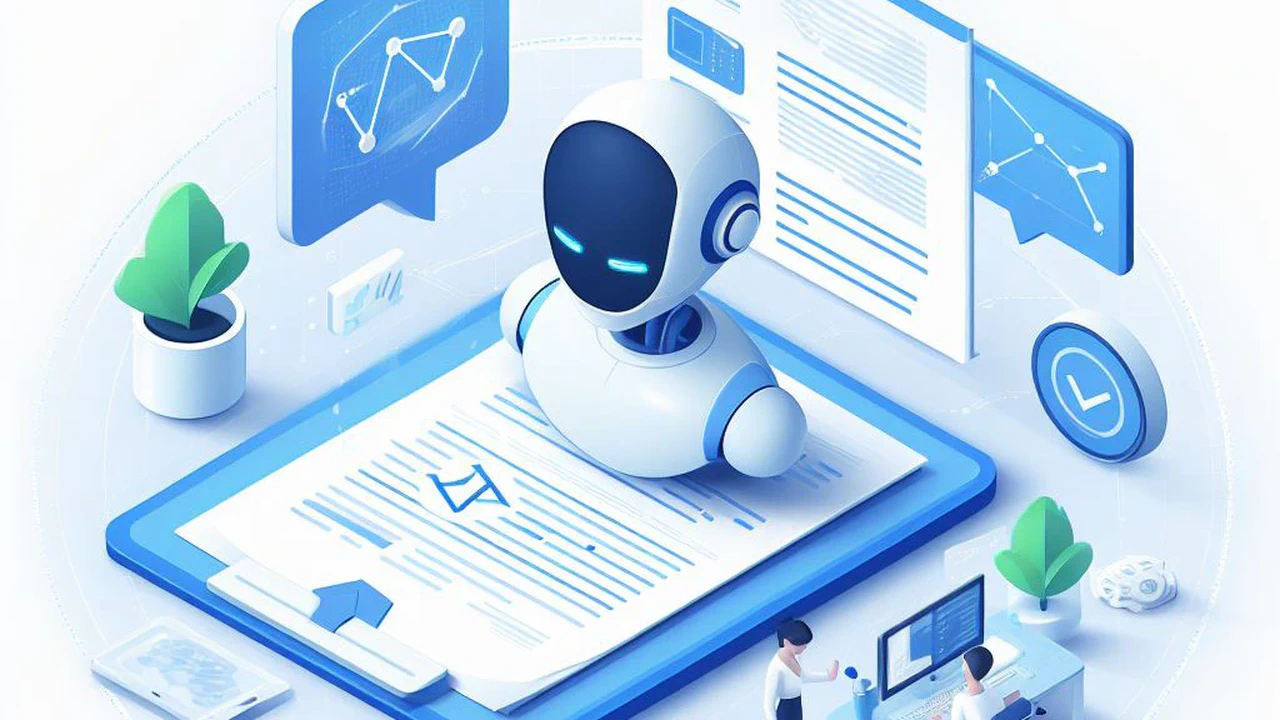
Papers is Alpha. This content is part of an effort to make research more accessible, and (most likely) has lost some details from the original. You can find the original paper here.
Introduction
Technology developers, researchers, policymakers, and others have identified the design and development process of artificial intelligence (AI) systems as a site for interventions to promote more ethical and just ends for AI systems. Recognizing this opportunity, researchers, practitioners, and activists have created a plethora of tools, resources, guides, and kits—of which the dominant paradigm is a “toolkit”—to promote ethics in AI design and development. Toolkits help technology practitioners and other stakeholders surface, discuss, or address ethical issues in their work. However, as the field appears to coalesce around this paradigm, it is critical to consider how these toolkits help to define and shape that work. Technologies that create standards (such as widely adopted toolkits), shape how people understand and interact with the worldPrior research in CSCW and related fields has advanced our understanding of the work required to implement AI ethics principles in practice. In addition, prior work in CSCW has also examined the politics of tools and other artifacts designed to support the work of pursuing values and ethics, such as security, privacy, and UX design. Previous reviews of AI ethics and fairness toolkits have primarily focused on their usability and functionalityor evaluating their efficacy in addressing ethical issues. In this paper, we contribute to these bodies of research by taking a more critical approach to understand how AI ethics toolkits, like all tools, enact values and assumptions about what it means to do the work of ethics. We start from the basis that simply creating toolkits will not be sufficient to address ethical issues. They must be adopted and used in practice within specific organizational contexts, but, as prior research has identified, adopting AI ethics tools and processes within organizational contexts presents challenges beyond usability and functionality. Therefore, by understanding how toolkits envision the work of AI ethics—particularly how those work practices may align (or not) with the organizational contexts in which they may be used—we may better identify opportunities to improve the design of toolkits and identify instances where additional processes or artifacts beyond toolkits may be useful. To investigate this, we ask:
-
What are the discourses of ethics that ethical AI toolkits draw on to legitimize their use?
-
Who do the toolkits imagine as doing the work of addressing ethics in AI?
-
What do toolkits imagine to be the specific work practices of addressing ethics in AI?
To do this, we compiled and qualitatively coded a corpus of 27 AI ethics toolkits (broadly construed) to identify the discourses about ethics, the imagined users of the toolkits, and the work practices the toolkits envision and support. We found that AI ethics toolkits largely frame the work of AI ethics as technical work for individual technical practitioners, even as those same toolkits call for engaging broader sets of stakeholders to grapple with social aspects of AI ethics. In addition, we find that toolkits do not contend with the organizational, labor, and political implications of AI ethics work in practice.In general, we found gaps between the types of stakeholders and work practices the toolkits call for and the support they provide. Despite framing ethics and fairness as sociotechnical issues that require diverse stakeholder involvement and engagement, many of the toolkits focused on technical approaches for individual technical practitioners to undertake. With few exceptions, toolkits lacked guidance on how to involve more diverse stakeholders or how to navigate organizational power dynamics when addressing AI ethics.=-1
We provide recommendations for designers of AI ethics toolkits—both future and existing—to (1) embrace the non-technical dimensions of AI ethics work; (2) support the work of engaging with stakeholders[Here, we use the term “stakeholder” expansively, to include both potential users of the toolkits, others who may be part of the AI design, development, and deployment process, as well as other direct and indirect stakeholders who may be impacted by AI systems. We take this expansive approach following Lucy Suchman’s work complicating the notion of the useras well as Forlizzi and Zimmerman’s work calling for more attention to stakeholders outside of the end users. In cases where we specifically mean the users of the toolkit, we use the term “user.”] from non-technical backgrounds; and (3) structure the work of AI ethics as a problem for collective action. We end with a discussion of how we, as a research community, can foster the design of toolkits that achieve these goals, and we grapple with how we might create metaphors and formats beyond toolkits that resist the solutionism[Although we provide suggestions for how to improve the design of AI ethics toolkits, we are wary of wholesale endorsing this form, as it may lead towards a technosolutionist approach. Nonetheless, this is the dominant paradigm for resources to support AI ethics in practice. As they are widely used, we believe there is value in exploring how the toolkit may be improved following the “practical turn” of values in design research, while simultaneously grappling with its limitations.] prevalent in today’s resources.
Background
Toolkits
As a genre
What sort of thing is a toolkit? At their core, toolkits are curated collections of tools and materials. Examples abound: do-it-yourself construction toolkits; first aid kits; traveling salesman kits; and research toolkits for (e.g.,) conducting participatory development efforts in rural communities, among many other examples. If we view them as a genre of communication, we can see how their design choices structure their users’ actions and interactions by conveying expectations for how they might be used. As Mattern has argued, toolkits make particular claims about the world through their design—they construct an imagined user, make an implicit argument about what forms of knowledge matter, and suggest visions for the way the world should be. As a genre of communication, toolkits suggest a set of practices in a commonly recognized form; they formalize complex processes, but in so doing, they may flatten nuance and suggest that the tools to solve complex problems lie within the confines of the kit. Although artifacts can make certain practices legible, understandable, and knowable across different contexts, they can also abstract away from locally situated practices. Moreover, toolkits work to configure what Goodwin calls professional vision: “socially organized ways of seeing and understanding events that are answerable to the distinctive interests of a particular social group”. This professional vision has political implications: in Goodwin’s analysis, U.S. policing creates “suspects” to whom “use of force” can be applied; it is thus critical to examine how toolkits may configure the professional vision of AI practitioners working on ethics.=-1
In AI ethics
In light of AI practitioners’ needs for support in addressing the ethical dimensions of AI, technology companies, researchers at CSCW, FAccT, CHI, and other venues, as well as other groups have developed numerous tools and resources to support that work, with many such resources taking the form of toolkits. Several papers have performed systemic meta-reviews and empirical analyses of AI ethics toolkits. For instance, one line of research performs descriptive analyses of AI ethics toolkits, including’s work identifying stakeholder types common across toolkits, and stages in the organizational lifecycle at which various toolkits are applied, as well as’s work proposing a typology of AI ethics approaches synthesized from a variety of toolkits, and’s analysis of 77 AI ethics toolkits, finding that many lack instructions or training to facilitate adoption. In addition, others have conducted more empirical examination of toolkits, including’s normative evaluation of six open source fairness toolkits, using surveys and interviews with practitioners to understand the strengths and weaknesses of these tools, as well as’s work conducting simulated ethics scenarios with ML practitioners, observing their experience using various ethics toolkits to inform recommendations for their design, and’s work exploring how practitioners use toolkits in their AI ethics work in practice.
In technology fields other than AI ethics, others have studied how design toolkits shape work practices. For instance,identify how toolkits operationalize ethics, identify their audience, and embody specific theories of change.’s analysis of cybersecurity toolkits reveals a complex set of “differentially” vulnerable persons, all attempting to achieve security for their socially situated needs. Building on prior empirical work evaluating the functionality and usability of AI ethics toolkits, we take a critical approach to understand the work practices that toolkits envision for their imagined users, and how those work practices might be enacted in particular sites of technology production. In other words, we focus our analysis on how toolkits help configure the organizational practice of AI ethics.
AI Ethics in Organizational Practice
As the field of AI ethics has moved from developing high-level principlesto operationalizing those principles in particular sets of practices, prior research has identified the crucial role that social and organizational dynamics play in whether and how those practices are enacted in the organizational contexts where AI systems are developed. Substantial prior work has identified the crucial role of organizational dynamics (e.g., workplace politics, institutional norms, organizational culture)in shaping technology design practices more broadly. Prior ethnographic research on the work practices of data scientists has identified how technical decisions are never just technical—that they are often contested and negotiated by multiple actors (e.g., data scientists, business team members, user researchers) within their situated contexts of work.discuss how such negotiations were shaped by the organizations’ business priorities, and how the culture and structure of those organizations legitimized technical knowledge over other types of knowledge and expertise, in ways that shaped how negotiations for technical design decisions were resolved. These dynamics are found across a range of technology practitioners, including user experience professionals, technical researchers, or privacy professionals.
Prior research on AI ethics work practices has similarly identified how the organizational contexts of AI development shape practitioners’ practices for addressing ethical concerns. Metcalf et al., explored the recent institutionalization of ethics in tech companies by tracing the roles and responsibilities of so-called “ethics owners”. In contrast with ethics owners who may have responsibility over ethical implications of AI,identified how the social pressures on AI practitioners (e.g., data scientists, ML engineers, AI product managers) to ship products on rapid timelines disincentivized them to raise concerns about potential ethical issues. Taking a wider view,discussed how AI development suffers from misaligned incentives and a lack of organizational accountability structures to support proactive anticipation of and work to address ethical AI issues. However, as resources to support AI ethics work have proliferated—including AI ethics toolkits—it is not clear to what extent the designers of those resources have learned the lessons of this research on how organizational dynamics may shape AI ethics work in practice.
Methods
Researchers’ positionality
The three authors share an interest in issues related to fairness and ethics in AI and ML systems, and have formal training in human-computer interaction and information studies, but also draw on interdisciplinary research fields studying the intersections of technology and society. All three authors are male, and live and work for academic and industry research institutions in the United States. One author’s prior research is situated in values in design, studying the practices used by user experience and other technology professionals to address ethical issues in their work, including the organizational power dynamics involved in these practices. Another author’s prior work has focused on how AI practitioners conceptualize fairness and address it in their work practices. He has conducted fairness research with AI practitioners, has contributed to multiple resources for fairness in AI, and has worked on fairness in AI at large technology companies. The third author has built course materials to teach undergraduate and graduate students how to identify and ameliorate bias in machine learning algorithms and has reflected on the ways that students do not get exposed to fairness in technical detail during their coursework. =-1
The corpus we developed may have been shaped by our positionality as researchers in academia and industry living in the U.S. and conducting the search in English. Our prior research with technology practitioners led us to focus on the artifact of the “toolkit,” which we have encountered in our prior work, although we recognize that this focus may obscure other artifacts and forms of action that are currently in use but that did not fit our conception of a toolkit. Furthermore, our familiarity with gaps between the corporate rhetoric of ethical action and actual practices related to ethical action (e.g.,) led us to focus our research questions and analysis to highlight potential gaps between the rhetoric or imaginaries embedded in toolkits and the practices or tensions we are familiar with from our prior work and experiences with practitioners. This framing is one particular lens with which to understand these artifacts, although there may be other lenses that may provide additional insights.
Corpus development
We conducted a review of existing ethics toolkits, curated to explore the breadth of ways that ethical issues are portrayed in relation to developing AI systems. We began by conducting a broad search for such artifacts in May-June 2021. We searched in two ways. First, we looked at references from recent research papers from CSCW, FAccT, and CHI that survey ethical toolkits. Second, following the approach in, we emulated the position of a practitioner looking for ethical toolkits and conducted a range of Google searches for artifacts using the terms: “AI ethics toolkit,” “AI values toolkit,” “AI fairness toolkit,” “ethics design toolkit,” “values design toolkit.” Several search results provided artifacts such as blog posts or lists of other toolkits, and many toolkits appeared in results from multiple search terms.[Although not all toolkits specifically focused on AI (some focused on “algorithms” or “design”), their content and their inclusion in search results made it reasonably likely that a practitioner would consult with the resource in deciding how to enact AI ethics.] We shared and discussed these resources with each other to discuss what might (not) be considered a toolkit (for instance, we decided to exclude ethical oaths or compilations of tools).[Note that the term toolkit is used in this paper is an analytical category chosen by the researchers to search for and describe the artifacts being studied. Not all the artifacts we analyzed explicitly described themselves using the term toolkit. See the Appendix for more details about the toolkits.] Although we broadly view toolkits as curated collections of tools and materials, we largely take an inductive approach to understanding what toolkits purport to be. From these search processes, we initially identified 57 unique candidate toolkits for analysis.
Our goal was to identify a subset of toolkits for deeper qualitative analysis in order to sample a variety of types of toolkits (rather than attempt to create an exhaustive or statistically representative sample). After reading through the toolkits, we discussed potential dimensions of variation, including: the source(s) of the toolkit (e.g., academia, industry, etc), the intended audience or user, form factor(s) of the toolkit and any guidance it provided (e.g., code, research papers, documentation, case studies, activity instructions, etc.), and its stated goal(s) or purpose(s). We also used the following criteria to narrow the corpus for deeper qualitative analysis:
- The toolkit’s audience should be a stakeholder related to the design, deployment, or use of AI systems. This led us to exclude toolkits such as Shen et al.’s value cards, designed primarily for use in a student or educational setting, but not to exclude toolkits such as, intended to be used by community advocates.
- We excluded five artifacts that focused on non-AI systems, and four designed to be used in classroom settings.
- =-1
- The toolkit should provide specific guidance or actionable items to its audience, which could be technical, organizational, or social actions. Artifacts that provided lists of other toolkits or only provided informational materials were excluded (e.g., a blog post advocating for greater use of value-sensitive design).
- We excluded five artifacts that were primarily informational or advocacy materials, four where we could not access enough information, such as paywalled services, and two that focused on professional education activities.
- Given our focus on practice, the toolkit should have some indication of use (by stakeolders either internal or external to companies). Although we are unable to validate the extent to which each toolkit has been adopted,
- we used a set of proxies to estimate which toolkits are likely to have been used by practitioners, including whether it appeared in practitioner-created lists of resources, its search results rankings, or (for open source code toolkits) indications of community use or contributions. One author also works in an industry institution, and was able to provide further insight into toolkit usage by industry teams. This excluded some toolkits that were created as part of academic papers, and which did not seem to be more broadly used by practitioners at the time of sampling, such as FairSight.
- We excluded seven artifacts that seemed to have low use, and two artifacts that were primarily academic research papers.
- In addition, due to the authors’ language limitations, we excluded one toolkit not in English.
We independently reviewed the toolkits for inclusion, exclusion, or discussion. As a group, we discussed toolkits that we either marked for discussion or that we rated differently. To resolve disagreements, we decided to aim for variation along multiple dimensions (a toolkit that overlapped a lot with an already included toolkit was less likely to be included). From the 57 candidates, 30 total were excluded. The final corpus includes 27 toolkits, which are summarized in Section section-corpus-description and fully listed in Appendix section-toolkit-list.
Corpus Analysis
In the first round of our analysis, we conducted an initial coding of the 27 toolkits based on the following dimensions: the source(s) of the toolkit (e.g., academia or industry), the intended audience or user, its stated goal(s), and references to the ML pipeline.[Although many of these were explicitly stated in the toolkits’ documentation, some required some interpretative coding. We resolved all disagreements through discussion amongst all three authors.] We used the results of this initial coding to inform our discussions of which toolkits to include in the corpus, as well as to inform our second round of analysis. We then began a second round of more open-ended inductive qualitative analysis based on our research questions (following). From reading through the toolkits, the authors discussed potential emerging themes. These initial themes included: what work do toolkits imagine is needed to address AI ethics; who do toolkits describe as doing the work of AI ethics; how does that compare to prior research about enacting AI ethics work in practice; what types of guidance are provided in toolkits; how do toolkits refer to the organizational contexts where they may be used; how do toolkits conceptualize social values (such as fairness or inclusion); when in or beyond the design process do the toolkits suggest they should be used; the toolkits’ different form factors; what social or technical background knowledge might be required to understand or use the toolkit; and whether toolkits describe any risks or limitations associated with their use. Our open-ended exploration of these themes helped us refine our research questions (to those presented in Section sec-intro).
Based on these themes, we decided to ask the following questions of each of the toolkits to further our analysis:
- What language does the toolkit use to describe values and ethics?
- What does the toolkit say about the users and other stakeholders of the AI systems to whom the toolkit aims its attention?
- What type of work is needed to enact the toolkit’s guidance in practice?
- What does the toolkit say about the organizational context in which workers must apply the toolkit?
Each author read closely through one third of the toolkits, found textual examples that addressed each of these questions, and posted those examples onto sticky notes in an online whiteboard. Collectively, all the authors conducted thematic analysis and affinity diagramming on the online whiteboard, inductively clustering examples into higher-level themes, which we report on in the findings section.
Corpus Description
We briefly describe our corpus of 27 toolkits based on our first round of analysis.[Multiple codes could be assigned to each toolkit, so the counts may sum to more than 27.] A full listing of toolkits is in Appendix section-toolkit-list, including details of our coding results in Table tab-toolkits-analysis. The toolkit authors include: technology companies (16 toolkits), university centers and academic researchers (6), non-profit organizations or institutes (6), open source communities (2), design agencies (2), a government agency (1), and an individual tech worker (1).
The toolkits’ form factors vary greatly as well. Many are technical in nature, such as open-source code (11 toolkits), proprietary code (1), documentation (12), tutorials (2), a software product (1), or a web-based tool (1). Other common forms include exercise or activity instructions (7), worksheets (5), guides or manuals (5), frameworks or guidelines (2), checklists (2), or cards (2). Several include informational websites or reading materials (4). Considering the toolkits’ audiences, most are targeted towards technical audiences such as developers (6 toolkits), data scientists (6), designers (5), technology professionals or builders (3), implementation or product teams (3), analysts (2), or UX teams (1). Some are aimed at different levels within organizations, including: managers or product/project managers (2), executive leadership (1), internal stakeholders (1), team members (1), or organizations broadly (1). Some toolkits’ audiences include people outside of technology companies, including: policymakers or government leaders (3), advocates (3), software clients or customers (1), vendors (1), civil society organizations (1), community groups (1), and users (1). We elaborate more on the toolkits’ intended audiences in Section stakeholders.=-1
Findings
We begin our findings with a description of the language toolkits use to describe and frame the work of AI ethics (RQ1). We then discuss the audiences envisioned to use the toolkits (RQ2); and close with what the toolkits envision to be the work of AI ethics (RQ3).
Language, framing, and discourses of ethics (RQ1)
Motivating Ethics: Harms, Risks, Opportunities, and Scale
We first look at how the toolkits motivate their use. Often, they articulate a problem that the toolkit will help address. One way of articulating a problem is identifying how AI systems can have effects that harm people. In such cases, toolkits motivate ethical problems by highlighting harms to people outside the design and development process—a group that Pfaffenberger terms the “impact constituency,” the “individuals, groups, and institutions who lose as a technology diffuses throughout society”. For instance, Fairlearn describes unfairness “in terms of its impact on people — i.e., in terms of harms — and not in terms of specific causes, such as societal biases, or in terms of intent, such as prejudice” [itm-t5-fairlearn]. Other toolkits gesture towards the “impact” [itm-t2-modelcards] or “unintended consequences” [itm-t9-aiethicscards] of systems.
Conversely, other toolkits frame problems by articulating how AI systems can present risks to the organizations developing or deploying them. They highlight potential business, financial, or reputational risks, or by relating AI ethics to issues of corporate risk management more broadly. The Ethics & Algorithms toolkit, aimed at governments and organizations who are procuring and deploying AI systems describes itself as “A risk management framework for governments (and other people too!) to approach ethical issues.” [itm-t7-ethicsandalgorithms]. Other toolkits suggest that they can help manage business risks, in part by generating governance and compliance reports. In contrast with the language of harms, which focuses on people who are affected by AI systems (often by acknowledging historical harms that different groups have experienced), the language of risk is more forward facing, focusing on the potential for something to go wrong and how it might affect the organization developing or deploying the AI system—leading the organization to try to find ways to prepare contingencies for the possible negative futures it can foresee for itself.
Not all toolkits frame AI ethics as avoiding negative outcomes, however. The integrate.ai guide uses the term “opportunity,” framing AI ethics in terms of pursuing positive opportunities or outcomes. The guide argues that AI ethics can be part of initiatives “incentivizing risk professionals to act for quick business wins and showing business leaders why fairness and transparency are good for business” [itm-t16-responsibleai]. The IDEO AI Ethics cards (which in some sections also frames AI ethics in terms of harms to people) also discusses capturing positive potential, writing: “In order to have a truly positive impact, AI-powered technologies must be grounded in human needs and work to extend and enhance our capabilities, not replace them” [itm-t9-aiethicscards]. In these examples, AI ethics is framed as a way for businesses or the impact constituency to capture “upside” benefits of technology through design, development, use, and business practices.
Some toolkits imagine that the positive or negative impacts of AI technologies will occur at a global scale. This is evidenced by statements such as: “your [technology builders’] work is global. Designing AI to be trustworthy requires creating solutions that reflect ethical principles deeply rooted in important and timeless values.”[itm-t28-harmsmodeling]; or “Data systems and algorithms can be deployed at unprecedented scale and speed—and unintended consequences will affect people with that same scale and speed” [itm-t9-aiethicscards]. Framing ethics globally perhaps draws attention to potential non-obvious harms or risks that might occur, prompting toolkit users to consider broader and more diverse populations who interact with AI systems. At the same time, the language of AI ethics operating at a global scale—and thus addressable at a global scale—also suggests a shared universal definition of social values, or suggests that social values have universally shared or similar impacts. This view of values as a stable, universal phenomenon has been critiqued by a range of scholars who discuss how social values are experienced in different ways, and are situated in local contexts and practices.
Sources of Legitimacy for Ethical Action
Toolkits’ use of language also claims authority from existing discourses about what constitutes an ethical problem and how problems should be addressed. These claims help connect the toolkits’ practices to a broader set of practices or frameworks that may be more widely accepted or understood, helping to legitimize the toolkits’ perspectives and practices, and providing a useful tactical alignment between the toolkit and existing organizational practices and resources.
Perhaps surprisingly, almost none of the toolkits provide an explicit discussion of philosophical ethical frameworks. (Although toolkits may implicitly draw on different ethical theories, our focus in this analysis is on the explicit theories, discourses, and frameworks that are referred to in the text of the toolkits and their supporting documentation). One exception to this is the Design Ethically toolkit, which provides a brief overview of deontological ethics and consequentialism, calling them “duty-based” and “results-based” [itm-t1-ethicskit]. Several toolkits adopt the language of “responsible innovation.” The Consequence Scanning toolkit was developed in the U.K. and calls itself “an Agile event for Responsible Innovators” [itm-t8-consequencescanning]. The integrate.ai toolkit is titled “Responsible AI in Consumer Enterprise” [itm-t16-responsibleai]. Fairlearn notes that its community consists of “responsible AI enthusiasts” [itm-t5-fairlearn]. Several toolkits in our corpus are listed as part of Microsoft’s “responsible AI” resources [t24-hax, itm-t27-communityjury, itm-t28-harmsmodeling]. There seems to be rhetorical power in aligning these toolkits with practices of responsible innovation, although questions about what people or groups the companies or toolkit users are responsible to are not explicitly discussed. More broadly, what it means to align toolkits with responsible innovation is itself an open question.[With origins in the rise of science and technology as a vector of political power in the 20th century, “responsible innovation” frames free enterprise as the agents of ethics, implicitly removing from frame policymakers, regulation, and other forms of popular governance or oversight. Future work should investigate more deeply what discursive work “responsible innovation” does in the context of AI ethics more broadly, particularly as it concerns private enterprise.]
Other toolkits look to external laws and standards as a legitimate basis for action; ethics is thus conceptualized as complying and acting in accordance with the law. Audit-AI, a tool that measures discriminatory patterns in data and machine learning predictions, explicitly cites U.S. labor regulations set by the Equal Employment Opportunity Commission (EEOC), writing that “According to the Uniform Guidelines on Employee Selection Procedures (UGESP; EEOC et al., 1978), all assessment tools should comply to fair standard of treatment for all protected groups” [itm-t19-auditai]. Audit-AI similarly draws on EEOC practices when choosing a p-value for statistical significance and choosing other metrics to define bias. This aligns the toolkit with a regulatory authority’s practices as the basis for ethics; however, it does not explicitly question whether this particular definition of fairness is applicable in contexts beyond the cultural and legal U.S. employment context.=-1
Several toolkits frame ethics as upholding human rights principles, drawing on the UN Declaration of Human Rights. In our dataset, this occurred most prominently in Microsoft’s Harms Modeling Toolkit: “As a part of our company’s dedication to the protection of human rights, Microsoft forged a partnership with important stakeholders outside of our industry, including the United Nations (UN)” [itm-t28-harmsmodeling]. Supported by the UN’s Guiding Principles on Business and Human Rights, many large technology companies have made commitments to upholding and promoting human rights.[It has been argued that involving businesses in the human rights agenda can provide legitimacy and disseminate human rights norms in broader ways than nation states could alone. However, more recent research and commentary has been critical of technology companies’ commitments to human rights, with a 2019 UN report stating that big technology companies “operate in an almost human rights-free zone.” This corresponds with prior research that shows how human rights discourses provide one source of values for AI ethics guidelines more broadly.Many companies have existing resources or practices around human rights, such as human rights impact assessments. Framing AI ethics as a human rights issue may help tactically align the toolkit with these pre-existing initiatives and practices.=-1
The envisioned users and other stakeholders for toolkits (RQ2)
This section asks, who is to do the work of AI ethics? The design and supporting documentation of toolkits presupposes a particular audience—or, asdescribes it, they “summon” particular users through the types of shared understanding, background knowledge, and expertise they draw on and presume their users to have. The toolkits in our corpus mention several specific job categories internal to the organizations in question: software engineers; data scientists; members of cross-functional or cross-disciplinary teams; risk or internal governance teams; C-level executives; board members. To a lesser extent, they mention designers. All of these categories of stakeholders pre-configure specific logics of labor and power in technology design. Toolkits that mention engineering and data science roles focus on ethics as the practical, humdrum work of creating engineering specifications and then meeting those specifications. (One toolkit, Deon, is a command-line utility for generating “ethics checklists”) [itm-t12-deon]. For C-level executives and board members, toolkits frame ethics as both a business risk and a strategic differentiator in a crowded market. As the integrate.ai Responsible AI guide states, “Sustainable innovation means incentivizing risk professionals to act for quick business wins and showing business leaders why fairness and transparency are good for business.” [itm-t16-responsibleai]
Of course, stakeholders involved in AI design and development always already have their roles pre-configured by their job titles and organizational positionality; roles that the toolkits invoke and summon in their description of potential toolkit users and other relevant stakeholders. They (for example, “business leaders”) are sensitized toward particular facets of ethics, which are made relevant to them through legible terms (for example, “risk”). As such, the nature of these internal (i.e., internal to the institutions developing AI) stakeholders’ participation in the work of ethics is bound to vary. On what terms do these internal stakeholders get to participate? Borrowing fromwho in turn channels, what are the “terms of inclusion” for each of these internal stakeholders?
Technically-oriented tooling (like Google’s What If tool [itm-t10-whatif]) envisions technical staff who contribute directly to production codebases. Although toolkits rarely address the organizational positioning of engineers (and their concerns) directly, they are specific about the mechanism of action and means of participation for these technical tools. One runs statistical tests, provides assurances around edge cases, and keeps track of statistical markers like disparate impact or the p% rule.=-1
For social and human-centered practices, the terms of participation are less clear. The rhetoric of these toolkits is one of participation—between cross-functional teams (comprised of different roles), between C-suite executives and tech labor, and between stakeholders both internal and external to the organization. But no toolkit quite specifies how this engagement should be enacted. Methodological detail is scant, let alone acknowledgements of power differentials between workers and executives, or tech workers and external stakeholders. Even those rare toolkits that do acknowledge power as a factor—for example, what the Ethics & Algorithms toolkit lists as its “mitigation #1”—under-specify how this power should be dealt with.
“Mitigation 1. Effective community engagement is people-centered, partnerships-driven, and power-aware. Engagement with the community should be social (using existing social networks and connections), technical (skills, tools, and digital spaces), physical (commons), and on equal terms (aware of and accounting for power).” [itm-t1-ethicskit]
Although this “mitigation” refers specifically to the need to be aware of power, to account for power, it offers no specific strategies to become aware, to do such “accounting.” Who does that work, and how?
This question brings us to the second broad category of stakeholders invoked by toolkits—stakeholders external to companies, described as “the community” above. This group variously includes clients, vendors, customers, users, civil society groups, journalists, advocacy groups, community members, and others impacted by AI systems. These stakeholders are imagined as outside the organization in question, sometimes by several degrees (although some, such as customers, clients, and vendors, may be variously entangled with the organization’s operations). For example, the Harms Modeling toolkit lists “non-customer stakeholders; direct and indirect stakeholders; marginalized populations” [itm-t28-harmsmodeling]. The Community Jury mentions “direct and indirect stakeholders impacted by the technology, representative of the diverse community in which the technology will be deployed” [itm-t27-communityjury]. Google’s Model Cards describes its artifacts as being for “everyone… experts and non-experts alike” [itm-t2-modelcards]. None of those toolkits, however, provide guidance on how to identify specific stakeholders, or how to engage with them once they have been identified. Indeed, the work these external stakeholders are imagined to do in these circumstances is under-specified. Their specific roles are under-imagined, relegated to the vague “raising concerns” or “providing input” from “on-the-ground perspectives.” We return to this point in the following section.
Work practices envisioned by toolkits (RQ3)
Much of the work of ethics as imagined by the toolkits focuses on technical work with ML models, in specific workflows and tooling suites, despite claims that fairness is sociotechnical (e.g., [itm-t5-fairlearn]). Many toolkits aimed at design and development teams call for engagement with stakeholders external to the team or company—and for such stakeholders to inform the team about potential ethical impacts, or for the AI design team to inform and communicate about ethical risks to stakeholders. However, there is little guidance provided by the tools on how to do this; these imagined roles for stakeholders beyond the development team are framed as informants or as recipients of information (without the ability to shape systems’ designs). Moreover, the technical orientation of many toolkits may preclude meaningful participation by non-technical stakeholders. As framed by the toolkits, the work of ethics is often imagined to be done by individual data scientists or ML teams, both of whom are imagined to have the power to influence key design decisions, without considering how organizational power dynamics may shape those processes. The imagined work of ethics here is largely individual self-reflection, or team discussions, but without a theory of change for how self-reflection or discussions might lead to meaningful organizational shifts.
Emphasis on technical work
Much of the work of ethics as imagined by the toolkits (and their designers) is focused on technical work with ML models, ML workflows, and ML tooling suites—with few exceptions, i.e., the Algorithmic Equity Toolkit [itm-t17-aekit] and others [itm-t8-consequencescanning, itm-t27-communityjury] (the forms of non-technical work that these few toolkits suggest is an area for further exploration, which we discuss in Section section-recommendations-design). This is in spite of the claims from some toolkits that “fairness is a sociotechnical problem” [itm-t5-fairlearn, itm-t27-communityjury]. In practice, this means that tools’ imagined (and suggested) uses are oriented around the ML lifecycle, often integrated into specific ML tool pipelines. For instance, Amazon’s SageMaker describes how it provides the ability to “measure biases that can occur during each stage of the ML lifecycle (data collection, model training and tuning, and monitoring of ML models deployed for inference)” [itm-t22-sagemaker]. Other toolkits go further, and are specifically designed to be implemented into particular ML programming tooling suites, such as Scala or Spark [itm-t18-lift], TensorFlow, or Google Cloud AI platform [itm-t10-whatif, itm-t20-tensorflow]. Some toolkits, albeit substantially fewer, provide recommendations for how toolkit users might make different choices about how to use the tool depending on where they are in their ML lifecycle [itm-t3-aif360].
However, this emphasis on technical functionality offered by the toolkits, as well as the fact that many are designed to fit into ML modeling workflows and tooling suites suggests that non-technical stakeholders (whether they are non-technical workers involved in the design of AI systems, or stakeholders external to technology companies) may have difficulty using these toolkits to contribute to the work of ethical AI. At the very least, it implies that the intended users must have sufficient technical knowledge to understand how they would use the toolkit in their work—and further reinforces that the work of AI ethics is technical in nature, despite claims to the contrary [itm-t5-fairlearn, itm-t27-communityjury]. In this envisioned work, what role is there for designers and user researchers, for domain experts, or for people impacted by AI systems, in doing the work of AI ethics?
Calls to engage stakeholders, but little guidance on how
One of the key elements of AI ethics work suggested by toolkits involves engaging stakeholders external to the development team or their company (as discussed in Sec. stakeholders). However, many toolkits lacked specific resources or approaches for how to do this engagement work. Toolkits often advocated for working with diverse groups of stakeholders to inform the development team about potential impacts of their systems, or to “seek more information from stakeholders that you identified as potentially experiencing harm” [itm-t28-harmsmodeling]. For some toolkits, this was envisioned to take the form of user research, recommending that teams “bring on a neutral user researcher to ensure everyone is heard” [itm-t27-communityjury] (what it means for a researcher to be “neutral” is left to the imagination), or to “help teams think through how people may interact with a design” [itm-t9-aiethicscards]. Others envisioned this information gathering as workshop sessions or discussions, as in the consequence scanning guide [itm-t8-consequencescanning] or community jury approach [itm-t27-communityjury].=-1
Although some toolkits called for AI development teams to learn about the impacts of their systems from external stakeholders, a smaller subset were designed to support external stakeholders or groups in better understanding the impacts of AI. For instance, the Algorithmic Equity Toolkit was designed to help citizens and community groups “find out more about a specific automated decision system” by providing a set of questions for people to ask to policymakers and technology vendors [itm-t17-aekit]. In addition, some developer-facing tools such as Model Cards were designed to provide information to “help advocacy groups better understand the impact of AI on their communities” [itm-t2-modelcards]. Despite these calls for engagement, toolkits lack concrete resources for precisely how to engage external stakeholders in either understanding the ethical impact of AI systems or involving them in the process of their design to support more ethical outcomes. Some toolkits explicitly name particular activities that would benefit from involving a wide range of stakeholders, such as the Harms Modeling toolkit: “You can complete this ideation activity individually, but ideally it is conducted as collaboration between developers, data scientists, designers, user researcher, business decision-makers, and other disciplines that are involved in building the technology” [itm-t28-harmsmodeling]. The stakeholders named by the Harms Modeling toolkit, however, are still “disciplines involved in building the technology” [itm-t28-harmsmodeling] and not, for instance, people who are harmed or otherwise impacted by the system outside of the company. Others, such as the Ethics & Algorithms toolkit, broaden the scope, recommending that “you will almost certainly need additional people to help - whether they are stakeholders, data analysts, information technology professionals, or representatives from a vendor that you are working with” [itm-t7-ethicsandalgorithms]. However, despite framing the activity as a “collaboration” [itm-t28-harmsmodeling] or “help” [itm-t7-ethicsandalgorithms] such toolkits provide little guidance for how to navigate the power dynamics or organizational politics involved in convening a diverse group to use the toolkit.
Theories of change
Ethical AI toolkits present different theories of change for how practitioners using the toolkits may effect change in the design, development, or deployment of AI/ML systems. For many toolkits, individuals within the organization are envisioned to be the catalysts for change via oaths [itm-t13-designethically] or “an individual exercise” [itm-t1-ethicskit] where individuals are prompted to “facilitat[e] your own reflective process” [itm-t1-ethicskit]. This approach is aligned with whatBoyd and others have referred to as developing ethical sensitivity. Some toolkits explicitly articulated the belief that individual practitioners who are aware of possible ethical issues may be able to change the direction of the design process. For instance, “The goal of Deon is to push that conversation forward and provide concrete, actionable reminders to the developers that have influence over how data science gets done” [itm-t12-deon]. However, this belief that individual data scientists “have influence over how data science gets done” may be at odds with the reality of organizational power structures that may lead to changes in AI design.
In other cases, the implicit theory of change involves product and development teams having conversations, which are then thought to lead to changes in design decisions towards more ethical design processes or outcomes. Some toolkits propose activities designed to “elicit conversation and encourage risk evaluation as a team” [itm-t7-ethicsandalgorithms]. Others start with individual ethical sensitivity, then move to team-level discussions, suggesting that the toolkit should “provoke discussion among good-faith actors who take their ethical responsibilities seriously” [itm-t12-deon]. Such group-level activities rely on having discussions with “good-faith actors,” presumably those who have developed some level of individual sensitivity to ethical issues. As one toolkit suggests for these group-level conversations, “There is a good chance someone else is having similar thoughts and these conversations will help align the team” [itm-t9-aiethicscards]. In this framing, the work of ethics involves finding like-minded individuals and getting to alignment within the team. However, this approach relies on the possibility of reaching alignment. As such, it may not provide sufficient support for individuals whose ethical views about AI may differ from their team. Individuals may feel social pressure from others on their team to stay silent, or not appear to be contrarian in the face of consensus from the rest of their team.
In fact, despite many toolkits’ claims to empower individual practitioners to raise issues, toolkits largely appeared not to address fundamental questions of worker power and collective action. For instance, the IDEO AI Ethics Cards state that “all team members should be empowered to trust their instincts and raise this Pause flag… at any point if a concept or feature does not feel human-centered” [itm-t9-aiethicscards], and similarly the Design Ethically Toolkit advises that “Having a variety of different thinkers who are all empowered to speak in the brainstorm session makes a world of a difference” [itm-t13-designethically]. However, the Design Ethically toolkit was the only example in our corpus that provided resources to support workplace organizing to meaningfully secure power for tech workers in driving change within their organizations.
Finally, other toolkits pose theories of change that suggest that pressure from external sources (i.e., media, public pressure or advocacy, or other civil society actors or organizations) may lead to changes in AI design and deployment (usually implied to be within corporate or government contexts). The Algorithmic Equity Kit in particular, is explicitly designed to provide resources for “community groups involved in advocacy campaigns” [itm-t17-aekit] to help support that advocacy work. Other toolkits, such as the Ethics & Algorithms Toolkit, focus on government agencies using AI that are “facing increasing pressure from the public, the media, and academic institutions to be more transparent and accountable about their use” [itm-t7-ethicsandalgorithms]. As such, the toolkit offers resources for government agencies to respond to such pressure and provide more transparency and accountability in their algorithmic systems.
More generally, many toolkits enact some form of solutionism—the belief that ethical issues that may arise in AI design can be solved with the right tool or process (typically the approach they propose). Some tools [e.g., itm-t2-modelcards, itm-t3-aif360, itm-t10-whatif, itm-t20-tensorflow] suggest that ethical values such as fairness can be achieved via technical tools alone: “If all fairness metrics are fair, The Bias Report will evaluate the current model as fair.” [itm-t6-aequitas]. Some toolkits (albeit fewer) do note the limitations of purely technical solutions to fundamentally sociotechnical problems [itm-t3-aif360, itm-t5-fairlearn, itm-t10-whatif], as in AIF360’s documentation, which states that “the metrics and algorithms in AIF360… clearly do not capture the full scope of fairness in all situations” [itm-t3-aif360]. As the What-If tool documentation states, “There is no one right [definition of fairness], but we probably can agree that humans, not computers, are the ones who should answer this question” [itm-t10-whatif]. However, even with these acknowledgements, the documentation goes on to note the important role that the toolkit plays in enabling humans to answer that question, as “What-If lets us play `what if’ with theories of fairness, see the trade-offs, and make the difficult decisions that only humans can make” [itm-t10-whatif].
These general framings suggest a particular flavor of solutionism, in which the work of ethics in AI design involves following a particular process (i.e., the one proposed by the toolkit). Toolkits propose ethical work practices that fit into existing development processes [e.g., itm-t12-deon], in ways that suggest that all that is needed is the addition of an activity or discussion prompt and not, for instance, fundamental changes to the corporate values systems or business models that may lead to harms from AI systems. Some toolkits were explicit that ethical AI work should not significantly disrupt existing corporate priorities, saying, “Business goals and ethics checks should guide technical choices; technical feasibility should influence scope and priorities; executives should set the right incentives and arbitrate stalemates” [itm-t16-responsibleai].=-1
Discussion
Throughout these toolkits, we observed a mismatch between the imagined roles and work practices for ethics in AI and the support the toolkits provided for achieving those roles and practices. Specifically, despite rhetoric from the documentation of many toolkits that the work of ethics is _socio_technical, involving contributions from a variety of stakeholders, the actual design and functionality of the majority of toolkits involved technical work for primarily developers and data scientists. Toolkits suggested multi-stakeholder approaches to addressing ethical issues in sociotechnical ways, but most toolkits provided little scaffolding for the social dimensions of ethics or for engaging stakeholders from multiple (non-technical) backgrounds. These technosolutionist approaches to AI ethics suggest that AI ethics toolkits may act as a “technology of de-politicization”, sublimating sociopolitical considerations in favor of technical fixes. With few exceptions [e.g., itm-t17-aekit], the toolkits took a decontextualized approach to ethics, largely divorced from the sociopolitical nuance of what ethics might mean in the contexts in which AI systems may be deployed, or how ethical work practices might be enacted within the organizational contexts of the sites of AI production (e.g., technology companies). In such a decontextualized view of ethics, toolkit designers envision individual users who have the agency to make decisions about their design of AI systems, and who are not beholden to the role of power dynamics within the workplace: organizational hierarchies, misaligned priorities, and incentives for ethical work practices—key considerations for the use of AI ethics toolkits, given the reality of business priorities and profit motives.=-1
When toolkits did attend to how ethical work might fit within business processes, many of them leveraged discourses of business risk and responsible innovation to help motivate adoption of ethics tools and processes. These discourses may function tacticallyas a way to allow toolkits to tap into existing institutional processes and resources they may not otherwise have access to (for example, mechanisms for managing legal liability). However, in so doing, companies may sidestep questions of how logics of capital accumulation themselves shape the capacity for AI systems to exert harms and shape the sociotechnical imaginariesfor what ethics might mean—or foreclose alternative ways of conceptualizing ethics. As a result, ethical concerns may be sublimated to the interests of capital. In the following sections, we unpack implications of our findings for AI ethics toolkit researchers and designers.=-1
Reflections and Implications for Research
As the prior sections suggest, the content and guidance provided by toolkits, as well as the metaphor and format of “toolkits” as a predominant way to address AI ethics, constructs particular ways of seeing the world—what constitutes an ethical problem, who should be responsible for addressing those problems, and what are the legitimate practices for addressing them. We underscore this point by using the metaphor of “seeing like a toolkit,” to draw attention to two ideas.
First, although toolkits provide a useful format for sharing information and practices across boundaries and contexts, an over-reliance on toolkits may risk decontextualizing or abstracting away from the social and political contexts where AI systems are deployed and governed, and from the organizational contexts in which those toolkits may be used. Toolkits, by design, are intended to be portable objects usable across a variety of contexts—but as a result, ethical AI toolkits may act as a “device for decontextualizing”. This portability may allow toolkits to be more generalizable or scalable by “mediating between the local and the universal”in order to support their adoption and use across multiple contexts. However, the flattening of local distinctiveness in order to be more easily transportable across contextsbrings with it particular risks for ethical AI. As Selbst et al., have written, efforts for fairness in AI run the risk of what they have referred to as “abstraction traps,” or abstracting away crucial elements of the social context in which AI systems are deployed and within which fairness and ethical considerations must be understood. As a result, toolkits that are explicitly designed to be decontextualized—both from the social context where AI systems will be deployed (and within which ethics must be understood) and from the organizational context in which those toolkits may be used—may inadvertently suggest to their users that either the context does not matter for the work of ethics, or that it is up to the toolkit user to do the work of _re_contextualizing, or translating its methods for their context of use and deployment (cf.). However, this is quite a burden for the toolkits to place on their users, particularly as the imagined users of many ethical AI toolkits appear to be largely technical practitioners who may not have the training or background to do such contextualization and translation work.
This pattern of decontextualization of toolkits mirrors Scott’s concepts of legibility and simplification in statecraft.[whose book Seeing Like a State informs the title of this paper] In order to govern, the state employs techniques such as standardized measurement or systems of private property ownership to make local heterogeneous practices legible, but this also serves to simplify and standardize understandings of social practices which may not equate with local experiences. Similarly, for toolkits to be legible among communities of practice and organizational structures that seek to build systems at scale, toolkits make ethical practices legible in ways that are often simplified and do not account for the hetereogeneity of contextual experiences and on the ground practices of doing AI ethics, requiring users who can do this difficult translation work.
Second, these toolkits represent a form of “professional vision” that may inadvertently promote a solutionist orientation to AI ethics. As Goodwin has argued, “professional vision” is how the discursive practices of professional cultures shape how we see the world in socially situated and historically constituted ways. Similarly, in Silbey’s work on industrial safety culture, she argues that disasters that are not spectacular or sudden—such as slow-acting oil leaks—are often ignored, “existing physically, but not in any organizationally cognizable form”. For ethics in AI, the discursive practices instantiated in our tools shape how the field sees the ethical terrain for action—what are the objects of concern, how might they be made legible or amenable to action, what resources might be marshalled to address them, and by whom. Likewise, problems left outside of toolkits’ purview may risk not being seen as legitimate ethical issues by practitioners.
The tools curated within a toolkit are intended to solve particular problems (here, problems related to the ethics of AI), but the metaphor of the toolkit itself may reinforce a solutionist framing, suggesting to their users that ethical problems can in fact, be solved by using the tools or processes therein—for instance, that AI systems can be “de-biased,” which they cannot be—rather than mitigating their potential for harm. This solutionist orientation is not limited to toolkits; indeed, Selbst et al. have written about the solutionist trap for fairness in sociotechnical systems more generally, but the genre of the toolkit may inadvertently reinforce the idea of ethics as a managerial exercise, or a technical solution to fundamentally contextual and contested challenges (cf.). As a result, this framing may inhibit investment (of time, attention, resources) into alternative approaches that do not fit within the confines of the solutionist orientation of a toolkit, or foreclose alternative theories of change (such as a focus on the political economy of AI development). This may also lead to false expectations (from practitioners using the toolkit as well as stakeholders and communities impacted by AI), potentially leading to frustration, resentment, and further harm when those expectations for solved problems are not met. Others have discussed how corporate dicourse of “solving” ethical issues are often rooted in public relations goals or economic self-interest.
This is a broader issue for the field. Metcalf and Moss discuss how ethics in Silicon Valley is in part framed through the lenses of technological solutionism and market fundamentalism—that an optimal set of tools, procedures, or criteria will lead to an ethical outcome, and that ethical solutions should be pursued within the boundaries of what the market finds profitable. These lenses miss out on the value of non-technical expertise and practices, as well as a broader array of potential ethical (if less profitable) alternatives. What do we lose when we fail to grapple with capital as a force in shaping the ethical considerations of AI? We note that these critiques are not a call to abandon toolkits altogether, but rather an interrogation of what politics we might (unintentionally) embed when framing an AI ethics intervention as a “toolkit.” What are the political choices one makes when one creates a toolkit, and how can we make those choices more intentional? Although we find that AI ethics toolkits tend to focus on technical practices in ways that may be decontextualized from the wider social and political context, we are inspired by toolkits in other domains that explicitly engage in questions of politics and power, for example toolkits that serve as methods of participatory engagement to purposefully include broader communities to consider issues of justice.
We also consider the politics of the choice of deciding to make a “toolkit” versus making something else. We thus ask what ways of “seeing” AI ethics do all toolkits miss? What are new ways of seeing that can produce new, practical interventions? New approaches might move beyond toolkits and look to other theories of change, such as political economy. However, we as authors note that our situatedness in particular debates in the West may occlude our sensitivity to alternative ethical frameworks. Indigenous notions of “making kin”could reveal radical new possibilities for what AI ethics could be, and by what processes it may be enacted. How can we, as a research community, make space for such alternatives? Following from this problem-posing orientation, we do not offer solutions here, but instead pose these as questions for researchers, practitioners, and communities to address through developing alternatives to the dominant paradigm of the toolkit. Some promising examples include the People’s Guide to AI zine; J. Khadijah Abdurahman’s and We Be Imagining’s call for lighting “alternate beacons” to help “organize for different futures” for technology development; and the AI Now Institute’s series on a new lexicon to offer narratives beyond those from the Global North to critically study AI, among others. We call on the CSCW community and others (e.g., FAccT, CHI) to amplify and expand these efforts.=-1
Recommendations for Toolkit Design
Practitioners will continue to require support in enacting ethics in AI, and toolkits are one potential approach to provide such support, as evidenced by their ongoing popularity. Although much of this paper has focused on a critical analysis of toolkits, we offer suggestions for toolkit design following the “practical turn” in values in design research—i.e., if we accept that toolkits can embody and promote particular social values, we might consider an additional (or alternative) set of values in the design of toolkits. We acknowledge that toolkits alone will not solve all the problems of addressing AI ethics, but they can nevertheless be improved to better consider the social and organizational contexts where they might be deployed.
Our findings suggest three concrete recommendations for improving toolkits’ potential to support the work of AI ethics. Toolkits should: (1) provide support for the non-technical dimensions of AI ethics work; (2) support the work of engaging with stakeholders from non-technical backgrounds; (3) structure the work of AI ethics as a problem for collective action.
Embrace the non-technical dimensions of ethics work
Despite emerging awareness that fairness is _socio_technical, the majority of toolkits provided resources to support technical work practices (although some toolkits called for their users to engage in other forms of work [e.g., itm-t5-fairlearn]). This might entail resources to support understanding the theories and concepts of ethics in non-technical ways,[Note that Fairlearn [itm-t5-fairlearn] has—since we conducted the data analysis for this paper—published resources in its user guide for understanding social science concepts such as construct validity for concepts such as fairnessand explanations of sociotechnical abstraction traps.] as well as resources drawing from the social sciences for understanding stakeholders’ situated experiences and perceptions of AI systems and their impacts. For instance, toolkit designers might incorporate methods from qualitative research, user research, or value-sensitive design (e.g.,), as some existing tools suggest (e.g., [itm-t27-communityjury]). Although some AI ethics education tools are beginning to be designed with these perspectives (e.g., value cards), fewer practitioner-oriented toolkits utilize them. As a precursor to this, practitioners may need support in identifying the stakeholders for their systems and use cases, in the contexts in which those systems are (or will be) deployed, including community members, data subjects, or others beyond the users, paying customers, or operators of a given AI system. Approaches such as stakeholder mapping from fields like Human-Computer Interactionmay be useful here, and such resources may be incorporated into AI ethics toolkits.
Support for engaging with stakeholders from non-technical backgrounds
Although many toolkits call for engaging stakeholders from different backgrounds and with different forms of expertise (internal stakeholders such as designers or business leaders; external stakeholders such as advocacy groups and policymakers), the toolkits themselves offer little support for how their users might bridge such disciplinary divides, further contributing to the mismatch between the rhetorical promise of toolkits and their current design. Toolkits should thus support this translational work.[Some emerging work is exploring the role of “boundary objects”to help practitioners align on key concepts and develop a shared language, e.g., https://events.withgoogle.com/pair-symposium-2020/PAIR Symposium 2020, although this work has not focused on ethics of AI specifically.] This might entail, for instance, asking what fairness means to the various stakeholders implicated in ethical AI, or communicating the output of algorithmic impact assessments (e.g., various fairness metrics) in ways that non-technical stakeholders can understand and work with. The Algorithmic Equity Toolkit (whose design process is discussed in) tackles this challenge from the perspective of community members and groups, providing resources to these external stakeholders to support their advocacy work [itm-t17-aekit]. Meanwhile, recent research has explored how to engage non-technical stakeholders in discussions about tradeoffs in model performance, or in participatory AI design processes more generally, although such approaches have largely not been incorporated into toolkits (with few recent exceptions). Moreover, approaches that involve stakeholders impacted by AI conducting “crowd audits” of algorithmic harmshave not yet made their way into the toolkits we analyzed, where the results of such crowd audits might be used to shape AI practitioners’ development practices.=-1
Structure the work of AI ethics as a problem for collective action
One question we found palpably missing in the toolkits we analyzed was, how do toolkits support stakeholders in grappling with organizational dynamics involved in doing the work of ethics? Silbey has written about the “safety culture” promoted in other high-stakes industries (e.g., fossil fuel extraction), where the responsibility to avoid catastrophe is too often located in the behaviors and attitudes of individual actors—typically those with the least power in the organization—rather than systemic processes or organizational oversight. To address this gap, toolkits could provide support for helping practitioners communicate to organizational leadership and advocate for the need to engage in ethical AI work practices, or advocate for additional time or resources to do this work. One form this might take is providing support for strategic alignment of ethics discourses with business priorities and discourses (e.g., business risk, responsible innovation, corporate social responsibility, etc). However, these discourses bring risks: the aims and values of ethical AI could be subverted by business priorities. For instance,discuss how business priorities for AI deployment across market tiers may subvert practitioners’ goals for fairness work. Given the risk that such an approach might smuggle in business logics that subvert ethical aims (see Sec. discourses), toolkit designers might instead consider how to support the users of their toolkits in becoming aware of the organizational power dynamics that may impact the work of ethics (e.g., power mapping exercises), including identifying institutional levers they can pull to shape organizational norms and practices from the bottom up. In addition, toolkits should structure ethical AI as a problem for collective action for multiple groups of stakeholders, rather than work for individual practitioners. This may involve supporting collective action by workers within tech companies, or fostering communities of practice of professionals working on ethical AI across institutions (to share knowledge and best practices, as well as shift professional norms and standards), or supporting collective efforts for ethical AI across industry professionals designing AI and communities impacted by AI. This might also involve providing support for organizing collective action in the workplaces, such as unions, tactical walkouts, or other uses of labor power based on their role in technology production. Prior research found that technology professionals pursuing design justice sought project- and institutional-level tools and interventions rather than individual-level ones. However, few toolkits we saw (with the Data Ethically toolkit as a notable exception [itm-t13-designethically]) provide resources to inform and support practitioners about the role of collective action in ethical AI.=-1
Limitations and Future Work
We examined a small subset of toolkits which may not be representative of all AI ethics toolkits. Most of the toolkits we examined were from tech companies and academia, and we may thus have missed out on toolkits developed by nonprofits, civil society, or government agencies. Furthermore, the toolkits we examined largely skewed towards industry practitioners as the envisioned users (with some exceptions; e.g., [itm-t17-aekit]), and were largely intended to fit into AI development processes (as suggested by the large proportion of toolkits that were open source code). As such, future work should explicitly target toolkits intended to be used by policymakers, civil society, or community stakeholders more generally. Recognizing that creating technical tools can re-inscirbe the harms they seek to address (e.g.,) in addition to re-designing the politics of toolkits, future work should also investigate other forms of political action that consider and address the social and institutional aspects of technology development.
In addition, our corpus was built from search queries; as such, searching for toolkits using terms we did not include here may result in identifying toolkits that we did not include in our corpus. More broadly, our positionality has shaped how we approach our research, including the research questions we chose, the toolkits we identified, and how we coded and interpreted our data. As Sambasivan et al.(among others, such as) have pointed out, AI ethics may mean different things in different cultural contexts, including relying on different legal frameworks, and aiming towards fundamentally different outcomes. Our corpus is necessarily partial and reflective of our positionality and cultural context.=-1
Conclusion
This paper investigates how AI ethics toolkits frame and embed particular visions for what it means to do the work of addressing ethics. Based on our findings, we recommend that designers of AI ethics toolkits should better support the social dimensions of ethics work, provide support for engaging with diverse stakeholders, and frame AI ethics as a problem for collective action rather than individual practice. Toolkit development should be tied more closely to empirical research that studies the social, organizational, and technical work required to surface and address ethical issues. Creating tools or resources in a format that challenges the notions of the “toolkit” per se may open up the design space to foster new approaches to AI ethics. Although no single artifact alone will solve all AI ethics problems, intentionally diversifying the forms of work that such artifacts envision and support may enable more effective ethical interventions in the work practices adopted by developers, designers, researchers, policymakers, and other stakeholders.
Thank you to Emma Lurie, Zoe Kahn, Ken Holstein, our colleagues at the UC Berkeley Center for Long-Term Cybersecurity and Microsoft Research, and the anonymous reviewers for their comments and feedback on this work.
Toolkit Listing and Analysis
Ethics Kit, http://ethicskit.org/tools.html
Model Cards, https://modelcards.withgoogle.com/about
AI Fairness 360, https://aif360.mybluemix.net/
InterpretML, https://github.com/interpretml/interpret
Fairlearn,https://fairlearn.github.io/
Aequitas,http://aequitas.dssg.io/
Ethics & Algorithms Toolkit https://ethicstoolkit.ai/
Consequence Scanning Kit,https://www.doteveryone.org.uk/project/consequence-scanning/
AI Ethics Cards, https://www.ideo.com/post/ai-ethics-collaborative-activities-for-designers
What If Tool, https://pair-code.github.io/what-if-tool/
Digital Impact Toolkit, https://digitalimpact.io/toolkit/
Deon Ethics Checklist, http://deon.drivendata.org/
Design Ethically Toolkit, https://www.designethically.com/toolkit
Lime, https://github.com/marcotcr/lime
Weights and Biases, https://wandb.ai/site
Responsible AI in Consumer Enterprise,https://static1.squarespace.com/static/5d387c126be524000116bbdb/t/5d77e37092c6df3a5151c866/1568138185862/Ethics-of-artificial-intelligence.pdf
Algorithmic Equity Toolkit (AEKit),https://www.aclu-wa.org/AEKit
LinkedIn Fairness Toolkit (LiFT), https://github.com/linkedin/LiFT , https://engineering.linkedin.com/blog/2020/lift-addressing-bias-in-large-scale-ai-applications
Audit AI, https://github.com/pymetrics/audit-ai
TensorFlow Fairness Indicators,https://github.com/tensorflow/fairness-indicators
Judgment Call,https://docs.microsoft.com/en-us/azure/architecture/guide/responsible-innovation/judgmentcall
SageMaker Clarify, https://sagemaker-examples.readthedocs.io/en/latest/sagemaker_processing/fairness_and_explainability/fairness_and_explainability.html
NLP CheckList, https://github.com/marcotcr/checklist
HAX Workbook and Playbook, https://www.microsoft.com/en-us/haxtoolkit/workbook/
Community Jury, https://docs.microsoft.com/en-us/azure/architecture/guide/responsible-innovation/community-jury/
Harms Modeling,https://docs.microsoft.com/en-us/azure/architecture/guide/responsible-innovation/harms-modeling/
Algorithmic Accountability Policy Toolkit,https://ainowinstitute.org/aap-toolkit.pdf
July 2022 [revised]October 2022 [accepted]January 2023
Bibliography
1@article{Pfaffenberger1992,
2 year = {1992},
3 volume = {17},
4 url = {https://estsjournal.org/index.php/ests/article/view/132 http://journals.sagepub.com/doi/10.1177/016224399201700302},
5 title = {{Technological Dramas}},
6 pages = {282--312},
7 number = {3},
8 month = {Jul},
9 journal = {Science, Technology, \& Human Values},
10 issn = {0162-2439},
11 file = {:C\:/Users/ryw9/AppData/Local/Mendeley Ltd./Mendeley Desktop/Downloaded/Pfaffenberger - 1992 - Technological Dramas.pdf:pdf},
12 doi = {10.1177/016224399201700302},
13 author = {Pfaffenberger, Bryan},
14 abstract = {This article examines the technological construction of political power, as well as resistance to political power, by means of an "ideal-typical" model called a technolog ical drama. In technological regularization, a design constituency creates artifacts whose features reveal an intention to shape the distribution of wealth, power, or status in society. The design constituency also creates myths, social contexts, and rituals to legitimate its intention and constitute the artifact's political impact. In reply, the people adversely affected by regularization engage in myth-, context-, or artifact-altering strate gies that represent an accommodation to the system (technological adjustment) or a conscious attempt to change it (technological reconstitution). A technological drama, in short, is a specifically technological form of political discourse. A key point is that throughout all three processes, political "intentions," no less than the facticity and hardness of the technology's "impact," are themselves constituted and constructed in reciprocal and discursive interaction with technological activities. Technology is not politics pursued by other means; it is politics constructed by technological means.},
15}
16
17@inproceedings{LeDantec2009Values,
18 year = {2009},
19 url = {http://dl.acm.org/citation.cfm?id=1518701.1518875 http://dl.acm.org/citation.cfm?doid=1518701.1518875},
20 title = {{Values as lived experience: Evolving value sensitive design in support of value discovery}},
21 publisher = {ACM Press},
22 pages = {1141},
23 keywords = {VSD,case study,critique,emergent,empirical methods,fieldwork,methodology,photo elicitation,pluralistic,values},
24 issn = {9781605582467},
25 isbn = {9781605582467},
26 file = {:C\:/Users/ryw9/AppData/Local/Mendeley Ltd./Mendeley Desktop/Downloaded/Le Dantec, Poole, Wyche - 2009 - Values as lived experience Evolving value sensitive design in support of value discovery.pdf:pdf},
27 doi = {10.1145/1518701.1518875},
28 booktitle = {Proceedings of the 27th international conference on Human factors in computing systems - CHI 09},
29 author = {{Le Dantec}, Christopher A. and Poole, Erika Shehan and Wyche, Susan P.},
30 address = {New York, New York, USA},
31 abstract = {The Value Sensitive Design (VSD) methodology provides a comprehensive framework for advancing a value-centered research and design agenda. Although VSD provides helpful ways of thinking about and designing value-centered computational systems, we argue that the specific mechanics of VSD create thorny tensions with respect to value sensitivity. In particular, we examine limitations due to value classifications, inadequate guidance on empirical tools for design, and the ways in which the design process is ordered. In this paper, we propose ways of maturing the VSD methodology to overcome these limitations and present three empirical case studies that illustrate a family of methods to effectively engage local expressions of values. The findings from our case studies provide evidence of how we can mature the VSD methodology to mitigate the pitfalls of classification and engender a commitment to reflect on and respond to local contexts of design.},
32}
33
34@inproceedings{Houston2016Values,
35 year = {2016},
36 url = {http://dl.acm.org/citation.cfm?doid=2858036.2858470},
37 title = {{Values in Repair}},
38 publisher = {ACM Press},
39 pages = {1403--1414},
40 mendeley-groups = {Post-Conference Reading/CHI 2016},
41 isbn = {9781450333627},
42 file = {:C\:/Users/ryw9/AppData/Local/Mendeley Ltd./Mendeley Desktop/Downloaded/Houston et al. - 2016 - Values in Repair.pdf:pdf},
43 doi = {10.1145/2858036.2858470},
44 booktitle = {Proceedings of the 2016 CHI Conference on Human Factors in Computing Systems - CHI '16},
45 author = {Houston, Lara and Jackson, Steven J and Rosner, Daniela K and Ahmed, Syed Ishtiaque and Young, Meg and Kang, Laewoo},
46 address = {New York, New York, USA},
47}
48
49@article{JafariNaimi2015ValuesHypotheses,
50 year = {2015},
51 volume = {31},
52 url = {http://www.mitpressjournals.org/doi/10.1162/DESI_a_00354},
53 title = {{Values as Hypotheses: Design, Inquiry, and the Service of Values}},
54 pages = {91--104},
55 number = {4},
56 month = {Oct},
57 journal = {Design Issues},
58 issn = {0747-9360},
59 isbn = {13978-3-923859-82-5},
60 file = {:C\:/Users/ryw9/AppData/Local/Mendeley Ltd./Mendeley Desktop/Downloaded/JafariNaimi, Nathan, Hargraves - 2015 - Values as Hypotheses Design, Inquiry, and the Service of Values.pdf:pdf},
61 doi = {10.1162/DESI_a_00354},
62 author = {JafariNaimi (Parvin), Nassim and Nathan, Lisa and Hargraves, Ian},
63 abstract = {(fr. hohe/ gehobene Schneiderei)},
64}
65
66@inproceedings{Shilton2014HowToSeeValues,
67 year = {2014},
68 url = {http://dl.acm.org/citation.cfm?id=2531602.2531625%5Cnhttp://dl.acm.org/citation.cfm?doid=2531602.2531625 https://dl.acm.org/doi/10.1145/2531602.2531625},
69 title = {{How to see values in social computing: Methods for Studying Values Dimensions}},
70 publisher = {ACM},
71 pages = {426--435},
72 month = {feb},
73 mendeley-tags = {dissertation,value centered design},
74 keywords = {dissertation,research methods,value centered design,value sensitive design,values in design},
75 isbn = {9781450325400},
76 file = {:C\:/Users/ryw9/AppData/Local/Mendeley Ltd./Mendeley Desktop/Downloaded/Shilton, Koepfler, Fleischmann - 2014 - How to see values in social computing Methods for Studying Values Dimensions.pdf:pdf},
77 doi = {10.1145/2531602.2531625},
78 booktitle = {Proceedings of the 17th ACM conference on Computer supported cooperative work \& social computing},
79 author = {Shilton, Katie and Koepfler, Jes A. and Fleischmann, Kenneth R.},
80 address = {New York, NY, USA},
81 abstract = {Human values play an important role in shaping the design and use of information technologies. Research on values in social computing is challenged by disagreement about indicators and objects of study as researchers distribute their focus across contexts of technology design, adoption, and use. This paper draws upon a framework that clarifies how to see values in social computing research by describing values dimensions, comprised of sources and attributes of values in sociotechnical systems. This paper uses the framework to compare how diverse research methods employed in social computing surface values and make them visible to researchers. The framework provides a tool to analyze the strengths and weaknesses of each method for observing values dimensions. By detailing how and where researchers might observe interactions between values and technology design and use, we hope to enable researchers to systematically identify and investigate values in social computing. Copyright {\textcopyright} 2014 ACM.},
82}
83
84@article{yates1992genres,
85 publisher = {Academy of Management Briarcliff Manor, NY 10510},
86 year = {1992},
87 pages = {299--326},
88 number = {2},
89 volume = {17},
90 journal = {Academy of management review},
91 author = {Yates, JoAnne and Orlikowski, Wanda J},
92 title = {Genres of organizational communication: A structurational approach to studying communication and media},
93}
94
95@inproceedings{shen2021valuecards,
96 series = {FAccT '21},
97 location = {Virtual Event, Canada},
98 keywords = {Value Cards, Machine Learning, CS Education, Deliberation, Fairness},
99 numpages = {12},
100 pages = {850–861},
101 booktitle = {Proceedings of the 2021 ACM Conference on Fairness, Accountability, and Transparency},
102 abstract = {Recently, there have been increasing calls for computer science curricula to complement existing technical training with topics related to Fairness, Accountability, Transparency and Ethics (FATE). In this paper, we present Value Cards, an educational toolkit to inform students and practitioners the social impacts of different machine learning models via deliberation. This paper presents an early use of our approach in a college-level computer science course. Through an in-class activity, we report empirical data for the initial effectiveness of our approach. Our results suggest that the use of the Value Cards toolkit can improve students' understanding of both the technical definitions and trade-offs of performance metrics and apply them in real-world contexts, help them recognize the significance of considering diverse social values in the development and deployment of algorithmic systems, and enable them to communicate, negotiate and synthesize the perspectives of diverse stakeholders. Our study also demonstrates a number of caveats we need to consider when using the different variants of the Value Cards toolkit. Finally, we discuss the challenges as well as future applications of our approach.},
103 doi = {10.1145/3442188.3445971},
104 url = {https://doi.org/10.1145/3442188.3445971},
105 address = {New York, NY, USA},
106 publisher = {Association for Computing Machinery},
107 isbn = {9781450383097},
108 year = {2021},
109 title = {Value Cards: An Educational Toolkit for Teaching Social Impacts of Machine Learning through Deliberation},
110 author = {Shen, Hong and Deng, Wesley H. and Chattopadhyay, Aditi and Wu, Zhiwei Steven and Wang, Xu and Zhu, Haiyi},
111}
112
113@misc{Shonhiwa2020humanValuesMedium,
114 year = {2020},
115 urldate = {2021-12-03},
116 url = {https://uxdesign.cc/human-values-matter-why-value-sensitive-design-should-be-part-of-every-ux-designers-toolkit-e53ffe7ec436},
117 title = {{Human values matter: why value-sensitive design should be part of every UX designer's toolkit}},
118 booktitle = {UX Collective},
119 author = {Shonhiwa, Mandla},
120}
121
122@techreport{Spitzberg2020,
123 year = {2020},
124 title = {{Principles at Work: Applying “Design Justice” in Professionalized Workplaces}},
125 pages = {1--5},
126 file = {:C\:/Users/ryw9/Box/Papers Archive/Spitzberg et al (2020) Principles at work- applying design justice in professionalized workplaces.pdf:pdf},
127 doi = {10.21428/93b2c832.e3a8d187},
128 booktitle = {CSCW 2020 Workshop on Collective Organizing and Social Responsibility},
129 author = {Spitzberg, Danny and Shaw, Kevin and Angevine, Colin and Wilkins, Marissa and Strickland, M and Yamashiro, Janel and Adams, Rhonda and Lockhart, Leah},
130}
131
132@article{Metcalf2019OwningEthics,
133 year = {2019},
134 volume = {86},
135 title = {{Owning ethics: Corporate logics, Silicon Valley, and the institutionalization of ethics}},
136 pages = {449--476},
137 number = {2},
138 journal = {Social Research},
139 issn = {0037783X},
140 file = {:C\:/Users/ryw9/AppData/Local/Mendeley Ltd./Mendeley Desktop/Downloaded/Metcalf, Moss, boyd - 2019 - Owning ethics Corporate logics, Silicon Valley, and the institutionalization of ethics.pdf:pdf},
141 author = {Metcalf, Jacob and Moss, Emanuel and danah Boyd},
142}
143
144@inproceedings{Khovanskaya2019dataRhetoric,
145 year = {2019},
146 url = {https://dl.acm.org/doi/10.1145/3322276.3323691},
147 title = {{Data Rhetoric and Uneasy Alliances: Data Advocacy in US Labor History}},
148 publisher = {ACM},
149 pages = {1391--1403},
150 month = {jun},
151 isbn = {9781450358507},
152 file = {:C\:/Users/ryw9/AppData/Local/Mendeley Ltd./Mendeley Desktop/Downloaded/Khovanskaya, Sengers - 2019 - Data Rhetoric and Uneasy Alliances Data Advocacy in US Labor History.pdf:pdf},
153 doi = {10.1145/3322276.3323691},
154 booktitle = {Proceedings of the 2019 on Designing Interactive Systems Conference},
155 author = {Khovanskaya, Vera and Sengers, Phoebe},
156 address = {New York, NY, USA},
157}
158
159@inproceedings{10.1145/3442188.3445938,
160 series = {FAccT '21},
161 location = {Virtual Event, Canada},
162 keywords = {surveillance, algorithmic justice, Participatory design, algorithmic equity, regulation, participatory action research, accountability},
163 numpages = {10},
164 pages = {772–781},
165 booktitle = {Proceedings of the 2021 ACM Conference on Fairness, Accountability, and Transparency},
166 abstract = {Motivated by the extensive documented disparate harms of artificial intelligence (AI), many recent practitioner-facing reflective tools have been created to promote responsible AI development. However, the use of such tools internally by technology development firms addresses responsible AI as an issue of closed-door compliance rather than a matter of public concern. Recent advocate and activist efforts intervene in AI as a public policy problem, inciting a growing number of cities to pass bans or other ordinances on AI and surveillance technologies. In support of this broader ecology of political actors, we present a set of reflective tools intended to increase public participation in technology advocacy for AI policy action. To this end, the Algorithmic Equity Toolkit (the AEKit) provides a practical policy-facing definition of AI, a flowchart for assessing technologies against that definition, a worksheet for decomposing AI systems into constituent parts, and a list of probing questions that can be posed to vendors, policy-makers, or government agencies. The AEKit carries an action-orientation towards political encounters between community groups in the public and their representatives, opening up the work of AI reflection and remediation to multiple points of intervention. Unlike current reflective tools available to practitioners, our toolkit carries with it a politics of community participation and activism.},
167 doi = {10.1145/3442188.3445938},
168 url = {https://doi.org/10.1145/3442188.3445938},
169 address = {New York, NY, USA},
170 publisher = {Association for Computing Machinery},
171 isbn = {9781450383097},
172 year = {2021},
173 title = {An Action-Oriented AI Policy Toolkit for Technology Audits by Community Advocates and Activists},
174 author = {Krafft, P. M. and Young, Meg and Katell, Michael and Lee, Jennifer E. and Narayan, Shankar and Epstein, Micah and Dailey, Dharma and Herman, Bernease and Tam, Aaron and Guetler, Vivian and Bintz, Corinne and Raz, Daniella and Jobe, Pa Ousman and Putz, Franziska and Robick, Brian and Barghouti, Bissan},
175}
176
177@article{goodwin2015professional,
178 year = {1994},
179 volume = {96},
180 urldate = {2023-01-14},
181 title = {Professional Vision},
182 publisher = {[American Anthropological Association, Wiley]},
183 pages = {606--633},
184 number = {3},
185 journal = {American Anthropologist},
186 author = {Charles Goodwin},
187 abstract = {Seeing is investigated as a socially situated, historically constituted body of practices through which the objects of knowledge that animate the discourse of a profession are constructed and shaped. Analysis of videotapes of archaeologists making maps and lawyers animating events visible on the Rodney King videotape focuses on practices that are articulated in a work-relevant way within sequences of human interaction, including coding schemes, highlighting, and graphic representations. Through the structure of talk in interaction, members of a profession hold each other accountable for, and contest the proper constitution and perception of, the objects that define their professional competence.},
188 url = {http://www.jstor.org/stable/682303},
189}
190
191@inproceedings{lee2021landscape,
192 series = {CHI '21},
193 location = {Yokohama, Japan},
194 keywords = {algorithm auditing, bias detection, fairness, open source toolkits, bias, bias mitigation, algorithmic fairness, fairness toolkits},
195 numpages = {13},
196 articleno = {699},
197 booktitle = {Proceedings of the 2021 CHI Conference on Human Factors in Computing Systems},
198 abstract = {With the surge in literature focusing on the assessment and mitigation of unfair outcomes in algorithms, several open source ‘fairness toolkits’ recently emerged to make such methods widely accessible. However, little studied are the differences in approach and capabilities of existing fairness toolkits, and their fit-for-purpose in commercial contexts. Towards this, this paper identifies the gaps between the existing open source fairness toolkit capabilities and the industry practitioners’ needs. Specifically, we undertake a comparative assessment of the strengths and weaknesses of six prominent open source fairness toolkits, and investigate the current landscape and gaps in fairness toolkits through an exploratory focus group, a semi-structured interview, and an anonymous survey of data science/machine learning (ML) practitioners. We identify several gaps between the toolkits’ capabilities and practitioner needs, highlighting areas requiring attention and future directions towards tooling that better support ‘fairness in practice.’},
199 doi = {10.1145/3411764.3445261},
200 url = {https://doi.org/10.1145/3411764.3445261},
201 address = {New York, NY, USA},
202 publisher = {Association for Computing Machinery},
203 isbn = {9781450380966},
204 year = {2021},
205 title = {The Landscape and Gaps in Open Source Fairness Toolkits},
206 author = {Lee, Michelle Seng Ah and Singh, Jat},
207}
208
209@inproceedings{richardson2021towards,
210 series = {CHI '21},
211 location = {Yokohama, Japan},
212 keywords = {algorithmic bias, machine learning fairness, ML, fairness, ethics, user-centric evaluation, AI},
213 numpages = {13},
214 articleno = {236},
215 booktitle = {Proceedings of the 2021 CHI Conference on Human Factors in Computing Systems},
216 abstract = {In order to support fairness-forward thinking by machine learning (ML) practitioners, fairness researchers have created toolkits that aim to transform state-of-the-art research contributions into easily-accessible APIs. Despite these efforts, recent research indicates a disconnect between the needs of practitioners and the tools offered by fairness research. By engaging 20 ML practitioners in a simulated scenario in which they utilize fairness toolkits to make critical decisions, this work aims to utilize practitioner feedback to inform recommendations for the design and creation of fair ML toolkits. Through the use of survey and interview data, our results indicate that though fair ML toolkits are incredibly impactful on users’ decision-making, there is much to be desired in the design and demonstration of fairness results. To support the future development and evaluation of toolkits, this work offers a rubric that can be used to identify critical components of Fair ML toolkits.},
217 doi = {10.1145/3411764.3445604},
218 url = {https://doi.org/10.1145/3411764.3445604},
219 address = {New York, NY, USA},
220 publisher = {Association for Computing Machinery},
221 isbn = {9781450380966},
222 year = {2021},
223 title = {Towards Fairness in Practice: A Practitioner-Oriented Rubric for Evaluating Fair ML Toolkits},
224 author = {Richardson, Brianna and Garcia-Gathright, Jean and Way, Samuel F. and Thom, Jennifer and Cramer, Henriette},
225}
226
227@incollection{morley2021initial,
228 publisher = {Springer},
229 year = {2021},
230 pages = {153--183},
231 booktitle = {Ethics, Governance, and Policies in Artificial Intelligence},
232 author = {Morley, Jessica and Floridi, Luciano and Kinsey, Libby and Elhalal, Anat},
233 title = {From what to how: an initial review of publicly available AI ethics tools, methods and research to translate principles into practices},
234}
235
236@article{ayling2021putting,
237 doi = {10.1007/s43681-021-00084-x},
238 publisher = {Springer},
239 year = {2021},
240 pages = {405--429},
241 number = {3},
242 volume = {2},
243 journal = {AI and Ethics},
244 author = {Ayling, Jacqui and Chapman, Adriane},
245 title = {Putting AI ethics to work: are the tools fit for purpose?},
246}
247
248@inproceedings{10.1145/3375627.3377141,
249 series = {AIES '20},
250 location = {New York, NY, USA},
251 keywords = {feminist theory, work and organizations, ai ethics, sts, data work, theory, social sciences},
252 numpages = {2},
253 pages = {5–6},
254 booktitle = {Proceedings of the AAAI/ACM Conference on AI, Ethics, and Society},
255 abstract = {Recent advances in artificial intelligence applications have sparked scholarly and public attention to the challenges of the ethical design of technologies. These conversations about ethics have been targeted largely at technology designers and concerned with helping to inform building better and fairer AI tools and technologies. This approach, however, addresses only a small part of the problem of responsible use and will not be adequate for describing or redressing the problems that will arise as more types of AI technologies are more widely used.Many of the tools being developed today have potentially enormous and historic impacts on how people work, how society organises, stores and distributes information, where and how people interact with one another, and how people's work is valued and compensated. And yet, our ethical attention has looked at a fairly narrow range of questions about expanding the access to, fairness of, and accountability for existing tools. Instead, I argue that scholars should develop much broader questions of about the reconfiguration of societal power, for which AI technologies form a crucial component.This talk will argue that AI ethics needs to expand its theoretical and methodological toolkit in order to move away from prioritizing notions of good design that privilege the work of good and ethical technology designers. Instead, using approaches from feminist theory, organization studies, and science and technology, I argue for expanding how we evaluate uses of AI. This approach begins with the assumption of socially informed technological affordances, or "imagined affordances" [1] shaping how people understand and use technologies in practice. It also gives centrality to the power of social institutions for shaping technologies-in-practice.},
256 doi = {10.1145/3375627.3377141},
257 url = {https://doi.org/10.1145/3375627.3377141},
258 address = {New York, NY, USA},
259 publisher = {Association for Computing Machinery},
260 isbn = {9781450371100},
261 year = {2020},
262 title = {From Bad Users and Failed Uses to Responsible Technologies: A Call to Expand the AI Ethics Toolkit},
263 author = {Neff, Gina},
264}
265
266@article{chivukula2021surveying,
267 numpages = {32},
268 year = {2021},
269 journal = {arXiv preprint arXiv:2102.08909},
270 author = {Chivukula, Shruthi Sai and Li, Ziqing and Pivonka, Anne C and Chen, Jingning and Gray, Colin M},
271 title = {Surveying the Landscape of Ethics-Focused Design Methods},
272}
273
274@article{pierce2018differential,
275 doi = {10.1145/3274408},
276 publisher = {ACM New York, NY, USA},
277 year = {2018},
278 pages = {1--24},
279 number = {CSCW},
280 volume = {2},
281 journal = {Proceedings of the ACM on Human-Computer Interaction},
282 author = {Pierce, James and Fox, Sarah and Merrill, Nick and Wong, Richmond},
283 title = {Differential vulnerabilities and a diversity of tactics: What toolkits teach us about cybersecurity},
284}
285
286@inproceedings{sambasivan2021reimagining,
287 series = {FAccT '21},
288 location = {Virtual Event, Canada},
289 keywords = {religion, decoloniality, India, feminism, algorithmic fairness, ability, caste, gender, class, anti-caste politics, critical algorithmic studies},
290 numpages = {14},
291 pages = {315–328},
292 booktitle = {Proceedings of the 2021 ACM Conference on Fairness, Accountability, and Transparency},
293 abstract = {Conventional algorithmic fairness is West-centric, as seen in its subgroups, values, and methods. In this paper, we de-center algorithmic fairness and analyse AI power in India. Based on 36 qualitative interviews and a discourse analysis of algorithmic deployments in India, we find that several assumptions of algorithmic fairness are challenged. We find that in India, data is not always reliable due to socio-economic factors, ML makers appear to follow double standards, and AI evokes unquestioning aspiration. We contend that localising model fairness alone can be window dressing in India, where the distance between models and oppressed communities is large. Instead, we re-imagine algorithmic fairness in India and provide a roadmap to re-contextualise data and models, empower oppressed communities, and enable Fair-ML ecosystems.},
294 doi = {10.1145/3442188.3445896},
295 url = {https://doi.org/10.1145/3442188.3445896},
296 address = {New York, NY, USA},
297 publisher = {Association for Computing Machinery},
298 isbn = {9781450383097},
299 year = {2021},
300 title = {Re-Imagining Algorithmic Fairness in India and Beyond},
301 author = {Sambasivan, Nithya and Arnesen, Erin and Hutchinson, Ben and Doshi, Tulsee and Prabhakaran, Vinodkumar},
302}
303
304@article{Jobin:2019bw,
305 doi = {10.1038/s42256-019-0088-2},
306 month = {Sep},
307 volume = {1},
308 pages = {1--11},
309 year = {2019},
310 journal = {Nature Machine Intelligence},
311 title = {{The global landscape of AI ethics guidelines}},
312 author = {Jobin, Anna and Ienca, Marcello and Vayena, Effy},
313}
314
315@article{Mittelstadt:2019ve,
316 doi = {10.48550/arXiv.1906.06668},
317 year = {2019},
318 howpublished = {CoRR arXiv:1906.06668},
319 author = {Mittelstadt, Brent},
320 title = {AI Ethics--Too Principled to Fail?},
321}
322
323@article{schiff2020principles,
324 doi = {10.48550/arXiv.2006.04707},
325 year = {2020},
326 journal = {arXiv preprint arXiv:2006.04707},
327 author = {Schiff, Daniel and Rakova, Bogdana and Ayesh, Aladdin and Fanti, Anat and Lennon, Michael},
328 title = {Principles to practices for responsible AI: Closing the gap},
329}
330
331@article{metcalf2019owning,
332 publisher = {Johns Hopkins University Press},
333 year = {2019},
334 pages = {449--476},
335 number = {2},
336 volume = {86},
337 journal = {Social Research: An International Quarterly},
338 author = {Metcalf, Jacob and Moss, Emanuel and others},
339 title = {Owning Ethics: Corporate Logics, Silicon Valley, and the Institutionalization of Ethics},
340}
341
342@inproceedings{madaio2020co,
343 series = {CHI '20},
344 location = {Honolulu, HI, USA},
345 keywords = {fairness, checklists, ML, AI, co-design, ethics},
346 numpages = {14},
347 pages = {1–14},
348 booktitle = {Proceedings of the 2020 CHI Conference on Human Factors in Computing Systems},
349 abstract = {Many organizations have published principles intended to guide the ethical development and deployment of AI systems; however, their abstract nature makes them difficult to operationalize. Some organizations have therefore produced AI ethics checklists, as well as checklists for more specific concepts, such as fairness, as applied to AI systems. But unless checklists are grounded in practitioners' needs, they may be misused. To understand the role of checklists in AI ethics, we conducted an iterative co-design process with 48 practitioners, focusing on fairness. We co-designed an AI fairness checklist and identified desiderata and concerns for AI fairness checklists in general. We found that AI fairness checklists could provide organizational infrastructure for formalizing ad-hoc processes and empowering individual advocates. We highlight aspects of organizational culture that may impact the efficacy of AI fairness checklists, and suggest future design directions.},
350 doi = {10.1145/3313831.3376445},
351 url = {https://doi.org/10.1145/3313831.3376445},
352 address = {New York, NY, USA},
353 publisher = {Association for Computing Machinery},
354 isbn = {9781450367080},
355 year = {2020},
356 title = {Co-Designing Checklists to Understand Organizational Challenges and Opportunities around Fairness in AI},
357 author = {Madaio, Michael A. and Stark, Luke and Wortman Vaughan, Jennifer and Wallach, Hanna},
358}
359
360@article{rakova2021responsible,
361 doi = {10.1145/3449081},
362 publisher = {ACM New York, NY, USA},
363 year = {2021},
364 pages = {1--23},
365 number = {CSCW1},
366 volume = {5},
367 journal = {Proceedings of the ACM on Human-Computer Interaction},
368 author = {Rakova, Bogdana and Yang, Jingying and Cramer, Henriette and Chowdhury, Rumman},
369 title = {Where responsible AI meets reality: Practitioner perspectives on enablers for shifting organizational practices},
370}
371
372@article{suchman2002located,
373 year = {2002},
374 pages = {7},
375 number = {2},
376 volume = {14},
377 journal = {Scandinavian journal of information systems},
378 author = {Suchman, Lucy},
379 title = {Located accountabilities in technology production},
380}
381
382@article{wong2021tactics,
383 doi = {10.1145/3479499},
384 publisher = {ACM New York, NY, USA},
385 year = {2021},
386 pages = {1--28},
387 number = {CSCW2},
388 volume = {5},
389 journal = {Proceedings of the ACM on Human-Computer Interaction},
390 author = {Wong, Richmond Y},
391 title = {Tactics of Soft Resistance in User Experience Professionals' Values Work},
392}
393
394@article{shilton2013values,
395 doi = {10.1177/0162243912436985},
396 publisher = {Sage Publications Sage CA: Los Angeles, CA},
397 year = {2013},
398 pages = {374--397},
399 number = {3},
400 volume = {38},
401 journal = {Science, Technology, \& Human Values},
402 author = {Shilton, Katie},
403 title = {Values levers: Building ethics into design},
404}
405
406@inproceedings{neff2020bad,
407 series = {AIES '20},
408 location = {New York, NY, USA},
409 keywords = {social sciences, data work, work and organizations, feminist theory, sts, theory, ai ethics},
410 numpages = {2},
411 pages = {5–6},
412 booktitle = {Proceedings of the AAAI/ACM Conference on AI, Ethics, and Society},
413 abstract = {Recent advances in artificial intelligence applications have sparked scholarly and public attention to the challenges of the ethical design of technologies. These conversations about ethics have been targeted largely at technology designers and concerned with helping to inform building better and fairer AI tools and technologies. This approach, however, addresses only a small part of the problem of responsible use and will not be adequate for describing or redressing the problems that will arise as more types of AI technologies are more widely used.Many of the tools being developed today have potentially enormous and historic impacts on how people work, how society organises, stores and distributes information, where and how people interact with one another, and how people's work is valued and compensated. And yet, our ethical attention has looked at a fairly narrow range of questions about expanding the access to, fairness of, and accountability for existing tools. Instead, I argue that scholars should develop much broader questions of about the reconfiguration of societal power, for which AI technologies form a crucial component.This talk will argue that AI ethics needs to expand its theoretical and methodological toolkit in order to move away from prioritizing notions of good design that privilege the work of good and ethical technology designers. Instead, using approaches from feminist theory, organization studies, and science and technology, I argue for expanding how we evaluate uses of AI. This approach begins with the assumption of socially informed technological affordances, or "imagined affordances" [1] shaping how people understand and use technologies in practice. It also gives centrality to the power of social institutions for shaping technologies-in-practice.},
414 doi = {10.1145/3375627.3377141},
415 url = {https://doi.org/10.1145/3375627.3377141},
416 address = {New York, NY, USA},
417 publisher = {Association for Computing Machinery},
418 isbn = {9781450371100},
419 year = {2020},
420 title = {From Bad Users and Failed Uses to Responsible Technologies: A Call to Expand the AI Ethics Toolkit},
421 author = {Neff, Gina},
422}
423
424@article{passi2018trust,
425 keywords = {organizational work, data science, trust, credibility, collaboration},
426 numpages = {28},
427 articleno = {136},
428 month = {Nov},
429 journal = {Proc. ACM Hum.-Comput. Interact.},
430 abstract = {The trustworthiness of data science systems in applied and real-world settings emerges from the resolution of specific tensions through situated, pragmatic, and ongoing forms of work. Drawing on research in CSCW, critical data studies, and history and sociology of science, and six months of immersive ethnographic fieldwork with a corporate data science team, we describe four common tensions in applied data science work: (un)equivocal numbers, (counter)intuitive knowledge, (in)credible data, and (in)scrutable models. We show how organizational actors establish and re-negotiate trust under messy and uncertain analytic conditions through practices of skepticism, assessment, and credibility. Highlighting the collaborative and heterogeneous nature of real-world data science, we show how the management of trust in applied corporate data science settings depends not only on pre-processing and quantification, but also on negotiation and translation. We conclude by discussing the implications of our findings for data science research and practice, both within and beyond CSCW.},
431 doi = {10.1145/3274405},
432 url = {https://doi.org/10.1145/3274405},
433 number = {CSCW},
434 volume = {2},
435 address = {New York, NY, USA},
436 publisher = {Association for Computing Machinery},
437 issue_date = {November 2018},
438 year = {2018},
439 title = {Trust in Data Science: Collaboration, Translation, and Accountability in Corporate Data Science Projects},
440 author = {Passi, Samir and Jackson, Steven J.},
441}
442
443@article{passi2020making,
444 publisher = {SAGE Publications Sage UK: London, England},
445 year = {2020},
446 numpages = {13},
447 doi = {10.1177/2053951720939605},
448 number = {2},
449 volume = {7},
450 journal = {Big Data \& Society},
451 author = {Passi, Samir and Sengers, Phoebe},
452 title = {Making data science systems work},
453}
454
455@inproceedings{passi2019problem,
456 series = {FAT* '19},
457 location = {Atlanta, GA, USA},
458 keywords = {Problem Formulation, Machine Learning, Target Variable, Fairness, Data Science},
459 numpages = {10},
460 pages = {39–48},
461 booktitle = {Proceedings of the Conference on Fairness, Accountability, and Transparency},
462 abstract = {Formulating data science problems is an uncertain and difficult process. It requires various forms of discretionary work to translate high-level objectives or strategic goals into tractable problems, necessitating, among other things, the identification of appropriate target variables and proxies. While these choices are rarely self-evident, normative assessments of data science projects often take them for granted, even though different translations can raise profoundly different ethical concerns. Whether we consider a data science project fair often has as much to do with the formulation of the problem as any property of the resulting model. Building on six months of ethnographic fieldwork with a corporate data science team---and channeling ideas from sociology and history of science, critical data studies, and early writing on knowledge discovery in databases---we describe the complex set of actors and activities involved in problem formulation. Our research demonstrates that the specification and operationalization of the problem are always negotiated and elastic, and rarely worked out with explicit normative considerations in mind. In so doing, we show that careful accounts of everyday data science work can help us better understand how and why data science problems are posed in certain ways---and why specific formulations prevail in practice, even in the face of what might seem like normatively preferable alternatives. We conclude by discussing the implications of our findings, arguing that effective normative interventions will require attending to the practical work of problem formulation.},
463 doi = {10.1145/3287560.3287567},
464 url = {https://doi.org/10.1145/3287560.3287567},
465 address = {New York, NY, USA},
466 publisher = {Association for Computing Machinery},
467 isbn = {9781450361255},
468 year = {2019},
469 title = {Problem Formulation and Fairness},
470 author = {Passi, Samir and Barocas, Solon},
471}
472
473@misc{mattern_2021,
474 month = {Jul},
475 year = {2021},
476 author = {Mattern, Shannon},
477 journal = {Toolshed},
478 url = {https://tool-shed.org/unboxing-the-toolkit/},
479 title = {Unboxing the Toolkit},
480}
481
482@misc{kelty_2018,
483 month = {Jun},
484 year = {2018},
485 author = {Kelty, Christopher M},
486 journal = {Limn},
487 url = {https://limn.it/articles/the-participatory-development-toolkit/},
488 title = {The Participatory Development Toolkit},
489}
490
491@inproceedings{Holstein:2019fr,
492 series = {CHI '19},
493 location = {Glasgow, Scotland UK},
494 keywords = {needfinding, algorithmic bias, fair machine learning, ux of machine learning, product teams, empirical study},
495 numpages = {16},
496 pages = {1–16},
497 booktitle = {Proceedings of the 2019 CHI Conference on Human Factors in Computing Systems},
498 abstract = {The potential for machine learning (ML) systems to amplify social inequities and unfairness is receiving increasing popular and academic attention. A surge of recent work has focused on the development of algorithmic tools to assess and mitigate such unfairness. If these tools are to have a positive impact on industry practice, however, it is crucial that their design be informed by an understanding of real-world needs. Through 35 semi-structured interviews and an anonymous survey of 267 ML practitioners, we conduct the first systematic investigation of commercial product teams' challenges and needs for support in developing fairer ML systems. We identify areas of alignment and disconnect between the challenges faced by teams in practice and the solutions proposed in the fair ML research literature. Based on these findings, we highlight directions for future ML and HCI research that will better address practitioners' needs.},
499 doi = {10.1145/3290605.3300830},
500 url = {https://doi.org/10.1145/3290605.3300830},
501 address = {New York, NY, USA},
502 publisher = {Association for Computing Machinery},
503 isbn = {9781450359702},
504 year = {2019},
505 author = {Holstein, Kenneth and Wortman Vaughan, Jennifer and Daum{\'e} III, Hal and Dudik, Miro and Wallach, Hanna},
506 title = {Improving fairness in machine learning systems: What do industry practitioners need?},
507}
508
509@inproceedings{boyd2020ethical,
510 series = {CSCW '20 Companion},
511 location = {Virtual Event, USA},
512 keywords = {ethical sensitivity, datasets, machine learning, ethics, work practices, technology development},
513 numpages = {6},
514 pages = {87–92},
515 booktitle = {Conference Companion Publication of the 2020 on Computer Supported Cooperative Work and Social Computing},
516 abstract = {Despite a great deal of attention to developing ethical mitigations for Machine Learning (ML) training data and models, we don't yet know how these interventions will be adopted by those who curate data and use them to train ML models. Will they help ML engineers find and address ethical concerns in their work? My proposed dissertation seeks to understand ML engineers? ethical sensitivity? their propensity to notice, analyze, and act on socially impactful aspects of their work-while curating training data and describe the effects of context documents and ethical guides as practice-based ethics interventions in this early stage of ML development. It asks how ML engineers recognize,particularize, and judge ethical questions while exploring new training data; introduces Ethical Sensitivity to the study of social computing; and will describe how Datasheets intervene in perception and particularization; and will develop a document that can help engineers move from particularization to judgment. It will accomplish these goals using a think aloud experiment with engineers working with unfamiliar training data (with or without a Datasheet), a Value Sensitive Design study that aims to fit an ethical mitigation guide to engineers? work practices, and a systematic review of ethical sensitivity.},
517 doi = {10.1145/3406865.3418359},
518 url = {https://doi.org/10.1145/3406865.3418359},
519 address = {New York, NY, USA},
520 publisher = {Association for Computing Machinery},
521 isbn = {9781450380591},
522 year = {2020},
523 title = {Ethical Sensitivity in Machine Learning Development},
524 author = {Boyd, Karen},
525}
526
527@article{weaver2008ethical,
528 publisher = {Wiley Online Library},
529 year = {2008},
530 pages = {607--618},
531 number = {5},
532 volume = {62},
533 journal = {Journal of advanced nursing},
534 author = {Weaver, Kathryn and Morse, Janice and Mitcham, Carl},
535 title = {Ethical sensitivity in professional practice: concept analysis},
536}
537
538@article{hitzig2020normative,
539 publisher = {Cambridge University Press},
540 year = {2020},
541 pages = {407--434},
542 number = {3},
543 volume = {36},
544 journal = {Economics \& Philosophy},
545 author = {Hitzig, Zo{\"e}},
546 title = {The normative gap: mechanism design and ideal theories of justice},
547}
548
549@misc{friedman2002value,
550 publisher = {Citeseer},
551 year = {2002},
552 number = {2-12},
553 journal = {University of Washington technical report},
554 author = {Friedman, Batya and Kahn, Peter and Borning, Alan},
555 title = {Value sensitive design: Theory and methods},
556}
557
558@article{yoo2018stakeholder,
559 doi = {10.1007/s10676-018-9474-4},
560 publisher = {Springer},
561 year = {2021},
562 pages = {1--5},
563 journal = {Ethics and Information Technology},
564 author = {Yoo, Daisy},
565 title = {Stakeholder Tokens: a constructive method for value sensitive design stakeholder analysis},
566}
567
568@incollection{star1989structure,
569 numpages = {18},
570 pages = {37–54},
571 booktitle = {Distributed Artificial Intelligence (Vol. 2)},
572 address = {San Francisco, CA, USA},
573 publisher = {Morgan Kaufmann Publishers Inc.},
574 isbn = {0273088106},
575 year = {1989},
576 author = {Star, Susan Leigh},
577 title = {The structure of ill-structured solutions: Boundary objects and heterogeneous distributed problem solving},
578}
579
580@article{silbey2009taming,
581 publisher = {Annual Reviews},
582 year = {2009},
583 pages = {341--369},
584 volume = {35},
585 journal = {Annual Review of Sociology},
586 author = {Silbey, Susan S},
587 title = {Taming Prometheus: Talk about safety and culture},
588}
589
590@book{redfield2013life,
591 address = {Berkeley},
592 publisher = {University of California Press},
593 year = {2013},
594 author = {Redfield, Peter},
595 title = {Life in crisis},
596}
597
598@inproceedings{selbst2019fairness,
599 series = {FAT* '19},
600 location = {Atlanta, GA, USA},
601 keywords = {Interdisciplinary, Fairness-aware Machine Learning, Sociotechnical Systems},
602 numpages = {10},
603 pages = {59–68},
604 booktitle = {Proceedings of the Conference on Fairness, Accountability, and Transparency},
605 abstract = {A key goal of the fair-ML community is to develop machine-learning based systems that, once introduced into a social context, can achieve social and legal outcomes such as fairness, justice, and due process. Bedrock concepts in computer science---such as abstraction and modular design---are used to define notions of fairness and discrimination, to produce fairness-aware learning algorithms, and to intervene at different stages of a decision-making pipeline to produce "fair" outcomes. In this paper, however, we contend that these concepts render technical interventions ineffective, inaccurate, and sometimes dangerously misguided when they enter the societal context that surrounds decision-making systems. We outline this mismatch with five "traps" that fair-ML work can fall into even as it attempts to be more context-aware in comparison to traditional data science. We draw on studies of sociotechnical systems in Science and Technology Studies to explain why such traps occur and how to avoid them. Finally, we suggest ways in which technical designers can mitigate the traps through a refocusing of design in terms of process rather than solutions, and by drawing abstraction boundaries to include social actors rather than purely technical ones.},
606 doi = {10.1145/3287560.3287598},
607 url = {https://doi.org/10.1145/3287560.3287598},
608 address = {New York, NY, USA},
609 publisher = {Association for Computing Machinery},
610 isbn = {9781450361255},
611 year = {2019},
612 title = {Fairness and Abstraction in Sociotechnical Systems},
613 author = {Selbst, Andrew D. and Boyd, Danah and Friedler, Sorelle A. and Venkatasubramanian, Suresh and Vertesi, Janet},
614}
615
616@inproceedings{blodgett2020language,
617 abstract = {We survey 146 papers analyzing {``}bias{''} in NLP systems, finding that their motivations are often vague, inconsistent, and lacking in normative reasoning, despite the fact that analyzing {``}bias{''} is an inherently normative process. We further find that these papers{'} proposed quantitative techniques for measuring or mitigating {``}bias{''} are poorly matched to their motivations and do not engage with the relevant literature outside of NLP. Based on these findings, we describe the beginnings of a path forward by proposing three recommendations that should guide work analyzing {``}bias{''} in NLP systems. These recommendations rest on a greater recognition of the relationships between language and social hierarchies, encouraging researchers and practitioners to articulate their conceptualizations of {``}bias{''}---i.e., what kinds of system behaviors are harmful, in what ways, to whom, and why, as well as the normative reasoning underlying these statements{---}and to center work around the lived experiences of members of communities affected by NLP systems, while interrogating and reimagining the power relations between technologists and such communities.},
618 pages = {5454--5476},
619 doi = {10.18653/v1/2020.acl-main.485},
620 url = {https://aclanthology.org/2020.acl-main.485},
621 publisher = {Association for Computational Linguistics},
622 address = {Online},
623 year = {2020},
624 month = {July},
625 booktitle = {Proceedings of the 58th Annual Meeting of the Association for Computational Linguistics},
626 author = {Blodgett, Su Lin and
627Barocas, Solon and
628Daum{\'e} III, Hal and
629Wallach, Hanna},
630 title = {Language (Technology) is Power: A Critical Survey of {``}Bias{''} in {NLP}},
631}
632
633@article{shen2020designing,
634 doi = {10.1145/3415224},
635 publisher = {ACM New York, NY, USA},
636 year = {2020},
637 pages = {1--22},
638 number = {CSCW2},
639 volume = {4},
640 journal = {Proceedings of the ACM on Human-Computer Interaction},
641 author = {Shen, Hong and Jin, Haojian and Cabrera, {\'A}ngel Alexander and Perer, Adam and Zhu, Haiyi and Hong, Jason I},
642 title = {Designing Alternative Representations of Confusion Matrices to Support Non-Expert Public Understanding of Algorithm Performance},
643}
644
645@inproceedings{cheng2021soliciting,
646 series = {CHI '21},
647 location = {Yokohama, Japan},
648 keywords = {algorithmic fairness, human-centered AI, child welfare, algorithm-assisted decision-making, machine learning},
649 numpages = {17},
650 articleno = {390},
651 booktitle = {Proceedings of the 2021 CHI Conference on Human Factors in Computing Systems},
652 abstract = {Recent work in fair machine learning has proposed dozens of technical definitions of algorithmic fairness and methods for enforcing these definitions. However, we still lack an understanding of how to develop machine learning systems with fairness criteria that reflect relevant stakeholders’ nuanced viewpoints in real-world contexts. To address this gap, we propose a framework for eliciting stakeholders’ subjective fairness notions. Combining a user interface that allows stakeholders to examine the data and the algorithm’s predictions with an interview protocol to probe stakeholders’ thoughts while they are interacting with the interface, we can identify stakeholders’ fairness beliefs and principles. We conduct a user study to evaluate our framework in the setting of a child maltreatment predictive system. Our evaluations show that the framework allows stakeholders to comprehensively convey their fairness viewpoints. We also discuss how our results can inform the design of predictive systems.},
653 doi = {10.1145/3411764.3445308},
654 url = {https://doi.org/10.1145/3411764.3445308},
655 address = {New York, NY, USA},
656 publisher = {Association for Computing Machinery},
657 isbn = {9781450380966},
658 year = {2021},
659 title = {Soliciting Stakeholders’ Fairness Notions in Child Maltreatment Predictive Systems},
660 author = {Cheng, Hao-Fei and Stapleton, Logan and Wang, Ruiqi and Bullock, Paige and Chouldechova, Alexandra and Wu, Zhiwei Steven Steven and Zhu, Haiyi},
661}
662
663@incollection{stark2021critical,
664 publisher = {Springer},
665 year = {2021},
666 pages = {257--280},
667 booktitle = {The Cultural Life of Machine Learning},
668 author = {Stark, Luke and Greene, Daniel and Hoffmann, Anna Lauren},
669 title = {Critical Perspectives on Governance Mechanisms for AI/ML Systems},
670}
671
672@article{freire1996pedagogy,
673 year = {1996},
674 journal = {New York: Continuum},
675 author = {Freire, Paolo},
676 title = {Pedagogy of the oppressed (revised)},
677}
678
679@article{malazita2019infrastructures,
680 publisher = {Taylor \& Francis},
681 year = {2019},
682 pages = {300--312},
683 number = {4},
684 volume = {30},
685 journal = {Digital Creativity},
686 author = {Malazita, James W and Resetar, Korryn},
687 title = {Infrastructures of abstraction: how computer science education produces anti-political subjects},
688}
689
690@article{ahn2020fairsight,
691 doi = {10.1109/TVCG.2019.2934262},
692 pages = {1086-1095},
693 number = {1},
694 volume = {26},
695 year = {2020},
696 title = {FairSight: Visual Analytics for Fairness in Decision Making},
697 journal = {IEEE Transactions on Visualization and Computer Graphics},
698 author = {Ahn, Yongsu and Lin, Yu-Ru},
699}
700
701@inproceedings{metcalf2021algorithmicImpactAssessments,
702 series = {FAccT '21},
703 location = {Virtual Event, Canada},
704 keywords = {harm, impact, algorithmic impact assessment, governance, accountability},
705 numpages = {12},
706 pages = {735–746},
707 booktitle = {Proceedings of the 2021 ACM Conference on Fairness, Accountability, and Transparency},
708 abstract = {Algorithmic impact assessments (AIAs) are an emergent form of accountability for organizations that build and deploy automated decision-support systems. They are modeled after impact assessments in other domains. Our study of the history of impact assessments shows that "impacts" are an evaluative construct that enable actors to identify and ameliorate harms experienced because of a policy decision or system. Every domain has different expectations and norms around what constitutes impacts and harms, how potential harms are rendered as impacts of a particular undertaking, who is responsible for conducting such assessments, and who has the authority to act on them to demand changes to that undertaking. By examining proposals for AIAs in relation to other domains, we find that there is a distinct risk of constructing algorithmic impacts as organizationally understandable metrics that are nonetheless inappropriately distant from the harms experienced by people, and which fall short of building the relationships required for effective accountability. As impact assessments become a commonplace process for evaluating harms, the FAccT community, in its efforts to address this challenge, should A) understand impacts as objects that are co-constructed accountability relationships, B) attempt to construct impacts as close as possible to actual harms, and C) recognize that accountability governance requires the input of various types of expertise and affected communities. We conclude with lessons for assembling cross-expertise consensus for the co-construction of impacts and building robust accountability relationships.},
709 doi = {10.1145/3442188.3445935},
710 url = {https://doi.org/10.1145/3442188.3445935},
711 address = {New York, NY, USA},
712 publisher = {Association for Computing Machinery},
713 isbn = {9781450383097},
714 year = {2021},
715 title = {Algorithmic Impact Assessments and Accountability: The Co-Construction of Impacts},
716 author = {Metcalf, Jacob and Moss, Emanuel and Watkins, Elizabeth Anne and Singh, Ranjit and Elish, Madeleine Clare},
717}
718
719@article{kemp2013humanRights,
720 url = {https://doi.org/10.1080/14615517.2013.782978},
721 doi = {10.1080/14615517.2013.782978},
722 publisher = {Taylor & Francis},
723 year = {2013},
724 pages = {86-96},
725 number = {2},
726 volume = {31},
727 journal = {Impact Assessment and Project Appraisal},
728 title = {Human rights and impact assessment: clarifying the connections in practice},
729 author = {Kemp, Deanna and Vanclay, Frank},
730}
731
732@techreport{UnitedNationsHumanRights2011,
733 year = {2011},
734 title = {{Guiding Principles on Business and Human Rights: Implementing the United Nations "Protect, Respect and Remedy" Framework}},
735 institution = {United Nations},
736 file = {:C\:/Users/ryw9/Box/Papers Archive/United Nations (2011)Guiding principles on business and human rights- implementing the United Nations protect, respect and remedy framework.pdf:pdf},
737 doi = {10.4324/9781351171922-3},
738 author = {{United Nations Human Rights Office of the High Commissioner}},
739}
740
741@unpublished{Ruggie2017SocialConstructionUN,
742 year = {2017},
743 title = {{The Social Construction of the UN Guiding Principles on Business \& Human Rights}},
744 file = {:C\:/Users/ryw9/AppData/Local/Mendeley Ltd./Mendeley Desktop/Downloaded/Ruggie - 2017 - The Social Construction of the UN Guiding Principles on Business & Human Rights.pdf:pdf},
745 doi = {10.2139/ssrn.2984901},
746 booktitle = {Harvard Kennedy School Faculty Research Working Paper Series},
747 author = {Ruggie, John Gerard},
748 abstract = {Academic proponents and opponents of the UN Guiding Principles on Business & Human Rights have generated a bourgeoning literature. And by now there are several years of practical experience to inform the debate. But the conceptual and theoretical understanding of global rulemaking that informed my development of the UNGPs, and to which I have contributed as a scholar, have not been fully articulated and debated. This chapter aims to close that gap, on the supposition that those ideas might have contributed to the UNGPs' relative success where previous efforts failed, and that in some measure they may be applicable in other complex and contested global policy domains.},
749}
750
751@article{Hoffmann2020terms,
752 issue = {12},
753 volume = {23},
754 year = {2020},
755 url = {http://journals.sagepub.com/doi/10.1177/1461444820958725},
756 title = {{Terms of inclusion: Data, discourse, violence}},
757 pages = {146144482095872},
758 month = {sep},
759 journal = {New Media \& Society},
760 issn = {1461-4448},
761 file = {:C\:/Users/ryw9/AppData/Local/Mendeley Ltd./Mendeley Desktop/Downloaded/Hoffmann - 2020 - Terms of inclusion Data, discourse, violence.pdf:pdf},
762 doi = {10.1177/1461444820958725},
763 author = {Hoffmann, Anna Lauren},
764 abstract = {Inclusion has emerged as an early cornerstone value for the emerging domain of “data ethics.” On the surface, appeals to inclusion appear to address the threat that biased data technologies making decisions or misrepresenting people in ways that reproduce longer standing patterns of oppression and violence. Far from a panacea for the threats of pervasive data collection and surveillance, however, these emerging discourses of inclusion merit critical consideration. Here, I use the lens of discursive violence to better theorize the relationship between inclusion and the violent potentials of data science and technology. In doing so, I aim to articulate the problematic and often perverse power relationships implicit in ideals of “inclusion” broadly, which—if not accompanied by dramatic upheavals in existing hierarchical power structures—too often work to diffuse the radical potential of difference and normalize otherwise oppressive structural conditions.},
765}
766
767@inproceedings{Greene2019betterNicer,
768 pages = {2122-2131},
769 year = {2019},
770 url = {http://hdl.handle.net/10125/59651},
771 title = {{Better, Nicer, Clearer, Fairer: A Critical Assessment of the Movement for Ethical Artificial Intelligence and Machine Learning}},
772 file = {:C\:/Users/ryw9/AppData/Local/Mendeley Ltd./Mendeley Desktop/Downloaded/Greene, Hoffmann, Stark - 2019 - Better, Nicer, Clearer, Fairer A Critical Assessment of the Movement for Ethical Artificial Intelligenc.pdf:pdf},
773 doi = {10.24251/HICSS.2019.258},
774 booktitle = {Proceedings of the 52nd Hawaii International Conference on System Sciences},
775 author = {Greene, Daniel and Hoffmann, Anna Lauren and Stark, Luke},
776 abstract = {This paper uses frame analysis to examine recent high-profile values statements endorsing ethical design for artificial intelligence and machine learning (AI/ML). Guided by insights from values in design and the sociology of business ethics, we uncover the grounding assumptions and terms of debate that make some conversations about ethical design possible while forestalling alternative visions. Vision statements for ethical AI/ML co-opt the language of some critics, folding them into a limited, technologically deterministic, expert-driven view of what ethical AI/ML means and how it might work.},
777}
778
779@book{Scott1998seeing,
780 year = {1998},
781 title = {{Seeing Like a State: How certain schemes to improve the human condition have failed}},
782 publisher = {Yale University Press},
783 author = {Scott, James C.},
784 address = {New Haven},
785}
786
787@article{braun2006using,
788 publisher = {Taylor \& Francis},
789 year = {2006},
790 pages = {77--101},
791 number = {2},
792 volume = {3},
793 journal = {Qualitative research in psychology},
794 author = {Braun, Virginia and Clarke, Victoria},
795 title = {Using thematic analysis in psychology},
796}
797
798@book{jasanoff2015dreamscapes,
799 address = {Chicago},
800 publisher = {University of Chicago Press},
801 year = {2015},
802 author = {Jasanoff, Sheila and Kim, Sang-Hyun},
803 title = {Dreamscapes of modernity: Sociotechnical imaginaries and the fabrication of power},
804}
805
806@article{madaio2021assessing,
807 year = {2021},
808 journal = {arXiv preprint arXiv:2112.05675},
809 author = {Madaio, Michael and Egede, Lisa and Subramonyam, Hariharan and Vaughan, Jennifer Wortman and Wallach, Hanna},
810 title = {Assessing the Fairness of AI Systems: AI Practitioners' Processes, Challenges, and Needs for Support},
811}
812
813@article{shen2021everyday,
814 keywords = {auditing algorithms, everyday users, algorithmic bias, everyday algorithm auditing, fair machine learning},
815 numpages = {29},
816 articleno = {433},
817 month = {oct},
818 journal = {Proc. ACM Hum.-Comput. Interact.},
819 abstract = {A growing body of literature has proposed formal approaches to audit algorithmic systems for biased and harmful behaviors. While formal auditing approaches have been greatly impactful, they often suffer major blindspots, with critical issues surfacing only in the context of everyday use once systems are deployed. Recent years have seen many cases in which everyday users of algorithmic systems detect and raise awareness about harmful behaviors that they encounter in the course of their everyday interactions with these systems. However, to date little academic attention has been granted to these bottom-up, user-driven auditing processes. In this paper, we propose and explore the concept of everyday algorithm auditing, a process in which users detect, understand, and interrogate problematic machine behaviors via their day-to-day interactions with algorithmic systems. We argue that everyday users are powerful in surfacing problematic machine behaviors that may elude detection via more centrally-organized forms of auditing, regardless of users' knowledge about the underlying algorithms. We analyze several real-world cases of everyday algorithm auditing, drawing lessons from these cases for the design of future platforms and tools that facilitate such auditing behaviors. Finally, we discuss work that lies ahead, toward bridging the gaps between formal auditing approaches and the organic auditing behaviors that emerge in everyday use of algorithmic systems.},
820 doi = {10.1145/3479577},
821 url = {https://doi.org/10.1145/3479577},
822 number = {CSCW2},
823 volume = {5},
824 address = {New York, NY, USA},
825 publisher = {Association for Computing Machinery},
826 issue_date = {October 2021},
827 year = {2021},
828 title = {Everyday Algorithm Auditing: Understanding the Power of Everyday Users in Surfacing Harmful Algorithmic Behaviors},
829 author = {Shen, Hong and DeVos, Alicia and Eslami, Motahhare and Holstein, Kenneth},
830}
831
832@misc{ding2018deciphering,
833 pages = {1-44},
834 url = {https://www.fhi.ox.ac.uk/wp-content/uploads/Deciphering_Chinas_AI-Dream.pdf},
835 year = {2018},
836 publisher = {Future of Humanity Institute Technical Report},
837 author = {Ding, Jeffrey},
838 title = {Deciphering China’s AI dream},
839}
840
841@article{STILGOE20131568,
842 abstract = {The governance of emerging science and innovation is a major challenge for contemporary democracies. In this paper we present a framework for understanding and supporting efforts aimed at ‘responsible innovation’. The framework was developed in part through work with one of the first major research projects in the controversial area of geoengineering, funded by the UK Research Councils. We describe this case study, and how this became a location to articulate and explore four integrated dimensions of responsible innovation: anticipation, reflexivity, inclusion and responsiveness. Although the framework for responsible innovation was designed for use by the UK Research Councils and the scientific communities they support, we argue that it has more general application and relevance.},
843 keywords = {Responsible innovation, Governance, Emerging technologies, Ethics, Geoengineering},
844 author = {Jack Stilgoe and Richard Owen and Phil Macnaghten},
845 url = {https://www.sciencedirect.com/science/article/pii/S0048733313000930},
846 doi = {https://doi.org/10.1016/j.respol.2013.05.008},
847 issn = {0048-7333},
848 year = {2013},
849 pages = {1568-1580},
850 number = {9},
851 volume = {42},
852 journal = {Research Policy},
853 title = {Developing a framework for responsible innovation},
854}
855
856@inproceedings{forlizzi2013promoting,
857 pages = {1-12},
858 year = {2013},
859 volume = {13},
860 booktitle = {Proceedings of the 5th International Congress of International Association of Societies of Design Research-IASDR},
861 author = {Forlizzi, Jodi and Zimmerman, John},
862 title = {Promoting service design as a core practice in interaction design},
863}
864
865@article{lewis2018making,
866 doi = {https://doi.org/10.21428/bfafd97b},
867 publisher = {PubPub},
868 year = {2018},
869 journal = {Journal of Design and Science},
870 author = {Lewis, Jason Edward and Arista, Noelani and Pechawis, Archer and Kite, Suzanne},
871 title = {Making kin with the machines},
872}
873
874@inproceedings{Chivukula2020DimensionsUX,
875 year = {2020},
876 url = {https://dl.acm.org/doi/10.1145/3313831.3376459},
877 title = {{Dimensions of UX Practice that Shape Ethical Awareness}},
878 publisher = {ACM},
879 pages = {1--13},
880 month = {apr},
881 isbn = {9781450367080},
882 file = {:C\:/Users/ryw9/AppData/Local/Mendeley Ltd./Mendeley Desktop/Downloaded/Chivukula et al. - 2020 - Dimensions of UX Practice that Shape Ethical Awareness.pdf:pdf},
883 doi = {10.1145/3313831.3376459},
884 booktitle = {Proceedings of the 2020 CHI Conference on Human Factors in Computing Systems},
885 author = {Chivukula, Shruthi Sai and Watkins, Chris Rhys and Manocha, Rhea and Chen, Jingle and Gray, Colin M.},
886 address = {New York, NY, USA},
887 abstract = {HCI researchers are increasingly interested in describing the complexity of design practice, including ethical, organiza- tional, and societal concerns. Recent studies have identified individual practitioners as key actors in driving the design process and culture within their respective organizations, and we build upon these efforts to reveal practitioner concerns re- garding ethics on their own terms. In this paper, we report on the results of an interview study with eleven UX practitioners, capturing their experiences that highlight dimensions of de- sign practice that impact ethical awareness and action. Using a bottom-up thematic analysis, we identified five dimensions of design complexity that influence ethical outcomes and span individual, collaborative, and methodological framing of UX activity. Based on these findings, we propose a set of impli- cations for the creation of ethically-centered design methods that resonate with this complexity and inform the education of future},
888}
889
890@book{Bamberger2015PrivacyGround,
891 year = {2015},
892 title = {{Privacy on the Ground: Driving Corporate Behavior in the United States and Europe}},
893 publisher = {The MIT Press},
894 author = {Bamberger, Kenneth A. and Mulligan, Deirdre K.},
895 address = {Cambridge, Massachusetts},
896}
897
898@article{Crockett2021BuildingTrustworthy,
899 doi = {10.1109/TAI.2021.3137091},
900 pages = {1-1},
901 number = {},
902 volume = {},
903 year = {2021},
904 title = {Building Trustworthy AI Solutions: A Case for Practical Solutions for Small Businesses},
905 journal = {IEEE Transactions on Artificial Intelligence},
906 author = {Crockett, Keeley Alexandra and Gerber, Luciano and Latham, Annabel and Colyer, Edwin},
907}
908
909@techreport{Alston2019UNReportPoverty,
910 year = {2019},
911 volume = {17564},
912 url = {https://undocs.org/A/74/493},
913 title = {{Report of the Special Rapporteur on extreme poverty and human rights}},
914 pages = {1--23},
915 number = {October},
916 institution = {United Nations},
917 file = {:C\:/Users/ryw9/Box/Papers Archive/Alston (2019) Report of the Special Rapporteur on extreme poverty and.pdf:pdf},
918 booktitle = {United Nations General Assembly},
919 author = {Alston, Philip},
920 abstract = {The digital welfare state is either already a reality or emerging in many countries across the globe. In these states, systems of social protection and assistance are increasingly driven by digital data and technologies that are used to automate, predict, identify, surveil, detect, target and punish. In the present report, the irresistible attractions for Governments to move in this direction are acknowledged, but the grave risk of stumbling, zombie-like, into a digital welfare dystopia is highlighted. It is argued that big technology companies (frequently referred to as “big tech”) operate in an almost human rights-free zone, and that this is especially problematic when the private sector is taking a leading role in designing, constructing and even operating significant parts of the digital welfare state. It is recommended in the report that, instead of obsessing about fraud, cost savings, sanctions, and market -driven definitions of efficiency, the starting point should be on how welfare budgets could be transformed through technology to ensure a higher standard of living for the vulnerable and disadvantaged.},
921}
922
923@book{ahmed2012being,
924 address = {Durham, NC},
925 publisher = {Duke University Press},
926 year = {2012},
927 author = {Ahmed, Sara},
928 title = {On being included},
929}
930
931@book{Onuoha2018PeoplesGuideAI,
932 year = {2018},
933 url = {https://alliedmedia.org/resources/peoples-guide-to-ai},
934 title = {{A People's Guide to AI}},
935 publisher = {Allied Media Projects},
936 file = {:C\:/Users/ryw9/Box/Papers Archive/Onuoha, Nucera (2018) People's Guide to AI.pdf:pdf},
937 author = {Onuoha, Mim and Nucera, Diana},
938}
939
940@article{Abdurahman2021Body,
941 year = {2021},
942 url = {https://logicmag.io/beacons/a-body-of-work-that-cannot-be-ignored/},
943 title = {{A Body of Work That Cannot Be Ignored}},
944 number = {15: Beacons},
945 journal = {Logic},
946 file = {:C\:/Users/ryw9/Box/Papers Archive/Abdurahman (2021) A Body of Work That Cannot Be Ignored.pdf:pdf},
947 author = {Abdurahman, J Khadijah},
948}
949
950@misc{Raval2021NewAILexicon,
951 year = {2021},
952 urldate = {2022-01-07},
953 url = {https://medium.com/a-new-ai-lexicon/a-new-ai-lexicon-responses-and-challenges-to-the-critical-ai-discourse-f2275989fa62},
954 title = {{A New AI Lexicon: Responses and Challenges to the Critical AI discourse}},
955 file = {:C\:/Users/ryw9/Box/Papers Archive/Raval, Kak (2021) A New AI Lexicon_ Responses and Challenges to the Critical AI discourse _ by AI Now Institute _ A New AI Lexicon _ Medium.pdf:pdf},
956 booktitle = {AI Now Institute},
957 author = {Raval, Noopur and Kak, Amba},
958}
959
960@misc{Ozoma2021TechWorkerHandbook,
961 year = {2021},
962 urldate = {2022-01-07},
963 url = {https://techworkerhandbook.org/},
964 title = {{The Tech Worker Handbook}},
965 booktitle = {The Tech Worker Handbook},
966 author = {Ozoma, Ifeoma},
967}
968
969@misc{LittleSis2017MapThePower,
970 year = {2017},
971 urldate = {2022-01-07},
972 url = {https://littlesis.org/toolkit},
973 title = {{Map the Power Toolkit}},
974 author = {LittleSis},
975}
976
977@inproceedings{mitchell2019model,
978 series = {FAT* '19},
979 location = {Atlanta, GA, USA},
980 keywords = {disaggregated evaluation, fairness evaluation, ethical considerations, ML model evaluation, model cards, documentation, datasheets},
981 numpages = {10},
982 pages = {220–229},
983 booktitle = {Proceedings of the Conference on Fairness, Accountability, and Transparency},
984 abstract = {Trained machine learning models are increasingly used to perform high-impact tasks in areas such as law enforcement, medicine, education, and employment. In order to clarify the intended use cases of machine learning models and minimize their usage in contexts for which they are not well suited, we recommend that released models be accompanied by documentation detailing their performance characteristics. In this paper, we propose a framework that we call model cards, to encourage such transparent model reporting. Model cards are short documents accompanying trained machine learning models that provide benchmarked evaluation in a variety of conditions, such as across different cultural, demographic, or phenotypic groups (e.g., race, geographic location, sex, Fitzpatrick skin type [15]) and intersectional groups (e.g., age and race, or sex and Fitzpatrick skin type) that are relevant to the intended application domains. Model cards also disclose the context in which models are intended to be used, details of the performance evaluation procedures, and other relevant information. While we focus primarily on human-centered machine learning models in the application fields of computer vision and natural language processing, this framework can be used to document any trained machine learning model. To solidify the concept, we provide cards for two supervised models: One trained to detect smiling faces in images, and one trained to detect toxic comments in text. We propose model cards as a step towards the responsible democratization of machine learning and related artificial intelligence technology, increasing transparency into how well artificial intelligence technology works. We hope this work encourages those releasing trained machine learning models to accompany model releases with similar detailed evaluation numbers and other relevant documentation.},
985 doi = {10.1145/3287560.3287596},
986 url = {https://doi.org/10.1145/3287560.3287596},
987 address = {New York, NY, USA},
988 publisher = {Association for Computing Machinery},
989 isbn = {9781450361255},
990 year = {2019},
991 title = {Model Cards for Model Reporting},
992 author = {Mitchell, Margaret and Wu, Simone and Zaldivar, Andrew and Barnes, Parker and Vasserman, Lucy and Hutchinson, Ben and Spitzer, Elena and Raji, Inioluwa Deborah and Gebru, Timnit},
993}
994
995@article{gebru2021datasheets,
996 doi = {10.1145/3458723},
997 publisher = {ACM New York, NY, USA},
998 year = {2021},
999 pages = {86--92},
1000 number = {12},
1001 volume = {64},
1002 journal = {Communications of the ACM},
1003 author = {Gebru, Timnit and Morgenstern, Jamie and Vecchione, Briana and Vaughan, Jennifer Wortman and Wallach, Hanna and Iii, Hal Daum{\'e} and Crawford, Kate},
1004 title = {Datasheets for datasets},
1005}
1006
1007@inproceedings{jacobs2021measurement,
1008 series = {FAccT '21},
1009 location = {Virtual Event, Canada},
1010 keywords = {construct reliability, construct validity, measurement, fairness},
1011 numpages = {11},
1012 pages = {375–385},
1013 booktitle = {Proceedings of the 2021 ACM Conference on Fairness, Accountability, and Transparency},
1014 abstract = {We propose measurement modeling from the quantitative social sciences as a framework for understanding fairness in computational systems. Computational systems often involve unobservable theoretical constructs, such as socioeconomic status, teacher effectiveness, and risk of recidivism. Such constructs cannot be measured directly and must instead be inferred from measurements of observable properties (and other unobservable theoretical constructs) thought to be related to them---i.e., operationalized via a measurement model. This process, which necessarily involves making assumptions, introduces the potential for mismatches between the theoretical understanding of the construct purported to be measured and its operationalization. We argue that many of the harms discussed in the literature on fairness in computational systems are direct results of such mismatches. We show how some of these harms could have been anticipated and, in some cases, mitigated if viewed through the lens of measurement modeling. To do this, we contribute fairness-oriented conceptualizations of construct reliability and construct validity that unite traditions from political science, education, and psychology and provide a set of tools for making explicit and testing assumptions about constructs and their operationalizations. We then turn to fairness itself, an essentially contested construct that has different theoretical understandings in different contexts. We argue that this contestedness underlies recent debates about fairness definitions: although these debates appear to be about different operationalizations, they are, in fact, debates about different theoretical understandings of fairness. We show how measurement modeling can provide a framework for getting to the core of these debates.},
1015 doi = {10.1145/3442188.3445901},
1016 url = {https://doi.org/10.1145/3442188.3445901},
1017 address = {New York, NY, USA},
1018 publisher = {Association for Computing Machinery},
1019 isbn = {9781450383097},
1020 year = {2021},
1021 title = {Measurement and Fairness},
1022 author = {Jacobs, Abigail Z. and Wallach, Hanna},
1023}
1024
1025@article{delgado2021stakeholder,
1026 numpages = {7},
1027 year = {2021},
1028 journal = {arXiv preprint arXiv:2111.01122},
1029 author = {Delgado, Fernando and Yang, Stephen and Madaio, Michael and Yang, Qian},
1030 title = {Stakeholder Participation in AI: Beyond" Add Diverse Stakeholders and Stir"},
1031}
1032
1033@article{sloane2020participation,
1034 series = {EAAMO '22},
1035 location = {Arlington, VA, USA},
1036 keywords = {machine learning, participatory methods, design},
1037 numpages = {6},
1038 articleno = {1},
1039 booktitle = {Equity and Access in Algorithms, Mechanisms, and Optimization},
1040 abstract = {This paper critiques popular modes of participation in design practice and machine learning. It examines three existing kinds of participation in design practice and machine learning participation as work, participation as consultation, and as participation as justice – to argue that the machine learning community must become attuned to possibly exploitative and extractive forms of community involvement and shift away from the prerogatives of context independent scalability. Cautioning against “participation washing”, it argues that the notion of “participation” should be expanded to acknowledge more subtle, and possibly exploitative, forms of community involvement in participatory machine learning design. Specifically, it suggests that it is imperative to recognize design participation as work; to ensure that participation as consultation is context-specific; and that participation as justice must be genuine and long term. The paper argues that such a development can only be scaffolded by a new epistemology around design harms, including, but not limited to, in machine learning. To facilitate such a development, the paper suggests developing we argue that developing a cross-sectoral database of design participation failures that is cross-referenced with socio-structural dimensions and highlights “edge cases” that can and must be learned from.},
1041 doi = {10.1145/3551624.3555285},
1042 url = {https://doi.org/10.1145/3551624.3555285},
1043 address = {New York, NY, USA},
1044 publisher = {Association for Computing Machinery},
1045 isbn = {9781450394772},
1046 year = {2022},
1047 title = {Participation Is Not a Design Fix for Machine Learning},
1048 author = {Sloane, Mona and Moss, Emanuel and Awomolo, Olaitan and Forlano, Laura},
1049}
1050
1051@article{madaio2022assessing,
1052 doi = {10.1145/3512899},
1053 publisher = {ACM New York, NY, USA},
1054 year = {2022},
1055 pages = {1--26},
1056 number = {CSCW1},
1057 volume = {6},
1058 journal = {Proceedings of the ACM on Human-Computer Interaction},
1059 author = {Madaio, Michael and Egede, Lisa and Subramonyam, Hariharan and Wortman Vaughan, Jennifer and Wallach, Hanna},
1060 title = {Assessing the Fairness of AI Systems: AI Practitioners' Processes, Challenges, and Needs for Support},
1061}
1062
1063@inproceedings{deng2022exploring,
1064 series = {FAccT '22},
1065 location = {Seoul, Republic of Korea},
1066 numpages = {12},
1067 pages = {473–484},
1068 booktitle = {2022 ACM Conference on Fairness, Accountability, and Transparency},
1069 abstract = {Recent years have seen the development of many open-source ML fairness toolkits aimed at helping ML practitioners assess and address unfairness in their systems. However, there has been little research investigating how ML practitioners actually use these toolkits in practice. In this paper, we conducted the first in-depth empirical exploration of how industry practitioners (try to) work with existing fairness toolkits. In particular, we conducted think-aloud interviews to understand how participants learn about and use fairness toolkits, and explored the generality of our findings through an anonymous online survey. We identified several opportunities for fairness toolkits to better address practitioner needs and scaffold them in using toolkits effectively and responsibly. Based on these findings, we highlight implications for the design of future open-source fairness toolkits that can support practitioners in better contextualizing, communicating, and collaborating around ML fairness efforts.},
1070 doi = {10.1145/3531146.3533113},
1071 url = {https://doi.org/10.1145/3531146.3533113},
1072 address = {New York, NY, USA},
1073 publisher = {Association for Computing Machinery},
1074 isbn = {9781450393522},
1075 year = {2022},
1076 title = {Exploring How Machine Learning Practitioners (Try To) Use Fairness Toolkits},
1077 author = {Deng, Wesley Hanwen and Nagireddy, Manish and Lee, Michelle Seng Ah and Singh, Jatinder and Wu, Zhiwei Steven and Holstein, Kenneth and Zhu, Haiyi},
1078}
1079
1080@article{shilton2018values,
1081 doi = {10.1561/1100000073},
1082 publisher = {Now Publishers, Inc.},
1083 year = {2018},
1084 pages = {107--171},
1085 number = {2},
1086 volume = {12},
1087 journal = {Foundations and Trends{\textregistered} in Human--Computer Interaction},
1088 author = {Shilton, Katie},
1089 title = {Values and ethics in human-computer interaction},
1090}
1091
1092@inproceedings{shen2022model,
1093 series = {FAccT '22},
1094 location = {Seoul, Republic of Korea},
1095 numpages = {12},
1096 pages = {440–451},
1097 booktitle = {2022 ACM Conference on Fairness, Accountability, and Transparency},
1098 abstract = {There have been increasing calls for centering impacted communities – both online and offline – in the design of the AI systems that will be deployed in their communities. However, the complicated nature of a community’s goals and needs, as well as the complexity of AI’s development procedures, outputs, and potential impacts, often prevents effective participation. In this paper, we present the Model Card Authoring Toolkit, a toolkit that supports community members to understand, navigate and negotiate a spectrum of machine learning models via deliberation and pick the ones that best align with their collective values. Through a series of workshops, we conduct an empirical investigation of the initial effectiveness of our approach in two online communities – English and Dutch Wikipedia, and document how our participants collectively set the threshold for a machine learning based quality prediction system used in their communities’ content moderation applications. Our results suggest that the use of the Model Card Authoring Toolkit helps improve the understanding of the trade-offs across multiple community goals on AI design, engage community members to discuss and negotiate the trade-offs, and facilitate collective and informed decision-making in their own community contexts. Finally, we discuss the challenges for a community-centered, deliberation-driven approach for AI design as well as potential design implications.},
1099 doi = {10.1145/3531146.3533110},
1100 url = {https://doi.org/10.1145/3531146.3533110},
1101 address = {New York, NY, USA},
1102 publisher = {Association for Computing Machinery},
1103 isbn = {9781450393522},
1104 year = {2022},
1105 title = {The Model Card Authoring Toolkit: Toward Community-Centered, Deliberation-Driven AI Design},
1106 author = {Shen, Hong and Wang, Leijie and Deng, Wesley H. and Brusse, Ciell and Velgersdijk, Ronald and Zhu, Haiyi},
1107}
1108
1109@article{watkins2022four,
1110 year = {2022},
1111 journal = {arXiv preprint arXiv:2202.09519},
1112 author = {Watkins, Elizabeth Anne and McKenna, Michael and Chen, Jiahao},
1113 title = {The four-fifths rule is not disparate impact: a woeful tale of epistemic trespassing in algorithmic fairness},
1114}
1115
1116@book{gray2019ghost,
1117 address = {Boston},
1118 publisher = {Houghton Mifflin Harcourt},
1119 year = {2019},
1120 author = {Gray, Mary L and Suri, Siddharth},
1121 title = {Ghost work: How to stop Silicon Valley from building a new global underclass},
1122}
1123
1124@inproceedings{bray2022radical,
1125 series = {CHI '22},
1126 location = {New Orleans, LA, USA},
1127 keywords = {participatory design, method, qualitative methods, design research methods, design methods},
1128 numpages = {13},
1129 articleno = {452},
1130 booktitle = {Proceedings of the 2022 CHI Conference on Human Factors in Computing Systems},
1131 abstract = {When considering the democratic intentions of co-design, designers and design researchers must evaluate the impact of power imbalances embedded in common design and research dynamics. This holds particularly true in work with and for marginalized communities, who are frequently excluded in design processes. To address this issue, we examine how existing design tools and methods are used to support communities in processes of community building or reimagining, considering the influence of race and identity. This paper describes our findings from 27 interviews with community design practitioners conducted to evaluate the Building Utopia toolkit, which employs an Afrofuturist lens for speculative design processes. Our research findings support the importance of design tools that prompt conversations on race in design, and tensions between the desire for imaginative design practice and the immediacy of social issues, particularly when designing with Black and brown communities.},
1132 doi = {10.1145/3491102.3501945},
1133 url = {https://doi.org/10.1145/3491102.3501945},
1134 address = {New York, NY, USA},
1135 publisher = {Association for Computing Machinery},
1136 isbn = {9781450391573},
1137 year = {2022},
1138 title = {Radical Futures: Supporting Community-Led Design Engagements through an Afrofuturist Speculative Design Toolkit},
1139 author = {Bray, Kirsten E and Harrington, Christina and Parker, Andrea G and Diakhate, N'Deye and Roberts, Jennifer},
1140}
1141
1142@inproceedings{wong2020beyondchecklists,
1143 year = {2020},
1144 url = {https://dl.acm.org/doi/10.1145/3406865.3418590},
1145 title = {{Beyond Checklist Approaches to Ethics in Design}},
1146 publisher = {ACM},
1147 pages = {511--517},
1148 month = {oct},
1149 isbn = {9781450380591},
1150 file = {:C\:/Users/ryw9/Box/Papers Archive/Wong et al (2020) Beyond check list approaches to ethics in design.pdf:pdf},
1151 doi = {10.1145/3406865.3418590},
1152 booktitle = {Conference Companion Publication of the 2020 on Computer Supported Cooperative Work and Social Computing},
1153 author = {Wong, Richmond Y and Boyd, Karen and Metcalf, Jake and Shilton, Katie},
1154 address = {New York, NY, USA},
1155}
1156
1157@inproceedings{luger2015playing,
1158 year = {2015},
1159 url = {http://dx.doi.org/10.1145/2702123.2702142 http://dl.acm.org/citation.cfm?doid=2702123.2702142},
1160 title = {{Playing the Legal Card: Using Ideation Cards to Raise Data Protection Issues within the Design Process}},
1161 publisher = {ACM Press},
1162 pages = {457--466},
1163 isbn = {9781450331456},
1164 file = {:C\:/Users/ryw9/AppData/Local/Mendeley Ltd./Mendeley Desktop/Downloaded/Luger et al. - 2015 - Playing the Legal Card Using Ideation Cards to Raise Data Protection Issues within the Design Process.pdf:pdf},
1165 doi = {10.1145/2702123.2702142},
1166 booktitle = {Proceedings of the 33rd Annual ACM Conference on Human Factors in Computing Systems - CHI '15},
1167 author = {Luger, Ewa and Urquhart, Lachlan and Rodden, Tom and Golembewski, Michael},
1168 address = {New York, New York, USA},
1169}
1170
1171@article{shilton2020rolepplaying,
1172 year = {2020},
1173 url = {http://link.springer.com/10.1007/s11948-020-00250-0},
1174 title = {{Role-Playing Computer Ethics: Designing and Evaluating the Privacy by Design (PbD) Simulation}},
1175 month = {Jul},
1176 journal = {Science and Engineering Ethics},
1177 issn = {1353-3452},
1178 doi = {10.1007/s11948-020-00250-0},
1179 author = {Shilton, Katie and Heidenblad, Donal and Porter, Adam and Winter, Susan and Kendig, Mary},
1180}
1181
1182@incollection{flanagan2014values,
1183 year = {2014},
1184 title = {{Groundwork for Values in Games}},
1185 publisher = {MIT Press},
1186 chapter = {1},
1187 booktitle = {Values at Play in Digital Games},
1188 author = {Flanagan, Mary and Nissenbaum, Helen},
1189 address = {Cambridge, Massachusetts},
1190}
1191
1192@article{boyd2021datasheets,
1193 year = {2021},
1194 volume = {5},
1195 url = {https://dl.acm.org/doi/10.1145/3479582},
1196 title = {{Datasheets for Datasets help ML Engineers Notice and Understand Ethical Issues in Training Data}},
1197 publisher = {Association for Computing Machinery},
1198 pages = {1--27},
1199 number = {CSCW2},
1200 month = {oct},
1201 keywords = {development practices,ethical sensitivity,ethics,machine learning,training data},
1202 journal = {Proceedings of the ACM on Human-Computer Interaction},
1203 issn = {2573-0142},
1204 file = {:C\:/Users/ryw9/Box/Papers Archive/Boyd (2021) Datasheets for datasets help ML engineers notice and understand ethical issues in training data.pdf:pdf},
1205 doi = {10.1145/3479582},
1206 author = {Boyd, Karen L},
1207}
1208
1209@book{bowker1999sorting,
1210 address = {Cambridge, MA},
1211 publisher = {MIT Press},
1212 year = {1999},
1213 volume = {4},
1214 journal = {Classification and its consequences},
1215 author = {Bowker, Geoffrey and Star, Susan Leigh},
1216 title = {Sorting things out},
1217}
1218
1219@article{hoffmann2019wherefairness,
1220 file = {Hoffmann - 2019 - Where fairness fails data, algorithms, and the li.pdf:C\:\\Users\\ryw9\\Zotero\\storage\\ZE5HZ5RX\\Hoffmann - 2019 - Where fairness fails data, algorithms, and the li.pdf:application/pdf},
1221 pages = {900--915},
1222 year = {2019},
1223 month = {June},
1224 author = {Hoffmann, Anna Lauren},
1225 journal = {Information, Communication \& Society},
1226 urldate = {2022-10-14},
1227 number = {7},
1228 language = {en},
1229 doi = {10.1080/1369118X.2019.1573912},
1230 url = {https://www.tandfonline.com/doi/full/10.1080/1369118X.2019.1573912},
1231 shorttitle = {Where fairness fails},
1232 issn = {1369-118X, 1468-4462},
1233 volume = {22},
1234 title = {Where fairness fails: data, algorithms, and the limits of antidiscrimination discourse},
1235}
1236
1237@article{green2021datascience,
1238 file = {Full Text:C\:\\Users\\ryw9\\Zotero\\storage\\LTBL88EB\\Green - 2021 - Data Science as Political Action Grounding Data S.pdf:application/pdf},
1239 pages = {249--265},
1240 year = {2021},
1241 month = {September},
1242 author = {Green, Ben},
1243 journal = {Journal of Social Computing},
1244 urldate = {2022-10-14},
1245 number = {3},
1246 doi = {10.23919/JSC.2021.0029},
1247 url = {https://ieeexplore.ieee.org/document/9684742/},
1248 shorttitle = {Data {Science} as {Political} {Action}},
1249 issn = {2688-5255},
1250 volume = {2},
1251 title = {Data {Science} as {Political} {Action}: {Grounding} {Data} {Science} in a {Politics} of {Justice}},
1252}
1253
1254@inproceedings{bietti2020ethicswashing,
1255 series = {FAT* '20},
1256 location = {Barcelona, Spain},
1257 keywords = {ethics, technology ethics, technology law, AI, moral philosophy, self-regulation, regulation},
1258 numpages = {10},
1259 pages = {210–219},
1260 booktitle = {Proceedings of the 2020 Conference on Fairness, Accountability, and Transparency},
1261 abstract = {The word 'ethics' is under siege in technology policy circles. Weaponized in support of deregulation, self-regulation or handsoff governance, "ethics" is increasingly identified with technology companies' self-regulatory efforts and with shallow appearances of ethical behavior. So-called "ethics washing" by tech companies is on the rise, prompting criticism and scrutiny from scholars and the tech community at large. In parallel to the growth of ethics washing, its condemnation has led to a tendency to engage in "ethics bashing." This consists in the trivialization of ethics and moral philosophy now understood as discrete tools or pre-formed social structures such as ethics boards, self-governance schemes or stakeholder groups.The misunderstandings underlying ethics bashing are at least threefold: (a) philosophy and "ethics" are seen as a communications strategy and as a form of instrumentalized cover-up or fa\c{c}ade for unethical behavior, (b) philosophy is understood in opposition and as alternative to political representation and social organizing and (c) the role and importance of moral philosophy is downplayed and portrayed as mere "ivory tower" intellectualization of complex problems that need to be dealt with in practice.This paper argues that the rhetoric of ethics and morality should not be reductively instrumentalized, either by the industry in the form of "ethics washing," or by scholars and policy-makers in the form of "ethics bashing." Grappling with the role of philosophy and ethics requires moving beyond both tendencies and seeing ethics as a mode of inquiry that facilitates the evaluation of competing tech policy strategies. In other words, we must resist narrow reductivism of moral philosophy as instrumentalized performance and renew our faith in its intrinsic moral value as a mode of knowledgeseeking and inquiry. Far from mandating a self-regulatory scheme or a given governance structure, moral philosophy in fact facilitates the questioning and reconsideration of any given practice, situating it within a complex web of legal, political and economic institutions. Moral philosophy indeed can shed new light on human practices by adding needed perspective, explaining the relationship between technology and other worthy goals, situating technology within the human, the social, the political. It has become urgent to start considering technology ethics also from within and not only from outside of ethics.},
1262 doi = {10.1145/3351095.3372860},
1263 url = {https://doi.org/10.1145/3351095.3372860},
1264 address = {New York, NY, USA},
1265 publisher = {Association for Computing Machinery},
1266 isbn = {9781450369367},
1267 year = {2020},
1268 title = {From Ethics Washing to Ethics Bashing: A View on Tech Ethics from within Moral Philosophy},
1269 author = {Bietti, Elettra},
1270}
1271
1272@inproceedings{mcmillan2019againstethical,
1273 series = {HTTF 2019},
1274 location = {Nottingham, United Kingdom},
1275 keywords = {Ethics, Artificial Intelligence, Policy, Human Rights, Algorithms},
1276 numpages = {3},
1277 articleno = {9},
1278 booktitle = {Proceedings of the Halfway to the Future Symposium 2019},
1279 abstract = {In this paper we use the EU guidelines on ethical AI, and the responses to it, as a starting point to discuss the problems with our community’s focus on such manifestos, principles, and sets of guidelines. We cover how industry and academia are at times complicit in ‘Ethics Washing’, how developing guidelines carries the risk of diluting our rights in practice, and downplaying the role of our own self interest. We conclude by discussing briefly the role of technical practice in ethics.},
1280 doi = {10.1145/3363384.3363393},
1281 url = {https://doi.org/10.1145/3363384.3363393},
1282 address = {New York, NY, USA},
1283 publisher = {Association for Computing Machinery},
1284 isbn = {9781450372039},
1285 year = {2019},
1286 title = {Against Ethical AI},
1287 author = {McMillan, Donald and Brown, Barry},
1288}
Attribution
arXiv:2202.08792v2
[cs.CY]
License: cc-by-4.0