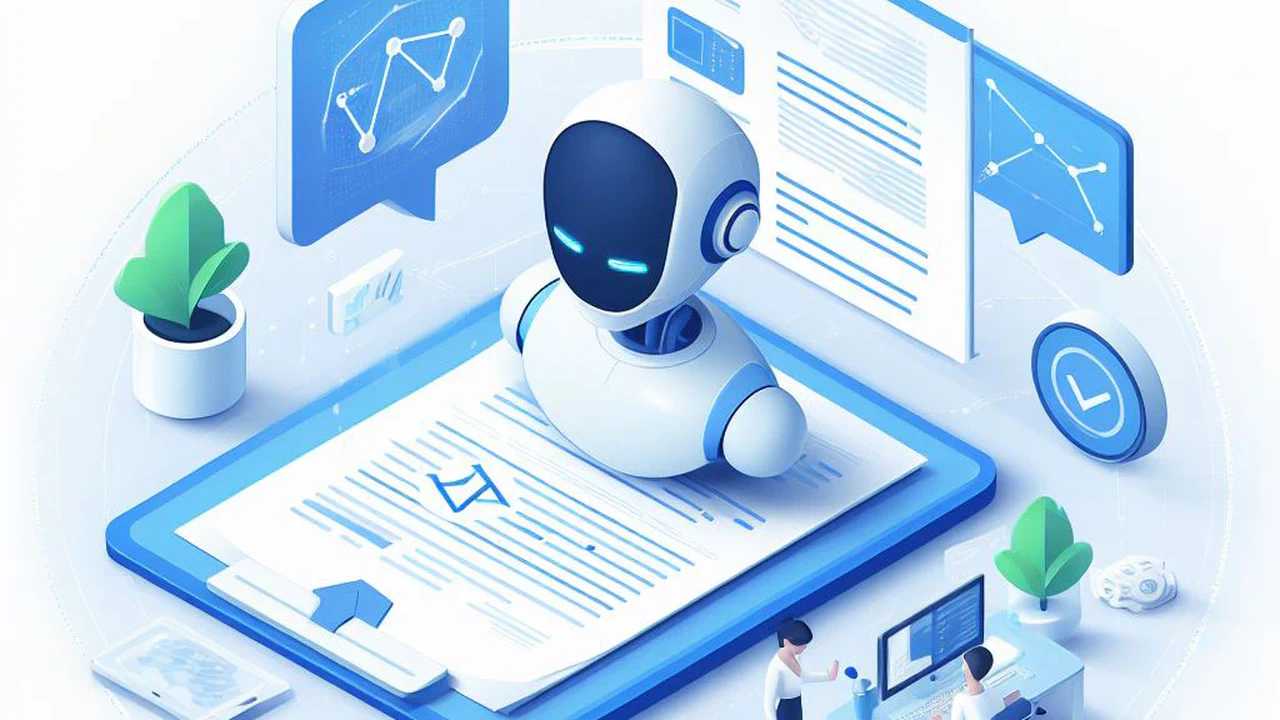
Papers is Alpha. This content is part of an effort to make research more accessible, and (most likely) has lost some details from the original. You can find the original paper here.
Introduction
As one of over 20,000 cases falsely flagged for unemployment benefit fraud by Michigan’s MIDAS algorithm, Brian Russell had to file for bankruptcy, undermining his ability to provide for his two young children. The state finally cleared him of the false charges two years later. RealPage, one of several automated tenant screening tools producing “cheap and fast—but not necessarily accurate—reports for an estimated nine out of 10 landlords across the country”, flagged Davone Jackson with a false arrest record, pushing him out of low income housing and into a small motel room with his 9-year-old daughter for nearly a year. Josiah Elleston-Burrell had his post-secondary admissions potentially revoked, Robert Williams was wrongfully arrested for a false facial recognition match, Tammy Dobbs lost critical access to healthcare benefits. The repercussions of AI-related functionality failures in high stakes scenarios cannot be overstated, and the impact reverberates in real lives for weeks, months and even years.
Despite the current public fervor over the great potential of AI, many deployed algorithmic products do not work. AI-enabled moderation tools regularly flag safe content, teacher assessment tools mark star instructors to be fired, hospital bed assignment algorithms prioritize healthy over sick patients, andmedical insurance service distribution and pricing systems gatekeep necessary care-taking resources. Deployed AI-enabled clinical support tools misallocate prescriptions, misread medical images, and misdiagnose. The New York MTA’s pilot of facial recognition had a reported 100% error rate, yet the program moved forward anyway. Some of these failures have already proven to disproportionately impact some more than others: moderation tool glitches target minoritized groups; facial recognition tools fail on darker skinned female faces; a hospital resource allocation algorithm’s misjudgements will mostly impact Black and lower income patients. However, all failures in sum reveal a broader pattern of a market saturated with dysfunctional, deployed AI products.
Importantly, the hype is not limited to AI’s boosters in corporations and the technology press; scholars and policymakers often assume functionality while discussing the dangers of algorithmic systems as well. In fact, many of the current critiques, policy positions and interventions in algorithmic accountability implicitly begin from thepremise that such deployed algorithmic systems work, echoing narratives of super-human ability, broad applicability, and consistency, espoused in corporate marketing materials, academic research papers and in mainstream media. These proposals thus often fall short of acknowledging the functionality issues in AI deployments and the role of the lack of functional safety in contributing to the harm perpetuated by these systems. The myth of functionality is one held dearly by corporate stakeholders and their investors. If a product works, we can weigh its costs and benefits. But if the product does not work, the judgment is no longer a matter of pros and cons, but a much simpler calculation, exposing that this product does not deserve its spot on the market. Although notions of accuracy and product expectations are stakeholder-dependent and can be contested, the assessment of such claims are often easier to empirically measure, grounding the discussion of harm in a way that is challenging to repudiate.
As an overlooked aspect of AI policy, functionality is often presented as a consideration secondary to other ethical challenges. In this paper, we argue that it is a primary concern that often precedes such problems. We start by calling out what we perceive to be a functionality assumption, prevalent in much of the discourse on AI risks. We then argue that this assumption does not hold in a large set of cases. Drawing on the AI, Algorithmic and Automation Incident and Controversy Repository (AAAIRC), we offer a taxonomy of the ways in which such failures can take form and the harms they cause, which differ from the more commonly cited critiques of AI. We then discuss the existing accountability tools to address functionality issues, that are often overlooked in AI policy literature and in practice, due in large part to this assumption of functionality.
Related Work
A review of past work demonstrates that although there is some acknowledgement that AI has a functionality problem, little has been done to systematically discuss the range of problems specifically associated with functionality. Recent work details that the AI research field suffers from scientific validity and evaluation problems.have demonstrated reproducibility failures in published work on predicting civil wars.found that advances in machine learning often “evaporate under closer scrutiny or turn out to be less widely applicable than originally hoped.”
There is also some work demonstrating that AI products are challenging to engineer correctly in practice. In a survey of practitioners,describe how developers often modify traditional software engineering practices due to unique challenges presented by ML, such as the increased effort required for testing and defining requirements. They also found that ML practitioners “tend to communicate less frequently with clients” and struggle to make accurate plans for the tasks required in the development process.have additionally argued that ML systems “have a special capacity for incurring technical debt.”
Other papers discuss how the AI label lends itself to inflated claims of functionality that the systems cannot meet.andcritique hyped narratives pushed in the AI industry, joined by many similar domain-specific critiques.recently popularized the metaphor of “snake oil” as a description of such AI products, raising concerns about the hyperbolic claims now common on the market today.has noted that despite the “intelligent” label, many deployed AI systems used by public agencies involve simple models defined by manually crafted heuristics. Similarly,argue that AI makes claims to generality while modeling behaviour that is determined by highly constrained and context-specific data. In a study of actual AI policy discussions,found that policymakers often define AI with respect to how human-like a system is, and concluded that this could lead to deprioritizing issues more grounded in reality.
Finally,has argued that even critics of technology often hype the very technologies that they critique, as a way of inflating the perception of their dangers. He refers to this phenomenon as “criti-hype”—criticism which both needs and feeds on hype. As an example, he points to disinformation researchers, who embrace corporate talking points of a recommendation model that can meaningfully influence consumer behavior to the point of controlling their purchases or voting activity—when in actuality, these algorithms have little ability to do either. Even the infamous Cambridge Analytica product was revealed to be “barely better than chance at applying the right [personality] scores to individuals”, and the company accused explicitly of “selling snake oil”.
The Functionality Assumption
It is unsurprising that promoters of AI do not tend to question its functionality. More surprising is the prevalence of criti-hype in the scholarship and political narratives around automation and machine learning—even amidst discussion of valid concerns such as trustworthiness, democratization, fairness, interpretability, and safety. These fears, though legitimate, are often premature “wishful worries”—fears that can only be realized once the technology works, or works “too well”, rather than being grounded in a reality where these systems do not always function as expected. In this section, we discuss how criti-hype in AImanifests as an unspoken assumption of functionality.
The functionality of AI systems is rarely explicitly mentioned in AI principle statements, policy proposals and AI ethics guidelines. In a recent review of the landscape of AI ethics guidelines,found that few acknowledge the possibility of AI not working as advertised. In guidelines about preventing malfeasance, the primary concern is malicious use of supposedly functional AI products by nefarious actors. Guidelines around “trust” are geared towards eliciting trust in AI systems from users or the public, implying that trusting these AI products would be to the benefit of these stakeholders and allow AI to “fulfill its world changing potential’’. Just one guideline of the hundreds reviewed in the survey “explicitly suggests that, instead of demanding understandability, it should be ensured that AI fulfills public expectations’’. Similarly, the U.S. National Institute of Standards and Technology (NIST) seeks to define “trustworthiness” based primarily on how much people are willing to use the AI systems they are interacting with. This framing puts the onus on people to trust in systems, and not on institutions to make their systems reliably operational, in order to earn that trust. NIST’s concept of trust is also limited, citing the “dependability” section of ISO/IEEE/IEC standards, but leaving out other critical concepts in these dependability engineering standards that represent basic functionality requirements, including assurance, claim veracity, integrity level, systematic failure, or dangerous condition. Similarly, the international trade group, the Organisation for Economic Co-operation and Development (OECD), mentions “robustness” and “trustworthy AI” in their AI principles but makes no explicit mention of expectations around basic functionality or performance assessment.
The ideal of “democratizing” AI systems, and the resulting AI innovation policy, is another effort premised on the assumed functionality of AI. This is the argument that access to AI tooling and AI skills should be expanded—with the corollary claim that it is problematic that only certain institutions, nations, or individuals have access to the ability to build these systems. A recent example of democratization efforts was the global push for the relaxation of oversight in data sharing in order to allow for more innovation in AI tool development in the wake of the COVID-19 pandemic. The goal of such efforts was to empower a wider range of non-AI domain experts to participate in AI tool development. This policy impact was long lasting and informed later efforts such as the AI National Resource (AINR) effort in the USand the National Medical Imaging Platform (NMIP) executed by National Health Services (NHS) in the UK. In this flurry of expedited activity, some parallel concerns were also raised about how the new COVID-19 AI tools would adequately address cybersecurity, privacy, and anti-discrimination challenges, but the functionality and utility of the systems remained untested for some time.
An extremely premature set of concerns are those of an autonomous agent becoming so intelligent that humans lose control of the system. While it is not controversial to claim that such concerns are far from being realized, this fear of misspecified objectives, runaway feedback loops, and AI alignment presumes the existence of an industry that can get AI systems to execute on any clearly declared objectives, and that the main challenge is to choose and design an appropriate goal. Needless to say, if one thinks the danger of AI is that it will work too well, it is a necessary precondition that it works at all.
The fear of hyper-competent AI systems also drives discussions on potential misuse. For example, expressed concerns around large language models centers on hyped narratives of the models’ ability to generate hyper-realistic online content, which could theoretically be used by malicious actors to facilitate harmful misinformation campaigns. While these are credible threats, concerns around large language models tend to dismiss the practical limitations of what these models can achieve, neglecting to address more mundane hazards tied to the premature deployment of a system that does not work. This pattern is evident in the EU draft AI regulation, where, even as the legislation does concern functionality to a degree, the primary concerns—questions of “manipulative systems,” “social scoring,” and “emotional or biometric categorization”—“border on the fantastical”. A major policy focus in recent years has been addressing issues of bias and fairness in AI. Fairness research is often centered around attempting to balance some notion of accuracy with some notion of fairness. This research question presumes that an unconstrained solution without fairness restrictions is the optimal solution to the problem. However, this intuition is only valid when certain conditions and assumptions are met, such as the measurement validity of the data and labels. Scholarship on fairness also sometimes presumes that unconstrained models will be optimal or at least useful.argued that U.S. anti-discrimination law would have difficulty addressing algorithmic bias because the “nature of data mining” means that in many cases we can assume the decision is at least statistically valid. Similarly, as an early example of technical fairness solutions,created a method to remove disparate impact from a model while preserving rank, which only makes sense if the unconstrained system output is correct in the first place. Industry practitioners then carry this assumption into how they approach fairness in AI deployments. For example, audits of AI hiring tools focus primarily on ensuring an 80% selection rate for protected classes (the so-called 4/5ths rule) is satisfied, and rarely mention product validation processes, demonstrating an assumed validity of the prediction task.
Another dominant theme in AI policy developments is that of explainability or interpretability. The purpose of making models explainable or interpretable differs depending on who is seen as needing to understand them. From the engineering side, interpretability is usually desired for debugging purposes, so it is focused on functionality. But on the legal or ethical side, things look different. There has been much discussion about whether the GDPR includes a “right to explanation” and what such a right entails. Those rights would serve different purposes. To the extent the purpose of explanation is to enable contestation, then functionality is likely included as an aspect of the system subject to challenge. To the extent explanation is desired to educate consumers about how to improve their chances in the future, such rights are only useful when the underlying model is functional. Similarly, to the extent regulators are looking into functionality, explanations aimed at regulators can assist oversight, but typically explanations are desired to check the basis for decisions, while assuming the systems work as intended. Not all recent policy developments hold the functionality assumption strongly. The Food and Drug Administration (FDA) guidelines for AI systems integrated into software as a medical device (SaMD) has a strong emphasis on functional performance, clearly not taking product performance as a given. The draft AI Act in the EU includes requirements for pre-marketing controls to establish products’ safety and performance, as well as quality management for high risk systems. These mentions suggest that functionality is not always ignored outright. Sometimes, it is considered in policy, but in many cases, that consideration lacks the emphasis of the other concerns presented.
The Many Dimensions of AI Dysfunction
Functionality can be difficult to define precisely. The dictionary definition of “fitness for a product’s intended use”is useful, but incomplete, as some intended uses are impossible. Functionality could also be seen as a statement that a product lives up to the vendor’s performance claims, but this, too, is incomplete; specifications chosen by the vendor could be insufficient to solve the problem at hand. Another possible definition is “meeting stakeholder expectations” more generally, but this is too broad as it sweeps in wider AI ethics concerns with those of performance or operation.
Lacking a perfectly precise definition of functionality, in this section we invert the question by creating a taxonomy that brings together disparate notions of product failure. Our taxonomy serves several other purposes, as well. Firstly, the sheer number of points of failure we were able to identify illustrates the scope of the problem. Secondly, we offer language in which to ground future discussions of functionality in research and policy. Finally, we hope that future proposals for interventions can use this framework to concretely illustrate the way any proposed interventions might work to prevent different kinds of failure.
Methodology
To challenge the functionality assumption and demonstrate the various ways in which AI doesn’t work, we developed a taxonomy of known AI failures through the systematic review of case studies. To do this, we partly relied on the AI, Algorithmic and Automation Incident and Controversy Repository (AIAAIC) spreadsheet crowdsourced from journalism professionals. Out of a database of over 800 cases, we filtered the cases down to a spreadsheet of 283 cases from 2012 to 2021 based on whether the technology involved claimed to be AI, ML or data-driven, and whether the harm reported was due to a failure of the technology. In particular, we focused on describing the ways in which the artifact itself was connected to the failure, as opposed to infrastructural or environmental “meta” failures which caused harm through the artifact. We split up the rows in the resulting set and used an iterative tagging procedure to come up with categories that associate each example with a different element or cause of failure. We updated, merged, and grouped our tags in meetings between tagging sessions, resulting in the following taxonomy. We then chose known case studies from the media and academic literature to illustrate and best characterize these failure modes.
Failure Taxonomy
Here, we present a taxonomy of AI system failures and provide examples of known instances of harm. Many of these cases are direct refutations of the specific instances of the functionality assumptions in Section assumption.
Impossible Tasks
In some situations, a system is not just “broken” in the sense that it needs to be fixed. Researchers across many fields have shown that certain prediction tasks cannot be solved with machine learning. These are settings in which no specific AI developed for the task can ever possibly work, and a functionality-centered critique can be made with respect to the task more generally. Since these general critiques sometimes rely on philosophical, controversial, or morally contested grounds, the arguments can be difficult to leverage practically and may imply the need for further evidence of failure modes along the lines of our other categories.
Conceptually Impossible
Certain classes of tasks have been scientifically or philosophically “debunked” by extensive literature. In these cases, there is no plausible connection between observable data and the proposed target of the prediction task. This includes what Stark and Hutson call “physiognomic artificial intelligence,” which attempts to infer or create hierarchies about personal characteristics from data about their physical appearance. Criticizing the EU Act’s failure to address this inconvenient truth,pointed out that “those claiming to detect emotion use oversimplified, questionable taxonomies; incorrectly assume universality across cultures and contexts; and risk `[taking] us back to the phrenological past’ of analysing character traits from facial structures.”
A notorious example of technology broken by definition are attempts to infer “criminality” from a person’s physical appearance. A paper claiming to do this “with no racial bias” was announced by researchers at Harrisburg University in 2020, prompting widespread criticism from the machine learning community. In an open letter, the Coalition for Critical Technology note that the only plausible relationship between a person’s appearance and their propensity to commit a crime is via the biased nature of the category of “criminality” itself. In this setting, there is no logical basis with which to claim functionality.
Practically Impossible There can be other, more practical reasons for why a machine learning model or algorithm cannot perform a certain task. For example, in the absence of any reasonable observable characteristics or accessible data to measure the model goals in question, attempts to represent these objectives end up being inappropriate proxies. As a construct validity issue, the constructs of the built model could not possibly meaningfully represent those relevant to the task at hand.
Criminal justice offers a wide variety of such practically impossible tasks, either through the lack of availability of the data.Many predictive policing tools are arguably practically impossible AI systems. Predictive policing attempts to predict crime at either the granularity of location or at an individual level. The data that would be required to do the task properly—accurate data about when and where crimes occur—does not and will never exist. While crime is a concept with a fairly fixed definition, it is practically impossible to predict because of structural problems in its collection. The problems with crime data are well-documented—whether in differential victim crime reporting rates, selection bias based on policing activities, dirty data from periods of recorded unlawful policing, and more.
What work is this discussion of thick concepts doing? Can we delete? Why is it in this subsection while it says they are conceptually impossible? Also, is it necessarily accurate that thick concept = conceptually impossible? Some of these are thick concepts – concepts that describe and evaluate simultaneously – which ideally are co-produced but are typically based on the values judgement of researchers. Common focus areas of criminal justice algorithms like “likelihood of flight”,“public safety”, or “dangerousness” represent thick concepts. A universal or legally valid version of these constructs may not be possible, making thempotentially conceptually impossible.
Due to upstream policy, data or societal choices, AI tasks can be practically impossible for one set of developers and not for another, or for different reasons in different contexts. The fragmentation, billing focus, and competing incentives of the US healthcare system have made multiple healthcare-related AI tasks practically impossible. US EHR data is often erroneous, miscoded, fragmented, and incomplete, creating a mismatch between available data and intended use. Many of these challenges appeared when IBM attempted to support cancer diagnoses. In one instance, this meant using synthetic as opposed to real patients for oncology prediction data, leading to “unsafe and incorrect” recommendations for cancer treatments. In another, IBM worked with MD Anderson to work on leukemia patient records, poorly extracting reliable insights from time-dependent information like therapy timelines—the components of care most likely to be mixed up in fragmented doctors’ notes.
Engineering Failures
Algorithm developers maintain enormous discretion over a host of decisions, and make choices throughout the model development lifecycle. These engineering choices include defining problem formulation, setting up evaluation criteria, and determining a variety of other details. Failures in AI systems can often be traced to these specific policies or decisions in the development process of the system.
Model Design Failures
Sometimes, the design specifications of a model are inappropriate for the task it is being developed for. For instance, in a classification model, choices such as which input and target variables to use, whether to prioritize accepting true positives or rejecting false negatives, and how to process the training data all factor into determining model outcomes. These choices are normative and may prioritize values such as efficiency over preventing harmful failures. In 2014, BBC Panorama uncovered evidence of international students systematically cheating on English language exams run by the UK’s Educational Testing Service by having others take the exam for them. The Home Office began an investigation and campaign to cancel the visas of anyone who was found to have cheated. In 2015, ETS used voice recognition technology to identify this type of cheating. According to the National Audit Office,
ETS identified 97% of all UK tests as “suspicious”. It classified 58% of 58,459 UK tests as “invalid” and 39% as “questionable”. The Home Office did not have the expertise to validate the results nor did it, at this stage, get an expert opinion on the quality of the voice recognition evidence. … but the Home Office started cancelling visas of those individuals given an “invalid” test.
The staggering number of accusations obviously included a number of false positives. The accuracy of ETS’s method was disputed between experts sought by the National Union of Students and the Home Office; the resulting estimates of error rates ranged from 1% to 30%. Yet out of 12,500 people who appealed their immigration decisions, only 3,600 won their cases—and only a fraction of these were won through actually disproving the allegations of cheating. This highly opaque system was thus notable for the disproportionate amount of emphasis that was put into finding cheaters rather than protecting those who were falsely accused. Although we cannot be sure the voice recognition model was trained to optimize for sensitivity rather than specificity, as the head of the NAO aptly put, “When the Home Office acted vigorously to exclude individuals and shut down colleges involved in the English language test cheating scandal, we think they should have taken an equally vigorous approach to protecting those who did not cheat but who were still caught up in the process, however small a proportion they might be”. This is an example of a system that was not designed to prevent a particular type of harmful failure.
Model Implementation Failures
Even if a model was conceptualized in a reasonable way, some component of the system downstream from the original plan can be executed badly, lazily, or wrong. In 2011, the state of Idaho attempted to build an algorithm to set Medicaid assistance limits for individuals with developmental and intellectual disabilities. When individuals reported sudden drastic cuts to their allowances, the ACLU of Idaho tried to find out how the allowances were being calculated, only to be told it was a trade secret. Theclass action lawsuit that followed resulted in a court-ordered disclosure of the algorithm, which was revealed to have critical flaws. According to Richard Eppink, Legal Director of the ACLU of Idaho,
There were a lot of things wrong with it. First of all, the data they used to come up with their formula for setting people’s assistance limits was corrupt. They were using historical data to predict what was going to happen in the future. But they had to throw out two-thirds of the records they had before they came up with the formula because of data entry errors and data that didn’t make sense.
Data validation is a critical step in the construction of a ML system, and the team that built the benefit system chose to use a highly problematic dataset to train their model. For this reason, we consider this to be an implementation failure.
Another way that failures can be attributed to poor implementation is when a testing framework was not appropriately implemented. One area in which a lack of sufficient testing has been observed in the development of AI is in the area of clinical medicine.systematically examined the methods and claims of studies which compared the performance of diagnostic deep learning computer vision algorithms against that of expert clinicians. In their literature review, they identified 10 randomized clinical trials and 81 non-randomized clinical trials. Of the 81 non-randomized studies, they found the median number of clinical experts compared to the AI was 4, full access to datasets and code were unavailable in over 90% of studies, the overall risk of bias was high, and adherence to reporting standards were suboptimal, and therefore poorly substantiate their claims. Similarly, the Epic sepsis prediction model, a product actually implemented at hundreds of hospitals, was recently externally validated by, who found that the model had poor calibration to other hospital settings and discriminated against under-represented demographics. These results suggest that the model’s testing prior to deployment may have been insufficient to estimate its real-world performance. Notably, the COVID-19 technology which resulted from innovation policy and democratization efforts mentioned in section assumption was later shown to be completely unsuitable for clinical deployment after the fact.
Missing Safety Features
Sometimes model failures are anticipated yet difficult to prevent; in this case, engineers can sometimes take steps to ensure these points of failure will not cause harm. In 2014, a Nest Labs smoke and carbon monoxide detector was recalled. The detector had a feature which allowed the user to turn it off with a “wave” gesture. However, the company discovered in testing that under certain circumstances, the sensor could be unintentionally deactivated. Detecting a wave gesture with complete accuracy is impossible, and Google acknowledges factors that contribute to the possibility of accidental wave triggering for its other home products. However, the lack of a failsafe to make sure the carbon monoxide detector could not be turned off accidentally made the product dangerous.
In the same way, the National Transportation Safety Board (NTSB) cited a lack of adequate safety measures—such as “a warning/alert when the driver’s hands are off the steering wheel”, “remote monitoring of vehicle operators” and even the companies’ “inadequate safety culture”—as the probable causes in at least two highly publicized fatal crashes of Uberand Teslaself-driving cars. As products in public beta-testing, this lack of functional safeguards was considered to be an even more serious operational hazard than any of the engineering failures involved (such as the vehicle’s inability to detect an incoming pedestrianor truck).
This category also encompasses algorithmic decision systems in critical settings that lack a functional appeals process. This has been a recurring feature in algorithms which allocate benefits on behalf of the government. Not all of these automated systems rely on machine learning, but many have been plagued by bugs and faulty data, resulting in the denial of critical resources owed to citizens. In the case of the Idaho data-driven benefit allocation system, even the people responsible for reviewing appeals were unable to act as a failsafe for the algorithm’s mistakes: “They would look at the system and say, `It’s beyond my authority and my expertise to question the quality of this result’ ”.
Deployment Failures
Sometimes, despite attempts to anticipate failure modes during the design phase, the model does not “fail” until it is exposed to certain external factors and dynamics that arise after it is deployed.
Robustness Issues
A well-documented source of failure is a lack of robustness to changing external conditions.have observed that the benchmarking methods used for evaluation in machine learning can suffer from both internal and external validity problems, where “internal validity refers to issues that arise within the context of a single benchmark” and “external validity asks whether progress on a benchmark transfers to other problems.” If a model is developed in a certain context without strong evaluation methods for external validity, it may perform poorly when exposed to real-world conditions that were not captured by the original context. For instance, while many computer vision models developed on ImageNet are tested on synthetic image perturbations in an attempt to measure and improve robustness, buthave found that these models are not robust to real-world distribution shifts such as a change in lighting or pose. Robustness issues are also of dangerous consequence in language models. For example, when large language models are used to process the queries of AI-powered web search, the models’ fragility to misspellings, or trivial changes toformatand contextcan lead to unexpected results. In one case, a large language model used in Google search could not adequately handle cases ofnegation– and so when queried with “what to do when having a seizure”, the model alarmingly sourced the information for what not to do, unable to differentiate between the two cases.
Failure under Adversarial Attacks
Failures can also be induced by the actions of an adversary—an actor deliberately trying to make the model fail. Real-world examples of this often appear in the context of facial recognition, in which adversaries have some evidence that they can fool face-detection systems with, such as 3d-printed masksor software-generated makeup. Machine learning researchers have studied what they call “adversarial examples,” or inputs that are designed to make a machine learning model fail. However, some of this research has been criticized by its lack of a believable threat model— in other words, not focusing on what real-world “adversaries” are actually likely to do.
Unanticipated Interactions
A model can also fail to account for uses or interactions that it was not initially conceived to handle. Even if an external actor or user is not deliberately trying to break a model, their actions may induce failure if they interact with the model in a way that was not planned for by the model’s designers. For instance, there is evidence that this happened at the Las Vegas Police Department:
As new records about one popular police facial recognition system show, the quality of the probe image dramatically affects the likelihood that the system will return probable matches. But that doesn’t mean police don’t use bad pictures anyway. According to documents obtained by Motherboard, the Las Vegas Metropolitan Police Department (LVMPD) used “non-suitable" probe images in almost half of all the facial recognition searches it made last year, greatly increasing the chances the system would falsely identify suspects, facial recognition researchers said.This aligns with reports fromabout other police departments inappropriately uploading sketch and celebrity photos to facial recognition tools. It is possible for designers to preempt misuse by implementing instructions, warnings, or error conditions, and failure to do so creates a system that does not function properly.
Communication Failures
As with other areas of software development, roles in AI development and deployment are becoming more specialized. Some roles focus on managing the data that feeds into models, others specialize in modeling, and others optimally engineer models for speed and scale. There are even those in “analytics translator” roles – managers dedicated to acting as communicators between data science work and non-technical business leaders. And, of course, there are salespeople. Throughout this chain of actors, potential miscommunications or outright lies can happen about the performance, functional safety or other aspects of deployed AI/ML systems. Communication failures often co-occur with other functional safety problems, and the lack of accountability for false claims – intentional or otherwise – makes these particularly pernicious and likely to occur as AI hype continues absent effective regulation.
Falsified or Overstated Capabilities
To pursue commercial or reputational interests, companies and researchers may explicitly make claims about models which are provably untrue. A common form of this are claims that a product is “AI”, when in fact it mainly involves humans making decisions behind the scenes. While this in and of itself may not create unsafe products, expectations based on unreasonable claims can create unearned trust, and a potential over-reliance that hurts parties who purchase the product. As an example, investors poured money into ScaleFactor, a startup that claimed to have AI that could replace accountants for small businesses, with the exciting (for accountants) tagline “Because evenings are for families, not finance”. Under the hood, however,
Instead of software producing financial statements, dozens of accountants did most of it manually from ScaleFactor’s Austin headquarters or from an outsourcing office in the Philippines, according to former employees. Some customers say they received books filled with errors, and were forced to re-hire accountants, or clean up the mess themselves.Even large well-funded entities misrepresent the capabilities of their AI products. Deceptively constructed evaluation schemes allow AI product creators to make false claims. In 2018, Microsoft created machine translation with “equal accuracy to humans in Chinese to English translations”. However, the study used to make this claim (still prominently displayed in press release materials) was quickly debunked by a series of outside researchers who found that at the document-level, when provided with context from nearby sentences, and/or compared to human experts, the machine translation model did not indeed achieve equal accuracy to human translators. This follows a pattern seen with machine learning products in general, where the advertised performance on a simple and static data benchmark, is much lower than the performance on the often more complex and diverse data encountered in practice.
Misrepresented Capabilities A simple way to deceive customers into using prediction services is to sell the product for a purpose you know it can’t reliably be used for. In 2018, the ACLU of Northern California revealed that Amazon effectively misrepresented capabilities to police departments in selling their facial recognition product, Rekognition. Building on previous work, the ACLU ran Rekognition with a database of mugshots against members of U.S. Congress using the default setting and found 28 members falsely matched within the database, with people of color shown as a disproportionate share of these errors. This result was echoed bymonths later. Amazon responded by claiming that for police use cases, the threshold for the service should be set at either 95% or 99% confidence. However, based on a detailed timeline of events, it is clear that in selling the service through blog posts and other campaigns that thresholds were set at 80% or 85% confidence, as the ACLU had used in its investigation. In fact, suggestions to shift that threshold were buried in manuals end-users did not read or use – even when working in partnership with Amazon. At least one of Amazon’s police clients also claimed being unaware of needing to modify the default threshold.
The hype surrounding IBM’s Watson in healthcare represents another example where a product that may have been fully capable of performing specific helpful tasks was sold as a panacea to health care’s ills. As discussed earlier, this is partially the result of functional failures like practical impossibility – but these failures were coupled with deceptively exaggerated claims. The backlash to this hype has been swift in recent years, with one venture capitalist claiming “I think what IBM is excellent at is using their sales and marketing infrastructure to convince people who have asymmetrically less knowledge to pay for something”. At Memorial-Sloan Kettering, after $62 million dollars spent and may years of effort, MD Anderson famously cancelled IBM Watson contracts with no results to show for it. This is particularly a problem in the context of algorithms developed by public agencies – where the AI systems can be adopted as symbols for progress, or smokescreens for undesirable policy outcomes, and thus liable to inflated narratives of performance.discusses how the celebrated success of “self-driving shuttles” in Columbus, Ohio omits its marked failure in the lower-income Linden neighborhood, where residents were now locked out of the transportation apps due to a lack of access to a bank account, credit cards, a data plan or Wi-Fi. Similarly,demonstrates how a $1.4 billion contract with a coalition of high-tech companies led an Indiana governor to stubbornly continue a welfare automation algorithm that resulted in a 54% increase in the denials of welfare applications.
Dealing With Dysfunction: Opportunities for Intervention on Functional Safety
The challenge of dealing with an influx of fraudulent or dysfunctional products is one that has plagued many industries, including food safety, medicine, financial modeling, civil aviationand the automobile industry. In many cases, it required the active advocacy of concerned citizens to lead to the policy interventions that would effectively change the tide of these industries. The AI field seems to now be facing this same challenge.
Thankfully, as AI operates as a general purpose technology prevalent in many of these industries, there already exists a plethora of governance infrastructure to address this issue in related fields of application. In fact, healthcare is the field where AI product failures appear to be the most visible, in part due to the rigor of pre-established evaluation processes. Similarly, the transportation industry has a rich history of thorough accident reports and investigations, through organizations such as the National Transportation and Safety Board (NTSB), who have already been responsible for assessing the damage from the few known cases of self-driving car crashes from Uber and Tesla.
In this section, we specifically outline the legal and organizational interventions necessary to address functionality issues in general context in which AI is developed and deployed into the market. In broader terms, the concept of functional safety in engineering design literaturewell encapsulates the concerns articulated in this paper—namely that a system can be deployed without working very well, and that such performance issues can cause harm worth preventing.
Legal/Policy Interventions
The law has several tools at its disposal to address product failures to work correctly. They mostly fall in the category of consumer protection law. This discussion will be U.S.-based, but analogues exist in most jurisdictions.
Consumer Protection
The Federal Trade Commission is the federal consumer protection agency within the United States with the broadest subject matter jurisdiction. Under Section 5 of the FTC Act, it has the authority to regulate “unfair and deceptive acts or practices” in commerce. This is abroad grant authority to regulate practices that injure consumers. The authority to regulate deceptive practices applies to any material misleading claims relating to a consumer product. The FTC need not show intent to deceive or that deception actually occurred, only that claims are misleading. Deceptive claims can be expressed explicitly—for example, representation in the sales materials that is inaccurate—or implied, such as an aspect of the design that suggests a functionality the product lacks. Many of the different failures, especially impossibility, can trigger a deceptive practices claim.
The FTC’s ability to address unfair practices is wider-ranging but more controversial. The FTC can reach any practice “likely to cause substantial injury to consumers[,] not reasonably avoidable by consumers themselves and not outweighed by countervailing benefits to consumers”. Thus, where dysfunctional AI is being sold and its failures causes substantial harm to consumers, the FTC could step in. Based on the FTC’s approach to data security, in which the Commission has sued companies for failing to adequately secure consumer data in their possession against unknown third-party attackers, even post-deployment failures—if foreseeable and harmful—can be included among unfair practices, though they partially attributable to external actors.
The FTC can use this authority to seek an injunction, requiring companies to cease the practice. Formally, the FTC does not have the power to issue fines under its Section 5 authority, but the Commission frequently enters into long-term consent decrees with companies that it sues, permitting continuing jurisdiction, monitoring, and fines for future violations. The Commission does not have general rulemaking authority, so most of its actions to date have taken the form of public education and enforcement. The Commission does, however, have authority to make rules regarding unfair or deceptive practices under the Magnuson-Moss Warranty Act. Though it has created no new rules since 1980, in July 2021, the FTC voted to change internal agency policies to make it easier to do so.
Other federal agencies also have the ability to regulate faulty AI systems, depending on their subject matter. The Consumer Product Safety Commission governs the risks of physical injury due to consumer products. They can create mandatory standards for products, can require certifications of adherence to those rules, and can investigate products that have caused harm, leading to bans or mandatory recalls. The National Highway Safety Administration offers similar oversight for automobiles specifically. The Consumer Finance Protection Bureau can regulate harms from products dealing with loans, banking, or other consumer finance issues.
In addition to various federal agencies, all states have consumer protection statutes that bar deceptive practices and many bar unfair practices as well, like the FTC Act. False advertising laws are related and also common. State attorneys general often take active roles as enforcers of those laws. Of course, the efficacy of such laws varies from state to state, but in principle, they become another source of law and enforcement to look to for the same reasons that the FTC can regulate under Section 5. One particular state law worth noting is California’s Unfair Competition Law, which allows individuals to sue for injunctive relief to halt conduct that violates other laws, even if individuals could not otherwise sue under that law.
It is certainly no great revelation that federal and state regulatory apparatuses exist. Rather, our point is that while concerns about discrimination and due process can lead to difficult questions about the operation of existing law and proposals for legal reform, thinking about the ways that AI is not working makes it look like other product failures that we know how to address. Where AI doesn’t work, suddenly regulatory authority is easy to find.
Products Liability Law
Another avenue for legal accountability may come from the tort of products liability, though there are some potential hurdles. In general, if a person is injured by a defective product, they can sue the producer or seller in products liability. The plaintiff need not have purchased or used the product; it is enough that they were injured by it, and the product has a defect that rendered it unsafe.
It would stand to reason that a functionality failure in an AI system could be deemed a product defect. But surprisingly, defective software has never led to a products liability verdict. One commonly cited reason is that products liability applies most clearly to tangible things, rather than information products, and that aside from a stray comment in one appellate case, no court has actually ruled that software is even a “product” for these purposes. This would likely not be a problem for software that resides within a physical system, but for non-embodied AI, it might pose a hurdle. In a similar vein, because most software harms have typically been economic in nature, with, for example, a software crash leading to a loss of work product, courts have rejected these claims as “pure economic loss” belonging more properly in contract law than tort. But these mostly reflect courts’ anxiety with intangible injuries, and as AI discourse has come to recognize many concrete harms, these concerns are less likely to be hurdles going forward.
Writing about software and tort law,identifies the complexity of software as a more fundamental type of hurdle. For software of nontrivial complexity, it is provably impossible to guarantee bug-free code. An important part of products liability is weighing the cost of improvements and more testing against the harms. But as no amount of testing can guarantee bug-free software, it will difficult to determine how much testing is enough to be considered reasonable or non-negligent. Choi analogizes this issue to car crashes: car crashes are inevitable, but courts developed the idea of crashworthiness to ask about the car’s contribution to the total harm, even if the initial injury was attributable to a product defect. While Choi looks to crashworthiness as a solution, the thrust of his argument is that software can cause exactly the type of injury that products liability aims to protect us from, and doctrine should reflect that.
While algorithmic systems have a similar sort of problem, the failure we describe here are more basic. Much as writing bug-free software is impossible, creating a model that handles every corner case perfectly is impossible. But the failures we address here are not about unforeseeable corner cases in models. We are concerned with easier questions of basic functionality, without which a system should never have been shipped. If a system is not functional, in the sense we describe, a court should have no problem finding that it is unreasonably defective. As discussed above, a product could be placed on the market claiming the ability to do something it cannot achieve in theory or in practice, or it can fail to be robust to unanticipated but foreseeable uses by consumers. Even where these errors might be difficult to classify in doctrinally rigid categories of defect, courts have increasingly been relying on “malfunction doctrine,” which allows for circumstantial evidence to be used as proof of defect where “a product fails to perform its manifestly intended function.”. Courts are increasingly relying on this doctrine and it could apply here. Products liability could especially easily apply to engineering failures, where the error was foreseeable and an alternative, working version of the product should have been built.
Warranties
Another area of law implicated by product failure is warranty law, which protects the purchasers of defunct AI and certain third parties who stand to benefit from the sale. Sales of goods typically come with a set of implied warranties. The implied warranty of merchantability applies to all goods and states, among other things, that the good is “fit for the ordinary purposes for which such goods are used”. The implied warranty of fitness for particular purpose applies when a seller knows that the buyer has a specific purpose in mind and the buyer is relying on the seller’s skill or judgment about the good’s fitness, stating that the good is fit for that purpose. Defunct AI will breach both these warranties. The remedy for such a breach is limited to contract damages. This area of law is concerned with ensuring that purchasers get what they pay for, so compensation will be limited roughly to value of the sale. Injuries not related to the breach of contract are meant to be worked out in tort law, as described above.
Fraud
In extreme cases, the sale of defunct AI may constitute fraud. Fraud has many specific meanings in law, but invariably it involves a knowing or intentional misrepresentation that the victim relied on in good faith. In contract law, proving that a person was defrauded can lead to contract damages. Restitution is another possible remedy for fraud. In tort law, a claim of fraud can lead to compensation necessary to rectify any harms that come from the fraud, as well as punitive damages in egregious cases. Fraud is difficult to prove, and our examples do not clearly indicate fraud, but it is theoretically possible if someone is selling snake oil. Fraud can lead to criminal liability as well.
Other Legal Avenues Already Being Explored
Finally, other areas of law that are already involved in the accountability discussion, such as discrimination and due process, become much easier cases to make when the AI doesn’t work. Disparate impact law requires that the AI tool used be adequately predictive of the desired outcome, before even getting into the question of whether it is too discriminatory or not. A lack of construct validity would easily subject a model’s user to liability. Due process requires decisions to not be arbitrary, and AI that doesn’t work loses its claim to making decisions on a sound basis. Where AI doesn’t work, legal cases in general become easier.
Organizational interventions
In addition to legal levers, there are many organizational interventions that can be deployed to address the range of functionality issues discussed. Due to clear conflicts of interest, the self-regulatory approaches described are far from adequate oversight for these challenges, and the presence of regulation does a lot to incentivise organizations to take these actions in the first place. However, they do provide an immediate path forward in addressing these issues.
Internal Audits & Documentation
After similar crises of performance in fields such as aerospace, finance and medicine, such processes evolved in those industries to enforce a new level of introspection in the form of internal audits. Taking the form of anything from documentation exercises to challenge datasets as benchmarks, these processes raised the bar for deployment criteria and matured the product development pipeline in the process. The AI field could certainly adopt similar techniques for increasing the scrutiny of their systems, especially given the nascent state of reflection and standardization common in ML evaluation processes. For example, the “Failure modes, effects, and diagnostic analysis (FMEDA)’’ documentation process from the aerospace industry could support the identification of functional safety issues prior to AI deployment, in addition to other resources from aerospace (such as the functional hazard analyses (FHA) or Functional Design Assurance Levels (FDALS)).
Ultimately, internal audits are a self-regulatory approach—though audits conducted by independent second parties such as a consultancy firm could provide a fresh perspective on quality control and performance in reference to articulated organizational expectations. The challenge with such audits, however, is that the results are rarely communicated externally and disclosure is not mandatory, nor is it incentivized. As a result, assessment outcomes are mainly for internal use only, often just to set internal quality assurance standards for deployment and prompt further engineering reflection during the evaluation process.
Product Certification & Standards
A trickier intervention is the avenue of product certification and standards development for AI products. This concept has already made its way into AI policy discourse; CEN (European Committee for Standardisation) and CENELEC (European Committee for Electrotechnical Standardisation), two of three European Standardisation Organisations (ESOs) were heavily involved in the creation of the EU’s draft AI Act. On the U.S. front, industry groups IEEE and ISO regularly shape conversations, with IEEE going so far as to attempt the development of a certification program. In the aviation industry, much of the establishment of engineering standards happened without active government intervention, between industry peers. These efforts resemble the Partnership on AI’s attempt to establish norms on model documentation processes. Collective industry-wide decision-making on critical issues can raise the bar for the entire industry and raise awareness within the industry of the importance of handling functionality challenges. Existing functional safety standards from the automobile (ISO 26262), aerospace (US RTCA DO-178C), defense (MIL-STD-882E) and electronics (IEEE IEC 61508 / IEC 61511) industries, amongst others, can provide a template on how to approach this challenge within the AI industry.
Other Interventions
There are several other organizational factors that can determine and assess the functional safety of a system. As a client making decisions on which projects to select, or permit for purchase, it can be good to set performance related requirements for procurement and leverage this procurement process in order to set expectations for functionality. Similarly, cultural expectations for safety and engineering responsibility impact the quality of the output from the product development process – setting these expectations internally and fostering a healthy safety culture can increase cooperation on other industry-wide and organizational measures. Also, as functionality is a safety risk aligned with profit-oriented goals, many model logging and evaluation operations tools are available for organizations to leverage in the internal inspection of their systems – including tools for more continuous monitoring of deployed systems.
Conclusion : The Road Ahead
We cannot take for granted that AI products work. Buying into the presented narrative of a product with at least basic utility or an industry that will soon enough “inevitably’’ overcome known functional issues causes us to miss important sources of harm and available legal and organizational remedies. Although functionality issues are not completely ignored in AI policy, the lack of awareness of the range in which these issues arise leads to the problems being inadequately emphasized and poorly addressed by the full scope of accountability tools available.
The fact that faulty AI products are on the market today makes this problem particularly urgent. Poorly vetted products permeate our lives, and while many readily accept the potential for harms as a tradeoff, the claims of the products’ benefits go unchallenged. But addressing functionality involves more than calling out demonstrably broken products. It also means challenging those who develop AI systems to better and more honestly understand, explore, and articulate the limits of their products prior to their release into the market or public use. Adequate assessment and communication of functionality should be a minimum requirement for mass deployment of algorithmic systems. Products that do not function should not have the opportunity to affect people’s lives.
We thank the Mozilla Foundation and the Algorithmic Justice League for providing financial support during this project.
Bibliography
1@article{shankar2021towards,
2 year = {2021},
3 journal = {arXiv preprint arXiv:2108.13557},
4 author = {Shankar, Shreya and Parameswaran, Aditya},
5 title = {Towards Observability for Machine Learning Pipelines},
6}
7
8@article{mulligan2019procurement,
9 publisher = {HeinOnline},
10 year = {2019},
11 pages = {773},
12 volume = {34},
13 journal = {Berkeley Tech. LJ},
14 author = {Mulligan, Deirdre K and Bamberger, Kenneth A},
15 title = {Procurement as policy: Administrative process for machine learning},
16}
17
18@article{richardson2021best,
19 year = {2021},
20 journal = {Available at SSRN 3855637},
21 author = {Richardson, Rashida},
22 title = {Best Practices for Government Procurement of Data-Driven Technologies},
23}
24
25@article{rubenstein2021acquiring,
26 year = {2021},
27 volume = {73},
28 journal = {Florida Law Review},
29 author = {Rubenstein, David S},
30 title = {Acquiring ethical AI},
31}
32
33@inproceedings{bender2020climbing,
34 year = {2020},
35 pages = {5185--5198},
36 booktitle = {Proceedings of the 58th Annual Meeting of the Association for Computational Linguistics},
37 author = {Bender, Emily M and Koller, Alexander},
38 title = {Climbing towards NLU: On meaning, form, and understanding in the age of data},
39}
40
41@misc{menegus2019defense,
42 publisher = {Gizmodo},
43 year = {2019},
44 author = {Menegus, Brian},
45 title = {Defense of amazon’s face recognition tool undermined by its only known police client},
46}
47
48@article{garvie2019garbage,
49 year = {2019},
50 journal = {Georgetown Law Center on Privacy \& Technology},
51 author = {Garvie, Clare},
52 title = {Garbage in, Garbage out. Face recognition on flawed data},
53}
54
55@misc{uber-crash,
56 url = {https://ntsb.gov/investigations/Pages/HWY18FH010.aspx},
57 file = {https://ntsb.gov/investigations/Pages/HWY18FH010.aspx},
58 year = {2018},
59 institution = {National Transportation Safety Board},
60 number = {HWY18MH010},
61 title = {Collision Between Vehicle Controlled by Developmental Automated Driving System and Pedestrian},
62 author = {National Transportation Safety Board},
63}
64
65@misc{uber-crash-2,
66 url = {https://www.ntsb.gov/news/press-releases/Pages/NR20191119c.aspx},
67 file = {https://www.ntsb.gov/news/press-releases/Pages/NR20191119c.aspx},
68 year = {2019},
69 institution = {National Transportation Safety Board},
70 number = {HWY18MH010},
71 title = {‘Inadequate Safety Culture’ Contributed to Uber Automated Test Vehicle Crash - NTSB Calls for Federal Review Process for Automated Vehicle Testing on Public Roads},
72 author = {Eric Weiss},
73}
74
75@misc{tesla-crash,
76 url = {https://ntsb.gov/investigations/Pages/HWY18FH010.aspx},
77 file = {https://ntsb.gov/investigations/Pages/HWY18FH010.aspx},
78 year = {2017},
79 institution = {National Transportation Safety Board},
80 number = {HWY16FH018},
81 title = {Collision Between a Car Operating With Automated Vehicle Control Systems
82and a Tractor-Semitrailer Truck},
83 author = {National Transportation Safety Board},
84}
85
86@misc{tesla-crash-2,
87 url = {https://www.ntsb.gov/news/press-releases/pages/pr20170912.aspx},
88 file = {https://www.ntsb.gov/news/press-releases/pages/pr20170912.aspx},
89 year = {2017},
90 institution = {National Transportation Safety Board},
91 number = {HWY16FH018},
92 title = {Driver Errors, Overreliance on Automation, Lack of Safeguards, Led to Fatal Tesla Crash},
93 author = {National Transportation Safety Board},
94}
95
96@article{ratner2019mlsys,
97 year = {2019},
98 journal = {arXiv preprint arXiv:1904.03257},
99 author = {Ratner, Alexander and Alistarh, Dan and Alonso, Gustavo and Andersen, David G and Bailis, Peter and Bird, Sarah and Carlini, Nicholas and Catanzaro, Bryan and Chayes, Jennifer and Chung, Eric and others},
100 title = {MLSys: The new frontier of machine learning systems},
101}
102
103@inproceedings{bender2020climbing,
104 year = {2020},
105 pages = {5185--5198},
106 booktitle = {Proceedings of the 58th Annual Meeting of the Association for Computational Linguistics},
107 author = {Bender, Emily M and Koller, Alexander},
108 title = {Climbing towards NLU: On meaning, form, and understanding in the age of data},
109}
110
111@article{sloane2022silicon,
112 publisher = {Elsevier},
113 year = {2022},
114 pages = {100425},
115 number = {2},
116 volume = {3},
117 journal = {Patterns},
118 author = {Sloane, Mona and Moss, Emanuel and Chowdhury, Rumman},
119 title = {A Silicon Valley love triangle: Hiring algorithms, pseudo-science, and the quest for auditability},
120}
121
122@article{nayak2019understanding,
123 year = {2019},
124 volume = {295},
125 journal = {The Keyword},
126 author = {Nayak, Pandu},
127 title = {Understanding searches better than ever before},
128}
129
130@misc{covid_us,
131 publisher = {POLITICO},
132 year = {2020},
133 author = {Overly, Steven},
134 title = {White House seeks Silicon Valley help battling coronavirus},
135}
136
137@misc{covid_china,
138 publisher = {Center for Security and Emerging Technology},
139 year = {2020},
140 author = {Weinstein, Emily },
141 title = {China's Use of AI in its COVID-19 Response},
142}
143
144@article{covid_greece,
145 author = {Nature Editorial},
146 language = {en},
147 year = {2021},
148 pages = {447--448},
149 number = {7877},
150 volume = {597},
151 journal = {Nature},
152 title = {Greece used {AI} to curb {COVID}: what other nations can learn},
153}
154
155@article{covid_africa,
156 year = {2021},
157 journal = {Available at SSRN 3787748},
158 author = {Mellado, Bruce and Wu, Jianhong and Kong, Jude Dzevela and Bragazzi, Nicola Luigi and Asgary, Ali and Kawonga, Mary and Choma, Nalomotse and Hayasi, Kentaro and Lieberman, Benjamin and Mathaha, Thuso and others},
159 title = {Leveraging Artificial Intelligence and Big Data to optimize COVID-19 clinical public health and vaccination roll-out strategies in Africa},
160}
161
162@article{covid_intl,
163 publisher = {Multidisciplinary Digital Publishing Institute},
164 year = {2020},
165 pages = {156--165},
166 number = {2},
167 volume = {1},
168 journal = {AI},
169 author = {Allam, Zaheer and Dey, Gourav and Jones, David S},
170 title = {Artificial intelligence (AI) provided early detection of the coronavirus (COVID-19) in China and will influence future Urban health policy internationally},
171}
172
173@article{havens2019principles,
174 publisher = {IEEE},
175 year = {2019},
176 pages = {69--72},
177 number = {4},
178 volume = {52},
179 journal = {Computer},
180 author = {Havens, John C and Hessami, Ali},
181 title = {From Principles and Standards to Certification},
182}
183
184@inproceedings{ieee_cert,
185 organization = {IEEE},
186 year = {2018},
187 pages = {3--18},
188 booktitle = {2018 IEEE Symposium on Security and Privacy (SP)},
189 author = {Gehr, Timon and Mirman, Matthew and Drachsler-Cohen, Dana and Tsankov, Petar and Chaudhuri, Swarat and Vechev, Martin},
190 title = {Ai2: Safety and robustness certification of neural networks with abstract interpretation},
191}
192
193@article{raji2019ml,
194 year = {2019},
195 journal = {arXiv preprint arXiv:1912.06166},
196 author = {Raji, Inioluwa Deborah and Yang, Jingying},
197 title = {About ml: Annotation and benchmarking on understanding and transparency of machine learning lifecycles},
198}
199
200@article{sloane2021ai,
201 year = {2021},
202 author = {Sloane, Mona and Chowdhury, Rumman and Havens, John C and Lazovich, Tomo and Rincon Alba, Luis},
203 title = {AI and Procurement-A Primer},
204}
205
206@article{UNESCO,
207 address = {Paris, France},
208 publisher = {UNESCO},
209 year = {2022},
210 journal = {Missing Links in AI Policy},
211 title = {Change From the Outside: Towards Credible Third-Party Audits of AI Systems},
212 author = {Raji, Inioluwa Deborah and Costanza-Chock, Sasha and Buolamwini, Joy},
213}
214
215@inproceedings{raji2020closing,
216 year = {2020},
217 pages = {33--44},
218 booktitle = {Proceedings of the 2020 conference on fairness, accountability, and transparency},
219 author = {Raji, Inioluwa Deborah and Smart, Andrew and White, Rebecca N and Mitchell, Margaret and Gebru, Timnit and Hutchinson, Ben and Smith-Loud, Jamila and Theron, Daniel and Barnes, Parker},
220 title = {Closing the AI accountability gap: Defining an end-to-end framework for internal algorithmic auditing},
221}
222
223@book{roland1991system,
224 publisher = {John Wiley \& Sons},
225 year = {1991},
226 author = {Roland, Harold E and Moriarty, Brian},
227 title = {System safety engineering and management},
228}
229
230@book{smith2004functional,
231 publisher = {Routledge},
232 year = {2004},
233 author = {Smith, David and Simpson, Kenneth},
234 title = {Functional safety},
235}
236
237@article{harris2019ntsb,
238 year = {2019},
239 journal = {IEEE Spectrum},
240 author = {Harris, M},
241 title = {NTSB investigation into deadly Uber self-driving car crash reveals lax attitude toward safety},
242}
243
244@article{benjamens2020state,
245 publisher = {Nature Publishing Group},
246 year = {2020},
247 pages = {1--8},
248 number = {1},
249 volume = {3},
250 journal = {NPJ digital medicine},
251 author = {Benjamens, Stan and Dhunnoo, Pranavsingh and Mesk{\'o}, Bertalan},
252 title = {The state of artificial intelligence-based FDA-approved medical devices and algorithms: an online database},
253}
254
255@article{rivera2020guidelines,
256 publisher = {British Medical Journal Publishing Group},
257 year = {2020},
258 volume = {370},
259 journal = {bmj},
260 author = {Rivera, Samantha Cruz and Liu, Xiaoxuan and Chan, An-Wen and Denniston, Alastair K and Calvert, Melanie J},
261 title = {Guidelines for clinical trial protocols for interventions involving artificial intelligence: the SPIRIT-AI extension},
262}
263
264@article{liu2020reporting,
265 publisher = {British Medical Journal Publishing Group},
266 year = {2020},
267 volume = {370},
268 journal = {bmj},
269 author = {Liu, Xiaoxuan and Rivera, Samantha Cruz and Moher, David and Calvert, Melanie J and Denniston, Alastair K},
270 title = {Reporting guidelines for clinical trial reports for interventions involving artificial intelligence: the CONSORT-AI extension},
271}
272
273@article{wu2021medical,
274 publisher = {Nature Publishing Group},
275 year = {2021},
276 pages = {582--584},
277 number = {4},
278 volume = {27},
279 journal = {Nature Medicine},
280 author = {Wu, Eric and Wu, Kevin and Daneshjou, Roxana and Ouyang, David and Ho, Daniel E and Zou, James},
281 title = {How medical AI devices are evaluated: limitations and recommendations from an analysis of FDA approvals},
282}
283
284@book{heppenheimer1995turbulent,
285 publisher = {Wiley New York},
286 year = {1995},
287 author = {Heppenheimer, Thomas A and Heppenheimer, Ta},
288 title = {Turbulent skies: the history of commercial aviation},
289}
290
291@book{silver2012signal,
292 publisher = {Penguin},
293 year = {2012},
294 author = {Silver, Nate},
295 title = {The signal and the noise: why so many predictions fail--but some don't},
296}
297
298@book{vinsel2019moving,
299 publisher = {JHU Press},
300 year = {2019},
301 author = {Vinsel, Lee},
302 title = {Moving Violations: Automobiles, Experts, and Regulations in the United States},
303}
304
305@article{nader1965unsafe,
306 year = {1965},
307 author = {Nader, Ralph},
308 title = {Unsafe at any speed. The designed-in dangers of the American automobile},
309}
310
311@book{bausell2009snake,
312 publisher = {Oxford University Press},
313 year = {2009},
314 author = {Bausell, R Barker},
315 title = {Snake oil science: The truth about complementary and alternative medicine},
316}
317
318@book{anderson2015snake,
319 publisher = {McFarland},
320 year = {2015},
321 author = {Anderson, Ann},
322 title = {Snake oil, hustlers and hambones: the American medicine show},
323}
324
325@book{blum2018poison,
326 publisher = {Penguin},
327 year = {2018},
328 author = {Blum, Deborah},
329 title = {The Poison Squad: One Chemist's Single-minded Crusade for Food Safety at the Turn of the Twentieth Century},
330}
331
332@article{moradi2021evaluating,
333 year = {2021},
334 journal = {arXiv preprint arXiv:2108.12237},
335 author = {Moradi, Milad and Samwald, Matthias},
336 title = {Evaluating the robustness of neural language models to input perturbations},
337}
338
339@article{pruthi2019combating,
340 year = {2019},
341 journal = {arXiv preprint arXiv:1905.11268},
342 author = {Pruthi, Danish and Dhingra, Bhuwan and Lipton, Zachary C},
343 title = {Combating adversarial misspellings with robust word recognition},
344}
345
346@article{berger2019mta,
347 year = {2019},
348 journal = {The Wall Street Journal},
349 author = {Berger, Paul},
350 title = {MTA’s Initial Foray Into Facial Recognition at High Speed Is a Bust},
351}
352
353@article{adadi2018peeking,
354 publisher = {IEEE},
355 year = {2018},
356 pages = {52138--52160},
357 volume = {6},
358 journal = {IEEE access},
359 author = {Adadi, Amina and Berrada, Mohammed},
360 title = {Peeking inside the black-box: a survey on explainable artificial intelligence (XAI)},
361}
362
363@inproceedings{bhatt2020explainable,
364 year = {2020},
365 pages = {648--657},
366 booktitle = {Proceedings of the 2020 Conference on Fairness, Accountability, and Transparency},
367 author = {Bhatt, Umang and Xiang, Alice and Sharma, Shubham and Weller, Adrian and Taly, Ankur and Jia, Yunhan and Ghosh, Joydeep and Puri, Ruchir and Moura, Jos{\'e} MF and Eckersley, Peter},
368 title = {Explainable machine learning in deployment},
369}
370
371@misc{OED,
372 howpublished = {\url{https://www.oed.com/view/Entry/54950742}},
373 year = {2021},
374 publisher = {Oxford University Press},
375 key = {OED Online},
376}
377
378@misc{AIAAIC,
379 howpublished = {\url{https://www.aiaaic.org/}},
380 year = {{2021}},
381 author = {{Charlie Pownall}},
382 title = {AI, Algorithmic and Automation Incident and Controversy Repository (AIAAIC)},
383}
384
385@misc{digiwatch,
386 howpublished = {\url{https://dig.watch/trends/covid-19-crisis-digital-policy-overview/}},
387 year = {{2021}},
388 author = {Digwatch},
389 publisher = {{Digwatch}},
390 title = {The COVID-19 crisis: A digital policy overview},
391}
392
393@article{krass2021us,
394 publisher = {British Medical Journal Publishing Group},
395 year = {2021},
396 volume = {372},
397 journal = {bmj},
398 author = {Krass, Mark and Henderson, Peter and Mello, Michelle M and Studdert, David M and Ho, Daniel E},
399 title = {How US law will evaluate artificial intelligence for covid-19},
400}
401
402@inproceedings{raghavan2020mitigating,
403 year = {2020},
404 pages = {469--481},
405 booktitle = {Proceedings of the 2020 conference on fairness, accountability, and transparency},
406 author = {Raghavan, Manish and Barocas, Solon and Kleinberg, Jon and Levy, Karen},
407 title = {Mitigating bias in algorithmic hiring: Evaluating claims and practices},
408}
409
410@inproceedings{wilson2021building,
411 year = {2021},
412 pages = {666--677},
413 booktitle = {Proceedings of the 2021 ACM Conference on Fairness, Accountability, and Transparency},
414 author = {Wilson, Christo and Ghosh, Avijit and Jiang, Shan and Mislove, Alan and Baker, Lewis and Szary, Janelle and Trindel, Kelly and Polli, Frida},
415 title = {Building and auditing fair algorithms: A case study in candidate screening},
416}
417
418@misc{engler2021independent,
419 year = {2021},
420 author = {Engler, Alex C},
421 title = {Independent auditors are struggling to hold AI companies accountable. FastCompany},
422}
423
424@incollection{shaban2021explainability,
425 publisher = {Springer},
426 year = {2021},
427 pages = {1--10},
428 booktitle = {Explainable AI in Healthcare and Medicine},
429 author = {Shaban-Nejad, Arash and Michalowski, Martin and Buckeridge, David L},
430 title = {Explainability and Interpretability: Keys to Deep Medicine},
431}
432
433@misc{missing_link_xai,
434 howpublished = {\url{https://www.h2o.ai/blog/interpretability-the-missing-link-between-machine-learning-healthcare-and-the-fda/}},
435 year = {2018},
436 author = {Andrew Langsner and Patrick Hall},
437 publisher = {H2O.ai Blog},
438 title = {Interpretability: The missing link between machine learning, healthcare, and the FDA?},
439}
440
441@misc{FDA,
442 howpublished = {\url{https://www.fda.gov/medical-devices/software-medical-device-samd/good-machine-learning-practice-medical-device-development-guiding-principles}},
443 year = {{2021}},
444 author = {U.S. Food and Drug Administration},
445 publisher = {{U.S. Food and Drug Administration}},
446 title = {Good Machine Learning Practice for Medical Device Development: Guiding Principles},
447}
448
449@misc{GDPR,
450 year = {2016},
451 title = {Regulation (EU) 2016/679 of the European Parliament and of the Council of 27 April 2016 on the protection of natural persons with regard to the processing of personal data and on the free movement of such data, and repealing Directive 95/46/EC (General Data Protection Regulation)},
452 key = {General Data Protection Regulation},
453}
454
455@article{maccarthy2019examination,
456 year = {2019},
457 journal = {Available at SSRN 3615731},
458 author = {MacCarthy, Mark},
459 title = {An Examination of the Algorithmic Accountability Act of 2019},
460}
461
462@inproceedings{feldman2015certifying,
463 year = {2015},
464 pages = {259--268},
465 booktitle = {proceedings of the 21th ACM SIGKDD international conference on knowledge discovery and data mining},
466 author = {Feldman, Michael and Friedler, Sorelle A and Moeller, John and Scheidegger, Carlos and Venkatasubramanian, Suresh},
467 title = {Certifying and removing disparate impact},
468}
469
470@article{veale_euact,
471 publisher = {Verlag Dr. Otto Schmidt},
472 year = {2021},
473 pages = {97--112},
474 number = {4},
475 volume = {22},
476 journal = {Computer Law Review International},
477 author = {Veale, Michael and Borgesius, Frederik Zuiderveen},
478 title = {Demystifying the Draft EU Artificial Intelligence Act—Analysing the good, the bad, and the unclear elements of the proposed approach},
479}
480
481@misc{goog_search_fail,
482 howpublished = {\url{https://itwire.com/home-it/how-a-google-search-could-end-up-endangering-a-life.html}},
483 year = {{2021}},
484 author = {Sam Varghese},
485 publisher = {{ITWire}},
486 title = {How a Google search could end up endangering a life},
487}
488
489@article{ettinger2020bert,
490 publisher = {MIT Press},
491 year = {2020},
492 pages = {34--48},
493 volume = {8},
494 journal = {Transactions of the Association for Computational Linguistics},
495 author = {Ettinger, Allyson},
496 title = {What BERT is not: Lessons from a new suite of psycholinguistic diagnostics for language models},
497}
498
499@misc{llmopenai,
500 primaryclass = {cs.CL},
501 archiveprefix = {arXiv},
502 eprint = {1908.09203},
503 year = {2019},
504 author = {Irene Solaiman and Miles Brundage and Jack Clark and Amanda Askell and Ariel Herbert-Voss and Jeff Wu and Alec Radford and Gretchen Krueger and Jong Wook Kim and Sarah Kreps and Miles McCain and Alex Newhouse and Jason Blazakis and Kris McGuffie and Jasmine Wang},
505 title = {Release Strategies and the Social Impacts of Language Models},
506}
507
508@article{llmdeepmind,
509 year = {2021},
510 journal = {arXiv preprint arXiv:2112.04359},
511 author = {Weidinger, Laura and Mellor, John and Rauh, Maribeth and Griffin, Conor and Uesato, Jonathan and Huang, Po-Sen and Cheng, Myra and Glaese, Mia and Balle, Borja and Kasirzadeh, Atoosa and others},
512 title = {Ethical and social risks of harm from Language Models},
513}
514
515@article{brundage2018malicious,
516 year = {2018},
517 journal = {arXiv preprint arXiv:1802.07228},
518 author = {Brundage, Miles and Avin, Shahar and Clark, Jack and Toner, Helen and Eckersley, Peter and Garfinkel, Ben and Dafoe, Allan and Scharre, Paul and Zeitzoff, Thomas and Filar, Bobby and others},
519 title = {The malicious use of artificial intelligence: Forecasting, prevention, and mitigation},
520}
521
522@article{jaques2019moral,
523 year = {2019},
524 volume = {10},
525 journal = {University of Miami School of Law},
526 author = {Jaques, Abby Everett},
527 title = {Why the moral machine is a monster},
528}
529
530@article{talat2021word,
531 year = {2021},
532 journal = {arXiv preprint arXiv:2111.04158},
533 author = {Talat, Zeerak and Blix, Hagen and Valvoda, Josef and Ganesh, Maya Indira and Cotterell, Ryan and Williams, Adina},
534 title = {A Word on Machine Ethics: A Response to Jiang et al.(2021)},
535}
536
537@article{jiang2021delphi,
538 year = {2021},
539 journal = {arXiv preprint arXiv:2110.07574},
540 author = {Jiang, Liwei and Hwang, Jena D and Bhagavatula, Chandra and Bras, Ronan Le and Forbes, Maxwell and Borchardt, Jon and Liang, Jenny and Etzioni, Oren and Sap, Maarten and Choi, Yejin},
541 title = {Delphi: Towards machine ethics and norms},
542}
543
544@article{awad2018moral,
545 publisher = {Nature Publishing Group},
546 year = {2018},
547 pages = {59--64},
548 number = {7729},
549 volume = {563},
550 journal = {Nature},
551 author = {Awad, Edmond and Dsouza, Sohan and Kim, Richard and Schulz, Jonathan and Henrich, Joseph and Shariff, Azim and Bonnefon, Jean-Fran{\c{c}}ois and Rahwan, Iyad},
552 title = {The moral machine experiment},
553}
554
555@misc{shane2019janelle,
556 year = {2019},
557 author = {Shane, J},
558 title = {Janelle Shane: The danger of AI is weirder than you think TED Talk, 10: 20. Katsottu 8.8, 2020},
559}
560
561@article{brundage2015taking,
562 publisher = {Elsevier},
563 year = {2015},
564 pages = {32--35},
565 volume = {72},
566 journal = {Futures},
567 author = {Brundage, Miles},
568 title = {Taking superintelligence seriously: Superintelligence: Paths, dangers, strategies by Nick Bostrom (Oxford University Press, 2014)},
569}
570
571@book{bostrom2014superintelligence,
572 publisher = {Oxford University Press},
573 year = {2014},
574 url = {https://books.google.com/books?id=7\_H8AwAAQBAJ},
575 lccn = {2013955152},
576 isbn = {9780199678112},
577 author = {Bostrom, N.},
578 title = {Superintelligence: Paths, Dangers, Strategies},
579}
580
581@inproceedings{prunkl2020beyond,
582 year = {2020},
583 pages = {138--143},
584 booktitle = {Proceedings of the AAAI/ACM Conference on AI, Ethics, and Society},
585 author = {Prunkl, Carina and Whittlestone, Jess},
586 title = {Beyond near-and long-term: Towards a clearer account of research priorities in AI ethics and society},
587}
588
589@article{atkinson2018going,
590 publisher = {IUP Publications},
591 year = {2018},
592 pages = {7--56},
593 number = {4},
594 volume = {12},
595 journal = {IUP Journal of Computer Sciences},
596 author = {Atkinson, Robert D},
597 title = {" It Is Going to Kill Us!" and Other Myths About the Future of Artificial Intelligence},
598}
599
600@article{crawford2016artificial,
601 year = {2016},
602 number = {06},
603 volume = {25},
604 journal = {The New York Times},
605 author = {Crawford, Kate},
606 title = {Artificial intelligence’s white guy problem},
607}
608
609@misc{covidfail_summ,
610 publisher = {MIT Technology Review},
611 year = {2021},
612 author = {Heaven, Will Douglas},
613 title = {Hundreds of AI tools have been built to catch covid. None of them helped},
614}
615
616@misc{covidfail3,
617 howpublished = {\url{https://www.turing.ac.uk/sites/default/files/2021-06/data-science-and-ai-in-the-age-of-covid_full-report_2.pdf}},
618 year = {{2021}},
619 author = {Inken von Borzyskowski, Anjali Mazumder, Bilal Mateen, Michael Wooldridge},
620 publisher = {{The Alan Turing Institute}},
621 title = {Data science and AI in the age of COVID-19},
622}
623
624@article{covidfail2,
625 publisher = {British Medical Journal Publishing Group},
626 year = {2020},
627 volume = {369},
628 journal = {bmj},
629 author = {Wynants, Laure and Van Calster, Ben and Collins, Gary S and Riley, Richard D and Heinze, Georg and Schuit, Ewoud and Bonten, Marc MJ and Dahly, Darren L and Damen, Johanna A and Debray, Thomas PA and others},
630 title = {Prediction models for diagnosis and prognosis of covid-19: systematic review and critical appraisal},
631}
632
633@article{covidfail1,
634 publisher = {Nature Publishing Group},
635 year = {2021},
636 pages = {199--217},
637 number = {3},
638 volume = {3},
639 journal = {Nature Machine Intelligence},
640 author = {Roberts, Michael and Driggs, Derek and Thorpe, Matthew and Gilbey, Julian and Yeung, Michael and Ursprung, Stephan and Aviles-Rivero, Angelica I and Etmann, Christian and McCague, Cathal and Beer, Lucian and others},
641 title = {Common pitfalls and recommendations for using machine learning to detect and prognosticate for COVID-19 using chest radiographs and CT scans},
642}
643
644@misc{NAIR,
645 howpublished = {\url{https://hai.stanford.edu/sites/default/files/2022-01/HAI_NRCR_v17.pdf}},
646 year = {{2021}},
647 author = {Daniel E. Ho, Jennifer King, Russell C. Wald, Christopher Wan},
648 publisher = {{Stanford University Human-centered Artificial Intelligence}},
649 title = {Building a National AI Research Resource: A Blueprint for the National Research Cloud},
650}
651
652@misc{NMIP,
653 howpublished = {\url{https://www.nhsx.nhs.uk/ai-lab/ai-lab-programmes/ai-in-imaging/national-medical-imaging-platform-nmip/}},
654 year = {{2021}},
655 author = {NHS AI Lab},
656 publisher = {{NHSx}},
657 title = {National Medical Imaging Platform (NMIP)},
658}
659
660@article{democratization2,
661 year = {2020},
662 author = {Awasthi, Pranjal and George, Jordana J},
663 title = {A case for Data Democratization},
664}
665
666@inproceedings{democratization3,
667 year = {2017},
668 pages = {5--3},
669 booktitle = {Datapower Conference Proceedings},
670 author = {Garvey, Colin K},
671 title = {On the Democratization of AI},
672}
673
674@incollection{democratization,
675 publisher = {transcript-Verlag},
676 year = {2020},
677 pages = {9--32},
678 booktitle = {The Democratization of Artificial Intelligence},
679 author = {Sudmann, Andreas},
680 title = {The Democratization of Artificial Intelligence},
681}
682
683@article{ahmed2020democratization,
684 year = {2020},
685 journal = {arXiv preprint arXiv:2010.15581},
686 author = {Ahmed, Nur and Wahed, Muntasir},
687 title = {The de-democratization of ai: Deep learning and the compute divide in artificial intelligence research},
688}
689
690@article{yeung2020recommendation,
691 publisher = {Cambridge University Press},
692 year = {2020},
693 pages = {27--34},
694 number = {1},
695 volume = {59},
696 journal = {International Legal Materials},
697 author = {Yeung, Karen},
698 title = {Recommendation of the council on artificial intelligence (oecd)},
699}
700
701@article{slota2020good,
702 publisher = {Wiley Online Library},
703 year = {2020},
704 pages = {e275},
705 number = {1},
706 volume = {57},
707 journal = {Proceedings of the Association for Information Science and Technology},
708 author = {Slota, Stephen C and Fleischmann, Kenneth R and Greenberg, Sherri and Verma, Nitin and Cummings, Brenna and Li, Lan and Shenefiel, Chris},
709 title = {Good systems, bad data?: Interpretations of AI hype and failures},
710}
711
712@inproceedings{raji2020saving,
713 year = {2020},
714 pages = {145--151},
715 booktitle = {Proceedings of the AAAI/ACM Conference on AI, Ethics, and Society},
716 author = {Raji, Inioluwa Deborah and Gebru, Timnit and Mitchell, Margaret and Buolamwini, Joy and Lee, Joonseok and Denton, Emily},
717 title = {Saving face: Investigating the ethical concerns of facial recognition auditing},
718}
719
720@article{barocas2021designing,
721 year = {2021},
722 journal = {arXiv preprint arXiv:2103.06076},
723 author = {Barocas, Solon and Guo, Anhong and Kamar, Ece and Krones, Jacquelyn and Morris, Meredith Ringel and Vaughan, Jennifer Wortman and Wadsworth, Duncan and Wallach, Hanna},
724 title = {Designing Disaggregated Evaluations of AI Systems: Choices, Considerations, and Tradeoffs},
725}
726
727@inproceedings{raji2019actionable,
728 year = {2019},
729 pages = {429--435},
730 booktitle = {Proceedings of the 2019 AAAI/ACM Conference on AI, Ethics, and Society},
731 author = {Raji, Inioluwa Deborah and Buolamwini, Joy},
732 title = {Actionable auditing: Investigating the impact of publicly naming biased performance results of commercial ai products},
733}
734
735@article{richardson2021defining,
736 year = {2021},
737 journal = {Maryland Law Review, Forthcoming},
738 author = {Richardson, Rashida},
739 title = {Defining and Demystifying Automated Decision Systems},
740}
741
742@article{narayanan2019recognize,
743 publisher = {Massachusetts Institute of Technology},
744 year = {2019},
745 journal = {Arthur Miller Lecture on Science and Ethics},
746 author = {Narayanan, Arvind},
747 title = {How to recognize AI snake oil},
748}
749
750@inproceedings{bender2021dangers,
751 year = {2021},
752 pages = {610--623},
753 booktitle = {Proceedings of the 2021 ACM Conference on Fairness, Accountability, and Transparency},
754 author = {Bender, Emily M and Gebru, Timnit and McMillan-Major, Angelina and Shmitchell, Shmargaret},
755 title = {On the Dangers of Stochastic Parrots: Can Language Models Be Too Big?},
756}
757
758@article{tennant2021attachments,
759 publisher = {SAGE Publications Sage UK: London, England},
760 year = {2021},
761 pages = {846--870},
762 number = {6},
763 volume = {51},
764 journal = {Social Studies of Science},
765 author = {Tennant, Chris and Stilgoe, Jack},
766 title = {The attachments of ‘autonomous’ vehicles},
767}
768
769@book{fake_ai,
770 publisher = {Meatspace Press},
771 year = {2021},
772 author = {Kaltheuner, Frederike and Birhane, Abeba and Raji, Inioluwa Deborah and Amironesei, Razvan and Denton, Emily and Hanna, Alex and Nicole, Hilary and Smart, Andrew and Oduro, Serena Dokuaa and Vincent, James and Reben, Alexander and Milne, Gemma and Black, Crofton and Harvey, Adam and Strait, Andrew and Parida, Tulsi and Ashok, Aparna and Jansen, Fieke and Cath, Corinne and Peppin, Aidan},
773 title = {Fake AI},
774}
775
776@book{broussard2018artificial,
777 publisher = {mit Press},
778 year = {2018},
779 author = {Broussard, Meredith},
780 title = {Artificial unintelligence: How computers misunderstand the world},
781}
782
783@article{sculley2015hidden,
784 year = {2015},
785 pages = {2503--2511},
786 volume = {28},
787 journal = {Advances in neural information processing systems},
788 author = {Sculley, David and Holt, Gary and Golovin, Daniel and Davydov, Eugene and Phillips, Todd and Ebner, Dietmar and Chaudhary, Vinay and Young, Michael and Crespo, Jean-Francois and Dennison, Dan},
789 title = {Hidden technical debt in machine learning systems},
790}
791
792@inproceedings{liao2021we,
793 year = {2021},
794 booktitle = {Thirty-fifth Conference on Neural Information Processing Systems Datasets and Benchmarks Track (Round 2)},
795 author = {Liao, Thomas and Taori, Rohan and Raji, Inioluwa Deborah and Schmidt, Ludwig},
796 title = {Are We Learning Yet? A Meta Review of Evaluation Failures Across Machine Learning},
797}
798
799@misc{kapoor_irreproducible_2021,
800 urldate = {2021-07-28},
801 url = {https://reproducible.cs.princeton.edu/},
802 howpublished = {\url{https://reproducible.cs.princeton.edu/}},
803 year = {2021},
804 pages = {6},
805 author = {Kapoor, Sayash and Narayanan, Arvind},
806 language = {en},
807 abstract = {The use of Machine Learning (ML) methods for prediction and forecasting has become widespread across the quantitative sciences. However, there are many known methodological pitfalls in ML-based research. As a case study of these pitfalls, we examine the subfield of civil war onset prediction in Political Science. Our main finding is that several recent studies published in top Political Science journals claiming superior performance of ML models over Logistic Regression models fail to reproduce. Our results provide two reasons to be skeptical of the use of ML methods in this research area, by both questioning their usefulness and highlighting the pitfalls of applying them correctly. Results identifying errors in studies that use ML methods have appeared in at least seven quantitative science fields. However, we go farther than most previous research to investigate whether the claims made in the reviewed studies survive once the errors are corrected. We argue that there is a reproducibility crisis brewing in research fields that use ML methods and discuss a few systemic interventions that could help resolve it.},
808 title = {({Ir}){Reproducible} {Machine} {Learning}: {A} {Case} {Study}},
809}
810
811@article{robertson2021engagement,
812 year = {2021},
813 journal = {arXiv preprint arXiv:2201.00074},
814 author = {Robertson, Ronald E and Green, Jon and Ruck, Damian and Ognyanova, Katya and Wilson, Christo and Lazer, David},
815 title = {Engagement Outweighs Exposure to Partisan and Unreliable News within Google Search},
816}
817
818@article{badnews,
819 howpublished = {\url{https://harpers.org/archive/2021/09/bad-news-selling-the-story-of-disinformation/}},
820 year = {2021},
821 journal = {Harper's Magazine},
822 author = {Bernstein, Joseph},
823 title = {Bad News},
824}
825
826@article{hern2018cambridge,
827 year = {2018},
828 volume = {6},
829 journal = {The Guardian},
830 author = {Hern, Alex},
831 title = {Cambridge Analytica: how did it turn clicks into votes},
832}
833
834@article{gibney2018scant,
835 year = {2018},
836 journal = {Nature},
837 author = {Gibney, Elizabeth},
838 title = {The scant science behind Cambridge Analytica’s controversial marketing techniques},
839}
840
841@article{matz2017psychological,
842 publisher = {National Acad Sciences},
843 year = {2017},
844 pages = {12714--12719},
845 number = {48},
846 volume = {114},
847 journal = {Proceedings of the national academy of sciences},
848 author = {Matz, Sandra C and Kosinski, Michal and Nave, Gideon and Stillwell, David J},
849 title = {Psychological targeting as an effective approach to digital mass persuasion},
850}
851
852@book{hwang2020subprime,
853 publisher = {FSG originals},
854 year = {2020},
855 author = {Hwang, Tim},
856 title = {Subprime attention crisis: advertising and the time bomb at the heart of the Internet},
857}
858
859@article{pineau2021improving,
860 publisher = {Microtome Publishing},
861 year = {2021},
862 volume = {22},
863 journal = {Journal of Machine Learning Research},
864 author = {Pineau, Joelle and Vincent-Lamarre, Philippe and Sinha, Koustuv and Larivi{\`e}re, Vincent and Beygelzimer, Alina and d’Alch{\'e}-Buc, Florence and Fox, Emily and Larochelle, Hugo},
865 title = {Improving reproducibility in machine learning research: a report from the NeurIPS 2019 reproducibility program},
866}
867
868@article{firestone2020performance,
869 publisher = {National Acad Sciences},
870 year = {2020},
871 pages = {26562--26571},
872 number = {43},
873 volume = {117},
874 journal = {Proceedings of the National Academy of Sciences},
875 author = {Firestone, Chaz},
876 title = {Performance vs. competence in human--machine comparisons},
877}
878
879@article{grover,
880 year = {2021},
881 journal = {arXiv preprint arXiv:2111.15366},
882 author = {Raji, Inioluwa Deborah and Bender, Emily M and Paullada, Amandalynne and Denton, Emily and Hanna, Alex},
883 title = {AI and the everything in the whole wide world benchmark},
884}
885
886@article{diaz2021double,
887 howpublished = {\url{https://www. brennancenter. org/sites/default/files/2021-08/Double\_Standards\_Content\_Moderation. pdf}},
888 year = {2021},
889 journal = {New York: Brennan Center for Justice},
890 author = {D{\'\i}az, {\'A}ngel and Hecht, Laura},
891 title = {Double Standards in Social Media Content Moderation},
892}
893
894@inproceedings{oakden2020hidden,
895 year = {2020},
896 pages = {151--159},
897 booktitle = {Proceedings of the ACM conference on health, inference, and learning},
898 author = {Oakden-Rayner, Luke and Dunnmon, Jared and Carneiro, Gustavo and R{\'e}, Christopher},
899 title = {Hidden stratification causes clinically meaningful failures in machine learning for medical imaging},
900}
901
902@article{freeman2021use,
903 publisher = {British Medical Journal Publishing Group},
904 year = {2021},
905 volume = {374},
906 journal = {bmj},
907 author = {Freeman, Karoline and Geppert, Julia and Stinton, Chris and Todkill, Daniel and Johnson, Samantha and Clarke, Aileen and Taylor-Phillips, Sian},
908 title = {Use of artificial intelligence for image analysis in breast cancer screening programmes: systematic review of test accuracy},
909}
910
911@misc{pain_wired,
912 howpublished = {\url{https://www.wired.com/story/opioid-drug-addiction-algorithm-chronic-pain/}},
913 year = {{2021}},
914 author = {Szalavitz, Maia},
915 publisher = {{Wired}},
916 title = {The Pain Was Unbearable. So Why Did Doctors Turn Her Away?},
917}
918
919@article{obermeyer2019dissecting,
920 publisher = {American Association for the Advancement of Science},
921 year = {2019},
922 pages = {447--453},
923 number = {6464},
924 volume = {366},
925 journal = {Science},
926 author = {Obermeyer, Ziad and Powers, Brian and Vogeli, Christine and Mullainathan, Sendhil},
927 title = {Dissecting racial bias in an algorithm used to manage the health of populations},
928}
929
930@article{paige2020houston,
931 publisher = {SAGE Publications Sage CA: Los Angeles, CA},
932 year = {2020},
933 pages = {350--359},
934 number = {5},
935 volume = {49},
936 journal = {Educational Researcher},
937 author = {Paige, Mark A and Amrein-Beardsley, Audrey},
938 title = {“Houston, We Have a Lawsuit”: A Cautionary Tale for the Implementation of Value-Added Models for High-Stakes Employment Decisions},
939}
940
941@article{richardson2019litigating,
942 year = {2019},
943 journal = {AI Now Institute, September},
944 author = {Richardson, Rashida and Schultz, Jason M and Southerland, Vincent M},
945 title = {Litigating Algorithms: 2019 US Report},
946}
947
948@misc{fb_nudity,
949 howpublished = {\url{https://www.businessinsider.com/facebook-mistakes-onions-for-sexualised-content-2020-10}},
950 year = {{2020}},
951 author = {Hamilton, Isobel Asher},
952 publisher = {{Business Insider}},
953 title = {Facebook's nudity-spotting AI mistook a photo of some onions for 'sexually suggestive' content},
954}
955
956@misc{fb_hoes,
957 howpublished = {\url{https://nypost.com/2021/07/20/facebook-cracks-down-on-discussing-hoes-in-gardening-group/}},
958 year = {{2021}},
959 author = {O’Neill, Jesse},
960 publisher = {{New York Post}},
961 title = {Facebook cracks down on discussing ‘hoes’ in gardening group},
962}
963
964@article{tiktokerror,
965 howpublished = {\url{https://i-d.vice.com/en_uk/article/m7epya/tiktoks-algorithm-reportedly-bans-creators-using-terms-black-and-blm}},
966 year = {2021},
967 journal = {The Verge},
968 author = {Kpakima, Kumba},
969 title = {Tiktok’s algorithm reportedly bans creators using terms 'Black' and 'BLM'},
970}
971
972@article{lecher2018happens,
973 year = {2018},
974 journal = {The Verge},
975 author = {Lecher, Colin},
976 title = {What happens when an algorithm cuts your health care},
977}
978
979@misc{alevels,
980 publisher = {The Guardian},
981 year = {2021},
982 author = {Lamont, Tom},
983 title = {The student and the algorithm: how the exam results fiasco threatened one pupil’s future},
984}
985
986@article{kippin2021covid,
987 publisher = {Springer},
988 year = {2021},
989 pages = {1--23},
990 journal = {British Politics},
991 author = {Kippin, Sean and Cairney, Paul},
992 title = {The COVID-19 exams fiasco across the UK: four nations and two windows of opportunity},
993}
994
995@article{hill2020wrongfully,
996 year = {2020},
997 volume = {24},
998 journal = {The New York Times},
999 author = {Hill, Kashmir},
1000 title = {Wrongfully accused by an algorithm},
1001}
1002
1003@article{kirchner2020access,
1004 journal = {The Markup},
1005 year = {2020},
1006 author = {Kirchner, Lauren and Goldstein, Matthew},
1007 title = {Access Denied: Faulty Automated Background Checks Freeze Out Renters},
1008}
1009
1010@article{kirchner2020automated,
1011 year = {2020},
1012 volume = {28},
1013 month = {May},
1014 journal = {The New York Times},
1015 author = {Kirchner, Lauren and Goldstein, Matthew},
1016 title = {How Automated Background Checks Freeze Out Renters},
1017}
1018
1019@misc{bankrupt_MIDAS,
1020 howpublished = {\url{https://www.freep.com/story/news/local/michigan/2019/12/22/government-artificial-intelligence-midas-computer-fraud-fiasco/4407901002/}},
1021 year = {{2019}},
1022 author = {Egan, Paul},
1023 booktitle = {{Detroit Free Press}},
1024 title = {State of Michigan's mistake led to man filing bankruptcy},
1025}
1026
1027@article{charette2018michigan,
1028 year = {2018},
1029 pages = {6},
1030 number = {3},
1031 volume = {18},
1032 journal = {IEEE Spectrum},
1033 author = {Charette, Robert},
1034 title = {Michigan’s MiDAS Unemployment System: Algorithm Alchemy Created Lead, Not Gold-IEEE Spectrum},
1035}
1036
1037@article{barocas2016big,
1038 publisher = {HeinOnline},
1039 year = {2016},
1040 pages = {671},
1041 volume = {104},
1042 journal = {Calif. L. Rev.},
1043 author = {Barocas, Solon and Selbst, Andrew D},
1044 title = {Big data's disparate impact},
1045}
1046
1047@article{kaminski2019right,
1048 year = {2019},
1049 pages = {189},
1050 volume = {34},
1051 journal = {Berkeley Technology Law Journal},
1052 author = {Kaminski, Margot E},
1053 title = {The Right to Explanation, Explained},
1054}
1055
1056@article{kaminski2021right,
1057 publisher = {JSTOR},
1058 year = {2021},
1059 pages = {1957--2048},
1060 number = {7},
1061 volume = {121},
1062 journal = {Columbia Law Review},
1063 author = {Kaminski, Margot E and Urban, Jennifer M},
1064 title = {The right to contest AI},
1065}
1066
1067@inproceedings{barocas2020hidden,
1068 year = {2020},
1069 pages = {80--89},
1070 booktitle = {Proceedings of the 2020 Conference on Fairness, Accountability, and Transparency},
1071 author = {Barocas, Solon and Selbst, Andrew D and Raghavan, Manish},
1072 title = {The hidden assumptions behind counterfactual explanations and principal reasons},
1073}
1074
1075@article{edwards2017slave,
1076 publisher = {HeinOnline},
1077 year = {2017},
1078 pages = {18},
1079 volume = {16},
1080 journal = {Duke L. \& Tech. Rev.},
1081 author = {Edwards, Lilian and Veale, Michael},
1082 title = {Slave to the algorithm: Why a right to an explanation is probably not the remedy you are looking for},
1083}
1084
1085@article{selbst2017meaningful,
1086 publisher = {Oxford University Press},
1087 year = {2017},
1088 pages = {233--242},
1089 number = {4},
1090 volume = {7},
1091 journal = {International Data Privacy Law},
1092 author = {Selbst, Andrew D and Powles, Julia},
1093 title = {Meaningful information and the right to explanation},
1094}
1095
1096@article{wachter2017right,
1097 publisher = {Oxford University Press},
1098 year = {2017},
1099 pages = {76--99},
1100 number = {2},
1101 volume = {7},
1102 journal = {International Data Privacy Law},
1103 author = {Wachter, Sandra and Mittelstadt, Brent and Floridi, Luciano},
1104 title = {Why a right to explanation of automated decision-making does not exist in the general data protection regulation},
1105}
1106
1107@book{hartzog2018privacy,
1108 publisher = {Harvard University Press},
1109 year = {2018},
1110 author = {Hartzog, Woodrow},
1111 title = {Privacy’s blueprint},
1112}
1113
1114@article{selbst2020negligence,
1115 publisher = {HeinOnline},
1116 year = {2020},
1117 pages = {1315},
1118 volume = {100},
1119 journal = {BUL Rev.},
1120 author = {Selbst, Andrew D},
1121 title = {Negligence and AI's human users},
1122}
1123
1124@book{hoofnagle2016federal,
1125 publisher = {Cambridge University Press},
1126 year = {2016},
1127 author = {Hoofnagle, Chris Jay},
1128 title = {Federal Trade Commission: Privacy Law and Policy},
1129}
1130
1131@article{calo2015robotics,
1132 publisher = {HeinOnline},
1133 year = {2015},
1134 pages = {513},
1135 volume = {103},
1136 journal = {Calif. L. Rev.},
1137 author = {Calo, Ryan},
1138 title = {Robotics and the Lessons of Cyberlaw},
1139}
1140
1141@article{engstrom20133D,
1142 publisher = {HeinOnline},
1143 year = {2013},
1144 pages = {35},
1145 volume = {162},
1146 journal = {U. Pa. L. Rev. Online},
1147 author = {Engstrom, Nora Freeman},
1148 title = {3-D printing and product liability: identifying the obstacles},
1149}
1150
1151@article{zollers2004no,
1152 publisher = {HeinOnline},
1153 year = {2004},
1154 pages = {745},
1155 volume = {21},
1156 journal = {Santa Clara Computer \& High Tech. LJ},
1157 author = {Zollers, Frances E and McMullin, Andrew and Hurd, Sandra N and Shears, Peter},
1158 title = {No more soft landings for software: Liability for defects in an industry that has come of age},
1159}
1160
1161@misc{Winter,
1162 year = {1991},
1163 key = {Winter v. G.P. Putnam's Sons, 938 F.2d 1033 (9th Cir. 1991)},
1164}
1165
1166@article{hubbard2014sophisticated,
1167 publisher = {HeinOnline},
1168 year = {2014},
1169 pages = {1803},
1170 volume = {66},
1171 journal = {Fla. L. Rev.},
1172 author = {Hubbard, F Patrick},
1173 title = {Sophisticated robots: balancing liability, regulation, and innovation},
1174}
1175
1176@article{owen2001manufacturing,
1177 publisher = {HeinOnline},
1178 year = {2001},
1179 pages = {851},
1180 volume = {53},
1181 journal = {SCL Rev.},
1182 author = {Owen, David G},
1183 title = {Manufacturing Defects},
1184}
1185
1186@article{geistfeld2017roadmap,
1187 publisher = {HeinOnline},
1188 year = {2017},
1189 pages = {1611},
1190 volume = {105},
1191 journal = {Calif. L. Rev.},
1192 author = {Geistfeld, Mark A},
1193 title = {A roadmap for autonomous vehicles: State tort liability, automobile insurance, and federal safety regulation},
1194}
1195
1196@article{choi2019crashworthy,
1197 publisher = {HeinOnline},
1198 year = {2019},
1199 pages = {39},
1200 volume = {94},
1201 journal = {Wash. L. Rev.},
1202 author = {Choi, Bryan H},
1203 title = {Crashworthy code},
1204}
1205
1206@misc{ThirdRestatement_S2,
1207 year = {},
1208 key = {Restatement (Third) of Torts: Products Liability § 2},
1209}
1210
1211@misc{ThirdRestatement_S3,
1212 year = {},
1213 key = {Restatement (Third) of Torts: Products Liability § 3},
1214}
1215
1216@misc{UCC_2-314,
1217 year = {},
1218 key = {Uniform Commercial Code § 2-314},
1219}
1220
1221@misc{UCC_2-315,
1222 year = {},
1223 key = {Uniform Commercial Code § 2-315},
1224}
1225
1226@misc{Section_5,
1227 year = {},
1228 key = {Federal Trade Commission Act, 15 U.S.C. § 45},
1229}
1230
1231@misc{CFPB_jx,
1232 year = {},
1233 key = {12 U.S.C. § 5511},
1234}
1235
1236@misc{CPSC_about,
1237 title = {About Us},
1238 howpublished = {\url{https://www.cpsc.gov/About-CPSC}},
1239 author = {Consumer Product Safety Commission},
1240}
1241
1242@article{citron2007technological,
1243 publisher = {HeinOnline},
1244 year = {2007},
1245 pages = {1249},
1246 volume = {85},
1247 journal = {Wash. UL Rev.},
1248 author = {Citron, Danielle Keats},
1249 title = {Technological due process},
1250}
1251
1252@misc{Zhang,
1253 year = {2013},
1254 title = {\textup{Zhang v. Superior Ct., 304 P.3d 163 (2013)}},
1255 author = {},
1256}
1257
1258@misc{AMG_FTC,
1259 year = {2021},
1260 title = {\textup{AMG Capital Management v. Federal Trade Commission, 141 S.Ct. 1341}},
1261 author = {},
1262}
1263
1264@article{mcgeveran2018duty,
1265 publisher = {HeinOnline},
1266 year = {2018},
1267 pages = {1135},
1268 volume = {103},
1269 journal = {Minn. L. Rev.},
1270 author = {McGeveran, William},
1271 title = {The Duty of Data Security},
1272}
1273
1274@misc{Snapchat_consent_decree,
1275 year = {2014},
1276 title = {\textup{In re Snapchat, Inc., File No. 132-3078, Docket No. C-4501 (consent decree)}},
1277 author = {Federal Trade Commission},
1278}
1279
1280@misc{FB_consent_decree,
1281 year = {2019},
1282 title = {\textup{Stipulated Order for Civil Penalty, Monetary Judgment, and Injunctive Relief, No. 1:19-cv-2184, Docket 2-1 (D.D.C. July 24, 2019) (fining Facebook \$5 billion for violating a prior consent decree)}},
1283 author = {},
1284}
1285
1286@misc{FTC_Mag_Moss,
1287 month = {July 1},
1288 year = {2021},
1289 howpublished = {\url{https://www.ftc.gov/news-events/press-releases/2021/07/ftc-votes-update-rulemaking-procedures-sets-stage-stronger}},
1290 title = {FTC Votes to Update Rulemaking Procedures, Sets Stage for Stronger Deterrence of Corporate Misconduct},
1291 author = {Federal Trade Commission},
1292}
1293
1294@article{citron2016privacy,
1295 publisher = {HeinOnline},
1296 year = {2016},
1297 pages = {747},
1298 volume = {92},
1299 journal = {Notre Dame L. Rev.},
1300 author = {Citron, Danielle Keats},
1301 title = {The Privacy Policymaking of State Attorneys General},
1302}
1303
1304@techreport{NCLC_Report,
1305 month = {02},
1306 year = {2009},
1307 author = {Carter, Carolyn L.},
1308 institution = {National Consumer Law Center},
1309 title = {Consumer Protection in the States},
1310}
1311
1312@article{methods_in_the_magic,
1313 url = {
1314https://doi.org/10.1080/03637751.2017.1375130
1315},
1316 doi = {10.1080/03637751.2017.1375130},
1317 publisher = {Routledge},
1318 year = {2018},
1319 pages = {57-80},
1320 number = {1},
1321 volume = {85},
1322 journal = {Communication Monographs},
1323 title = {Situating methods in the magic of Big Data and AI},
1324 author = {M. C. Elish and danah boyd},
1325}
1326
1327@article{ml_software_practices,
1328 doi = {10.1109/TSE.2019.2937083},
1329 pages = {1857-1871},
1330 number = {9},
1331 volume = {47},
1332 year = {2021},
1333 title = {How does Machine Learning Change Software Development Practices?},
1334 journal = {IEEE Transactions on Software Engineering},
1335 author = {Wan, Zhiyuan and Xia, Xin and Lo, David and Murphy, Gail C.},
1336}
1337
1338@misc{nao_ets,
1339 author = {National Audit Office},
1340 month = {Jul},
1341 year = {2020},
1342 journal = {National Audit Office},
1343 url = {https://www.nao.org.uk/press-release/investigation-into-the-response-to-cheating-in-english-language-tests/},
1344 title = {Investigation into the response to cheating in English language tests - national audit office (NAO) press release},
1345}
1346
1347@article{Mitchell2021-pk,
1348 year = {2021},
1349 month = {March},
1350 pages = {141--163},
1351 number = {1},
1352 volume = {8},
1353 publisher = {Annual Reviews},
1354 journal = {Annu. Rev. Stat. Appl.},
1355 abstract = {A recent wave of research has attempted to define fairness
1356quantitatively. In particular, this work has explored what
1357fairness might mean in the context of decisions based on the
1358predictions of statistical and machine learning models. The
1359rapid growth of this new field has led to wildly inconsistent
1360motivations, terminology, and notation, presenting a serious
1361challenge for cataloging and comparing definitions. This article
1362attempts to bring much-needed order. First, we explicate the
1363various choices and assumptions made?often implicitly?to justify
1364the use of prediction-based decision-making. Next, we show how
1365such choices and assumptions can raise fairness concerns and we
1366present a notationally consistent catalog of fairness
1367definitions from the literature. In doing so, we offer a concise
1368reference for thinking through the choices, assumptions, and
1369fairness considerations of prediction-based decision-making.},
1370 author = {Mitchell, Shira and Potash, Eric and Barocas, Solon and D'Amour,
1371Alexander and Lum, Kristian},
1372 title = {Algorithmic Fairness: Choices, Assumptions, and Definitions},
1373}
1374
1375@article{Raji2019-od,
1376 eprint = {1912.06166},
1377 primaryclass = {cs.CY},
1378 archiveprefix = {arXiv},
1379 year = {2019},
1380 month = {December},
1381 abstract = {We present the ``Annotation and Benchmarking on
1382Understanding and Transparency of Machine Learning
1383Lifecycles'' (ABOUT ML) project as an initiative to
1384operationalize ML transparency and work towards a standard
1385ML documentation practice. We make the case for the
1386project's relevance and effectiveness in consolidating
1387disparate efforts across a variety of stakeholders, as well
1388as bringing in the perspectives of currently missing voices
1389that will be valuable in shaping future conversations. We
1390describe the details of the initiative and the gaps we hope
1391this project will help address.},
1392 author = {Raji, Inioluwa Deborah and Yang, Jingying},
1393 title = {{ABOUT} {ML}: Annotation and Benchmarking on Understanding
1394and Transparency of Machine Learning Lifecycles},
1395}
1396
1397@inproceedings{Karimi2021-jo,
1398 location = {Virtual Event, Canada},
1399 keywords = {consequential recommendations, algorithmic recourse, explainable
1400artificial intelligence, causal inference, counterfactual
1401explanations, contrastive explanations, minimal interventions},
1402 address = {New York, NY, USA},
1403 year = {2021},
1404 month = {March},
1405 series = {FAccT '21},
1406 pages = {353--362},
1407 publisher = {Association for Computing Machinery},
1408 abstract = {As machine learning is increasingly used to inform consequential
1409decision-making (e.g., pre-trial bail and loan approval), it
1410becomes important to explain how the system arrived at its
1411decision, and also suggest actions to achieve a favorable
1412decision. Counterfactual explanations -``how the world would
1413have (had) to be different for a desirable outcome to occur''-
1414aim to satisfy these criteria. Existing works have primarily
1415focused on designing algorithms to obtain counterfactual
1416explanations for a wide range of settings. However, it has
1417largely been overlooked that ultimately, one of the main
1418objectives is to allow people to act rather than just
1419understand. In layman's terms, counterfactual explanations
1420inform an individual where they need to get to, but not how to
1421get there. In this work, we rely on causal reasoning to caution
1422against the use of counterfactual explanations as a
1423recommendable set of actions for recourse. Instead, we propose a
1424shift of paradigm from recourse via nearest counterfactual
1425explanations to recourse through minimal interventions, shifting
1426the focus from explanations to interventions.},
1427 author = {Karimi, Amir-Hossein and Sch{\"o}lkopf, Bernhard and Valera,
1428Isabel},
1429 booktitle = {Proceedings of the 2021 {ACM} Conference on Fairness,
1430Accountability, and Transparency},
1431 title = {Algorithmic Recourse: from Counterfactual Explanations to
1432Interventions},
1433}
1434
1435@article{Jobin2019-oa,
1436 language = {en},
1437 year = {2019},
1438 month = {September},
1439 pages = {389--399},
1440 number = {9},
1441 volume = {1},
1442 publisher = {Nature Publishing Group},
1443 journal = {Nature Machine Intelligence},
1444 abstract = {In the past five years, private companies, research institutions
1445and public sector organizations have issued principles and
1446guidelines for ethical artificial intelligence (AI). However,
1447despite an apparent agreement that AI should be `ethical', there
1448is debate about both what constitutes `ethical AI' and which
1449ethical requirements, technical standards and best practices are
1450needed for its realization. To investigate whether a global
1451agreement on these questions is emerging, we mapped and analysed
1452the current corpus of principles and guidelines on ethical AI.
1453Our results reveal a global convergence emerging around five
1454ethical principles (transparency, justice and fairness,
1455non-maleficence, responsibility and privacy), with substantive
1456divergence in relation to how these principles are interpreted,
1457why they are deemed important, what issue, domain or actors they
1458pertain to, and how they should be implemented. Our findings
1459highlight the importance of integrating guideline-development
1460efforts with substantive ethical analysis and adequate
1461implementation strategies. As AI technology develops rapidly, it
1462is widely recognized that ethical guidelines are required for
1463safe and fair implementation in society. But is it possible to
1464agree on what is `ethical AI'? A detailed analysis of 84 AI
1465ethics reports around the world, from national and international
1466organizations, companies and institutes, explores this question,
1467finding a convergence around core principles but substantial
1468divergence on practical implementation.},
1469 author = {Jobin, Anna and Ienca, Marcello and Vayena, Effy},
1470 title = {The global landscape of {AI} ethics guidelines},
1471}
1472
1473@misc{noonetrustai-xo,
1474 note = {Accessed: 2022-1-6},
1475 howpublished = {\url{https://cpr.unu.edu/publications/articles/ai-global-governance-no-one-should-trust-ai.html}},
1476 title = {{AI} \& Global Governance: No One Should Trust {AI} - United
1477Nations University Centre for Policy Research},
1478 author = {Bryson,Joanna},
1479}
1480
1481@unpublished{Stanton2021-oa,
1482 year = {2021},
1483 month = {March},
1484 author = {Stanton, Brian and Jensen, Theodore},
1485 title = {Trust and Artificial Intelligence},
1486}
1487
1488@misc{aclu-comment-trust,
1489 author = {ACLU},
1490 month = {September},
1491 year = {2021},
1492 note = {Accessed: 2022-1-6},
1493 howpublished = {\url{https://www.aclu.org/letter/aclu-comment-nists-proposal-managing-bias-ai}},
1494 title = {{ACLU} Comment on {NIST's} Proposal for Managing Bias in {AI}},
1495}
1496
1497@article{ieee_dictionary_dependability,
1498 keywords = {Standards;IEEE Standards;Patents;Software
1499measurement;Dictionaries;Warranties;Trademarks;availability;dependability;maintainability;and
1500reliability},
1501 year = {2006},
1502 month = {May},
1503 pages = {1--41},
1504 author = {IEEE},
1505 journal = {IEEE Std 982. 1-2005 (Revision of IEEE Std 982. 1-1988)},
1506 abstract = {A Standard Dictionary of Measures of the Software Aspects of
1507Dependability for assessing and predicting the reliability,
1508maintainability, and availability of any software system; in
1509particular, it applies to mission critical software systems.},
1510 title = {{IEEE} Standard Dictionary of Measures of the Software Aspects of
1511Dependability},
1512}
1513
1514@inproceedings{Passi2019-av,
1515 location = {Atlanta, GA, USA},
1516 keywords = {Problem Formulation, Machine Learning, Fairness, Data Science,
1517Target Variable},
1518 address = {New York, NY, USA},
1519 year = {2019},
1520 month = {January},
1521 series = {FAT* '19},
1522 pages = {39--48},
1523 publisher = {Association for Computing Machinery},
1524 abstract = {Formulating data science problems is an uncertain and difficult
1525process. It requires various forms of discretionary work to
1526translate high-level objectives or strategic goals into
1527tractable problems, necessitating, among other things, the
1528identification of appropriate target variables and proxies.
1529While these choices are rarely self-evident, normative
1530assessments of data science projects often take them for
1531granted, even though different translations can raise profoundly
1532different ethical concerns. Whether we consider a data science
1533project fair often has as much to do with the formulation of the
1534problem as any property of the resulting model. Building on six
1535months of ethnographic fieldwork with a corporate data science
1536team---and channeling ideas from sociology and history of
1537science, critical data studies, and early writing on knowledge
1538discovery in databases---we describe the complex set of actors
1539and activities involved in problem formulation. Our research
1540demonstrates that the specification and operationalization of
1541the problem are always negotiated and elastic, and rarely worked
1542out with explicit normative considerations in mind. In so doing,
1543we show that careful accounts of everyday data science work can
1544help us better understand how and why data science problems are
1545posed in certain ways---and why specific formulations prevail in
1546practice, even in the face of what might seem like normatively
1547preferable alternatives. We conclude by discussing the
1548implications of our findings, arguing that effective normative
1549interventions will require attending to the practical work of
1550problem formulation.},
1551 author = {Passi, Samir and Barocas, Solon},
1552 booktitle = {Proceedings of the Conference on Fairness, Accountability, and
1553Transparency},
1554 title = {Problem Formulation and Fairness},
1555}
1556
1557@article{Passi2020-dr,
1558 year = {2020},
1559 month = {July},
1560 pages = {2053951720939605},
1561 number = {2},
1562 volume = {7},
1563 publisher = {SAGE Publications Ltd},
1564 journal = {Big Data \& Society},
1565 abstract = {How are data science systems made to work? It may seem that
1566whether a system works is a function of its technical design,
1567but it is also accomplished through ongoing forms of
1568discretionary work by many actors. Based on six months of
1569ethnographic fieldwork with a corporate data science team, we
1570describe how actors involved in a corporate project negotiated
1571what work the system should do, how it should work, and how to
1572assess whether it works. These negotiations laid the foundation
1573for how, why, and to what extent the system ultimately worked.
1574We describe three main findings. First, how already-existing
1575technologies are essential reference points to determine how and
1576whether systems work. Second, how the situated resolution of
1577development challenges continually reshapes the understanding of
1578how and whether systems work. Third, how business goals, and
1579especially their negotiated balance with data science
1580imperatives, affect a system?s working. We conclude with
1581takeaways for critical data studies, orienting researchers to
1582focus on the organizational and cultural aspects of data
1583science, the third-party platforms underlying data science
1584systems, and ways to engage with practitioners? imagination of
1585how systems can and should work.},
1586 author = {Passi, Samir and Sengers, Phoebe},
1587 title = {Making data science systems work},
1588}
1589
1590@inproceedings{Muller2019-cy,
1591 location = {Glasgow, Scotland Uk},
1592 keywords = {work practice, data science},
1593 address = {New York, NY, USA},
1594 year = {2019},
1595 month = {May},
1596 series = {CHI EA '19},
1597 pages = {1--8},
1598 number = {Paper W15},
1599 publisher = {Association for Computing Machinery},
1600 abstract = {With the rise of big data, there has been an increasing need to
1601understand who is working in data science and how they are doing
1602their work. HCI and CSCW researchers have begun to examine these
1603questions. In this workshop, we invite researchers to share
1604their observations, experiences, hypotheses, and insights, in
1605the hopes of developing a taxonomy of work practices and open
1606issues in the behavioral and social study of data science and
1607data science workers.},
1608 author = {Muller, Michael and Feinberg, Melanie and George, Timothy and
1609Jackson, Steven J and John, Bonnie E and Kery, Mary Beth and
1610Passi, Samir},
1611 booktitle = {Extended Abstracts of the 2019 {CHI} Conference on Human Factors
1612in Computing Systems},
1613 title = {{Human-Centered} Study of Data Science Work Practices},
1614}
1615
1616@article{Passi2018-jt,
1617 keywords = {collaboration, organizational work, data science, trust,
1618credibility},
1619 address = {New York, NY, USA},
1620 year = {2018},
1621 month = {November},
1622 pages = {1--28},
1623 number = {CSCW},
1624 volume = {2},
1625 publisher = {Association for Computing Machinery},
1626 journal = {Proc. ACM Hum.-Comput. Interact.},
1627 abstract = {The trustworthiness of data science systems in applied and
1628real-world settings emerges from the resolution of specific
1629tensions through situated, pragmatic, and ongoing forms of work.
1630Drawing on research in CSCW, critical data studies, and history
1631and sociology of science, and six months of immersive
1632ethnographic fieldwork with a corporate data science team, we
1633describe four common tensions in applied data science work:
1634(un)equivocal numbers, (counter)intuitive knowledge,
1635(in)credible data, and (in)scrutable models. We show how
1636organizational actors establish and re-negotiate trust under
1637messy and uncertain analytic conditions through practices of
1638skepticism, assessment, and credibility. Highlighting the
1639collaborative and heterogeneous nature of real-world data
1640science, we show how the management of trust in applied
1641corporate data science settings depends not only on
1642pre-processing and quantification, but also on negotiation and
1643translation. We conclude by discussing the implications of our
1644findings for data science research and practice, both within and
1645beyond CSCW.},
1646 author = {Passi, Samir and Jackson, Steven J},
1647 title = {Trust in Data Science: Collaboration, Translation, and
1648Accountability in Corporate Data Science Projects},
1649}
1650
1651@misc{Lehr_undated-aq,
1652 note = {Accessed: 2021-8-10},
1653 howpublished = {\url{https://lawreview.law.ucdavis.edu/issues/51/2/Symposium/51-2_Lehr_Ohm.pdf}},
1654 author = {Lehr, David and Ohm, Paul},
1655 title = {Playing with the data: What legal scholars should learn about
1656machine learning},
1657}
1658
1659@article{Henke2018-ua,
1660 year = {2018},
1661 month = {February},
1662 journal = {Harvard Business Review},
1663 abstract = {It's easier for companies to train existing employees for it than
1664to hire new ones.},
1665 author = {Henke, Nicolaus and Levine, Jordan and McInerney, Paul},
1666 title = {You Don't Have to Be a Data Scientist to Fill This {Must-Have}
1667Analytics Role},
1668}
1669
1670@article{scalefactor,
1671 language = {en},
1672 year = {2020},
1673 month = {July},
1674 journal = {Forbes Magazine},
1675 abstract = {Kurt Rathmann told his big-name investors he had developed
1676groundbreaking AI to do the books for small businesses. In
1677reality, humans did most of the work.},
1678 author = {Jeans, David},
1679 title = {{ScaleFactor} Raised \$100 Million In A Year Then Blamed Covid-19
1680For Its Demise. Employees Say It Had Much Bigger Problems},
1681}
1682
1683@misc{Translator2018-ki,
1684 language = {en},
1685 note = {Accessed: 2022-1-12},
1686 howpublished = {\url{https://www.microsoft.com/en-us/translator/blog/2018/03/14/human-parity-for-chinese-to-english-translations/}},
1687 year = {2018},
1688 month = {March},
1689 abstract = {Microsoft announced today that its researchers have developed
1690an AI machine translation system that can translate with the
1691same accuracy as a human from Chinese to English. To validate
1692the results, the researchers used an industry standard test
1693set of news stories (newstest2017) to compare human and
1694machine translation results. To further ensure accuracy of
1695the evaluation, the team also....},
1696 author = {Translator, Microsoft},
1697 booktitle = {Microsoft Translator Blog},
1698 title = {Neural Machine Translation reaches historic milestone: human
1699parity for Chinese to English translations},
1700}
1701
1702@article{mulligan2019thing,
1703 publisher = {ACM New York, NY, USA},
1704 year = {2019},
1705 pages = {1--36},
1706 number = {CSCW},
1707 volume = {3},
1708 journal = {Proceedings of the ACM on Human-Computer Interaction},
1709 author = {Mulligan, Deirdre K and Kroll, Joshua A and Kohli, Nitin and Wong, Richmond Y},
1710 title = {This thing called fairness: disciplinary confusion realizing a value in technology},
1711}
1712
1713@article{CambridgeAnalytica,
1714 url = {https://www.latimes.com/politics/la-na-pol-cambridge-analytica-20180321-story.html},
1715 journal = {Los Angeles Times},
1716 title = {Was Cambridge Analytica a digital Svengali or snake-oil salesman?},
1717 date = {2018-03-21},
1718 author = {Halper, Evan},
1719}
1720
1721@article{Toral2018-wn,
1722 eprint = {1808.10432},
1723 primaryclass = {cs.CL},
1724 archiveprefix = {arXiv},
1725 year = {2018},
1726 month = {August},
1727 abstract = {We reassess a recent study (Hassan et al., 2018) that
1728claimed that machine translation (MT) has reached human
1729parity for the translation of news from Chinese into
1730English, using pairwise ranking and considering three
1731variables that were not taken into account in that previous
1732study: the language in which the source side of the test set
1733was originally written, the translation proficiency of the
1734evaluators, and the provision of inter-sentential context.
1735If we consider only original source text (i.e. not
1736translated from another language, or translationese), then
1737we find evidence showing that human parity has not been
1738achieved. We compare the judgments of professional
1739translators against those of non-experts and discover that
1740those of the experts result in higher inter-annotator
1741agreement and better discrimination between human and
1742machine translations. In addition, we analyse the human
1743translations of the test set and identify important
1744translation issues. Finally, based on these findings, we
1745provide a set of recommendations for future human
1746evaluations of MT.},
1747 author = {Toral, Antonio and Castilho, Sheila and Hu, Ke and Way, Andy},
1748 title = {Attaining the Unattainable? Reassessing Claims of Human
1749Parity in Neural Machine Translation},
1750}
1751
1752@article{Laubli2018-sn,
1753 eprint = {1808.07048},
1754 primaryclass = {cs.CL},
1755 archiveprefix = {arXiv},
1756 year = {2018},
1757 month = {August},
1758 abstract = {Recent research suggests that neural machine translation
1759achieves parity with professional human translation on the
1760WMT Chinese--English news translation task. We empirically
1761test this claim with alternative evaluation protocols,
1762contrasting the evaluation of single sentences and entire
1763documents. In a pairwise ranking experiment, human raters
1764assessing adequacy and fluency show a stronger preference
1765for human over machine translation when evaluating documents
1766as compared to isolated sentences. Our findings emphasise
1767the need to shift towards document-level evaluation as
1768machine translation improves to the degree that errors which
1769are hard or impossible to spot at the sentence-level become
1770decisive in discriminating quality of different translation
1771outputs.},
1772 author = {L{\"a}ubli, Samuel and Sennrich, Rico and Volk, Martin},
1773 title = {Has Machine Translation Achieved Human Parity? A Case for
1774Document-level Evaluation},
1775}
1776
1777@article{Dobbe2019-ms,
1778 eprint = {1911.09005},
1779 primaryclass = {cs.AI},
1780 archiveprefix = {arXiv},
1781 year = {2019},
1782 month = {November},
1783 abstract = {As AI systems become prevalent in high stakes domains such
1784as surveillance and healthcare, researchers now examine how
1785to design and implement them in a safe manner. However, the
1786potential harms caused by systems to stakeholders in complex
1787social contexts and how to address these remains unclear. In
1788this paper, we explain the inherent normative uncertainty in
1789debates about the safety of AI systems. We then address this
1790as a problem of vagueness by examining its place in the
1791design, training, and deployment stages of AI system
1792development. We adopt Ruth Chang's theory of intuitive
1793comparability to illustrate the dilemmas that manifest at
1794each stage. We then discuss how stakeholders can navigate
1795these dilemmas by incorporating distinct forms of dissent
1796into the development pipeline, drawing on Elizabeth
1797Anderson's work on the epistemic powers of democratic
1798institutions. We outline a framework of sociotechnical
1799commitments to formal, substantive and discursive challenges
1800that address normative uncertainty across stakeholders, and
1801propose the cultivation of related virtues by those
1802responsible for development.},
1803 author = {Dobbe, Roel and Gilbert, Thomas Krendl and Mintz, Yonatan},
1804 title = {Hard Choices in Artificial Intelligence: Addressing
1805Normative Uncertainty through Sociotechnical Commitments},
1806}
1807
1808@misc{Buolamwini_undated-dd,
1809 note = {Accessed: 2022-1-12},
1810 howpublished = {\url{http://proceedings.mlr.press/v81/buolamwini18a/buolamwini18a.pdf}},
1811 abstract = {Recent studies demonstrate that machine learning algorithms
1812can discriminate based on classes like race and gender. In
1813this work, we present an approach to evaluate bias present in
1814automated facial analysis algorithms and datasets with
1815respect to phenotypic subgroups. Using the dermatologist
1816approved Fitzpatrick Skin Type classification system, we
1817characterize the gender and skin type distribution of two
1818facial analysis benchmarks, IJB-A and Adience. We find that
1819these datasets are overwhelmingly composed of lighter-skinned
1820subjects (79.6\% for IJB-A and 86.2\% for Adience) and
1821introduce a new facial analysis dataset which is balanced by
1822gender and skin type. We evaluate 3 commercial gender
1823classification systems using our dataset and show that
1824darker-skinned females are the most misclassified group (with
1825error rates of up to 34.7\%). The maximum error rate for
1826lighter-skinned males is 0.8\%. The substantial disparities
1827in the accuracy of classifying darker females, lighter
1828females, darker males, and lighter males in gender
1829classification systems require urgent attention if commercial
1830companies are to build genuinely fair, transparent and
1831accountable facial analysis algorithms.},
1832 author = {Buolamwini, Joy and Friedler, Sorelle A and Wilson, Christo},
1833 title = {Gender shades: Intersectional accuracy disparities in
1834commercial gender classification},
1835}
1836
1837@misc{Snow2018-vw,
1838 language = {en},
1839 note = {Accessed: 2022-1-12},
1840 howpublished = {\url{https://www.aclu.org/blog/privacy-technology/surveillance-technologies/amazons-face-recognition-falsely-matched-28}},
1841 year = {2018},
1842 month = {July},
1843 abstract = {Amazon's face surveillance technology is the target of
1844growing opposition nationwide, and today, there are 28 more
1845causes for concern. In a test the ACLU recently conducted of
1846the facial recognition tool, called ``Rekognition,'' the
1847software incorrectly matched 28 members of Congress,
1848identifying them as other people who have been arrested for a
1849crime. The members of Congress},
1850 author = {Snow, Jacob},
1851 booktitle = {American Civil Liberties Union},
1852 title = {Amazon's Face Recognition Falsely Matched 28 Members of
1853Congress With Mugshots},
1854}
1855
1856@misc{Wood_undated-ek,
1857 howpublished = {\url{https://aws.amazon.com/blogs/aws/thoughts-on-machine-learning-accuracy/}},
1858 author = {Wood, Matt},
1859 title = {Thoughts On Machine Learning Accuracy},
1860}
1861
1862@misc{aclu_response_response_fr,
1863 author = {ACLU},
1864 month = {July},
1865 year = {2018},
1866 language = {en},
1867 note = {Accessed: 2022-1-12},
1868 howpublished = {\url{https://www.aclu.org/press-releases/aclu-comment-new-amazon-statement-responding-face-recognition-technology-test}},
1869 abstract = {SAN FRANCISCO -- Amazon today issued an additional statement
1870in response to the American Civil Liberties Union Foundation
1871of Northern California test of Rekognition, the company's
1872face recognition technology. The test revealed that
1873Rekognition falsely matched 28 current members of Congress
1874with images in an arrest photo database.},
1875 booktitle = {American Civil Liberties Union},
1876 title = {{ACLU} Comment on New Amazon Statement Responding to Face
1877Recognition Technology Test},
1878}
1879
1880@misc{Ross2018-nn,
1881 language = {en},
1882 note = {Accessed: 2022-1-13},
1883 howpublished = {\url{https://www.statnews.com/2018/07/25/ibm-watson-recommended-unsafe-incorrect-treatments/?utm_source=STAT+Newsletters&utm_campaign=beb06f048d-MR_COPY_08&utm_medium=email&utm_term=0_8cab1d7961-beb06f048d-150085821}},
1884 year = {2018},
1885 month = {July},
1886 abstract = {Slide decks presented last summer by an IBM Watson Health
1887executive largely blame the problems on the training of
1888Watson for Oncology by IBM engineers and doctors at the
1889renowned Memorial Sloan Kettering Cancer Center.},
1890 author = {Ross, Casey and Swetlitz, Ike and Cohrs, Rachel and
1891Dillingham, Ian and {STAT Staff} and Florko, Nicholas and
1892Bender, Maddie},
1893 booktitle = {{STAT}},
1894 title = {{IBM's} Watson supercomputer recommended 'unsafe and
1895incorrect' cancer treatments, internal documents show},
1896}
1897
1898@article{md_anderson_benches_watson,
1899 language = {en},
1900 year = {2017},
1901 month = {February},
1902 journal = {Forbes Magazine},
1903 abstract = {MD Anderson has placed a much-ballyhooed 'Watson for cancer'
1904product it was developing with IBM on hold -- and is looking for
1905a new partner.},
1906 author = {Herper, Matthew},
1907 title = {{MD} Anderson Benches {IBM} Watson In Setback For Artificial
1908Intelligence In Medicine},
1909}
1910
1911@misc{Wojcik_undated-nb,
1912 note = {Accessed: 2022-1-13},
1913 howpublished = {\url{https://www.cnbc.com/2017/05/08/ibms-watson-is-a-joke-says-social-capital-ceo-palihapitiya.html}},
1914 author = {Wojcik, Natalia},
1915 booktitle = {{CNBC}},
1916 title = {{IBM's} Watson `is a joke,' says Social Capital {CEO}
1917Palihapitiya},
1918}
1919
1920@article{Simon2019-ed,
1921 language = {en},
1922 keywords = {Artificial intelligence application in medicine; Clinical
1923decision support; Closing the cancer care gap; Democratization of
1924evidence‐based care; Virtual expert advisor},
1925 year = {2019},
1926 month = {June},
1927 pages = {772--782},
1928 number = {6},
1929 volume = {24},
1930 journal = {Oncologist},
1931 abstract = {BACKGROUND: Rapid advances in science challenge the timely
1932adoption of evidence-based care in community settings. To bridge
1933the gap between what is possible and what is practiced, we
1934researched approaches to developing an artificial intelligence
1935(AI) application that can provide real-time patient-specific
1936decision support. MATERIALS AND METHODS: The Oncology Expert
1937Advisor (OEA) was designed to simulate peer-to-peer consultation
1938with three core functions: patient history summarization,
1939treatment options recommendation, and management advisory.
1940Machine-learning algorithms were trained to construct a dynamic
1941summary of patients cancer history and to suggest approved
1942therapy or investigative trial options. All patient data used
1943were retrospectively accrued. Ground truth was established for
1944approximately 1,000 unique patients. The full Medline database of
1945more than 23 million published abstracts was used as the
1946literature corpus. RESULTS: OEA's accuracies of searching
1947disparate sources within electronic medical records to extract
1948complex clinical concepts from unstructured text documents
1949varied, with F1 scores of 90\%-96\% for non-time-dependent
1950concepts (e.g., diagnosis) and F1 scores of 63\%-65\% for
1951time-dependent concepts (e.g., therapy history timeline). Based
1952on constructed patient profiles, OEA suggests approved therapy
1953options linked to supporting evidence (99.9\% recall; 88\%
1954precision), and screens for eligible clinical trials on
1955ClinicalTrials.gov (97.9\% recall; 96.9\% precision). CONCLUSION:
1956Our results demonstrated technical feasibility of an AI-powered
1957application to construct longitudinal patient profiles in context
1958and to suggest evidence-based treatment and trial options. Our
1959experience highlighted the necessity of collaboration across
1960clinical and AI domains, and the requirement of clinical
1961expertise throughout the process, from design to training to
1962testing. IMPLICATIONS FOR PRACTICE: Artificial intelligence
1963(AI)-powered digital advisors such as the Oncology Expert Advisor
1964have the potential to augment the capacity and update the
1965knowledge base of practicing oncologists. By constructing dynamic
1966patient profiles from disparate data sources and organizing and
1967vetting vast literature for relevance to a specific patient, such
1968AI applications could empower oncologists to consider all therapy
1969options based on the latest scientific evidence for their
1970patients, and help them spend less time on information ``hunting
1971and gathering'' and more time with the patients. However,
1972realization of this will require not only AI technology
1973maturation but also active participation and leadership by
1974clincial experts.},
1975 author = {Simon, George and DiNardo, Courtney D and Takahashi, Koichi and
1976Cascone, Tina and Powers, Cynthia and Stevens, Rick and Allen,
1977Joshua and Antonoff, Mara B and Gomez, Daniel and Keane, Pat and
1978Suarez Saiz, Fernando and Nguyen, Quynh and Roarty, Emily and
1979Pierce, Sherry and Zhang, Jianjun and Hardeman Barnhill, Emily
1980and Lakhani, Kate and Shaw, Kenna and Smith, Brett and Swisher,
1981Stephen and High, Rob and Futreal, P Andrew and Heymach, John and
1982Chin, Lynda},
1983 title = {Applying Artificial Intelligence to Address the Knowledge Gaps in
1984Cancer Care},
1985}
1986
1987@misc{Strickland_undated-ng,
1988 note = {Accessed: 2022-1-13},
1989 howpublished = {\url{https://spectrum.ieee.org/how-ibm-watson-overpromised-and-underdelivered-on-ai-health-care}},
1990 abstract = {After its triumph on Jeopardy!, IBM's AI seemed poised to
1991revolutionize medicine. Doctors are still waiting},
1992 author = {Strickland, Eliza},
1993 title = {{IBM} Watson Heal Thyself: How {IBM} Watson Overpromised And
1994Underdeliverd On {AI} Health Care},
1995}
1996
1997@article{Gianfrancesco2018-vl,
1998 language = {en},
1999 year = {2018},
2000 month = {November},
2001 pages = {1544--1547},
2002 number = {11},
2003 volume = {178},
2004 journal = {JAMA Intern. Med.},
2005 abstract = {A promise of machine learning in health care is the avoidance of
2006biases in diagnosis and treatment; a computer algorithm could
2007objectively synthesize and interpret the data in the medical
2008record. Integration of machine learning with clinical decision
2009support tools, such as computerized alerts or diagnostic support,
2010may offer physicians and others who provide health care targeted
2011and timely information that can improve clinical decisions.
2012Machine learning algorithms, however, may also be subject to
2013biases. The biases include those related to missing data and
2014patients not identified by algorithms, sample size and
2015underestimation, and misclassification and measurement error.
2016There is concern that biases and deficiencies in the data used by
2017machine learning algorithms may contribute to socioeconomic
2018disparities in health care. This Special Communication outlines
2019the potential biases that may be introduced into machine
2020learning-based clinical decision support tools that use
2021electronic health record data and proposes potential solutions to
2022the problems of overreliance on automation, algorithms based on
2023biased data, and algorithms that do not provide information that
2024is clinically meaningful. Existing health care disparities should
2025not be amplified by thoughtless or excessive reliance on
2026machines.},
2027 author = {Gianfrancesco, Milena A and Tamang, Suzanne and Yazdany, Jinoos
2028and Schmajuk, Gabriela},
2029 title = {Potential Biases in Machine Learning Algorithms Using Electronic
2030Health Record Data},
2031}
2032
2033@inproceedings{Jacobs2021-rk,
2034 location = {Virtual Event Canada},
2035 conference = {FAccT '21: 2021 ACM Conference on Fairness, Accountability, and
2036Transparency},
2037 address = {New York, NY, USA},
2038 year = {2021},
2039 month = {March},
2040 publisher = {ACM},
2041 author = {Jacobs, Abigail Z and Wallach, Hanna},
2042 booktitle = {Proceedings of the 2021 {ACM} Conference on Fairness,
2043Accountability, and Transparency},
2044 title = {Measurement and Fairness},
2045}
2046
2047@article{Alexandrova_undated-mx,
2048 journal = {Eur. J. Philos. Sci.},
2049 author = {Alexandrova, Anna and Fabian, Mark},
2050 title = {Democratising Measurement: Or Why Thick Concepts Call for
2051Coproduction},
2052}
2053
2054@article{Jacobs2021-og,
2055 eprint = {2109.05658},
2056 primaryclass = {cs.CY},
2057 archiveprefix = {arXiv},
2058 year = {2021},
2059 month = {September},
2060 abstract = {Measurement of social phenomena is everywhere, unavoidably,
2061in sociotechnical systems. This is not (only) an academic
2062point: Fairness-related harms emerge when there is a
2063mismatch in the measurement process between the thing we
2064purport to be measuring and the thing we actually measure.
2065However, the measurement process -- where social, cultural,
2066and political values are implicitly encoded in
2067sociotechnical systems -- is almost always obscured.
2068Furthermore, this obscured process is where important
2069governance decisions are encoded: governance about which
2070systems are fair, which individuals belong in which
2071categories, and so on. We can then use the language of
2072measurement, and the tools of construct validity and
2073reliability, to uncover hidden governance decisions. In
2074particular, we highlight two types of construct validity,
2075content validity and consequential validity, that are useful
2076to elicit and characterize the feedback loops between the
2077measurement, social construction, and enforcement of social
2078categories. We then explore the constructs of fairness,
2079robustness, and responsibility in the context of governance
2080in and for responsible AI. Together, these perspectives help
2081us unpack how measurement acts as a hidden governance
2082process in sociotechnical systems. Understanding measurement
2083as governance supports a richer understanding of the
2084governance processes already happening in AI -- responsible
2085or otherwise -- revealing paths to more effective
2086interventions.},
2087 author = {Jacobs, Abigail Z},
2088 title = {Measurement as governance in and for responsible {AI}},
2089}
2090
2091@misc{Mayson_dangdefendants,
2092 note = {Accessed: 2022-1-15},
2093 howpublished = {\url{https://www.yalelawjournal.org/article/dangerous-defendants}},
2094 abstract = {Bail reformers aspire to untether pretrial detention from
2095wealth and condition it instead on the risk that a defendant
2096will commit crime if released. In setting this risk
2097threshold, this Article argues that there is no clear
2098constitutional, moral, or practical basis for distinguishing
2099between equally dangerous defendants and non-defendants.},
2100 author = {Mayson, Sandra G},
2101 title = {Dangerous Defendants},
2102}
2103
2104@article{Lum2016-hz,
2105 language = {en},
2106 year = {2016},
2107 month = {October},
2108 pages = {14--19},
2109 number = {5},
2110 volume = {13},
2111 publisher = {Wiley},
2112 journal = {Signif. (Oxf.)},
2113 abstract = {Predictive policing systems are used increasingly by law
2114enforcement to try to prevent crime before it occurs. But what
2115happens when these systems are trained using biased data?
2116Kristian Lum and William Isaac consider the evidence ? and the
2117social consequences},
2118 author = {Lum, Kristian and Isaac, William},
2119 title = {To predict and serve?},
2120}
2121
2122@article{Ferguson2016-bs,
2123 year = {2016},
2124 publisher = {HeinOnline},
2125 journal = {Wash. UL Rev.},
2126 abstract = {… This article examines predictive policing's evolution with the
2127goal ofproviding the first practical and theoretical critique of
2128this new policing … assessment throughout the criminal justice
2129system, this article provides an analytical framework to police
2130new predictive technologies. …},
2131 author = {Ferguson, A G},
2132 title = {Policing predictive policing},
2133}
2134
2135@article{Hoffman2013-ms,
2136 language = {en},
2137 year = {2013},
2138 pages = {497--538},
2139 number = {4},
2140 volume = {39},
2141 journal = {Am. J. Law Med.},
2142 abstract = {Very large biomedical research databases, containing electronic
2143health records (EHR) and genomic data from millions of patients,
2144have been heralded recently for their potential to accelerate
2145scientific discovery and produce dramatic improvements in medical
2146treatments. Research enabled by these databases may also lead to
2147profound changes in law, regulation, social policy, and even
2148litigation strategies. Yet, is ``big data'' necessarily better
2149data? This paper makes an original contribution to the legal
2150literature by focusing on what can go wrong in the process of
2151biomedical database research and what precautions are necessary
2152to avoid critical mistakes. We address three main reasons for
2153approaching such research with care and being cautious in relying
2154on its outcomes for purposes of public policy or litigation.
2155First, the data contained in biomedical databases is surprisingly
2156likely to be incorrect or incomplete. Second, systematic biases,
2157arising from both the nature of the data and the preconceptions
2158of investigators, are serious threats to the validity of research
2159results, especially in answering causal questions. Third, data
2160mining of biomedical databases makes it easier for individuals
2161with political, social, or economic agendas to generate
2162ostensibly scientific but misleading research findings for the
2163purpose of manipulating public opinion and swaying policymakers.
2164In short, this paper sheds much-needed light on the problems of
2165credulous and uninformed acceptance of research results derived
2166from biomedical databases. An understanding of the pitfalls of
2167big data analysis is of critical importance to anyone who will
2168rely on or dispute its outcomes, including lawyers, policymakers,
2169and the public at large. The Article also recommends technical,
2170methodological, and educational interventions to combat the
2171dangers of database errors and abuses.},
2172 author = {Hoffman, Sharona and Podgurski, Andy},
2173 title = {The use and misuse of biomedical data: is bigger really better?},
2174}
2175
2176@article{Hoffman2013-oa,
2177 language = {en},
2178 year = {2013},
2179 month = {March},
2180 pages = {56--60},
2181 volume = {41 Suppl 1},
2182 journal = {J. Law Med. Ethics},
2183 abstract = {The accelerating adoption of electronic health record (EHR)
2184systems will have far-reaching implications for public health
2185research and surveillance, which in turn could lead to changes in
2186public policy, statutes, and regulations. The public health
2187benefits of EHR use can be significant. However, researchers and
2188analysts who rely on EHR data must proceed with caution and
2189understand the potential limitations of EHRs. Because of
2190clinicians' workloads, poor user-interface design, and other
2191factors, EHR data can be erroneous, miscoded, fragmented, and
2192incomplete. In addition, public health findings can be tainted by
2193the problems of selection bias, confounding bias, and measurement
2194bias. These flaws may become all the more troubling and important
2195in an era of electronic ``big data,'' in which a massive amount
2196of information is processed automatically, without human checks.
2197Thus, we conclude the paper by outlining several regulatory and
2198other interventions to address data analysis difficulties that
2199could result in invalid conclusions and unsound public health
2200policies.},
2201 author = {Hoffman, Sharona and Podgurski, Andy},
2202 title = {Big bad data: law, public health, and biomedical databases},
2203}
2204
2205@article{Agrawal2020-rs,
2206 language = {en},
2207 year = {2020},
2208 month = {April},
2209 pages = {525--534},
2210 number = {4},
2211 volume = {124},
2212 journal = {Heredity},
2213 abstract = {Big Data will be an integral part of the next generation of
2214technological developments-allowing us to gain new insights from
2215the vast quantities of data being produced by modern life. There
2216is significant potential for the application of Big Data to
2217healthcare, but there are still some impediments to overcome,
2218such as fragmentation, high costs, and questions around data
2219ownership. Envisioning a future role for Big Data within the
2220digital healthcare context means balancing the benefits of
2221improving patient outcomes with the potential pitfalls of
2222increasing physician burnout due to poor implementation leading
2223to added complexity. Oncology, the field where Big Data
2224collection and utilization got a heard start with programs like
2225TCGA and the Cancer Moon Shot, provides an instructive example as
2226we see different perspectives provided by the United States (US),
2227the United Kingdom (UK) and other nations in the implementation
2228of Big Data in patient care with regards to their centralization
2229and regulatory approach to data. By drawing upon global
2230approaches, we propose recommendations for guidelines and
2231regulations of data use in healthcare centering on the creation
2232of a unique global patient ID that can integrate data from a
2233variety of healthcare providers. In addition, we expand upon the
2234topic by discussing potential pitfalls to Big Data such as the
2235lack of diversity in Big Data research, and the security and
2236transparency risks posed by machine learning algorithms.},
2237 author = {Agrawal, Raag and Prabakaran, Sudhakaran},
2238 title = {Big data in digital healthcare: lessons learnt and
2239recommendations for general practice},
2240}
2241
2242@article{Ensign2017-vi,
2243 eprint = {1706.09847},
2244 primaryclass = {cs.CY},
2245 archiveprefix = {arXiv},
2246 year = {2017},
2247 month = {June},
2248 abstract = {Predictive policing systems are increasingly used to
2249determine how to allocate police across a city in order to
2250best prevent crime. Discovered crime data (e.g., arrest
2251counts) are used to help update the model, and the process
2252is repeated. Such systems have been empirically shown to be
2253susceptible to runaway feedback loops, where police are
2254repeatedly sent back to the same neighborhoods regardless of
2255the true crime rate. In response, we develop a mathematical
2256model of predictive policing that proves why this feedback
2257loop occurs, show empirically that this model exhibits such
2258problems, and demonstrate how to change the inputs to a
2259predictive policing system (in a black-box manner) so the
2260runaway feedback loop does not occur, allowing the true
2261crime rate to be learned. Our results are quantitative: we
2262can establish a link (in our model) between the degree to
2263which runaway feedback causes problems and the disparity in
2264crime rates between areas. Moreover, we can also demonstrate
2265the way in which \textbackslashemph\{reported\} incidents of
2266crime (those reported by residents) and
2267\textbackslashemph\{discovered\} incidents of crime (i.e.
2268those directly observed by police officers dispatched as a
2269result of the predictive policing algorithm) interact: in
2270brief, while reported incidents can attenuate the degree of
2271runaway feedback, they cannot entirely remove it without the
2272interventions we suggest.},
2273 author = {Ensign, Danielle and Friedler, Sorelle A and Neville, Scott
2274and Scheidegger, Carlos and Venkatasubramanian, Suresh},
2275 title = {Runaway Feedback Loops in Predictive Policing},
2276}
2277
2278@unpublished{Richardson2019-cn,
2279 keywords = {Policing, Predictive Policing, Civil Rights, Bias, Justice, Data,
2280AI, Machine Learning},
2281 year = {2019},
2282 month = {February},
2283 abstract = {Law enforcement agencies are increasingly using predictive
2284policing systems to forecast criminal activity and allocate
2285police resources. Yet in numerous jurisdictions, these systems
2286are built on data produced during documented periods of flawed,
2287racially biased, and sometimes unlawful practices and policies
2288(``dirty policing''). These policing practices and policies shape
2289the environment and the methodology by which data is created,
2290which raises the risk of creating inaccurate, skewed, or
2291systemically biased data (``dirty data''). If predictive policing
2292systems are informed by such data, they cannot escape the
2293legacies of the unlawful or biased policing practices that they
2294are built on. Nor do current claims by predictive policing
2295vendors provide sufficient assurances that their systems
2296adequately mitigate or segregate this data.In our research, we
2297analyze thirteen jurisdictions that have used or developed
2298predictive policing tools while under government commission
2299investigations or federal court monitored settlements, consent
2300decrees, or memoranda of agreement stemming from corrupt,
2301racially biased, or otherwise illegal policing practices. In
2302particular, we examine the link between unlawful and biased
2303police practices and the data available to train or implement
2304these systems. We highlight three case studies: (1) Chicago, an
2305example of where dirty data was ingested directly into the city's
2306predictive system; (2) New Orleans, an example where the
2307extensive evidence of dirty policing practices and recent
2308litigation suggests an extremely high risk that dirty data was or
2309could be used in predictive policing; and (3) Maricopa County,
2310where despite extensive evidence of dirty policing practices, a
2311lack of public transparency about the details of various
2312predictive policing systems restricts a proper assessment of the
2313risks. The implications of these findings have widespread
2314ramifications for predictive policing writ large. Deploying
2315predictive policing systems in jurisdictions with extensive
2316histories of unlawful police practices presents elevated risks
2317that dirty data will lead to flawed or unlawful predictions,
2318which in turn risk perpetuating additional harm via feedback
2319loops throughout the criminal justice system. The use of
2320predictive policing must be treated with high levels of caution
2321and mechanisms for the public to know, assess, and reject such
2322systems are imperative.},
2323 author = {Richardson, Rashida and Schultz, Jason and Crawford, Kate},
2324 title = {Dirty Data, Bad Predictions: How Civil Rights Violations Impact
2325Police Data, Predictive Policing Systems, and Justice},
2326}
2327
2328@unpublished{Stevenson2021-fr,
2329 keywords = {pretrial detention, consequentialism, risk assessments, bail
2330reform},
2331 year = {2021},
2332 month = {February},
2333 abstract = {How dangerous must a person be to justify the state in locking
2334her up for the greater good? The bail reform movement, which
2335aspires to limit pretrial detention to the truly dangerous---and
2336which has looked to algorithmic risk assessments to quantify
2337danger---has brought this question to the fore. Constitutional
2338doctrine authorizes pretrial detention when the government's
2339interest in safety ``outweighs'' an individual's interest in
2340liberty, but it does not specify how to balance these goods. If
2341detaining ten presumptively innocent people for three months is
2342projected to prevent one robbery, is it worth it?This Article
2343confronts the question of what degree of risk justifies pretrial
2344preventive detention if one takes the consequentialist approach
2345of current law seriously. Surveying the law, we derive two
2346principles: 1) detention must avert greater harm (by preventing
2347crime) than it inflicts (by depriving a person of liberty) and 2)
2348prohibitions against pretrial punishment mean that the harm
2349experienced by the detainee cannot be discounted in the
2350cost-benefit calculus. With this conceptual framework in place,
2351we develop a novel empirical method for estimating the relative
2352harms of incarceration and crime victimization that we call
2353``Rawlsian cost-benefit analysis'': a survey method that asks
2354respondents to choose between being the victim of certain crimes
2355or being jailed for varying time periods. The results suggest
2356that even short periods of incarceration impose grave harms, such
2357that a person must pose an extremely high risk of serious crime
2358in order for detention to be justified. No existing risk
2359assessment tool is sufficient to identify individuals who warrant
2360detention. The empirical results demonstrate that the stated
2361consequentialist rationale for pretrial detention cannot begin to
2362justify our current detention rates, and suggest that the
2363existing system veers uncomfortably close to pretrial punishment.
2364The degree of discord between theory and practice demands a
2365rethinking of pretrial law and policy.},
2366 author = {Stevenson, Megan T and Mayson, Sandra G},
2367 title = {Pretrial detention and the value of liberty},
2368}
2369
2370@misc{Gouldin_undated-oc,
2371 note = {Accessed: 2022-1-14},
2372 howpublished = {\url{https://lawreview.uchicago.edu/sites/lawreview.uchicago.edu/files/02\%20Gouldin_ART_SA\%20\%28JPM\%29.pdf}},
2373 abstract = {Our illogical and too-well-traveled paths to pretrial
2374detention have created staggering costs for defendants who
2375spend unnecessary time in pretrial detention and for
2376taxpayers who fund a broken system. These problems remain
2377recalcitrant even as a third generation of reform efforts
2378makes impressive headway. They are likely to remain so until
2379judges, attorneys, legislators, and scholars address a
2380fundamental definitional problem: the collapsing of very
2381different types of behavior that result in failures to appear
2382in court into a single, undifferentiated category of
2383nonappearance risk. That single category muddies critical
2384distinctions that this Article's new taxonomy of pretrial
2385nonappearance risks clarifies. This taxonomy (i) isolates
2386true flight risk (the risk that a defendant will flee the
2387jurisdiction) from other forms of ``local'' nonappearance
2388risk and (ii) distinguishes between local nonappearance risks
2389based on persistence, willfulness, amenability to
2390intervention, and cost. Upon examination, it is clear that
2391flight and nonappearance are not simply interchangeable names
2392for the same concept, nor are they merely different degrees
2393of the same type of risk. In the context of measuring and
2394managing risks, many defendants who merely fail to appear
2395differ in important ways from their fugitive cousins.
2396Precision about these distinctions is constitutionally
2397mandated and statutorily required. It is also essential for
2398current reform efforts that are aimed at identifying less
2399intrusive and lower-cost interventions that can effectively
2400manage the full range of nonappearance and flight risks.
2401These distinctions are not reflected in the pretrial
2402risk-assessment tools that are increasingly being employed
2403across the country. But they should be. A more nuanced
2404understanding of these differences},
2405 author = {Gouldin, Lauryn P and Appleman, Laura and Baughman, Shima
2406Baradaran and Berger, Todd and Bybee, Keith and Cahill,
2407Michael and Commandeur, Nicolas and Eaglin, Jessica and
2408Futrell, Nicole Smith and Godsoe, Cynthia and Gold, Russell
2409and Kohn, Nina and Lain, Corinna and Levine, Kate and Mayson,
2410Sandy and Moore, Janet and Ouziel, Lauren and Podgor, Ellen
2411and Roberts, Anna and Sacharoff, Laurent and Schnacke, Tim
2412and Simonson, Jocelyn and True-Frost, Cora},
2413 title = {Defining flight risk},
2414}
2415
2416@article{Slobogin2003-ou,
2417 language = {en},
2418 year = {2003},
2419 publisher = {Elsevier BV},
2420 journal = {SSRN Electron. J.},
2421 abstract = {This article addresses the state's police power authority to
2422deprive people of liberty based on predictions of antisocial
2423behavior. Most conspicuously exercised against so-called
2424``sexual predators,'' this authority purportedly justifies a
2425wide array of other state interventions as well, ranging from
2426police stops to executions. Yet there still is no general theory
2427of preventive detention. This article is a preliminary effort in
2428that regard. The article first surveys the various objections to
2429preventive detention: the unreliability objection; the
2430punishment-in-disguise objection; the legality objection; and
2431the dehumanization objection. None of these objections justifies
2432a complete prohibition on the state's power to detain people
2433based on dangerousness. But they do suggest significant
2434limitations on that power regarding acceptable methods of
2435prediction, the nature and duration of preventive detention, the
2436threshold conduct that can trigger such detention, and the
2437extent to which it can replace punishment as the official
2438response to antisocial behavior. On the latter issue, the
2439central conclusion is that preventive detention which functions
2440as a substitute for punishment, as in the case of sexual
2441predator statutes, is only permissible if certain psychological
2442and predictive criteria are met. The rest of the paper develops
2443these criteria. It argues that the psychological criterion
2444should be undeterrability, defined as the characteristic
2445ignorance that one's criminal activity is criminal or a
2446characteristic willingness to commit crime despite certain and
2447significant punishment, a definition that differs from both the
2448usual academic stance and the Supreme Court's
2449inability-to-control formulation. The paper next argues that
2450selection of a prediction criterion should be informed by two
2451principles, the proportionality principle (which varies the
2452legally requisite level of dangerousness with the nature and
2453duration of the state's intervention) and the consistency
2454principle (which takes as a reference point the implicit
2455dangerousness assessments in the law of crimes). Finally, the
2456paper explores some of the implications of the latter principle
2457for the criminal law, including the possibility that some crimes
2458- in particular various possession offenses, reckless
2459endangerment and vagrancy - violate the fundamental norms of the
2460police power authority.},
2461 author = {Slobogin, Christopher},
2462 title = {A jurisprudence of dangerousness},
2463}
2464
2465@inproceedings{Akpinar2021-fb,
2466 location = {Virtual Event, Canada},
2467 address = {New York, NY, USA},
2468 year = {2021},
2469 month = {March},
2470 series = {FAccT '21},
2471 pages = {838--849},
2472 publisher = {Association for Computing Machinery},
2473 abstract = {Police departments around the world have been experimenting with
2474forms of place-based data-driven proactive policing for over two
2475decades. Modern incarnations of such systems are commonly known
2476as hot spot predictive policing. These systems predict where
2477future crime is likely to concentrate such that police can
2478allocate patrols to these areas and deter crime before it
2479occurs. Previous research on fairness in predictive policing has
2480concentrated on the feedback loops which occur when models are
2481trained on discovered crime data, but has limited implications
2482for models trained on victim crime reporting data. We
2483demonstrate how differential victim crime reporting rates across
2484geographical areas can lead to outcome disparities in common
2485crime hot spot prediction models. Our analysis is based on a
2486simulation1 patterned after district-level victimization and
2487crime reporting survey data for Bogot{\'a}, Colombia. Our
2488results suggest that differential crime reporting rates can lead
2489to a displacement of predicted hotspots from high crime but low
2490reporting areas to high or medium crime and high reporting
2491areas. This may lead to misallocations both in the form of
2492over-policing and under-policing.},
2493 author = {Akpinar, Nil-Jana and De-Arteaga, Maria and Chouldechova,
2494Alexandra},
2495 booktitle = {Proceedings of the 2021 {ACM} Conference on Fairness,
2496Accountability, and Transparency},
2497 title = {The effect of differential victim crime reporting on predictive
2498policing systems},
2499}
2500
2501@article{vinsel_critihype,
2502 url = {https://sts-news.medium.com/youre-doing-it-wrong-notes-on-criticism-and-technology-hype-18b08b4307e5},
2503 author = {Vinsel, Lee},
2504 title = {You’re Doing It Wrong: Notes on Criticism and Technology Hype},
2505}
2506
2507@inbook{krafft_et_al,
2508 numpages = {7},
2509 pages = {72–78},
2510 booktitle = {Proceedings of the AAAI/ACM Conference on AI, Ethics, and Society},
2511 url = {https://doi.org/10.1145/3375627.3375835},
2512 address = {New York, NY, USA},
2513 publisher = {Association for Computing Machinery},
2514 isbn = {9781450371100},
2515 year = {2020},
2516 title = {Defining AI in Policy versus Practice},
2517 author = {Krafft, P. M. and Young, Meg and Katell, Michael and Huang, Karen and Bugingo, Ghislain},
2518}
2519
2520@inproceedings{hidden_technical_debt,
2521 year = {2015},
2522 volume = {28},
2523 url = {https://proceedings.neurips.cc/paper/2015/file/86df7dcfd896fcaf2674f757a2463eba-Paper.pdf},
2524 title = {Hidden Technical Debt in Machine Learning Systems},
2525 publisher = {Curran Associates, Inc.},
2526 pages = {},
2527 editor = {C. Cortes and N. Lawrence and D. Lee and M. Sugiyama and R. Garnett},
2528 booktitle = {Advances in Neural Information Processing Systems},
2529 author = {Sculley, D. and Holt, Gary and Golovin, Daniel and Davydov, Eugene and Phillips, Todd and Ebner, Dietmar and Chaudhary, Vinay and Young, Michael and Crespo, Jean-Fran\c{c}ois and Dennison, Dan},
2530}
2531
2532@article{stark_and_hutson,
2533 url = {https://papers.ssrn.com/sol3/papers.cfm?abstract_id=3927300},
2534 year = {2022},
2535 journal = {forthcoming in Fordham Intellectual Property, Media \& Entertainment Law Journal XXXII},
2536 author = {Stark, Luke and Hutson, Jevan},
2537 title = {Physiognomic Artificial Intelligence},
2538}
2539
2540@article{wired_criminality,
2541 url = {https://www.wired.com/story/algorithm-predicts-criminality-based-face-sparks-furor/},
2542 journal = {Wired},
2543 title = {An Algorithm That ‘Predicts’ Criminality Based on a Face Sparks a Furor},
2544 date = {2020-06-24},
2545 author = {Fussell, Sidney},
2546}
2547
2548@article{ai_vs_clinicians,
2549 journal = {BMJ},
2550 eprint = {https://www.bmj.com/content/368/bmj.m689.full.pdf},
2551 url = {https://www.bmj.com/content/368/bmj.m689},
2552 publisher = {BMJ Publishing Group Ltd},
2553 doi = {10.1136/bmj.m689},
2554 year = {2020},
2555 elocation-id = {m689},
2556 volume = {368},
2557 title = {Artificial intelligence versus clinicians: systematic review of design, reporting standards, and claims of deep learning studies},
2558 author = {Nagendran, Myura and Chen, Yang and Lovejoy, Christopher A and Gordon, Anthony C and Komorowski, Matthieu and Harvey, Hugh and Topol, Eric J and Ioannidis, John P A and Collins, Gary S and Maruthappu, Mahiben},
2559}
2560
2561@article{sepsis_validation,
2562 eprint = {https://jamanetwork.com/journals/jamainternalmedicine/articlepdf/2781307/jamainternal\_wong\_2021\_oi\_210027\_1627674961.11707.pdf},
2563 url = {https://doi.org/10.1001/jamainternmed.2021.2626},
2564 doi = {10.1001/jamainternmed.2021.2626},
2565 issn = {2168-6106},
2566 month = {08},
2567 year = {2021},
2568 pages = {1065-1070},
2569 number = {8},
2570 volume = {181},
2571 journal = {JAMA Internal Medicine},
2572 title = {{External Validation of a Widely Implemented Proprietary Sepsis Prediction Model in Hospitalized Patients}},
2573 author = {Wong, Andrew and Otles, Erkin and Donnelly, John P. and Krumm, Andrew and McCullough, Jeffrey and DeTroyer-Cooley, Olivia and Pestrue, Justin and Phillips, Marie and Konye, Judy and Penoza, Carleen and Ghous, Muhammad and Singh, Karandeep},
2574}
2575
2576@article{aclu_idaho,
2577 url = {https://www.aclu.org/blog/privacy-technology/pitfalls-artificial-intelligence-decisionmaking-highlighted-idaho-aclu-case},
2578 journal = {ACLU Blogs},
2579 title = {Pitfalls of Artificial Intelligence Decisionmaking Highlighted In Idaho ACLU Case},
2580 date = {2017-06-02},
2581 author = {Stanley, Jay},
2582}
2583
2584@article{verge_aclu_idaho,
2585 url = {https://www.theverge.com/2018/3/21/17144260/healthcare-medicaid-algorithm-arkansas-cerebral-palsy},
2586 journal = {The Verge},
2587 title = {What Happens When an Algorithm Cuts Your Health Care},
2588 date = {2018-03-21},
2589 author = {Lecher, Colin},
2590}
2591
2592@misc{democratizing_h20,
2593 howpublished = {\url{https://www.h2o.ai/democratizing-ai/}},
2594 year = {2022},
2595 title = {H2O.ai is Democratizing Artificial Intelligence},
2596}
2597
2598@article{democratizing_deloitte,
2599 journal = {Deloitte Insights - Signals for Strategists},
2600 url = {https://www2.deloitte.com/content/dam/insights/us/articles/4602_Democratizing-data-science/DI_Democratizing-data-science.pdf},
2601 author = {Schatsky, David and Chauhan, Rameeta and Muraskin, Craig},
2602 year = {2018},
2603 title = {Democratizing data science to bridge the talent gap},
2604}
2605
2606@article{de_democratizing,
2607 bibsource = {dblp computer science bibliography, https://dblp.org},
2608 biburl = {https://dblp.org/rec/journals/corr/abs-2010-15581.bib},
2609 timestamp = {Tue, 03 Nov 2020 11:44:23 +0100},
2610 eprint = {2010.15581},
2611 eprinttype = {arXiv},
2612 url = {https://arxiv.org/abs/2010.15581},
2613 year = {2020},
2614 volume = {abs/2010.15581},
2615 journal = {CoRR},
2616 title = {The De-democratization of {AI:} Deep Learning and the Compute Divide
2617in Artificial Intelligence Research},
2618 author = {Nur Ahmed and
2619Muntasir Wahed},
2620}
2621
2622@article{wired_paperclips,
2623 url = {https://www.wired.com/story/the-way-the-world-ends-not-with-a-bang-but-a-paperclip/},
2624 journal = {Wired},
2625 title = {The Way the World Ends: Not with a Bang But a Paperclip},
2626 date = {2017-10-21},
2627 author = {Rogers, Adam},
2628}
2629
2630@misc{coalition,
2631 howpublished = {\url{https://medium.com/@CoalitionForCriticalTechnology/abolish-the-techtoprisonpipeline-9b5b14366b16}},
2632 title = {Abolish the \#TechToPrisonPipeline},
2633 date = {2020-06-22},
2634 author = {Coalition for Critical Technology},
2635}
2636
2637@misc{google_nest_help,
2638 howpublished = {\url{https://support.google.com/googlenest/answer/6294727?hl=en}},
2639 title = {Wave control - Google Nest Help},
2640}
2641
2642@inproceedings{measuring_robustness_to_natural_distribution_shifts,
2643 year = {2020},
2644 volume = {33},
2645 url = {https://proceedings.neurips.cc/paper/2020/file/d8330f857a17c53d217014ee776bfd50-Paper.pdf},
2646 title = {Measuring Robustness to Natural Distribution Shifts in Image Classification},
2647 publisher = {Curran Associates, Inc.},
2648 pages = {18583--18599},
2649 editor = {H. Larochelle and M. Ranzato and R. Hadsell and M. F. Balcan and H. Lin},
2650 booktitle = {Advances in Neural Information Processing Systems},
2651 author = {Taori, Rohan and Dave, Achal and Shankar, Vaishaal and Carlini, Nicholas and Recht, Benjamin and Schmidt, Ludwig},
2652}
2653
2654@misc{arvind-reproducibility,
2655 urldate = {2021-07-28},
2656 url = {https://reproducible.cs.princeton.edu/},
2657 howpublished = {\url{https://reproducible.cs.princeton.edu/}},
2658 year = {2021},
2659 pages = {6},
2660 author = {Kapoor, Sayash and Narayanan, Arvind},
2661 language = {en},
2662 title = {({Ir}){Reproducible} {Machine} {Learning}: {A} {Case} {Study}},
2663}
2664
2665@inproceedings{fairness_tradeoffs_neurips,
2666 year = {2019},
2667 volume = {32},
2668 url = {https://proceedings.neurips.cc/paper/2019/file/373e4c5d8edfa8b74fd4b6791d0cf6dc-Paper.pdf},
2669 title = {Unlocking Fairness: a Trade-off Revisited},
2670 publisher = {Curran Associates, Inc.},
2671 pages = {},
2672 editor = {H. Wallach and H. Larochelle and A. Beygelzimer and F. d\textquotesingle Alch\'{e}-Buc and E. Fox and R. Garnett},
2673 booktitle = {Advances in Neural Information Processing Systems},
2674 author = {Wick, Michael and panda, swetasudha and Tristan, Jean-Baptiste},
2675}
2676
2677@article{nest,
2678 month = {Apr},
2679 year = {2014},
2680 author = {Wingfield, Nick},
2681 journal = {The New York Times},
2682 url = {https://www.nytimes.com/2014/04/04/technology/nest-labs-citing-flaw-halts-smoke-detector-sales.html},
2683 title = {Nest Labs Stops Selling Its Smoke Detector},
2684}
2685
2686@misc{catherine_olsson,
2687 url = {https://medium.com/@catherio/unsolved-research-problems-vs-real-world-threat-models-e270e256bc9e},
2688 howpublished = {\url{https://medium.com/@catherio/unsolved-research-problems-vs-real-world-threat-models-e270e256bc9e}},
2689 year = {2019},
2690 author = {Olsson, Catherine},
2691 language = {en},
2692 title = {Unsolved research problems vs. real-world threat models},
2693}
2694
2695@article{3d_printed_masks,
2696 url = {https://www.theverge.com/2019/12/13/21020575/china-facial-recognition-terminals-fooled-3d-mask-kneron-research-fallibility},
2697 journal = {The Verge},
2698 title = {Researchers fooled Chinese facial recognition terminals with just a mask},
2699 date = {2019-12-13},
2700 author = {Peters, Jay},
2701}
2702
2703@article{makeup,
2704 bibsource = {dblp computer science bibliography, https://dblp.org},
2705 biburl = {https://dblp.org/rec/journals/corr/abs-2109-06467.bib},
2706 timestamp = {Tue, 21 Sep 2021 17:46:04 +0200},
2707 eprint = {2109.06467},
2708 eprinttype = {arXiv},
2709 url = {https://arxiv.org/abs/2109.06467},
2710 year = {2021},
2711 volume = {abs/2109.06467},
2712 journal = {CoRR},
2713 title = {Dodging Attack Using Carefully Crafted Natural Makeup},
2714 author = {Nitzan Guetta and
2715Asaf Shabtai and
2716Inderjeet Singh and
2717Satoru Momiyama and
2718Yuval Elovici},
2719}
2720
2721@article{vegas_pd,
2722 url = {https://www.vice.com/en/article/pkyxwv/las-vegas-cops-used-unsuitable-facial-recognition-photos-to-make-arrests},
2723 journal = {Vice},
2724 title = {Las Vegas Cops Used ‘Unsuitable’ Facial Recognition Photos To Make Arrests},
2725 date = {2020-08-07},
2726 author = {Feathers, Todd},
2727}
2728
2729@inproceedings{LiaoAreWe2021,
2730 url = {https://openreview.net/forum?id=mPducS1MsEK},
2731 year = {2021},
2732 booktitle = {Thirty-fifth Conference on Neural Information Processing Systems Datasets and Benchmarks Track (Pre-Proceedings)},
2733 author = {Thomas Liao and Rohan Taori and Inioluwa Deborah Raji and Ludwig Schmidt},
2734 title = {Are We Learning Yet? A Meta Review of Evaluation Failures Across Machine Learning},
2735}
2736
2737@inproceedings{friedler2019comparative,
2738 year = {2019},
2739 pages = {329--338},
2740 booktitle = {Proceedings of the conference on fairness, accountability, and transparency},
2741 author = {Friedler, Sorelle A and Scheidegger, Carlos and Venkatasubramanian, Suresh and Choudhary, Sonam and Hamilton, Evan P and Roth, Derek},
2742 title = {A comparative study of fairness-enhancing interventions in machine learning},
2743}
2744
2745@inproceedings{fish2016confidence,
2746 organization = {SIAM},
2747 year = {2016},
2748 pages = {144--152},
2749 booktitle = {Proceedings of the 2016 SIAM International Conference on Data Mining},
2750 author = {Fish, Benjamin and Kun, Jeremy and Lelkes, {\'A}d{\'a}m D},
2751 title = {A confidence-based approach for balancing fairness and accuracy},
2752}
2753
2754@article{impossibility_of_fairness,
2755 numpages = {8},
2756 pages = {136–143},
2757 month = {mar},
2758 journal = {Commun. ACM},
2759 abstract = {What does it mean to be fair?},
2760 doi = {10.1145/3433949},
2761 url = {https://doi.org/10.1145/3433949},
2762 issn = {0001-0782},
2763 number = {4},
2764 volume = {64},
2765 address = {New York, NY, USA},
2766 publisher = {Association for Computing Machinery},
2767 issue_date = {April 2021},
2768 year = {2021},
2769 title = {The (Im)Possibility of Fairness: Different Value Systems Require Different Mechanisms for Fair Decision Making},
2770 author = {Friedler, Sorelle A. and Scheidegger, Carlos and Venkatasubramanian, Suresh},
2771}
2772
2773@inproceedings{disparate_impact_suresh,
2774 series = {KDD '15},
2775 location = {Sydney, NSW, Australia},
2776 keywords = {disparate impact, fairness, machine learning},
2777 numpages = {10},
2778 pages = {259–268},
2779 booktitle = {Proceedings of the 21th ACM SIGKDD International Conference on Knowledge Discovery and Data Mining},
2780 doi = {10.1145/2783258.2783311},
2781 url = {https://doi.org/10.1145/2783258.2783311},
2782 address = {New York, NY, USA},
2783 publisher = {Association for Computing Machinery},
2784 isbn = {9781450336642},
2785 year = {2015},
2786 title = {Certifying and Removing Disparate Impact},
2787 author = {Feldman, Michael and Friedler, Sorelle A. and Moeller, John and Scheidegger, Carlos and Venkatasubramanian, Suresh},
2788}
2789
2790@article{adversarial_examples,
2791 year = {2014},
2792 journal = {arXiv preprint arXiv:1412.6572},
2793 author = {Goodfellow, Ian J and Shlens, Jonathon and Szegedy, Christian},
2794 title = {Explaining and harnessing adversarial examples},
2795}
2796
2797@article{De_Mauro2018-mi,
2798 year = {2018},
2799 month = {September},
2800 pages = {807--817},
2801 number = {5},
2802 volume = {54},
2803 journal = {Inf. Process. Manag.},
2804 author = {De Mauro, Andrea and Greco, Marco and Grimaldi, Michele and
2805Ritala, Paavo},
2806 title = {Human resources for Big Data professions: A systematic
2807classification of job roles and required skill sets},
2808}
2809
2810@book{virginia_eubanks,
2811 address = {New York},
2812 publisher = {St. Martin's Press},
2813 year = {2018},
2814 author = {Eubanks, Virginia},
2815 title = {Automating Inequality: How High-Tech Tools Profile, Police, and Punish the Poor},
2816}
2817
2818@book{green2019smart,
2819 publisher = {MIT Press},
2820 year = {2019},
2821 author = {Green, Ben},
2822 title = {The smart enough city: putting technology in its place to reclaim our urban future},
2823}
2824
2825@article{haibe_kains,
2826 bdsk-url-2 = {http://dx.doi.org/10.1038/s41586-020-2766-y},
2827 bdsk-url-1 = {https://doi.org/10.1038/s41586-020-2766-y},
2828 year = {2020},
2829 volume = {586},
2830 url = {https://doi.org/10.1038/s41586-020-2766-y},
2831 ty = {JOUR},
2832 title = {Transparency and reproducibility in artificial intelligence},
2833 pages = {E14--E16},
2834 number = {7829},
2835 journal = {Nature},
2836 isbn = {1476-4687},
2837 id = {Haibe-Kains2020},
2838 doi = {10.1038/s41586-020-2766-y},
2839 date-modified = {2022-01-21 23:46:00 +0000},
2840 date-added = {2022-01-21 23:46:00 +0000},
2841 da = {2020/10/01},
2842 author = {Haibe-Kains, Benjamin and Adam, George Alexandru and Hosny, Ahmed and Khodakarami, Farnoosh and Shraddha, Thakkar and Kusko, Rebecca and Sansone, Susanna-Assunta and Tong, Weida and Wolfinger, Russ D. and Mason, Christopher E. and Jones, Wendell and Dopazo, Joaquin and Furlanello, Cesare and Waldron, Levi and Wang, Bo and McIntosh, Chris and Goldenberg, Anna and Kundaje, Anshul and Greene, Casey S. and Broderick, Tamara and Hoffman, Michael M. and Leek, Jeffrey T. and Korthauer, Keegan and Huber, Wolfgang and Brazma, Alvis and Pineau, Joelle and Tibshirani, Robert and Hastie, Trevor and Ioannidis, John P. A. and Quackenbush, John and Aerts, Hugo J. W. L. and Massive Analysis Quality Control (MAQC) Society Board of Directors},
2843}
2844
2845@article{mit_replication,
2846 year = {2020},
2847 month = {November},
2848 journal = {MIT Technology Review},
2849 author = {Douglas Heaven, Will},
2850 title = {AI is wrestling with a replication crisis},
2851}
Attribution
arXiv:2206.09511v2
[cs.LG]
License: cc-by-4.0