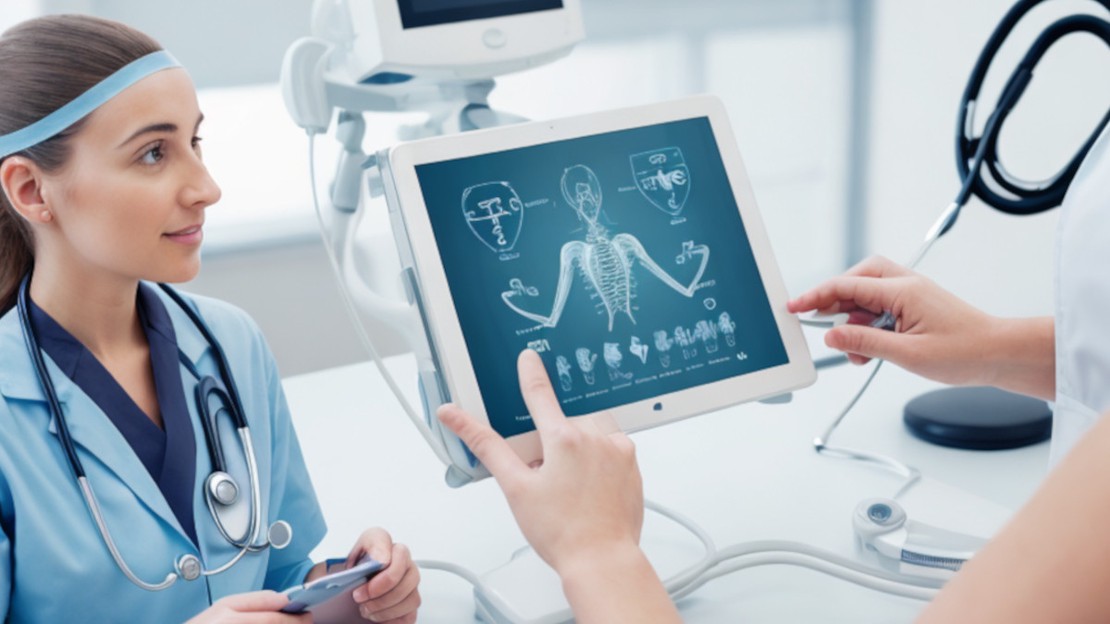
AI Healthcare & Medicine
- Justin Riddiough
- June 30, 2023
Artificial Intelligence in Healthcare & Medicine
Artificial Intelligence (AI) has been making significant strides in revolutionizing healthcare, and open source initiatives are playing a crucial role in accelerating progress. In this article, we will delve into three notable open source AI efforts: MONAI, HeartKit, and VinDr Lab. Each project brings a unique approach to the application of AI in healthcare, fostering collaboration and advancing the field.
Project MONAI: Driving Collaboration in Healthcare Imaging
One prominent open source AI effort in healthcare is Project MONAI, an inclusive community formed by NVIDIA and King’s College London. Its primary aim is to establish best practices for AI in healthcare imaging across academia and enterprise researchers. Since its inception, Project MONAI has expanded to include industry leaders and researchers in medical imaging.
MONAI offers a suite of open-source PyTorch-based frameworks that cover the entire medical AI life cycle. The MONAI Core provides a domain-specific framework for training AI models in healthcare imaging, ensuring reproducibility and easy integration. Researchers can leverage MONAI Label, an intelligent image labeling tool, to annotate new datasets efficiently. The MONAI Deploy App SDK allows developers to convert AI models into applications seamlessly. Lastly, the MONAI Model Zoo offers a collection of pre-trained medical imaging models.
HeartKit: Empowering Real-Time Heart Monitoring
HeartKit, an initiative by Ambiq.ai, focuses on enabling real-time heart-monitoring applications on battery-powered edge devices. HeartKit employs an optimized open-source TinyML model designed for efficiency, explainability, and extensibility.
The architecture of HeartKit comprises an ECG segmentation model and three upstream heads: HRV head, arrhythmia head, and beat head. The ECG segmentation model annotates each sample as P-wave, QRS, T-wave, or none. The arrhythmia head detects the presence of Atrial Fibrillation (AFIB) or Atrial Flutter (AFL). The HRV head calculates heart rate, rhythm, and heart rate variability, while the beat head identifies irregular beats.
With HeartKit, healthcare professionals can leverage edge devices to perform real-time heart monitoring, providing timely insights and improving patient care.
VinDr Lab: Building High-Quality Medical AI Algorithms
VinDr Lab, developed by the Medical Imaging team at Vingroup Big Data Institute (VinBigdata), offers a powerful data platform for medical AI. It enables the creation of high-quality datasets and algorithms with advanced annotation features and a streamlined process.
VinDr Lab provides a comprehensive set of features for managing medical data, tracking project progress, and creating and sharing label groups. It offers annotation tools such as bounding boxes, polygons, brushes, and notes, facilitating precise and detailed annotations. The platform also allows for version control of exported labels, ensuring reproducibility and traceability.
VinDr Lab empowers researchers and developers to build robust and accurate medical AI algorithms, fueling advancements in diagnosis, treatment, and research.
The Power of Open Source in Healthcare
The advent of open source AI efforts in healthcare brings numerous opportunities for collaboration, knowledge sharing, and innovation. By embracing open source frameworks like MONAI, HeartKit, and VinDr Lab, researchers and developers can leverage established best practices, high-quality software, and community support to accelerate their AI projects.
These initiatives foster an environment of collaboration, enabling researchers from academia and industry to work together, share results, and build upon each other’s work. Open source AI efforts also enhance reproducibility, transparency, and accessibility, contributing to the democratization of healthcare AI.
As open source projects continue to evolve, the healthcare community can leverage these advancements to address complex challenges, improve patient outcomes, and pave the way for a future where AI plays an integral role in healthcare delivery.
We invite you to browse some of our favorite free healthcare models.
How is AI used in Healthcare?
- Medical Imaging: AI helps analyze medical images, such as X-rays and MRIs, to detect abnormalities, aid in diagnosis, and improve accuracy.
- Drug Discovery: AI accelerates the process of discovering and developing new drugs by analyzing vast amounts of data and identifying potential candidates.
- Genomics: AI is used to analyze genomic data, identify disease markers, predict patient outcomes, and personalize treatment plans.
- Electronic Health Records (EHR): AI algorithms analyze EHR data to identify patterns, predict diseases, improve clinical decision-making, and enhance patient care.
- Virtual Assistants: AI-powered chatbots and virtual assistants help patients with appointment scheduling, medication reminders, and answering basic health-related queries.
- Precision Medicine: AI enables personalized treatment plans by analyzing patient data, genetic information, and medical literature to identify the most effective interventions.
What are the benefits of using AI in Healthcare?
- Improved Diagnosis: AI algorithms can analyze medical images and patient data with high accuracy, aiding in early detection and diagnosis of diseases.
- Enhanced Treatment Planning: AI helps identify the most effective treatment options based on individual patient characteristics, leading to personalized and targeted therapies.
- Efficient Healthcare Operations: AI automates administrative tasks, streamlines workflows, and optimizes resource allocation, resulting in improved efficiency and reduced healthcare costs.
- Real-time Monitoring: AI enables continuous monitoring of patient vital signs and health parameters, providing early warnings of deteriorating conditions and allowing timely interventions.
- Predictive Analytics: AI algorithms can analyze large datasets to predict disease outbreaks, identify at-risk populations, and optimize public health interventions.
- Research and Development: AI accelerates drug discovery, aids in genomics research, and facilitates data-driven clinical trials, leading to faster advancements in healthcare.
What are the challenges of using AI in Healthcare?
- Data Quality and Privacy: AI models require large amounts of high-quality data, and ensuring data privacy and security can be complex.
- Regulatory and Ethical Considerations: The use of AI in healthcare raises concerns around regulatory compliance, transparency, accountability, and potential biases in algorithmic decision-making.
- Integration and Adoption: Integrating AI systems into existing healthcare infrastructure and workflows can be challenging, requiring careful planning, training, and change management.
- Interpretability and Explainability: AI models often operate as black boxes, making it difficult to understand and explain the reasoning behind their predictions, which can impact trust and acceptance.
- Evaluation and Validation: Establishing robust evaluation metrics and validation processes for AI models in healthcare is crucial to ensure their safety, efficacy, and reliability.
- Workforce Impact: AI adoption may require upskilling healthcare professionals and addressing concerns about job displacement.
How does AI contribute to medical imaging?
- Image Segmentation: AI algorithms can accurately segment medical images to identify and delineate specific anatomical structures or regions of interest.
- Disease Detection: AI aids in the automated detection of abnormalities, such as tumors or lesions, in medical images, improving diagnostic accuracy and efficiency.
- Image Reconstruction: AI techniques can enhance image quality, remove noise, and reconstruct high-resolution images from low-quality or limited data, improving diagnostic capabilities.
- Image Interpretation: AI algorithms can analyze complex patterns in medical images and assist radiologists in interpreting and prioritizing cases, reducing interpretation time and potential errors.
- Workflow Optimization: AI helps automate image analysis tasks, such as organ segmentation or lesion quantification, freeing up radiologists’ time for more critical tasks.
Follow AI Models on Google News
An easy & free way to support AI Models is to follow our google news feed! More followers will help us reach a wider audience!
Google News: AI Models