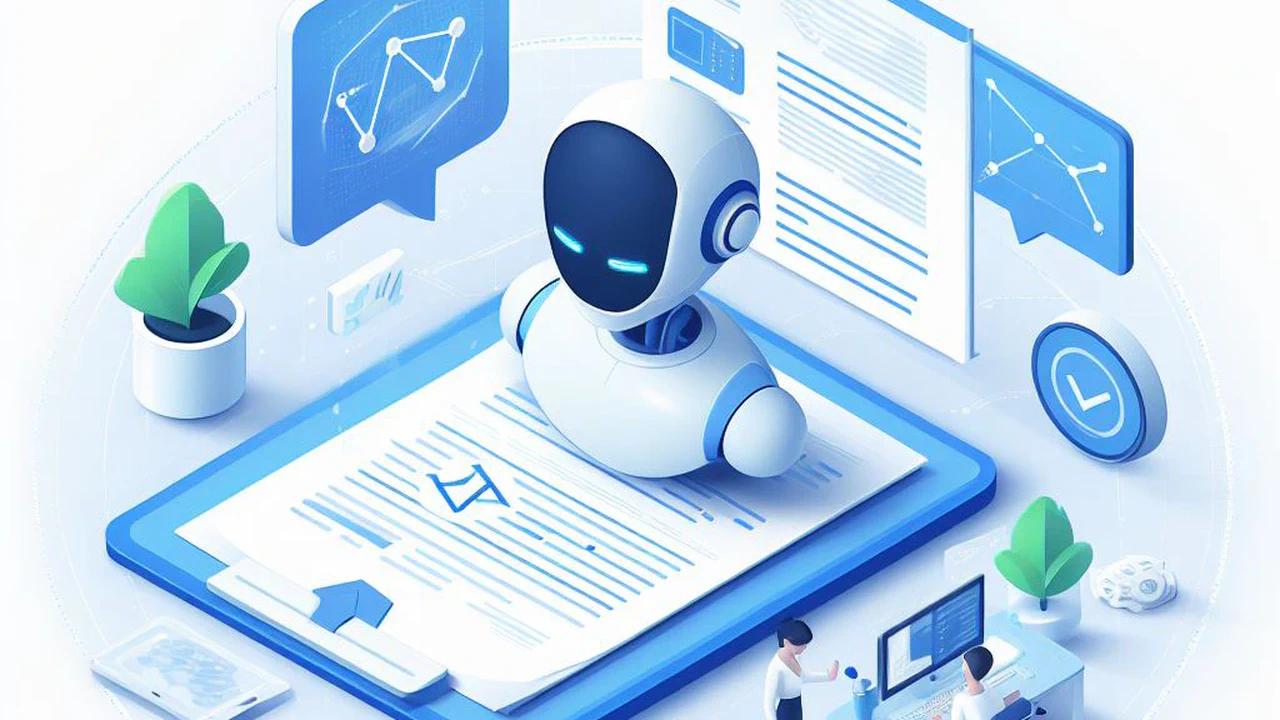
Papers is Alpha. This content is part of an effort to make research more accessible, and (most likely) has lost some details from the original. You can find the original paper here.
Introduction
In November 2021, the UN Educational, Scientific, and Cultural Organization (UNESCO) signed a historic agreement outliningshared values needed to ensure the development of Responsible Artificial Intelligence (RAI). RAI is an umbrella term that comprises different human values, principles, and actions to develop AI ethically and responsibly. Through UNESCO’s agreement, for the first time, 193 countries have standardized recommendations on the ethics of AI. While unprecedented, this agreement is just one of several efforts providing recommendations on different RAI values to be implemented within AI/ML systems.
In response, several industry organizations have begun to implement the recommendations, creating cross-functional RAI institutional structures and activities that enable practitioners to engage with the RAI values. For instance, several big-tech companies are implementing common RAI values, such as Fairness, Transparency, Accountability, and Privacy, as part of their RAI initiatives. However, such RAI values have minimal overlap with the values prescribed by UNESCO’s framework that promotes non-maleficence, diversity, inclusion, and harmony. Scholars have attributed the lack of overlap to different business and institutional contexts involved in developing AI/ML [hereafter, we use AI/ML systems to refer to both AI products and ML models] systems. Subsequently, it is essential to understand these contexts by engaging with practitioners across multiple roles who come together to co-produce and enact such RAI values. Co-production is an iterative process through which organizations produce collective knowledge. During co-production, individual practitioners may hold certain values (e.g., social justice), yet their teams might prioritize other values.hints at potential challenges that can arise due to such mismatches in RAI values. Our study builds on this critical gap by giving a detailed analysis of those challenges and strategies (if any) devised to overcome such strains as practitioners co-produce AI/ML systems.
We interviewed 23 practitioners across a variety of roles to understand their RAI value practices and challenges. Our findings show that institutional structures around RAI value co-production contributed to key challenges for the practitioners. We also discovered multiple tensions that arose between roles and organizations during prioritization, deliberation, and implementation. Interestingly, we also observed development of ten different RAI value levers. These are creative activities meant to engage individuals in value conversations that help reduce value tensions. In the remainder of the paper, we first discuss related work about collective values in Responsible AI from an HCI perspective and outline our research questions. We then present the research methodology and results of the study. We conclude with a discussion of our contributions in improving RAI co-production practices of AI practitioners. Overall, this work makes several contributions. First, we describe the experiences and the organizational environment within which AI/ML practitioners co-produce RAI values. Second, we illustrate multiple challenges faced by AI practitioners owing to different organizational structures, resulting in several tensions in co-production. Third, we unpack ten RAI value levers as strategies to overcome challenges and map them on to the RAI values. Lastly, we provide essential strategies at the different levels (individual, and organizational) to better facilitate and sustain RAI value co-production.
Related Work
The Field of Responsible AI
In the last decade, Responsible AI (RAI) has grown into an overarching field that aims to make AI/ML more accountable to its outcomes. One of the field’s roots lies in Ethical AI, where critical engagement with ethical values in the otherwise traditional AI/ML field have been encouraged. Example studies include engagement with core ethical values to provide nuance in technical AI/ML discourse, translating ethical values into implementation scenarios, and AI/ML guidelines, and seminal studies that brought critical ethical problems to the forefront.
RAI has also drawn its inspiration from AI for Social Good (AI4SG) research to study human values more broadly,going beyond “ethical” values. AI4SG helped the RAI field to translate such values embedded in AI/ML systems into positive community outcomesby eliciting specific values (e.g., solidarity), developing methods (e.g., capabilities approach), and producing representations (e.g., explanations) that strongly align with community goals (e.g., the UN Sustainable Development Goals). For example, studies have explicitly engaged with underserved communities to examine the impact of the embedded values within AI/ML systems in their lives (e.g., in agriculture, health, and educationdomains). More recent studies have shed light on how certain practitioners’ (e.g., data and crowd workers) practices, contributions, and values are often ignored while developing the AI/ML systems. Some have looked at different values (e.g., fairness) that are often ignored by the discriminatory algorithms. More recent work at the intersection of these two byhas also highlighted the impact of data workers of marginalized communities on the AI/ML algorithms to highlight complexity when building for RAI values like equity.
Lastly, RAI has also drawn motivation from recent movements associated with specific value(s). One such movement is around the value of explainability (or explainable AI ) that arose from the need to make AI/ML systems more accountable and trustworthy. A similar movement within the RAI’s purview focused on FATE values (Fairness, Accountability, Transparency, and Ethics/Explainability). While both movements have challenged the notion of universal applicability of RAI values, our study illustrates how these challenges do indeed appear in practice and the strategies used by practitioners on the ground to resolve these challenges.
Taken together, RAI has emerged as an umbrella term that encapsulates the above movements, encouraging critical value discourses to produce a positive impact. At the same time, departing from previous movements that focused on specific issues within AI/ML practices, RAI takes a broad institutional approach that promotes disparate AI/ML practitioners to come together, share, and enact on key values. Our study expands RAI discipline by surfacing on-ground challenges of diverse AI/ML practitioners attempting to engage in shared RAI responsibilities, such as collaborative value discourse and implementation. In the next section, we further unpack the notion of values, examine their roots in HCI and their current role in RAI.
Collective Values in Responsible AI: HCI perspectives
Science & Technology Studies field has long examined how values are embedded in technology systems in various social and political contexts. In recent years, studies within HCI have built on this foundation to bring a critical lens into the development of technology. Initial studies conducted by Nissenbaum and colleagues argued against the previously held belief that technology is “value-neutral”, showcasing how practitioners embed specific values through their deliberate design decisionsValue-sensitive Design_ (VSD) bywas another step in this direction. It has been used as a reflective lens to explore technological affordances (through conceptual and empirical inquiry) as well as an action lens to create technological solutions (technological inquiry). While VSD’s core philosophy remained the same, it has been extended, often in response to its criticisms.
A criticism relevant to this study is practitioners’ ease in applying VSD in the industry contexts. VSD is perceived to have a relatively long turnaround time,often requiring specialists for implementation. To overcome these challenges, Shilton proposed `value levers’ , a low-cost entry point for value-oriented conversations while building technology artifacts in the organization. Value levers are open-ended activities that engage participants in value-oriented discourses to develop common ground. With creative representations, value levers can transform slow and cumbersome value conversations into creative and fruitful engagements. While previous studies have applied and shaped the notion of value levers in a very specific set of contexts, such as showcasing how designers employ them in their practices, this work shows a broader utility of value levers among a diverse set of practitioners while navigating the complex space of RAI value discourse.
Within AI/ML research, initial exploration of values were still primarily computational in nature, such as performance, generalizability, and efficiency. With the advent of HCI and critical studies focusing on discriminatory algorithmsand responsible AI , the discussions shifted to much broader values, such as societal and ethical values, within the AI/ML field. These studies focused on exposing inherent biases in the models due to absence of substantive social and ethical values. For instance,demonstrated how complex ML models have inherent interpretability issues stemming from a lack of transparency about how predictions were achieved. Another set of studies byandscrutinized several algorithms governing the digital infrastructures employed in our daily lives to expose discriminatory behaviors within different situations, especially in the context of fairness,against marginalized populations. In a similar vein, several studies have explored individual values that they felt were critical for models, such as fairness, explainability, non-malfeasance, and justice, reflecting societal norms. A common underlying factor among several of these studies was that they focused on individual values enacted in their own spaces. Recently, however, a few studies have adopted contrasting perspectives which argue that values do not exist in isolation, but often occupy overlapping and contested spaces. Our study aims to provide much-needed deeper insights within this complex space by showing how practitioners engage with and prioritize multiple values in a contested space.
Another value dimension explored is – “whose values should be considered while producing AI/ML algorithms?” Most studies have engaged with end-users values, lending a critical lens to the deployed models and their implications on society. These studies challenged whether developing fair algorithms should be primarily a technical task without considering end-users’ values. Subsequently, researchers leveraged action research (e.g., participatory approaches) to design toolkits, frameworks, and guidelines that accommodated end-user values in producing ML models.
A more relevant set of studies have recognized the importance of understanding the values that different practitioner roles embedded while producing responsible algorithms. Such practitioner-focused studies are critical in understanding “how” and “why” particular values are embedded in AI/ML models early on in the life cycle. However, these studies have explored particular practitioners’ values in silos, leaving much to be learned about their collective value deliberations. A nascent group of studies has answered this call. For examplefocused on controlled settings, where specific practitioners’ values could co-design a fairness checklist as one of their RAI values.explored a broader set of values of practitioners and compared it with end-users in an experimental setting. Another relevant study byhas explored RAI decision-making in an organizational setting, laying a roadmap to get from the current conditions to aspirational RAI practices.
Our study contributes to this developing literature in four ways. First, within the context of Responsible AI practices, our study goes beyond the scenario-based, controlled settings, or experimental setups by focusing on natural work settings, which echoes the sentiment of some of the previous open-ended qualitative studies that were conducted in the organizations, but not in the context of Responsible AI practices. Second, we focus on a diversity of stakeholder roles, who are making an explicit effort to recognize and incorporate RAI values, unlike siloed studies previously. Third, we leverage the lens of co-productionto study RAI values in natural work settings. Fourth, our study extendsby explicitly unpacking the co-production challenges deeply rooted in RAI values. To this end, we answer two research questions:
(RQ-1) : What challenges do AI/ML practitioners face when co-producing and implementing RAI values ?
(RQ-2) : In response, what strategies do practitioners use to overcome challenges as they implement RAI values ?
Co-production as a Lens
To answer our research questions, we employed the conceptual framework of co-production proposed by. She defined co-production as a symbiotic process in which collective knowledge and innovations produced by knowledge societies are inseparable from the social order that governs society. Jasanoff characterized knowledge societies broadly to include both state actors (e.g., governments) and non-state actors (e.g., corporations, non-profits) that have an enormous impact on the communities they serve. Studying co-production can help scholars visualize the relationship between knowledge and practice. Such a relationship offers new ways to not only understand how establishments organize or express themselves but also what they value and how they assume responsibility for their innovations.
To operationalize co-production in our study, we invoke three investigation sites, as Jasanoff proposed. The first site of exploration is the institutions containing different structures that empower or hinder individuals to co-produce. The second site examines different types of discourse that occur as part of the co-production activities. Solving technological problems often involve discourses producing new knowledge and linking such knowledge to practice. The last site of co-production is representations produced both during co-production to facilitate discourses and after co-production in the form of the end-product. The three sites of the co-production framework are appropriate for understanding the current industry challenges around RAI innovation for several reasons. Technological corporations developing AI/ML innovations have a robust bi-directional relationship with their end-user communities. Moreover, for successful RAI value implementation, practitioners need to leverage the complex structures within their organizations that are invisible to external communities. RAI value implementations occur through strategic discourses and deliberations that translate knowledge to effective execution. Lastly, in the process of RAI value deliberations, individuals co-create representations that further the implementation efforts of RAI.
Methods
To answer our research questions, we conducted a qualitative study consisting of 23 interviews with active AI/ML practitioners from 10 different organizations that engaged in RAI practices. After receiving internal ethics approval from our organization, we conducted a three month study (April-June, 2022). In this section, we briefly talk about the recruitment methods and participant details.
Participants Recruitment and Demographics
To recruit AI/ML practitioners who actively think and apply RAI values in their day-to-day work, we partnered with a recruitment agency that had strong ties with different types of corporate organizations working in the AI/ML space. We provided diverse recruitment criteria to the agency based on several factors, including gender, role in the company, organization size, sector, type of AI/ML project, and their involvement in different kinds of RAI activities. Using quota sampling technique, the agency advertised and explained the purpose of our study in diverse avenues, such as social media, newsletters, mailing lists, and internal forums of different companies. For the participants that responded with interest, the agency arranged a phone call to capture their AI/ML experience, as well as their experience with different RAI values. Based on the information, we shortlisted and conducted interviews with 23 AI/ML practitioners who fit the diverse criteria mentioned above. The aforementioned factors were used to prioritize diverse participants with experience working on RAI projects within their team in different capacities. For example, while shortlisting, we excluded students working on responsible AI projects as part of their internships and included individuals who were running startup RAI consultancy firms.
Out of the 23 practitioners, 10 identified themselves as women. Participants comprised of product-facing roles, such as UX designers, UX researchers, program/product mangers, content & support executives, model-focused roles, such as engineers, data scientists, and governance focused-roles, such as policy advisors and auditors. Out of 23 practitioners, all but one participant worked for a U.S. based organization. However, participants were geographically based in both Global North and Global South. Participants also worked in a wide variety of domains, including health, energy, social media, personal apps, finance and business among other, lending diversity to the captured experiences. Three participants worked for independent organizations that focused exclusively on RAI initiatives and AI governance. twelve participants had a technical background (e.g., HCI, computer-programming), four had business background, two had law background and one each specialized in journalism and ethics. For more details, please refer to Tabletab-org-participants.
Procedure
We conducted semi-structured interviews remotely via video calls. Before the start of the each session, we obtained informed consent from the participants. We also familiarized participants with the objective of the study and explicitly mentioned the voluntary nature of the research. The interviews lasted between 40 minutes and 2 hours (avg.= 65 mins.) and were conducted in English. Interviews were recorded, if participants provided consent. Our interview questions covered different co-production practices. First, in order to understand different co-production challenges (RQ-1), we asked questions about (1) how practitioners faced challenges when sharing RAI values across roles (e.g., “Can you describe a situation when you encountered problems in sharing your values? ’’ ) and (2) how practitioners faced challenges when collaborating with different stakeholders (e.g., “What challenges did you face in your collaboration to arrive at shared common responsible values? ’’). Second, to understand different co-production strategies (RQ-2) we asked (3) how practitioners handled conflicts (e.g., “Can you give an example where you resisted opposing peers’ values? ’’) and (4) how practitioners sought assistance to achieve the alignment in RAI values (e.g., “What was the most common strategy you took to resolve the conflict ?’’). To invoke conversations around RAI values, we used a list of RAI values prepared byas an anchor to our conversations. After first few rounds of interviews, we revised the interview script to ask newer questions that provided deeper understanding to our research questions. We stopped our interviews once we reached a theoretical saturation within our data. We compensated participants with a 75$ gift-voucher for participation.
Data collection and Analysis
Out of 23 participants, only three denied permission to record audio. We relied on extensive notes for these users. Overall 25.5 hours of audio-recorded interviews (transcribed verbatim) and several pages of interview notes were captured. We validated accuracy of notes with the respective participants. Subsequently, we engaged in thematic analysis using the NVivo tool. We started the analysis by undertaking multiple passes of our transcribed data to understand the breadth of the interviewee’s accounts. During this stage, we also started creating memos. Subsequently, we conducted open-coding on the transcribed data while avoiding any preconceived notions, presupposed codes, or theoretical assumptions, resulting in 72 codes. We finalized our codes through several iterations of merging the overlapping codes and discarding the duplicate ones. To establish validity and to reduce bias in our coding process, all the authors were involved in prolonged engagement over multiple weeks. Important disagreements were resolved through peer-debriefing. The resultant codebook consisted of 54 codes. Example codes included, social factors’,
prior experience’, enablers’,
RAI pushback’.As a final step, we used an abductive approachto further map, categorize, and structure the codes under appropriate themes. To achieve this, we used three key instruments of co-production framework developed by, namely, making institutions , making discourses , and making representations . Examples of the resultant themes based on the co-production instruments included value ambiguity’,
exploration rigidity’, value conflicts’, and
value lever strategies’. Based on the instruments of co-production framework, we have present our resultant findings in the next section.
Findings
Our overall findings are divided based on different sites of exploration proposed by. The first section answers RQ-1 by exploring several institutional challenges that hinder the co-production of RAI values among practitioners (Section section-1). The second section explores subsequent knock-on challenges that unstable institutional structures create in co-production discourses (Section section-2). The last section answers the RQ-2 by presenting carefully thought out representations that overcome challenges deliberation and execution of RAI values using the concept of value levers. (Section section-3).
RAI Value Challenges within the Institutional Structures
Institutional structures are essential in enabling co-production of new knowledge. It is these structures that facilitate relationships for deliberation, standardize democratic methods, and validate safety of new technological systems before information is disseminated into the society. We found two key institutional structures that facilitated deliberation around RAI values within AI/ML companies. These structures brought about different RAI challenges.
Bottom-up: Burdened Vigilantes The First type of structures were bottom-up. Within these structures, RAI conversations developed through RAI value sharing in the lower echelons of organizations, often within AI/ML practitioners’ own teams. In our interviews, eight practitioners, namely a UX researcher, designer, content designer, and program manager from two mid-size organizations and two large-size organizations experienced or initiated bottom-up practices that engaged with RAI values. One of the enablers for such bottom-up innovation was individuals’ sense of responsibility towards producing AI/ML models that did not contribute to any harm in society. A few other practitioners paid close attention to `social climate ’ (e.g., LGBTQ month, hate speech incidents) to elicit particular RAI values. For instance, P08, a program manager in a large-scale technology company took responsibility for RAI practices in their team but soon started supporting team members to come together and share RAI values:
“We cater to projects that are very self-determined, very bottom-up aligned with our values and priorities within the organization …These are what I call responsible innovation vigilantes around the company. I also started that way but have grown into something more than that. You’ll see this at the product or research team level, where somebody will speak up and say, `Hey, I want to be responsible for these RAI values, make this my job and find solutions’. So you start to see individuals in different pockets of the company popping up to do RAI stuff. ”
A key challenge with such bottom-up structures was that the responsibility of engaging with RAI value conversations implicitly fell on a few individual “vigilantes ”. They had to become stalwarts of particular RAI values and take out substantial time out of their work to encourage and convince their teams to engage with RAI values. They also actively seeked out RAI programs available within and outside their organization. When such RAI programs were not available, individuals took it upon themselves to create engagement opportunities with other members within the organization. These bottom-up structures were useful in breaking the norms of “boundary-work” that are often set within AI and similar technical organizational work where only engineers and high-officials in the company maintain control. It allowed non-technical roles such as user experience researchers, product managers, analysts, and content designers to a create safe space and lead the RAI efforts. While such efforts early on in AI/ML lifecycle minimized the potential harm of their ML models or AI products, it often happened at the cost of their overworked jobs.
Bottom-up: Burdened with Educational Efforts Apart from self-motivated vigilantes, the burden of RAI value exploration also fell on a few practitioners who were implicitly curious about RAI innovation. Unlike the vigilantes, these participants were pushed to become the face of their team’s RAI initiatives since there was no-one else who would. P14, a product manager working for two years at a medium size company within the energy sector shared,
“When I came in to this team, nobody really believed in it [RAI] or they really didn’t think it [RAI] was important. I was personally interested so I was reading about some of these principles …When there was an indication of a future compliance requirement, people didn’t want to take up this additional work …somebody had to do it. ”
Similarly P05, a technical manager leading their team on data collection for the development of knowledge graphs, revealed how they were considered “the face of privacy ” for the team. Therefore, P05 was expected to foster awareness and common understanding among internal stakeholders and external partners and ensure they strove towards similar RAI standards and appreciated data-hygiene practices (e.g., data cleaning and de-identification). Practitioners like P14 and P05 had to assume the responsibility of figuring out the RAI space by presenting their team’s needs and asking formative questions even when their objectives around RAI were often not clear, such as which values to consider (e.g., “privacy or transparency? ”), what certain values mean (e.g., “what trustworthiness as an RAI value should mean to the model and the team ”), how to operationalize specific values (e.g., “How does trustworthiness apply to rule-based models? What kind of RAI values to invoke while collecting data?’’ , and how to interpret outcomes and map them on to their team’s objectives.
Participants (n=5) shared how leading such RAI initiatives burdened their professional lives in various ways. Multiple participants reported that the RAI field was still in its infancy and taking up responsibilities in such conditions meant that their efforts were not deemed a priority or sometimes even officially recognized as AI/ML work. Consequently, the practitioners possessed limited understanding of the direction to take to educate their team, convert their efforts into tangible outcomes, and effectively align their educational outcomes to the team’s objectives. P13, an RAI enthusiast and an engineer at a large-scale social media company shared how their RAI effort seemed like an endless effort, “At this point, I probably know more about what things (RAI values) we don’t want in it (model) than what we do want in it …It’s like I am just learning and figuring out what’s missing as I take every step …It is unclear which [RAI] direction will benefit the the team.” Moreover, the burden of educating multiple team members was on the shoulders of a very few practitioners tantamounting to substantial pressure.in their paper around technology ethics put forward the term _ethic owners_ '. This role shares similarity with the bottom-up vigilantes and the educators as they both are motivated and self-aware practitioners, invested in foregrounding human values by providing awareness and active assistance while institutionalizing the processes. However, Metcalf's ethic owners' responsibilities were clearly defined. Their tasks of working with teams or higher management were perceived as visible, prioritized work for which they would be credited for career growth or otherwise.While bottom-up informal roles in our research performed similar set of tasks, their efforts were seen as tangential,
administrative’, and underappreciated. It is not just that there was an additional burden, but even the outcomes of taking on this additional burden for bottom-up informal roles were dissimilar to the ethic owners. Taking up informal RAI work was more problematic when the requirements in the later stages of ML were unprompted, compelling practitioners to focus on these efforts at expense of their own work.
In our findings, one form of the need came as academic criticism or critique around particular values that were seen concerning a particular product (e.g., “what steps are you taking to ensure that your model is equitable?’’ ). Another form of need came from end-users’ behavior who experienced the models through a particular product. P20, a user experience researcher working with deep learning models in finance, shared how user feedback brought about new RAI needs that became their responsibility:
“Once the users use our product and we see the feedback, it makes us realize, oh, people are sometimes using this feature in an unintended way that might in turn impact the way we are going about certain values, say transparency' …. Initially we were like,
We should strive for transparency by adding a lot of explanations around how our model gave a particular output’. Later we realized too many explanations [for transparency] fostered inappropriate trust over the feature…UXR represents user needs so its on me to update the team on the issues and suggest improvements.
A few practitioners (n=2) also mentioned how the constant juggling between their own role-based work and the unpredictability of the RAI work pushed them to give-up the RAI responsibilities all-together.
Top-down: Rigidity in Open-discovery While the burden of ownership and execution of RAI values in bottom-up structures were on small group of individuals, they had the flexibility to choose RAI values that were contextual and mattered to their team’s ML models or projects. On the contrary, we found that top-down institutional structures limited the teams’ engagement to “key ” RAI values that impacted organization’s core business values. For instance, P15’s company had trust as a key value baked into their business, requiring P15 to focus on RAI values that directly reduced specific model’s biases, thereby increasing the company’s trust among their users. Consequently, several RAI practitioners had to skip RAI value exploration and sharing. Instead they directly implemented predetermined RAI values by the management just before the deployment. P06, an engineer at a large tech company working in conversational analysis models, described this lack of choice:
“To be honest, I imagine lots of the conversations, around the sort of values that need to go into the model, happened above my pay grade. By the time the project landed on my desk to execute, the ethics of it was cleared and we had specific values that we were implementing. ”
Public-oriented legal issues and ethical failures, especially when launching innovative models (e.g., transformer networks), also determined the RAI values that were prioritized and the subsequent formal RAI structures that were established by the organizations. P19, a policy director at a RAI consultation firm facilitating such structures, shared how such impromptu structures were quite common in response to ever-changing laws around AI governance:
“Even if you’re conservative, the current climate is such that it’s going to be a year or two max from now, where you will start to have an established, robust regulatory regime for several of these (RAI) issues. So a good way to be prepared is to create the [RAI] programs in whatever capacity that enables companies to comply with the new regulations, even if they are changing because if you have companies doing Responsible AI programs, it eventually gets compliance and executive buy-in. ”
Instead of investing in RAI structures to comply with different regulations in Tech Ethics such as codes of ethics, statements of principle, checklists, and ethics training as meaningful, organizations perceive them as instruments of risk that they have to mitigate. In line with the previous literature, our findings indicate that practitioners often find false solace in such structures as they run the risk of being superficial and relatively ineffective in making structures and practices accountable and effective in their organizations. However, adding nuance to this argument in the case of RAI practices, we found that practitioners more broadly devoted time and energy to follow established and prioritized values (e.g., trust or privacy) due to directed and concerted focus. It allowed for organization-wide impact since the “buy-in” already existed.
Top-down: Under-developed Centralized Support However, in the case of less clearly defined values (e.g., non-maleficence or safety) we observed a limited scope for nuance and despite best efforts, the centralized concerted direction does not always pan out as intended. Further, while laws continue to evolve in this space, participants felt that pre-mediated RAI values might not longitudinally satisfy the growing complexity of the ML models being implemented (e.g., multimodal models). Hence, while it might seem that setting up a centralized top-down approach might be efficient, the current execution leaves much to be desired. In fact, based on data from over half the participants, we found that five top-down structured companies integrated lesser known RAI values into their workflows in multiple ways without establishing a centralized workflow. Those who did establish centralize workflows created consulting teams to advise on RAI Practices (similar to ethic owners).
However, these top-down centralized RAI consulting teams were not always set up to succeed. As is the nature of consulting, people did not always know the point-of-contact or when and how to reach out. The consulting teams needed to also consider opportunities to advertise about themselves and engagement mechanisms, which was difficult due to the lack of context and nuance around the teams’ projects. Consequently, it was difficult for such teams to generate organic interest, unless the teams were already aware of their RAI requirements and knew a point of contact. P10, a manager who facilitated one such top-down RAI program in a large-scale technology company for AI/ML teams, described lack of fixed ways in which teams engaged with them on RAI values, making it a difficult engagement:
“We have a bunch of internal Web pages that point you in all different types of directions. We don’t have a singular voice that the individuals can speak with …. Its currently hodgepodge. Some teams come to us willingly. They had already thought about some harms that could occur. They say, `Here’s our list of harms, here’s some ideas on what we want to do’ They’d already done pre-work and are looking for some feedback. Other teams come to us because they’ve been told to. …They haven’t thought much about RAI and need longer conversations …Other teams were told to go track down an individual or team because they are doing ML stuff that will require RAI assistance, but they don’t know about us ’’
Challenges within RAI value Discourses
Fruitful co-production requires well-established institutional structures that can empower stakeholders to engage in stable democratic discourses with an aim of knowledge production. In the previous section, we uncovered different structural challenges at the institutional level that contributed to knock-on effects, creating further challenges for practitioners during the co-production and implementation of RAI values. Discourse: Insufficient RAI Knowledge A key challenge that many practitioners experienced in co-producing RAI values in team was the difficulty in engaging deeply with new and unfamiliar RAI values deemed important by the team members. P07, a policy advisor in a large technology company, who regularly interacted with those who implemented RAI values, described the “superficial engagement ” with values as an act of “ineffective moralizing ”, wherein practitioners struggled to develop deeper interpretations of the team’s shared values and contextualize them in relation to the ML models they were developing.
P07 mentioned several key critical thinking questions that AI/ML practitioners did not deliberate within their teams, such as “Is this RAI value applicable to our product? ”, “how does this value translate in diverse use cases? ”, or “should this value be enabled through the product? ” The need for deeper engagement becomes particularly important in a high-stakes situation, such as healthcare, where certain conditions have an unequal impact on particular demographics. P12 experienced this complexity while overseeing the development of a ML model focused on health recommendations:
“So a lot of models are geared towards ensuring that we have we are predictive about a health event and that almost always depends on different clinical conditions. For example, certain ethnic groups can have more proclivity to certain health risks. So, if your model is learning correctly, it should make positive outcomes for this group more than the other groups. Now, if you blindly apply RAI values without thinking deeply about the context, it might seem that the model is biased against this group when in reality these group of people are just more likely to have this condition, which is a correct conclusion, not a biased one. ”
Such deeper analysis requires hands-on practice and contextual training in the field and formal RAI education. In our findings, top-down structures were only effective to fill the gap for key values that aligned with the company’s vision, leaving a much needed requirement for contextual, high-quality RAI education for more emergent RAI values that could be modularized for specific teams. P02, a content designer for a large health technology company shared how this gap looked like for their team that was designing content for a machine translation team,
“One thing that would have been beneficial is if I or my team could somehow get more insights on how to think about trustworthiness in the context of the content produced by our machine translation model and probably evaluate it …Often time, I just go to someone who is known to have done some work in this [RAI] and say, `Hey, we want to design and publish the content for the model like in a month from now, what is bare minimum we could do from [RAI] principles point of view?’…Sometimes it’s never-ending because they say I have not thought about this at all and that it is going to take a month or maybe much more longer to get these principles implemented.”
Participants like P02 had no alternative but to reach out to their bottom-up structures to seek assistance, discuss, and reduce gaps in their RAI knowledge. On occasions, such avenues of discussion were non-conclusive. Prior literature in AI/ML and organization studies have shown how such unequal dependence on bottom-up structures over top-down in deliberation can contribute to tensions, and in turn propose an “open, federated system ” linking different actors, resources, and institutions to provide a community based support.
Discourse: Deprioritized Unfamiliar & Abstract Values Naturally, practitioners tried to solve the superficial engagement problem by de-prioritizing values that they found unfamiliar. In our study, most practitioners (n=18) said that they were familiar and comfortable talking about RAI values like privacy and security as they were already “established ” and had “matured over time ”. They sharply contrasted this perceived familiarity with other RAI values like explainability and robustness . The familiar values were well backed with stable top-down structures and dedicated teams, such as compliance departments and dedicate RAI personnel , making it easy for practitioners to develop mental models of deeper engagement. P20 shared their experience in this regard in their organization:
“The ideal situation would be like, Oh, I have certain RAI principles that I want to make sure our product has or addresses'. In reality not all the principles are thought out the same way and applied in the first go. It usually happens in layers. First and foremost, people will look at privacy because that's super established, which means everyone knows about it, they already have done probably some work around it, so its easy to implement. And then after that, they're like,
Okay, now let’s look at fairness or explainability’ …We usually have to be quick with turnaround like one or two months. Its nice to bring up values that are new but naturally they also require familiarizing and implementation effort within the team and people see that ”
Other practitioners (n=3) also followed a similar de-prioritization process for RAI values that they felt were abstract and did not have a measurement baseline (benchmarks) as opposed RAI values that could be easily measured quantitatively against a baseline. An example observed in this category was the contrast between RAI values like interpretability, which had concrete implementation techniques and measurements (e.g., LIME) and non-maleficence, which did not have a clear implementation technique or measurements. Similarly, practitioners (n=2) who went out of their way to understand and suggest new interpretability techniques for model debugging techniques (e.g., Integrated Gradients, SHAP) found it disempowering when their team members often negotiated for easier and computationally cheaper values like accuracy (e.g., P/E ratio) for implementation.
Discourse: Value Interpretation Tensions Even in situations, when different practitioners took a similar balanced approach to prioritization, tensions emerged as different roles interpreted and contextualized the RAI values differently during the value deliberations. We found these tensions occurring within practitioners when different practitioners defined RAI values (e.g., equity) and mapped them to RAI features and metric (e.g., skin tone) differently. P18, a senior data scientist leading an AI team in a non-profit institute, shared one such similar tension among their team members working on the same project,
“Probably half of my colleagues do believe that there is a cultural, and historical set of RAI values that can be applied to all the products organization wide. Other half are vehemently opposed to that concept and say that [RAI] values are always model and project dependent. So if you are talking about our long-term goal to establish a set of RAI principles, whose perspectives should be considered?…This is an uneasy space that needs careful navigation. ”
While deliberations might occur between team-members, they might occur within a practitioner, or between the team and the end-consumers of the product/service. Latter two usually surfaced with user-facing roles, e.g., Product Managers or UX Researchers. These roles have the responsibility to understand, internalize, and embody end-user values in addition to their own values. Overall, we found that practitioners in these roles had to invest more effort to tease out their own values from that of the end-users. P04 was a user experience researcher working on interfacing a large language model for natural conversations with users. While P04 was interested in eliciting better insights from the model’s behavior issues (i.e. interpretability), end-users were interested in a simplified understanding of the model’s opaque behavior (i.e. comprehensibility). A UX Researcher is, however, expected to be the voice of the user in the process. Consequently, they had the constant burden to elicit both sets of values appropriately.
Another set of tensions also occurred between practitioners and end-users . P22, an analyst in a financial firm, described how ML practitioners perceived RAI values to be mutable and negotiable, allowing them to implement a particular RAI value in stages instead of all at once. Such a process allowed P22 (and three other participants who reported similar narratives) to build the required experience and embed the value in the ML model or AI product. However, end-users expected these embedded RAI values as absolute and non-negotiable and not on a “sliding spectrum ” because they are “they are often the list of ignored rights ”, leading to practitioner-user RAI tensions.
Our findings show that tensions that arose from non-uniform RAI value knowledge and subsequent disparate value interpretations were unproductive and a significant obstacle in the overall co-production process of RAI values. This can be attributed to a nascent RAI field that has given rise to new forms of values (e.g., explainability, interpretability) whose definitions and contexts which keep changing. This is in contrast with prior value studies in HCI studies where the tensions and conflicts around relatively more established values (e.g., privacy) do not occur until the implementation stage. Our findings show that the majority of value tensions occur much earlier in the value interpretation stage, often contributing to the abandonment of the value discussions altogether.
Implementation: RAI Values and Conflicts within Implementation of RAI values was also not a straight forward process as implementing certain RAI values created conflict with other RAI values. For instance, P1, an engineer working on classification models in VR environments shared how their decision to improve accuracy by excluding instances of objects with sensitive cultural meanings (e.g., objects with LGBTQ references) also had direct repercussions on the diversity and fairness of the model. Implementing RAI values also created cascading dependencies on the inclusion of other RAI values. For instance, P16, a program manager working as an RAI facilitator for a big tech company, shared the issues team members experienced around cascading RAI values:
“One common issue I see is with teams that are dealing with model Fairness issues. Most often the solution for them is to improve their datasets or sometimes even collect new forms of demographic data to retrain their model and that opens up another rabbit hole around privacy that the team now has to navigate through and ensure that their data adhere to our privacy standards. More often than not, teams don’t even realize they are creating a new issue while trying to solve their existing problem. ”
Implementation challenges also occurred when organization’ business values were in tension with those of external clients. In such cases, the team’s commitment to engage with RAI was at odds with clients’ business priorities. P02, a technical program manager for a service company that developed ML models for clients in the energy sector, had a similar issue when their team was building a model for street light automation. After P02’s team received the data and started developing the model, they pushed for the value of safety. However, it was at odds with the company’s value of efficiency,
“We should prioritize model optimization in those areas where there are higher crime rates …we don’t want blackouts, right? …Their argument was if there was a very high crime rate, such areas will also have high rate of purposefully damaging the lighting infrastructure. Prioritizing service to such areas will only create high amounts of backlogs as people will just vandalize it again. …So they just have different priorities. After that, our team just stopped following it up as it went into the backlog. ”
P02’s team gave up RAI value deliberation and implementationaltogether after their clients either deprioritized their multiple attempts to make them RAI ready or took an extremely long time to approve their requests.
Implementation: Unexpected Late-stage Value Changes Another challenge practitioners faced was encountering new RAI values during late stages of implementation. These values were not initially shortlisted. Instead, they were brought out later and sometimes championed by a very vocal practitioner, who felt deeply about it. Such late-stage RAI values also became a part of the discussion when practitioners in the team uncovered last-moment issues (e.g., bias) during implementation that significantly impacted the model. Several participants (n=3) shared how such late-stage RAI values decreased the productivity of their overall RAI discourse and implementation efforts, leading to a negative experience. While such last-minute changes are not welcomed, P12, an engineer shared how it also gives an opportunity to the developers to ship a better product before any harm might have been done. This tension between potentially better outcomes and slower implementation was visible in how the implementation efforts and timelines were impacted.
Such values also disrupted a planned implementation by taking the spotlight and pushing the team in navigating the company’s non-standardized approvals, thereby significantly altering the project timeline. For example, P23, an ML engineer shared how when they received issues around fairness from other stakeholders, it meant “substantial changes to the model from the ground-up, because most of the time, issues with fairness stem from the data ”. It meant revisiting the data and redoing data collection or further debugging to remove the issues. Moreover, when new and untested RAI values assumed prominence (e.g., interpretability), more time and effort was required from the practitioners during implementation. RAI facilitators are essential in easing the tension in such situations by engaging in back-and-forth conversations with the teams to reduce the effort, streamline the process, and help practitioners appreciate the eventual consequences of implementing the RAI values.
Implementation: Perceived Misuse of RAI values Lastly, we also observed tensions between individual efforts in implementing RAI values and their organization’s use of such efforts for the overall business purposes. For instance, P15, research director of a large-scale technology company overseeing research in large language models, shared how he was actively supporting a few teams in his company to co-produce and embed explainability into their models. However, he also expressed his concern about how companies could misrepresent such embedded RAI values,
“I worry that explainable AI is largely an exercise in persuasion. This is why you should trust our software' rather than
This is why our software is trustworthy’ …I’m not saying everybody who does explainable AI is doing that kind of propaganda work, but it’s a risk. Why do we want our AI to be explainable? Well, we’d like people to accept it and use it …Explainability part is ethically complicated …even for explainability for the practitioners the company wants it to be explainable, transparent, reliable, all those things as a means to an end. And the end is `please like our model, please buy our software’ ”
We found two more practitioners who raised similar concerns with other RAI values, such as privacy and trust. They were concerned that making their product “completely responsible ” could enable companies to market their products as nearly perfect, leading to overtrust and over-reliance. These findings align with the ethics-washing phenomenon within the tech ethics literature which argues that companies sometimes invest in ethics teams and infrastructure, adopting the language of ethics to minimize external controversies and superficiallyengage with the proposed regulations. Practitioners who expressed these sentiments were quite dissatisfied with their RAI implementation work as they felt their actions were merely a “band-aid” solution for the organization, instead of meaningfully altering organization’s culture and practices.
Representational Strategies to Mitigate RAI Challenges
In response to the challenges mentioned in the aforementioned sections, we saw several strategies used by the practitioners to overcome the limitations in RAI value co-production. To present the strategies, we use a form of representations called values levers, a set of activities that facilitate opportunities to share and collaborate around values. We show how different practitioners use value levers to build upon current RAI institutional structures and make their RAI co-productionmanageable. In an ideal situation, value levers can also be employed in any situation of RAI co-production. For example, organizations created several formal RAI structures for practitioners to facilitate sharing and deliberation of values. These included top-down standardized guidelines, such as guidebooks (e.g., PAIR, HAX) around established RAI values, bringing in experts to share their experiences around co-production (lectures), and enabling shared spaces for co-production. However, in this section, we will be looking at value levers specifically developed in response to the challenges experienced in RAI value co-production.
Institutional Value Levers: External Expertise and Certifications to reduce Ambivalence One of the ways in which organizations brought stability to their inconsistent top-down RAI institutional structures was by taking assistance of independent agencies or professionals who specialized in establishing values levers that helped streamline their existing structures. One such value lever was `Responsible AI certifications ’ that were designed to bring different recognized and informal RAI co-production activities under one-roof. These programs act as facilitators between the non-technical and technical workforce by enabling co-production around RAI values to make them compliant with upcoming regulations. Participants reported that different activities were packaged into the RAI certification program, such as getting buy-in for particular RAI values, leveraging trusted partners for running impact assessments, engaging key actors in value discovery and prioritization, and implementing appropriate RAI methods. P19, policy director of one such RAI certification organization, shared how these certifications are effective in sectors, such as energy, mining, and human resources that often have a limited technology workforce. They described effort of facilitating RAI value conversations within their client teams as a key part of the certification process:
“It is important to have everybody on board for those [RAI] value conversations. So we try really hard to have all the different teams like internal or external audit, legal, business, data and AI team come together, brainstorm, discuss different [RAI] issues in specific contexts and shortlist [the RAI values], even if we just get a little bit of their time …everyone needs to be brought in early because we conduct a lot of activities likes audit analysis, bias testing…it saves time, addresses several concerns, and establish streamlined [RAI] processes. …For simplicity, we just package all of the different activities we do under RAI certification. …Some times few activities are already being executed by the organization, we just do the job of aligning them in a way that works for the organization. ”
Such external expertise and certifications can provide an opportunity for open discovery, bolster existing centralized support, and identify RAI values that might otherwise be discovered at the last stages.
Institutional Value Levers: Activities to Distribute RAI burden We also found several nascent but more focused value levers in bottom-up institutions focused on distributing the burden experienced by a few roles more widely within the team. These value levers provided opportunities for increased participation from stakeholders, especially in the starting stages by enabling them to bring-in complementary RAI values into the team. Most commonly used levers in this context included scenario-based narratives and role plays, and open-ended activities that engaged practitioners in opinion formation and sharing. Other value levers included conducting a literature review of specific RAI values and applicable cutting-edge methods, definitions, and guidelines around them to share and invoke feedback from the team. We also observed more experimental value levers that were geared towards bringing-in complementary RAI values of external stakeholders (e.g., end-users) into the team.
For example, P18, a data scientist working in a startup, hosted a panel to capture complementary perspectives around AI explainability. Visibility into how explainability was perceived differently by different community members, such as NGOs and government, contributed to a better understanding and alignment within the team to develop explainable models. In a similar example, P09, an engineer working on a reinforcement learning model in the context of healthcare for low resources in India, facilitated field visits to the end-user communities. Such exposure helped roles that were passive in sharing their values as well as roles that were thinking about new values, such as social justice, in the RAI discourse. Overall, these value levers (narratives, role-plays, literature reviews, panels, and field visits) focused on primarily bottom-up structures, which helped reduce pressure on specific roles and limit superficial value engagements.
Discourse Value Levers: Facilitating Disagreements Moving our focus to RAI value co-production, we saw user-facing practitioners create explicit opportunities for disagreements and healthy conflicts to tackle the problem of superficial value engagement and improve the quality of their teams’ deliberations. Disagreements in co-production phase allowed practitioners like UX researchers and product managers to think inclusively, capture diverse perspectives and expert knowledge, and more importantly predict future value conflicts. For example, P04, a UX researcher, created bottom-up adversarial prioritization framework. In the starting phases of this framework, the UX researcher pushed team members to go broad and co-produce values by wearing other practitioner’s hats and invoking their RAI values. This practice allowed them to bring forward interesting disagreements between different roles that were then resolved and prioritized to achieve a small set of meaningful RAI values. P04 recalled two of the values that received maximum disagreements were diversity and inclusion. Wearing complementary user hats enabled practitioners to familiarize with these values that were otherwise unfamiliar in their own roles. Other top-down RAI programs also facilitated similar structures, explicitly providing narratives that brought out disagreements, e.g.,:
“Usually I will write something in the prompts that I think that the team absolutely needs to hear about but is controversial and opposing. But what I do is I put it in the voice of their own team so that it is not coming from us. It is not us scrutinizing them. That promotes interpersonal negotiation that pushes individuals to really defend their values with appropriate reasoning. ”
According to P19, having such RAI system in place early also allows companies to judge its ML models benchmarks when compared to their competition. Leveraging the adversarial prioritization framework appropriately in both top-down and bottom-up structures can enable open-discovery, and surface the values and related conflicts for resolution.
Discourse Value Levers: Model Cards & Visual Tools to Reduce Abstractness from Values We found that practitioners also created succinct representations and simplified documentation to bring much needed clarity to various RAI values and simply associated models. For instance, engineers shared documentation of model and data cards, making it easier for non-engineering and engineering roles to grasp the information. P23, a senior engineer at an AI startup looking into sustainability, shared the process:
`Even we have introduced this concept of a model card, wherein if a model is developed, the model card has to be filled out. So what is a model card? A model card is a series of questions that captures the basic facts about a model at a model level at an individual model level. What did you use to build a model? What was the population that was used? What is the scoring population? It is like, having all of that in a centralized standard format. Goes a long way to roll it up because the product can be very complex as well, right? With multiple players and whatnot. But having that information collected in this way benefits other roles that own the product to think about different values that are missing '
UI/UX designers, UX researchers, and analysts also used similar documentation tools to initiate discussions and receive feedback from other practitioners in the team. P20, a UX researcher, used presentation slides containing model features to facilitate brainstorming sessions and receive feedback from other roles. They also repurposed tools and methods used in their own work to give shape to their peers’ abstract values. For example, P20 reused online jam-boards containing key RAI values and user findings for affinity diagramming, enabling the team to “categorize the findings and map them to specific RAI values ”. Other RAI levers in this category included designing and sharing infographics and regular RAI standups where practitioners took it upon themselves to be stewards of RAI principles for the team to give updates, receive feedback and learn about team’s perspectives on specific RAI values.
Implementation Value Levers: Office Hours, User stories, Safe Spaces to Reduce Tensions
A few value levers that were part of top-down RAI programs were also effective in reducing various value tensions that occurred between different practitioners(n=2). One such program was RAI office hours that was available for elicitation and production, but were also extremely effective for tension-resolution. A typical office hour was 30 minutes in which practitioners engaged with a relevant expert and an experienced facilitator. One key way experts solved the tensions in these sessions was by collecting and providing concrete case-study examples. For example, P21, an RAI office hour facilitator, shared an example about the use of his office hours. The practitioners were at odds with each other in implementing explainability and trustworthy features. During the office hours, P21 responded by sharing an edge case scenario where even good explanations might backfire, such as, “If a pregnant woman had a miscarriage, showing even good end-user explanations around why they are seeing infant-related content can be very problematic. Explainability should be carefully teased out based on the context in which it is applied.”
Another set of value levers used especially by the roles facing end-users were user stories and scenarios to influence and persuade users to change their value priorities and align with rest of the team. These levers were also used by the roles to converge on key values after engaging in healthy conflicts within the divergence phase. For example, P04, exposed different pain points and key user journeys by “highlighting the clip of a user that is really, really amazing story that is either very painful or poignant ”. Interestingly, P04 was aware how such value levers had to be evoked carefully,
“If that story is not representative, I’m manipulating the system. If it is representative, I’m influencing the system…I will have to be careful not operate on the side of manipulation and try to be very squarely on the side of influence. So, I do like regular checks for myself to make sure that I am operating on influence, not manipulation, in terms of the stories that I am allowing people to amplify.”
Lastly, in order to tackle several types of value conflicts in the co-production of RAI values, we found different RAI value levers that focused on improving value alignment. One key alignment strategy was to create structures and activities that aligned the team’s RAI values pretty early in the process. One such activity that we saw in both practitioner’s and RAI programs was providing a safe space to encourage open discussions among individuals to empathize with other members. P09 shared,
“One alignment strategy was open discussion with the safe space, where team members could fail, be called out and to learn from each other as we were developing values. So say someone finds the value of democratization really important, they are made to articulate what they mean by it.…. It is easy if there are different buckets in which they can categorize and explain because then people can easily surface all the different ways they think and prioritize values and that helps with alignment ”
Discussion and Future Work
Overall, our findings show that co-production of RAI values in practice is complicated by institutional structures that either support top-down decision-making by leadership or are inhabited by bottom-up practitioners exercising voluntary agency (section section-1). In other case, multiple challenges exist when practitioners have to reconcile within their internally held values and RAI values expected from their roles; and between themselves and their team-members. Our findings also show that discourse around alignment and prioritization of RAI values can sometimes be unproductive, non-conclusive, and disempowering when practitioners have to implement said RAI values (section section-2). We observed a lack of transparency, and unequal participation within organizations; and between organizations and end-users of their products/services (section section-2). Despite the relatively complicated lay of the land, practitioners have been pushing ahead and discovering multiple strategies on how to make progress (section section-3). In the subsections below we will unpack these challenges, strategies and potential future work across the three sites of co-production: institutions, discourse, and representations.
Envisioning balanced Institutions: Middle-out RAI Structures
Inequity in Burden According to, strong institutions provide a stable environment for effective knowledge co-production. They can also act as safe spaces for nurturing and transforming contested ideas to effective practices leading to long-lasting impact on the immediate ecosystem. Recent scholarship byhas put faith in an aspirational future where organizations would have deployed strong institutional frameworks for RAI issues. Our findings show that as of today, top-down structures are underdeveloped. Organizations have deployed structures that range from being reactive to external forces (e.g., compliance, public outcry) by tracking teams that implement RAI to proactively establishing structures that make teams RAI-ready (e.g., office hours). Furthermore, stable workflows have been established for a limited number of values or use-cases, restricting the number of teams that could leverage such workflows.
Being in the midst of restrictive structures, particular practitioner roles embraced the persona of bottom-up vigilantes and self-delegated themselves to be champions of lesser known RAI values (e.g., non-maleficence and trustworthiness). They initiate open-ended exploration for value discourses and subsequent value implementation. However, such bottom-up structures also put burden and occupational stress on selective roles, risking the implementation success of such RAI projects. In particular, we have found that roles like UX researchers, designers, product managers, project managers, ethicists have been taking the brunt of this work. These findings build on the previous work, highlighting existing inequity and subsequent individual activism being performed by some - either by volition or due to lack of alternatives. Enabling Equal Participation Going forward, there is a need for a holistic middle-out approach that seeks a balanced synergy between top-down and bottom-up structures while balancing for the challenges that each of the structures provide. For instance, organizations can work with RAI value stalwarts and champions to formalize and streamline bottom-up workflows, making it a standard practice for all teams to engage in open-ended exploration of RAI values. Such a process can enable teams to look beyond loosely applicable organization-recommended RAI values and shortlist those values that actually matter and apply to their team. To standardize the structure, organizations can leverage independent (flat) team/roles that can guide the target team through the team while giving enough room for exploration.
Organizations can also use a middle-out approach to reduce the burden and occupational stress on specific roles through several top-down activities. One such way is to facilitate structures that can lower the barrier for diverse internal stakeholders to engage in RAI value co-production, regardless of their proximity to AI products or ML models. For instance, data-workers and teams/roles that do internal testing of the models (dogfooding) can contribute to the RAI value co-production. Same structures can also enable engagement with external stakeholders, such as end-user communities, policy, experts, and governance agencies in the initial stages of value co-production. Consequently,practitioners’ chances to foresee or anticipate changing requirements could improve, especially in the later stages of AI/ML lifecycle. Better yet, this could potentially improve not just the “user experience” of value discourse, but also the efficiency of implementation - a goal valued by private companies. This could be a win-win situation for multiple stakeholders by helping the top-down RAI structures align with business goals. While, our research uncovered only top-down and bottom-up structures that were mutually exclusive, other structures might exist. For example, while we envisage middle-out structures to be advantageous, future research is needed to operationalize and simulate such structures; and discover existing implementations. There might be some challenges uniquely inherent in those structures. We encourage future researchers to continue this line of enquiry
Envisioning better Discourses: Enabling Critical RAI Value Deliberations
Negativity in Deliberations The ultimate aim of co-production discourse is to engage in competing epistemological questions. Jasanoff calls it interactional co-productionbecause it deals with explicitly acknowledging and bringing conflicts between two competing orders: scientific order brought about by technological innovations and social order brought about by prevalent sociocultural practices within the community. In our findings, several underlying conflicts surfaced between scientific and social order (section section-2). In one instance, practitioners had to choose between socially impactful but lesser explored RAI values (social order) and lesser applicable but established values with measurable scientific benchmark (scientific order). In another instance of tension an RAI value occupied competing social spaces (e.g., equity). The underlying issue was not the conflicts itself but lack of systematic structures that enabled positive conflict resolution around the conflict. Such implicit conflicts often met with deprioritization and conversations ended unresolved. There is an urgent need to transform such implicit conflicts to explicit positive deliberations. Organizations aiming for successful RAI co-production need to be more reflexive, mobilize resources to create safe spaces and encourage explicit disagreements among practitioners positively, enable them to constantly question RAI values or co-production procedures that help embed them. While we saw some instances of these explicit practices in the form of value lever strategies, such instances were sporadic and localized to very few teams.
Acknowledging Differences Safely Our findings around challenges within RAI value discourse also showcase the politically charged space that RAI values occupy in an organization. In their critical piece around implementation of values in organization,bring out a few value characteristics that are applicable to our study. First, values are not universal. Values are recognized, prioritized, and embedded differently based on a bunch of factors, such as practitioner’s role, organizational priorities and business motivations which make RAI as a complex space. In our findings, roles prioritized those RAI values that were incentivized by the organizations and the AI/ML community through computational benchmarks focused on the RAI value outcomes. This is problematic as the RAI values that have computational benchmarks and have implicit organizational incentives might not map onto the RAI issues that are pertinent in the community. One way to solve this mismatch is by rethinking the definition of benchmarks from value outcome to value processes taken up by different roles (or teams). For example, organizations can encourage teams to document their co-production journeys around lesser known RAI values that can act as RAI benchmarks.
The second issue thatbring out is whose value interpretations should be prioritized and considered? In our findings, tensions emerged as same values were interpreted and prioritized differently by different stakeholders. End-users viewed RAI values as immutable and uncompromisable, whereas practitioners viewed them as flexible and iterable. Similar tensions were also observed between theinternal stakeholders in contextualizing the same values. While we saw a few strategic value levers, such as office hours, they were mainly targeted at the stakeholders that were within the same team. Extending this line of value levers, we propose participatory approaches that take a balanced approach for both internal and external stakeholders. In particular, we take inspiration from participatory design fictions , a participatory approach using speculative design scenarios, to find alignment on polyvocal speculations around embedded values in the emergent technologies. Deliberations around the fiction narratives can be used to arrive at a common ground RAI value implementation that are both contextual and implementable.
Shaping Institutional Structures to Improve Discourse Employing co-production as a lens has also allowed us to reveal a very delicate, symbiotic relationship between the practitioners’ discourses and the institutional structures. Several of the discourse challenges observed in our findings, such as issues pertaining to the lack of RAI knowledge and deprioritization of lesser known values, stemmed not only from the lack of mature top-down structures but also dependency on a singular institutional structure. The limitations of top-down structures pushed several practitioners in-turn to create informal bottom-up structures, shifting the pressure from one structure to the other. We argue that RAI-focused organizations need to seek a balance between stability (top-down structures) and flexibility (bottom-up). In addition to new middle-out internal -facing institutional structures, RAI discourses can benefit from external -facing institutional structures that can help such discourses be impactful. This can be achieved by bringing in diverse external collaborators, such as NGOs, social enterprises, governmental institutes, and advocacy groups. It will also help avoid the issue of co-optationfor true meaningful impact of such structures on the practices.
Envisioning better Representations: Value Levers
Value Levers Enabling Progress As the third site of co-production, representations are essential manifestations of knowledge deliberations. They provide surrogate markers for progress on value alignments or even responses to tensions between stakeholders. In our study, we saw several value levers that were deployed within organizations at different stages to tackle co-production challenges. These value levers enabled progress during knowledge deliberations (section section-3). For instance, during deliberation, practitioners used role-specific value levers (e.g., visual tools by UX researchers & designers) as a window into their thought process around specific RAI values. Similarly, enabling safe spaces provided opportunities of RAI value alignment among different roles. As representations, these value levers improved the transitions between internal stages and acted as vital markers to improve alignment. We call them internal representations . Prioritization Framework employed to resolve value related conflicts across individuals was another example of internal representation. In contrast, we also found a few externally represented value levers, such as RAI certifications, that enabled organizations to reduce ambivalence in their structures while showing their RAI readiness and compliance to the outside community. Interestingly, we uncovered limited evidence of external representations that engaged with end-user communities directly. We posit that the lack of external representations can be attributed to the deprioritized perception of the end-users’ role in RAI value conversations. External representations have the potential to act as stable participatory structures that enable participation of external stakeholders, making RAI value co-production successful. This might also be due to lack of sufficient incentives to enable such participatory structures. We hope that recent progress made by UNESCO’s historic agreementmight provide the much needed push for organizations to share and learn together.
Value Levers Enabling Practitioners As we end this discussion, some readers might assume that we now have strategies to manage the multiple challenges coming our way as we deliberate and implement RAI values in products. Our research with 23 practitioners, points to the opposite - we are far from it. As one practitioner said, “It’s currently hodgepodge”. Multiple individuals and organizations are trying to surmount this incredibly complex challenge at institutional and individual level. While value levers discussed in this work were successful in helping practitioners make progress, the discovery of these value levers has at best been serendipitous. Sufficient support structures, skills training, and knowledge dissemination will be needed to enable practitioners to overcome these and unknown challenges yet. One can liken these value levers as a tool-belt for practitioners, as summarized in Table tab-strategies.
There is a subsequent need to design systems that can provide access to these tools in a systematic way. This would require centering research amongst practitioners who are making products. We hope that future educators, researchers, and designers will pursue this opportunity to uncover further challenges, learn from existing strategies, develop better strategies as they iterate, and create design systems to support practitioners. Further, we need to find more tools, and we need to share the tool-belt with other practitioners.
Limitations & Conclusions
Based on qualitative research with 23 AI/ML practitioners, we discovered several challenges in RAI value co-production. Due to their roles and individual value system, practitioners were overburdened with upholding RAI values leading to inequitable workload. Further, we found that implementing RAI values on-the-ground is challenging as sometimes these values conflict within, and with those of team-members. Owing to the nascent stage of RAI values, current institutional structures are learning to adapt. Practitioners are also adapting by discovering strategies serendipitously to overcome the challenges. However, more support is needed by educators to educate RAI/RAI values, researchers to unpack further challenges/strategies, and for community to focus on aiding AI/ML practitioners as we collectively find a common-ground. Our work also has several limitations. First, our overall study is shaped by our prior HCI research experience in responsible AI. While all the participants had rich experience working with ethics in the context of social good in both global north and global south contexts, we acknowledge that our perspectives around responsible AI and ethical values in AI might be constrained. Second, our insights are also limited by the overall methodological limitations of qualitative enquiry, such as sample size of 23 participants across 10 organizations. Future work is required to generalize our insights across different organizational sectors and ML models using mixed-methods approach.
Bibliography
1@article{creswell2000determining,
2 publisher = {Taylor \& Francis},
3 year = {2000},
4 pages = {124--130},
5 number = {3},
6 volume = {39},
7 journal = {Theory into practice},
8 author = {Creswell, John W and Miller, Dana L},
9 title = {Determining validity in qualitative inquiry},
10}
11
12@article{morris1956varieties,
13 url = {https://doi.org/10.1037/10819-000},
14 doi = {10.1037/10819-000},
15 publisher = {University of Chicago Press},
16 year = {1956},
17 author = {Morris, Charles},
18 title = {Varieties of human value.},
19}
20
21@inproceedings{goyal2016effects,
22 year = {2016},
23 pages = {288--302},
24 booktitle = {Proceedings of the 19th ACM Conference on Computer-Supported Cooperative Work \& Social Computing},
25 author = {Goyal, Nitesh and Fussell, Susan R},
26 title = {Effects of sensemaking translucence on distributed collaborative analysis},
27}
28
29@inproceedings{Chouldechova2018,
30 url = {https://proceedings.mlr.press/v81/chouldechova18a.html},
31 pdf = {http://proceedings.mlr.press/v81/chouldechova18a/chouldechova18a.pdf},
32 publisher = {PMLR},
33 month = {23--24 Feb},
34 series = {Proceedings of Machine Learning Research},
35 volume = {81},
36 editor = {Friedler, Sorelle A. and Wilson, Christo},
37 year = {2018},
38 pages = {134--148},
39 booktitle = {Proceedings of the 1st Conference on Fairness, Accountability and Transparency},
40 author = {Chouldechova, Alexandra and Benavides-Prado, Diana and Fialko, Oleksandr and Vaithianathan, Rhema},
41 title = {A case study of algorithm-assisted decision making in child maltreatment hotline screening decisions},
42}
43
44@inproceedings{varanasi2022,
45 series = {CHI '22},
46 location = {New Orleans, LA, USA},
47 keywords = {crowdsourcing app, mobile crowdsourcing, crowd work, crowdsourcing work, HCI4D, Women},
48 numpages = {18},
49 articleno = {298},
50 booktitle = {Proceedings of the 2022 CHI Conference on Human Factors in Computing Systems},
51 doi = {10.1145/3491102.3501834},
52 url = {https://doi.org/10.1145/3491102.3501834},
53 address = {New York, NY, USA},
54 publisher = {Association for Computing Machinery},
55 isbn = {9781450391573},
56 year = {2022},
57 title = {Feeling Proud, Feeling Embarrassed: Experiences of Low-Income Women with Crowd Work},
58 author = {Varanasi, Rama Adithya and Siddarth, Divya and Seshadri, Vivek and Bali, Kalika and Vashistha, Aditya},
59}
60
61@book{mackenzie1999social,
62 publisher = {Open university press},
63 year = {1999},
64 author = {MacKenzie, Donald and Wajcman, Judy},
65 title = {The social shaping of technology},
66}
67
68@inbook{Friedman2013,
69 url = {https://doi.org/10.1007/978-94-007-7844-3_4},
70 doi = {10.1007/978-94-007-7844-3_4},
71 isbn = {978-94-007-7844-3},
72 pages = {55--95},
73 address = {Dordrecht},
74 publisher = {Springer Netherlands},
75 year = {2013},
76 booktitle = {Early engagement and new technologies: Opening up the laboratory},
77 title = {Value Sensitive Design and Information Systems},
78 editor = {Doorn, Neelke
79and Schuurbiers, Daan
80and van de Poel, Ibo
81and Gorman, Michael E.},
82 author = {Friedman, Batya
83and Kahn, Peter H.
84and Borning, Alan
85and Huldtgren, Alina},
86}
87
88@book{Gonzalez_2020,
89 month = {Nov},
90 year = {2020},
91 author = {González, Felipe and Ortiz, Teresa and Sánchez Ávalos, Roberto},
92 publisher = {Inter-American Development Bank},
93 doi = {10.18235/0002876},
94 url = {https://publications.iadb.org/es/node/2939},
95 title = {Responsible use of AI for public policy: Data science toolkit},
96}
97
98@article{latour1992missing,
99 publisher = {Mit Press Cambridge, MA},
100 year = {1992},
101 pages = {225--258},
102 volume = {1},
103 journal = {Shaping technology/building society: Studies in sociotechnical change},
104 author = {Latour, Bruno and others},
105 title = {Where are the missing masses? The sociology of a few mundane artifacts},
106}
107
108@book{batya97,
109 numpages = {20},
110 url = {https://dl.acm.org/doi/abs/10.5555/27821},
111 pages = {21–40},
112 publisher = {Cambridge University Press},
113 year = {1997},
114 number = {72},
115 author = {Friedman, Batya},
116 title = {Human values and the design of computer technology},
117}
118
119@misc{unesco2020agreement,
120 howpublished = {\url{https://unesdoc.unesco.org/ark:/48223/pf0000373434}},
121 year = {2020},
122 month = {September},
123 title = {Recommendation on the Ethics of Artificial Intelligence},
124 author = {UNESCO},
125}
126
127@misc{google2022,
128 howpublished = {\url{https://ai.google/responsibilities/responsible-ai-practices/}},
129 year = {2022},
130 title = {Responsible AI practices},
131 author = {Google},
132}
133
134@misc{Fb2021,
135 howpublished = {\url{https://ai.facebook.com/blog/facebooks-five-pillars-of-responsible-ai/ }},
136 year = {2021},
137 month = {June},
138 title = {Facebook’s five pillars of Responsible AI},
139 author = {Facebook},
140}
141
142@misc{IBM2022,
143 howpublished = {\url{https://www.ibm.com/artificial-intelligence/ethics}},
144 year = {2022},
145 month = {September},
146 title = {AI Ethics},
147 author = {IBM},
148}
149
150@misc{microsoft2022,
151 howpublished = {\url{https://query.prod.cms.rt.microsoft.com/cms/api/am/binary/RE4ZPmV}},
152 year = {2022},
153 title = {Microsoft Responsible AI Standard (version 2)},
154 author = {Microsoft},
155}
156
157@misc{dod2021,
158 howpublished = {\url{https://www.diu.mil/responsible-ai-guidelines}},
159 year = {2021},
160 month = {November},
161 title = {Responsible AI Initiative},
162 author = {Defence Innovation Unit},
163}
164
165@misc{eu2019,
166 howpublished = {\url{https://digital-strategy.ec.europa.eu/en/library/ethics-guidelines-trustworthy-ai}},
167 year = {2019},
168 month = {April},
169 title = {Ethics guidelines for trustworthy AI},
170 author = {European Union},
171}
172
173@misc{unesco2021,
174 howpublished = {\url{https://news.un.org/en/story/2021/11/1106612}},
175 year = {2021},
176 month = {November},
177 title = {193 countries adopt first-ever global agreement on the Ethics of Artificial Intelligence},
178 author = {UN},
179}
180
181@article{Friedman2021,
182 language = {en},
183 pages = {1–3},
184 year = {2021},
185 author = {Friedman, Batya and Harbers, Maaike and Hendry, David G. and van den Hoven, Jeroen and Jonker, Catholijn and Logler, Nick},
186 journal = {Ethics and Information Technology},
187 number = {1},
188 doi = {10.1007/s10676-021-09585-z},
189 url = {https://link.springer.com/10.1007/s10676-021-09585-z},
190 volume = {23},
191 title = {Introduction to the special issue: value sensitive design: charting the next decade},
192}
193
194@article{Hendry2021,
195 language = {en},
196 pages = {39–44},
197 year = {2021},
198 author = {Hendry, David G. and Friedman, Batya and Ballard, Stephanie},
199 journal = {Ethics and Information Technology},
200 number = {1},
201 doi = {10.1007/s10676-021-09579-x},
202 url = {https://link.springer.com/10.1007/s10676-021-09579-x},
203 volume = {23},
204 title = {Value sensitive design as a formative framework},
205}
206
207@article{flanagan2008embodying,
208 doi = {10.1017/CBO9780511498725.017},
209 url = {https://doi.org/10.1017/CBO9780511498725.017},
210 year = {2008},
211 pages = {24},
212 volume = {322},
213 journal = {Information technology and moral philosophy},
214 author = {Flanagan, Mary and Howe, Daniel C and Nissenbaum, Helen},
215 title = {Embodying values in technology: Theory and practice},
216}
217
218@article{Birhane_2021,
219 language = {en},
220 pages = {100205},
221 month = {Feb},
222 year = {2021},
223 author = {Birhane, Abeba},
224 journal = {Patterns},
225 number = {2},
226 doi = {10.1016/j.patter.2021.100205},
227 url = {https://linkinghub.elsevier.com/retrieve/pii/S2666389921000155},
228 issn = {26663899},
229 volume = {2},
230 title = {Algorithmic injustice: a relational ethics approach},
231}
232
233@article{hampton2021,
234 bibsource = {dblp computer science bibliography, https://dblp.org},
235 biburl = {https://dblp.org/rec/journals/corr/abs-2101-09869.bib},
236 timestamp = {Sat, 30 Jan 2021 18:02:51 +0100},
237 eprint = {2101.09869},
238 eprinttype = {arXiv},
239 url = {https://arxiv.org/abs/2101.09869},
240 year = {2021},
241 volume = {abs/2101.09869},
242 journal = {CoRR},
243 title = {Black Feminist Musings on Algorithmic Oppression},
244 author = {Lelia Marie Hampton},
245}
246
247@article{FriedlerSV16,
248 bibsource = {dblp computer science bibliography, https://dblp.org},
249 biburl = {https://dblp.org/rec/journals/corr/FriedlerSV16.bib},
250 timestamp = {Mon, 13 Aug 2018 16:47:25 +0200},
251 eprint = {1609.07236},
252 eprinttype = {arXiv},
253 url = {http://arxiv.org/abs/1609.07236},
254 year = {2016},
255 volume = {abs/1609.07236},
256 journal = {CoRR},
257 title = {On the (im)possibility of fairness},
258 author = {Sorelle A. Friedler and
259Carlos Scheidegger and
260Suresh Venkatasubramanian},
261}
262
263@inproceedings{birhane2022,
264 series = {FAccT ’22},
265 location = {Seoul, Republic of Korea},
266 keywords = {algorithmic systems, data-driven governance, accountability theory, algorithmic accountability},
267 booktitle = {Proceedings of the 2022 Conference on Fairness, Accountability, and Transparency},
268 doi = {10.1145/3531146.3533083},
269 url = {https://doi.org/10.1145/3531146.3533083},
270 address = {New York, NY, USA},
271 publisher = {Association for Computing Machinery},
272 year = {2022},
273 title = {The Values Encoded in Machine Learning Research},
274 author = {Abeba Birhane and
275Pratyusha Kalluri and
276Dallas Card and
277William Agnew and
278Ravit Dotan and
279Michelle Bao},
280}
281
282@inproceedings{sambasivan2022,
283 series = {CHI '22},
284 location = {New Orleans, LA, USA},
285 keywords = {data quality, ML, field workers, domain expertise, labour studies, data collection, deskilling, Taylorism},
286 numpages = {14},
287 articleno = {587},
288 booktitle = {Proceedings of the 2022 CHI Conference on Human Factors in Computing Systems},
289 doi = {10.1145/3491102.3517578},
290 url = {https://doi.org/10.1145/3491102.3517578},
291 address = {New York, NY, USA},
292 publisher = {Association for Computing Machinery},
293 isbn = {9781450391573},
294 year = {2022},
295 title = {The Deskilling of Domain Expertise in AI Development},
296 author = {Sambasivan, Nithya and Veeraraghavan, Rajesh},
297}
298
299@article{Floridi_2018,
300 language = {en},
301 pages = {689–707},
302 month = {Dec},
303 year = {2018},
304 author = {Floridi, Luciano and Cowls, Josh and Beltrametti, Monica and Chatila, Raja and Chazerand, Patrice and Dignum, Virginia and Luetge, Christoph and Madelin, Robert and Pagallo, Ugo and Rossi, Francesca and Schafer, Burkhard and Valcke, Peggy and Vayena, Effy},
305 journal = {Minds and Machines},
306 number = {4},
307 doi = {10.1007/s11023-018-9482-5},
308 url = {http://link.springer.com/10.1007/s11023-018-9482-5},
309 issn = {0924-6495, 1572-8641},
310 volume = {28},
311 title = {AI4People—An Ethical Framework for a Good AI Society: Opportunities, Risks, Principles, and Recommendations},
312}
313
314@article{Ghallab_2019,
315 language = {en},
316 pages = {3},
317 month = {Dec},
318 year = {2019},
319 author = {Ghallab, Malik},
320 journal = {AI Perspectives},
321 number = {1},
322 doi = {10.1186/s42467-019-0003-z},
323 url = {https://aiperspectives.springeropen.com/articles/10.1186/s42467-019-0003-z},
324 issn = {2523-398X},
325 volume = {1},
326 title = {Responsible AI: requirements and challenges},
327}
328
329@inproceedings{chaudhuri2009,
330 series = {ICML '09},
331 location = {Montreal, Quebec, Canada},
332 numpages = {8},
333 pages = {129–136},
334 booktitle = {Proceedings of the 26th Annual International Conference on Machine Learning},
335 doi = {10.1145/1553374.1553391},
336 url = {https://doi.org/10.1145/1553374.1553391},
337 address = {New York, NY, USA},
338 publisher = {Association for Computing Machinery},
339 isbn = {9781605585161},
340 year = {2009},
341 title = {Multi-View Clustering via Canonical Correlation Analysis},
342 author = {Chaudhuri, Kamalika and Kakade, Sham M. and Livescu, Karen and Sridharan, Karthik},
343}
344
345@inproceedings{espeholt2018,
346 url = {https://proceedings.mlr.press/v80/espeholt18a.html},
347 pdf = {http://proceedings.mlr.press/v80/espeholt18a/espeholt18a.pdf},
348 publisher = {PMLR},
349 month = {10--15 Jul},
350 series = {Proceedings of Machine Learning Research},
351 volume = {80},
352 editor = {Dy, Jennifer and Krause, Andreas},
353 year = {2018},
354 pages = {1407--1416},
355 booktitle = {Proceedings of the 35th International Conference on Machine Learning},
356 author = {Espeholt, Lasse and Soyer, Hubert and Munos, Remi and Simonyan, Karen and Mnih, Vlad and Ward, Tom and Doron, Yotam and Firoiu, Vlad and Harley, Tim and Dunning, Iain and Legg, Shane and Kavukcuoglu, Koray},
357 title = {{IMPALA}: Scalable Distributed Deep-{RL} with Importance Weighted Actor-Learner Architectures},
358}
359
360@article{jain2008online,
361 year = {2008},
362 volume = {21},
363 journal = {Advances in neural information processing systems},
364 author = {Jain, Prateek and Kulis, Brian and Dhillon, Inderjit and Grauman, Kristen},
365 title = {Online metric learning and fast similarity search},
366}
367
368@inproceedings{Azra2021,
369 series = {CHI '21},
370 location = {Yokohama, Japan},
371 keywords = {Social Good, AI, India, Qualitative, HCI4D, Healthcare},
372 numpages = {21},
373 articleno = {598},
374 booktitle = {Proceedings of the 2021 CHI Conference on Human Factors in Computing Systems},
375 doi = {10.1145/3411764.3445130},
376 url = {https://doi.org/10.1145/3411764.3445130},
377 address = {New York, NY, USA},
378 publisher = {Association for Computing Machinery},
379 isbn = {9781450380966},
380 year = {2021},
381 title = {AI in Global Health: The View from the Front Lines},
382 author = {Ismail, Azra and Kumar, Neha},
383}
384
385@inproceedings{chinasa2021,
386 series = {CHI '21},
387 location = {Yokohama, Japan},
388 keywords = {HCI4D, mHealth, Community health worker, ICTD, CHW, Artificial Intelligence, AI},
389 numpages = {20},
390 articleno = {701},
391 booktitle = {Proceedings of the 2021 CHI Conference on Human Factors in Computing Systems},
392 doi = {10.1145/3411764.3445420},
393 url = {https://doi.org/10.1145/3411764.3445420},
394 address = {New York, NY, USA},
395 publisher = {Association for Computing Machinery},
396 isbn = {9781450380966},
397 year = {2021},
398 title = {“It Cannot Do All of My Work”: Community Health Worker Perceptions of AI-Enabled Mobile Health Applications in Rural India},
399 author = {Okolo, Chinasa T. and Kamath, Srujana and Dell, Nicola and Vashistha, Aditya},
400}
401
402@inproceedings{hooker2018,
403 series = {AIES '18},
404 location = {New Orleans, LA, USA},
405 keywords = {accountable ai, deontology, kantian ai, explainable ai, artificial intelligence ethics, machine ethics, autonomous machine ethics, modal logic},
406 numpages = {7},
407 pages = {130–136},
408 booktitle = {Proceedings of the 2018 AAAI/ACM Conference on AI, Ethics, and Society},
409 doi = {10.1145/3278721.3278753},
410 url = {https://doi.org/10.1145/3278721.3278753},
411 address = {New York, NY, USA},
412 publisher = {Association for Computing Machinery},
413 isbn = {9781450360128},
414 year = {2018},
415 title = {Toward Non-Intuition-Based Machine and Artificial Intelligence Ethics: A Deontological Approach Based on Modal Logic},
416 author = {Hooker, John N. and Kim, Tae Wan N.},
417}
418
419@article{Haubermann_Lutge_2022,
420 language = {en},
421 pages = {341–362},
422 year = {2022},
423 author = {Häußermann, Johann Jakob and Lütge, Christoph},
424 journal = {AI and Ethics},
425 number = {2},
426 doi = {10.1007/s43681-021-00047-2},
427 url = {https://link.springer.com/10.1007/s43681-021-00047-2},
428 volume = {2},
429 title = {Community-in-the-loop: towards pluralistic value creation in AI, or—why AI needs business ethics},
430}
431
432@inproceedings{jakesh2022how,
433 series = {FAccT '22},
434 location = {Seoul, Republic of Korea},
435 keywords = {value-sensitive design, empirical ethics, Responsible AI},
436 numpages = {14},
437 pages = {310–323},
438 booktitle = {2022 ACM Conference on Fairness, Accountability, and Transparency},
439 doi = {10.1145/3531146.3533097},
440 url = {https://doi.org/10.1145/3531146.3533097},
441 address = {New York, NY, USA},
442 publisher = {Association for Computing Machinery},
443 isbn = {9781450393522},
444 year = {2022},
445 title = {How Different Groups Prioritize Ethical Values for Responsible AI},
446 author = {Jakesch, Maurice and Bu\c{c}inca, Zana and Amershi, Saleema and Olteanu, Alexandra},
447}
448
449@misc{kizilcec2020,
450 copyright = {arXiv.org perpetual, non-exclusive license},
451 year = {2020},
452 publisher = {arXiv},
453 title = {Algorithmic Fairness in Education},
454 keywords = {Computers and Society (cs.CY), Artificial Intelligence (cs.AI), Machine Learning (cs.LG), FOS: Computer and information sciences, FOS: Computer and information sciences},
455 author = {Kizilcec, René F. and Lee, Hansol},
456 url = {https://arxiv.org/abs/2007.05443},
457 doi = {10.48550/ARXIV.2007.05443},
458}
459
460@inbook{smith2020,
461 numpages = {14},
462 pages = {1–14},
463 booktitle = {Proceedings of the 2020 CHI Conference on Human Factors in Computing Systems},
464 url = {https://doi.org/10.1145/3313831.3376783},
465 address = {New York, NY, USA},
466 publisher = {Association for Computing Machinery},
467 isbn = {9781450367080},
468 year = {2020},
469 title = {Keeping Community in the Loop: Understanding Wikipedia Stakeholder Values for Machine Learning-Based Systems},
470 author = {Smith, C. Estelle and Yu, Bowen and Srivastava, Anjali and Halfaker, Aaron and Terveen, Loren and Zhu, Haiyi},
471}
472
473@inproceedings{robertson2021,
474 series = {CHI '21},
475 location = {Yokohama, Japan},
476 keywords = {mechanism design, value sensitive design, student assignment},
477 numpages = {14},
478 articleno = {589},
479 booktitle = {Proceedings of the 2021 CHI Conference on Human Factors in Computing Systems},
480 doi = {10.1145/3411764.3445748},
481 url = {https://doi.org/10.1145/3411764.3445748},
482 address = {New York, NY, USA},
483 publisher = {Association for Computing Machinery},
484 isbn = {9781450380966},
485 year = {2021},
486 title = {Modeling Assumptions Clash with the Real World: Transparency, Equity, and Community Challenges for Student Assignment Algorithms},
487 author = {Robertson, Samantha and Nguyen, Tonya and Salehi, Niloufar},
488}
489
490@inproceedings{shen2022The,
491 series = {FAccT '22},
492 location = {Seoul, Republic of Korea},
493 numpages = {12},
494 pages = {440–451},
495 doi = {10.1145/3531146.3533110},
496 url = {https://doi.org/10.1145/3531146.3533110},
497 address = {New York, NY, USA},
498 publisher = {Association for Computing Machinery},
499 isbn = {9781450393522},
500 year = {2022},
501 title = {The Model Card Authoring Toolkit: Toward Community-Centered, Deliberation-Driven AI Design},
502 author = {Shen, Hong and Wang, Leijie and Deng, Wesley H. and Brusse, Ciell and Velgersdijk, Ronald and Zhu, Haiyi},
503}
504
505@article{Hagendorff_2020,
506 language = {en},
507 pages = {99–120},
508 month = {Mar},
509 year = {2020},
510 author = {Hagendorff, Thilo},
511 doi = {10.1007/s11023-020-09517-8},
512 url = {http://link.springer.com/10.1007/s11023-020-09517-8},
513 issn = {0924-6495, 1572-8641},
514 volume = {30},
515 title = {The Ethics of AI Ethics: An Evaluation of Guidelines},
516}
517
518@misc{peter2019,
519 copyright = {Creative Commons Attribution 4.0 International},
520 year = {2019},
521 publisher = {arXiv},
522 title = {Impossibility and Uncertainty Theorems in AI Value Alignment (or why your AGI should not have a utility function)},
523 keywords = {Artificial Intelligence (cs.AI), FOS: Computer and information sciences, FOS: Computer and information sciences},
524 author = {Eckersley, Peter},
525 url = {https://arxiv.org/abs/1901.00064},
526 doi = {10.48550/ARXIV.1901.00064},
527}
528
529@article{Robbins2019,
530 language = {en},
531 pages = {719–735},
532 month = {Jun},
533 year = {2019},
534 author = {van Wynsberghe, Aimee and Robbins, Scott},
535 journal = {Science and Engineering Ethics},
536 number = {3},
537 doi = {10.1007/s11948-018-0030-8},
538 url = {http://link.springer.com/10.1007/s11948-018-0030-8},
539 issn = {1353-3452, 1471-5546},
540 volume = {25},
541 title = {Critiquing the Reasons for Making Artificial Moral Agents},
542}
543
544@book{Dignum_2019,
545 language = {eng},
546 collection = {Artificial Intelligence foundations, theory, and algorithms},
547 year = {2019},
548 author = {Dignum, Virginia},
549 publisher = {Springer},
550 isbn = {9783030303716},
551 title = {Responsible artificial intelligence: how to develop and use AI in a responsible way},
552 series = {Artificial Intelligence foundations, theory, and algorithms},
553 address = {Cham},
554}
555
556@inproceedings{barry2017,
557 series = {CHI '17},
558 location = {Denver, Colorado, USA},
559 keywords = {mHealth, virtue ethics, psychological wellbeing, human flourishing, maternal mental health, phronesis, ethical design},
560 numpages = {49},
561 pages = {2708–2756},
562 booktitle = {Proceedings of the 2017 CHI Conference on Human Factors in Computing Systems},
563 doi = {10.1145/3025453.3025918},
564 url = {https://doi.org/10.1145/3025453.3025918},
565 address = {New York, NY, USA},
566 publisher = {Association for Computing Machinery},
567 isbn = {9781450346559},
568 year = {2017},
569 title = {MHealth for Maternal Mental Health: Everyday Wisdom in Ethical Design},
570 author = {Barry, Marguerite and Doherty, Kevin and Marcano Belisario, Jose and Car, Josip and Morrison, Cecily and Doherty, Gavin},
571}
572
573@article{wong2021,
574 keywords = {values work, ethics, values, soft resistance, ethics work, work practice, UX practice, values in design, UX professionals},
575 numpages = {28},
576 articleno = {355},
577 month = {oct},
578 journal = {Proc. ACM Hum.-Comput. Interact.},
579 doi = {10.1145/3479499},
580 url = {https://doi.org/10.1145/3479499},
581 number = {CSCW2},
582 volume = {5},
583 address = {New York, NY, USA},
584 publisher = {Association for Computing Machinery},
585 issue_date = {October 2021},
586 year = {2021},
587 title = {Tactics of Soft Resistance in User Experience Professionals' Values Work},
588 author = {Wong, Richmond Y.},
589}
590
591@inbook{Aleven_2019,
592 language = {en},
593 pages = {157–171},
594 year = {2019},
595 editor = {Isotani, Seiji and Millán, Eva and Ogan, Amy and Hastings, Peter and McLaren, Bruce and Luckin, Rose},
596 author = {Holstein, Kenneth and McLaren, Bruce M. and Aleven, Vincent},
597 publisher = {Springer International Publishing},
598 booktitle = {Artificial Intelligence in Education},
599 doi = {10.1007/978-3-030-23204-7_14},
600 url = {http://link.springer.com/10.1007/978-3-030-23204-7_14},
601 isbn = {9783030232030},
602 volume = {11625},
603 title = {Designing for Complementarity: Teacher and Student Needs for Orchestration Support in AI-Enhanced Classrooms},
604 address = {Cham},
605}
606
607@article{asken2019therole,
608 bibsource = {dblp computer science bibliography, https://dblp.org},
609 biburl = {https://dblp.org/rec/journals/corr/abs-1907-04534.bib},
610 timestamp = {Wed, 17 Jul 2019 10:27:36 +0200},
611 eprint = {1907.04534},
612 eprinttype = {arXiv},
613 url = {http://arxiv.org/abs/1907.04534},
614 year = {2019},
615 volume = {abs/1907.04534},
616 journal = {CoRR},
617 title = {The Role of Cooperation in Responsible {AI} Development},
618 author = {Amanda Askell and
619Miles Brundage and
620Gillian K. Hadfield},
621}
622
623@article{Awad_2018,
624 language = {en},
625 pages = {59–64},
626 month = {Nov},
627 year = {2018},
628 author = {Awad, Edmond and Dsouza, Sohan and Kim, Richard and Schulz, Jonathan and Henrich, Joseph and Shariff, Azim and Bonnefon, Jean-François and Rahwan, Iyad},
629 journal = {Nature},
630 number = {7729},
631 doi = {10.1038/s41586-018-0637-6},
632 url = {http://www.nature.com/articles/s41586-018-0637-6},
633 issn = {0028-0836, 1476-4687},
634 volume = {563},
635 title = {The Moral Machine experiment},
636}
637
638@inbook{khan1995,
639 numpages = {13},
640 pages = {253–265},
641 booktitle = {Android Epistemology},
642 address = {Cambridge, MA, USA},
643 publisher = {MIT Press},
644 isbn = {0262061848},
645 year = {1995},
646 title = {The Ethics of Autonomous Learning Systems},
647 author = {Khan, A. F. Umar},
648}
649
650@article{Dignum_2021,
651 language = {en},
652 month = {Jan},
653 year = {2021},
654 author = {Dignum, Virginia},
655 journal = {London Review of Education},
656 number = {1},
657 doi = {10.14324/LRE.19.1.01},
658 url = {https://scienceopen.com/hosted-document?doi=10.14324/LRE.19.1.01},
659 issn = {1474-8479},
660 volume = {19},
661 title = {The role and challenges of education for responsible AI},
662}
663
664@incollection{Gips1994,
665 publisher = {MIT Press},
666 year = {1994},
667 author = {James Gips},
668 title = {Toward the Ethical Robot},
669 editor = {Kenneth M. Ford and C. Glymour and Patrick Hayes},
670 booktitle = {Android Epistemology},
671 pages = {243--252},
672}
673
674@book{abrassart_2017,
675 year = {2017},
676 author = {Abrassart, Christophe and Bengio, Yoshua and Chicoisne, Guillaume and De Marcellis-warin, Nathalie and Dilhac, Marc-Antoine and Gamb, Sebastien and Gautrais, Vincent and Giber, Martin And Langlois, Lyse And Laviolett, François},
677 institution = {Université de Montréal},
678 journal = {Montréal declaration for responsible development of artifcial intelligence},
679 url = {https://www.montrealdeclaration-responsibleai.com/_files/ugd/ebc3a3_506ea08298cd4f8196635545a16b071d.pdf},
680}
681
682@misc{ostrom:2009,
683 year = {2009,
684howpublished={\url{https://www.nobelprize.org/prizes/economic-sciences/2009/ostrom/lecture/}}},
685 title = {Elinor Ostrom Prize Lecture},
686 author = {Ostrom Elinor},
687}
688
689@inproceedings{wang2021,
690 series = {CHI '21},
691 location = {Yokohama, Japan},
692 keywords = {Human AI Interaction, Workflow, CDSS, Healthcare, Rural Clinic, Developing Country, Decision Making, Human AI Collaboration, AI, Clinical Decision Making, Future of Work, Trust AI, Collaborative AI, China, Implementation, AI Deployment},
693 numpages = {18},
694 articleno = {697},
695 doi = {10.1145/3411764.3445432},
696 url = {https://doi.org/10.1145/3411764.3445432},
697 address = {New York, NY, USA},
698 publisher = {Association for Computing Machinery},
699 isbn = {9781450380966},
700 year = {2021},
701 title = {“Brilliant AI Doctor” in Rural Clinics: Challenges in AI-Powered Clinical Decision Support System Deployment},
702 author = {Wang, Dakuo and Wang, Liuping and Zhang, Zhan and Wang, Ding and Zhu, Haiyi and Gao, Yvonne and Fan, Xiangmin and Tian, Feng},
703}
704
705@article{Barredo_2020,
706 language = {en},
707 pages = {82–115},
708 month = {Jun},
709 year = {2020},
710 author = {Barredo Arrieta, Alejandro and Díaz-Rodríguez, Natalia and Del Ser, Javier and Bennetot, Adrien and Tabik, Siham and Barbado, Alberto and Garcia, Salvador and Gil-Lopez, Sergio and Molina, Daniel and Benjamins, Richard and Chatila, Raja and Herrera, Francisco},
711 journal = {Information Fusion},
712 doi = {10.1016/j.inffus.2019.12.012},
713 url = {https://linkinghub.elsevier.com/retrieve/pii/S1566253519308103},
714 issn = {15662535},
715 volume = {58},
716 title = {Explainable Artificial Intelligence (XAI): Concepts, taxonomies, opportunities and challenges toward responsible AI},
717}
718
719@article{moore2019ai,
720 publisher = {Frontiers Media SA},
721 year = {2019},
722 pages = {32},
723 url = {https://www.frontiersin.org/articles/10.3389/fdata.2019.00032/full},
724 volume = {2},
725 journal = {Frontiers in Big Data},
726 author = {Moore, Jared},
727 title = {AI for not bad},
728}
729
730@article{Shin_Park_2019,
731 language = {en},
732 pages = {277–284},
733 month = {Sep},
734 year = {2019},
735 author = {Shin, Donghee and Park, Yong Jin},
736 journal = {Computers in Human Behavior},
737 doi = {10.1016/j.chb.2019.04.019},
738 url = {https://linkinghub.elsevier.com/retrieve/pii/S0747563219301591},
739 issn = {07475632},
740 volume = {98},
741 title = {Role of fairness, accountability, and transparency in algorithmic affordance},
742}
743
744@article{kroll165accountable,
745 pages = {633},
746 volume = {165},
747 journal = {University of Pennsylvania Law Review},
748 author = {Kroll, Joshua A and Huey, Joanna and Barocas, Solon and Felten, Edward W and Reidenberg, Joel R and Robinson, David G and Yu, Harlan},
749 title = {Accountable algorithms’(2017)},
750}
751
752@article{Licht_2020,
753 language = {en},
754 pages = {917–926},
755 month = {Dec},
756 year = {2020},
757 author = {de Fine Licht, Karl and de Fine Licht, Jenny},
758 journal = {AI \& SOCIETY},
759 number = {4},
760 doi = {10.1007/s00146-020-00960-w},
761 url = {http://link.springer.com/10.1007/s00146-020-00960-w},
762 issn = {0951-5666, 1435-5655},
763 volume = {35},
764 title = {Artificial intelligence, transparency, and public decision-making: Why explanations are key when trying to produce perceived legitimacy},
765}
766
767@inproceedings{green2019good,
768 year = {2019},
769 volume = {17},
770 booktitle = {Proceedings of the AI for Social Good workshop at NeurIPS},
771 url = {https://aiforsocialgood.github.io/neurips2019/accepted/track3/pdfs/67_aisg_neurips2019.pdf},
772 author = {Green, Ben},
773 title = {Good” isn’t good enough},
774}
775
776@inproceedings{ashurst2022,
777 series = {FAccT '22},
778 location = {Seoul, Republic of Korea},
779 numpages = {12},
780 pages = {2057–2068},
781 booktitle = {2022 ACM Conference on Fairness, Accountability, and Transparency},
782 doi = {10.1145/3531146.3533781},
783 url = {https://doi.org/10.1145/3531146.3533781},
784 address = {New York, NY, USA},
785 publisher = {Association for Computing Machinery},
786 isbn = {9781450393522},
787 year = {2022},
788 title = {Disentangling the Components of Ethical Research in Machine Learning},
789 author = {Ashurst, Carolyn and Barocas, Solon and Campbell, Rosie and Raji, Deborah},
790}
791
792@article{Mitchell_2021,
793 language = {en},
794 pages = {141–163},
795 month = {Mar},
796 year = {2021},
797 author = {Mitchell, Shira and Potash, Eric and Barocas, Solon and D’Amour, Alexander and Lum, Kristian},
798 journal = {Annual Review of Statistics and Its Application},
799 number = {1},
800 doi = {10.1146/annurev-statistics-042720-125902},
801 url = {https://www.annualreviews.org/doi/10.1146/annurev-statistics-042720-125902},
802 issn = {2326-8298, 2326-831X},
803 volume = {8},
804 title = {Algorithmic Fairness: Choices, Assumptions, and Definitions},
805}
806
807@inproceedings{bondi2021,
808 series = {AIES '21},
809 location = {Virtual Event, USA},
810 keywords = {artificial intelligence for social good, participatory design, capabilities approach},
811 numpages = {12},
812 pages = {425–436},
813 booktitle = {Proceedings of the 2021 AAAI/ACM Conference on AI, Ethics, and Society},
814 doi = {10.1145/3461702.3462612},
815 url = {https://doi.org/10.1145/3461702.3462612},
816 address = {New York, NY, USA},
817 publisher = {Association for Computing Machinery},
818 isbn = {9781450384735},
819 year = {2021},
820 title = {Envisioning Communities: A Participatory Approach Towards AI for Social Good},
821 author = {Bondi, Elizabeth and Xu, Lily and Acosta-Navas, Diana and Killian, Jackson A.},
822}
823
824@inproceedings{madaan2019,
825 series = {COMPASS '19},
826 location = {Accra, Ghana},
827 keywords = {commodities, anomaly, time series, analysis, agriculture, prices},
828 numpages = {13},
829 pages = {52–64},
830 doi = {10.1145/3314344.3332488},
831 url = {https://doi.org/10.1145/3314344.3332488},
832 address = {New York, NY, USA},
833 publisher = {Association for Computing Machinery},
834 isbn = {9781450367141},
835 year = {2019},
836 title = {Price Forecasting \& Anomaly Detection for Agricultural Commodities in India},
837 author = {Madaan, Lovish and Sharma, Ankur and Khandelwal, Praneet and Goel, Shivank and Singla, Parag and Seth, Aaditeshwar},
838}
839
840@inproceedings{cannanure2020,
841 series = {ICTD2020},
842 location = {Guayaquil, Ecuador},
843 numpages = {5},
844 articleno = {24},
845 doi = {10.1145/3392561.3397577},
846 url = {https://doi.org/10.1145/3392561.3397577},
847 address = {New York, NY, USA},
848 publisher = {Association for Computing Machinery},
849 isbn = {9781450387620},
850 year = {2020},
851 title = {DIA: A Human AI Hybrid Conversational Assistant for Developing Contexts},
852 author = {Cannanure, Vikram Kamath and Brown, Timothy X and Ogan, Amy},
853}
854
855@article{Berendt_2019,
856 language = {en},
857 pages = {44–65},
858 month = {Jan},
859 year = {2019},
860 author = {Berendt, Bettina},
861 journal = {Paladyn, Journal of Behavioral Robotics},
862 number = {1},
863 doi = {10.1515/pjbr-2019-0004},
864 url = {https://www.degruyter.com/document/doi/10.1515/pjbr-2019-0004/html},
865 issn = {2081-4836},
866 volume = {10},
867 title = {AI for the Common Good?! Pitfalls, challenges, and ethics pen-testing},
868}
869
870@inproceedings{wang2022,
871 series = {CHI '22},
872 location = {New Orleans, LA, USA},
873 keywords = {future of work, AI labour, data annotation, qualitative study},
874 numpages = {16},
875 articleno = {582},
876 booktitle = {Proceedings of the 2022 CHI Conference on Human Factors in Computing Systems},
877 doi = {10.1145/3491102.3502121},
878 url = {https://doi.org/10.1145/3491102.3502121},
879 address = {New York, NY, USA},
880 publisher = {Association for Computing Machinery},
881 isbn = {9781450391573},
882 year = {2022},
883 title = {Whose AI Dream? In Search of the Aspiration in Data Annotation.},
884 author = {Wang, Ding and Prabhat, Shantanu and Sambasivan, Nithya},
885}
886
887@article{ryan2020,
888 bibsource = {dblp computer science bibliography, https://dblp.org},
889 biburl = {https://dblp.org/rec/journals/corr/abs-2001-01818.bib},
890 timestamp = {Fri, 10 Jan 2020 13:10:19 +0100},
891 eprint = {2001.01818},
892 eprinttype = {arXiv},
893 url = {http://arxiv.org/abs/2001.01818},
894 year = {2020},
895 volume = {abs/2001.01818},
896 journal = {CoRR},
897 title = {Artificial Intelligence for Social Good: {A} Survey},
898 author = {Zheyuan Ryan Shi and
899Claire Wang and
900Fei Fang},
901}
902
903@article{Mittelstadt_2019,
904 language = {en},
905 pages = {501–507},
906 month = {Nov},
907 year = {2019},
908 author = {Mittelstadt, Brent},
909 journal = {Nature Machine Intelligence},
910 number = {11},
911 doi = {10.1038/s42256-019-0114-4},
912 url = {http://www.nature.com/articles/s42256-019-0114-4},
913 issn = {2522-5839},
914 volume = {1},
915 title = {Principles alone cannot guarantee ethical AI},
916}
917
918@misc{yurrita2022,
919 copyright = {Creative Commons Attribution 4.0 International},
920 year = {2022},
921 publisher = {arXiv},
922 title = {Towards a multi-stakeholder value-based assessment framework for algorithmic systems},
923 keywords = {Machine Learning (cs.LG), Human-Computer Interaction (cs.HC), FOS: Computer and information sciences, FOS: Computer and information sciences},
924 author = {Yurrita, Mireia and Murray-Rust, Dave and Balayn, Agathe and Bozzon, Alessandro},
925 url = {https://arxiv.org/abs/2205.04525},
926 doi = {10.48550/ARXIV.2205.04525},
927}
928
929@article{burrell2016,
930 eprint = { https://doi.org/10.1177/2053951715622512},
931 url = {
932https://doi.org/10.1177/2053951715622512
933},
934 doi = {10.1177/2053951715622512},
935 year = {2016},
936 pages = {2053951715622512},
937 number = {1},
938 volume = {3},
939 journal = {Big Data \& Society},
940 title = {How the machine ‘thinks’: Understanding opacity in machine learning algorithms},
941 author = {Jenna Burrell},
942}
943
944@inproceedings{dantec2009,
945 series = {CHI '09},
946 location = {Boston, MA, USA},
947 keywords = {fieldwork, values, empirical methods, methodology, value sensitive design, photo elicitation},
948 numpages = {10},
949 pages = {1141–1150},
950 booktitle = {Proceedings of the SIGCHI Conference on Human Factors in Computing Systems},
951 doi = {10.1145/1518701.1518875},
952 url = {https://doi.org/10.1145/1518701.1518875},
953 address = {New York, NY, USA},
954 publisher = {Association for Computing Machinery},
955 isbn = {9781605582467},
956 year = {2009},
957 title = {Values as Lived Experience: Evolving Value Sensitive Design in Support of Value Discovery},
958 author = {Le Dantec, Christopher A. and Poole, Erika Shehan and Wyche, Susan P.},
959}
960
961@article{Jafari2015a,
962 pages = {91–104},
963 year = {2015},
964 author = {JafariNaimi, Nassim and Nathan, Lisa and Hargraves, Ian},
965 journal = {Design Issues},
966 number = {4},
967 url = {http://www.jstor.org/stable/43830434},
968 issn = {07479360, 15314790},
969 volume = {31},
970 title = {Values as Hypotheses: Design, Inquiry, and the Service of Values},
971}
972
973@article{Star_1999,
974 language = {en},
975 pages = {377–391},
976 year = {1999},
977 author = {Star, Susan Leigh},
978 journal = {American Behavioral Scientist},
979 number = {3},
980 doi = {10.1177/00027649921955326},
981 url = {http://journals.sagepub.com/doi/10.1177/00027649921955326},
982 volume = {43},
983 title = {The Ethnography of Infrastructure},
984}
985
986@inproceedings{houston2016,
987 series = {CHI '16},
988 location = {San Jose, California, USA},
989 keywords = {maintenance, repair, ethnography, values, design},
990 numpages = {12},
991 pages = {1403–1414},
992 booktitle = {Proceedings of the 2016 CHI Conference on Human Factors in Computing Systems},
993 doi = {10.1145/2858036.2858470},
994 url = {https://doi.org/10.1145/2858036.2858470},
995 address = {New York, NY, USA},
996 publisher = {Association for Computing Machinery},
997 isbn = {9781450333627},
998 year = {2016},
999 title = {Values in Repair},
1000 author = {Houston, Lara and Jackson, Steven J. and Rosner, Daniela K. and Ahmed, Syed Ishtiaque and Young, Meg and Kang, Laewoo},
1001}
1002
1003@misc{delgado2021,
1004 copyright = {Creative Commons Attribution 4.0 International},
1005 year = {2021},
1006 publisher = {arXiv},
1007 title = {Stakeholder Participation in AI: Beyond "Add Diverse Stakeholders and Stir"},
1008 keywords = {Artificial Intelligence (cs.AI), Computers and Society (cs.CY), Human-Computer Interaction (cs.HC), FOS: Computer and information sciences, FOS: Computer and information sciences},
1009 author = {Delgado, Fernando and Yang, Stephen and Madaio, Michael and Yang, Qian},
1010 url = {https://arxiv.org/abs/2111.01122},
1011 doi = {10.48550/ARXIV.2111.01122},
1012}
1013
1014@inproceedings{thakkar2022,
1015 series = {CHI '22},
1016 location = {New Orleans, LA, USA},
1017 keywords = {Data work, valuation, public health, India},
1018 numpages = {16},
1019 articleno = {322},
1020 booktitle = {Proceedings of the 2022 CHI Conference on Human Factors in Computing Systems},
1021 doi = {10.1145/3491102.3501868},
1022 url = {https://doi.org/10.1145/3491102.3501868},
1023 address = {New York, NY, USA},
1024 publisher = {Association for Computing Machinery},
1025 isbn = {9781450391573},
1026 year = {2022},
1027 title = {When is Machine Learning Data Good?: Valuing in Public Health Datafication},
1028 author = {Thakkar, Divy and Ismail, Azra and Kumar, Pratyush and Hanna, Alex and Sambasivan, Nithya and Kumar, Neha},
1029}
1030
1031@inproceedings{Vakkuri_Abrahamsson_2018,
1032 pages = {1–6},
1033 month = {Jun},
1034 year = {2018},
1035 author = {Vakkuri, Ville and Abrahamsson, Pekka},
1036 publisher = {IEEE},
1037 booktitle = {2018 IEEE International Conference on Engineering,
1038Technology and Innovation (ICE/ITMC)},
1039 doi = {10.1109/ICE.2018.8436265},
1040 url = {https://ieeexplore.ieee.org/document/8436265/},
1041 isbn = {9781538614693},
1042 title = {The Key Concepts of Ethics of Artificial Intelligence},
1043 address = {Stuttgart},
1044}
1045
1046@article{Timmermans_Tavory_2012a,
1047 language = {en},
1048 pages = {167–186},
1049 month = {Sep},
1050 year = {2012},
1051 author = {Timmermans, Stefan and Tavory, Iddo},
1052 journal = {Sociological Theory},
1053 number = {3},
1054 doi = {10.1177/0735275112457914},
1055 url = {http://journals.sagepub.com/doi/10.1177/0735275112457914},
1056 issn = {0735-2751, 1467-9558},
1057 volume = {30},
1058 title = {Theory Construction in Qualitative Research: From Grounded Theory to Abductive Analysis},
1059}
1060
1061@article{Harris_Anthis_2021a,
1062 language = {en},
1063 pages = {53},
1064 month = {Aug},
1065 year = {2021},
1066 author = {Harris, Jamie and Anthis, Jacy Reese},
1067 journal = {Science and Engineering Ethics},
1068 number = {4},
1069 doi = {10.1007/s11948-021-00331-8},
1070 url = {https://link.springer.com/10.1007/s11948-021-00331-8},
1071 issn = {1353-3452, 1471-5546},
1072 volume = {27},
1073 title = {The Moral Consideration of Artificial Entities: A Literature Review},
1074}
1075
1076@article{Kalluri_2020,
1077 language = {en},
1078 pages = {169–169},
1079 month = {Jul},
1080 year = {2020},
1081 author = {Kalluri, Pratyusha},
1082 journal = {Nature},
1083 number = {7815},
1084 doi = {10.1038/d41586-020-02003-2},
1085 url = {http://www.nature.com/articles/d41586-020-02003-2},
1086 issn = {0028-0836, 1476-4687},
1087 volume = {583},
1088 title = {Don’t ask if artificial intelligence is good or fair, ask how it shifts power},
1089}
1090
1091@article{Mohamed2020,
1092 language = {en},
1093 pages = {659–684},
1094 month = {Dec},
1095 year = {2020},
1096 author = {Mohamed, Shakir and Png, Marie-Therese and Isaac, William},
1097 journal = {Philosophy \& Technology},
1098 number = {4},
1099 doi = {10.1007/s13347-020-00405-8},
1100 url = {https://link.springer.com/10.1007/s13347-020-00405-8},
1101 issn = {2210-5433, 2210-5441},
1102 volume = {33},
1103 title = {Decolonial AI: Decolonial Theory as Sociotechnical Foresight in Artificial Intelligence},
1104}
1105
1106@article{Jobin_Ienca_Vayena_2019,
1107 language = {en},
1108 pages = {389–399},
1109 year = {2019},
1110 author = {Jobin, Anna and Ienca, Marcello and Vayena, Effy},
1111 journal = {Nature Machine Intelligence},
1112 number = {9},
1113 doi = {10.1038/s42256-019-0088-2},
1114 url = {http://www.nature.com/articles/s42256-019-0088-2},
1115 volume = {1},
1116 title = {The global landscape of AI ethics guidelines},
1117}
1118
1119@misc{robertson2020,
1120 copyright = {arXiv.org perpetual, non-exclusive license},
1121 year = {2020},
1122 publisher = {arXiv},
1123 title = {What If I Don't Like Any Of The Choices? The Limits of Preference Elicitation for Participatory Algorithm Design},
1124 keywords = {Computers and Society (cs.CY), Human-Computer Interaction (cs.HC), Machine Learning (cs.LG), FOS: Computer and information sciences, FOS: Computer and information sciences},
1125 author = {Robertson, Samantha and Salehi, Niloufar},
1126 url = {https://arxiv.org/abs/2007.06718},
1127 doi = {10.48550/ARXIV.2007.06718},
1128}
1129
1130@inbook{madaio2020codesign,
1131 numpages = {14},
1132 pages = {1–14},
1133 booktitle = {Proceedings of the 2020 CHI Conference on Human Factors in Computing Systems},
1134 url = {https://doi.org/10.1145/3313831.3376445},
1135 address = {New York, NY, USA},
1136 publisher = {Association for Computing Machinery},
1137 isbn = {9781450367080},
1138 year = {2020},
1139 title = {Co-Designing Checklists to Understand Organizational Challenges and Opportunities around Fairness in AI},
1140 author = {Madaio, Michael A. and Stark, Luke and Wortman Vaughan, Jennifer and Wallach, Hanna},
1141}
1142
1143@article{Sheuerman2020how,
1144 keywords = {race and ethnicity, facial analysis, facial recognition, training and evaluation data, classification, gender, facial classification, computer vision, facial detection, identity},
1145 numpages = {35},
1146 articleno = {58},
1147 month = {may},
1148 journal = {Proc. ACM Hum.-Comput. Interact.},
1149 doi = {10.1145/3392866},
1150 url = {https://doi.org/10.1145/3392866},
1151 number = {CSCW1},
1152 volume = {4},
1153 address = {New York, NY, USA},
1154 publisher = {Association for Computing Machinery},
1155 issue_date = {May 2020},
1156 year = {2020},
1157 title = {How We've Taught Algorithms to See Identity: Constructing Race and Gender in Image Databases for Facial Analysis},
1158 author = {Scheuerman, Morgan Klaus and Wade, Kandrea and Lustig, Caitlin and Brubaker, Jed R.},
1159}
1160
1161@inproceedings{Bietti2020,
1162 series = {FAT* '20},
1163 location = {Barcelona, Spain},
1164 keywords = {ethics, regulation, technology ethics, moral philosophy, technology law, self-regulation, AI},
1165 numpages = {10},
1166 pages = {210–219},
1167 booktitle = {Proceedings of the 2020 Conference on Fairness, Accountability, and Transparency},
1168 doi = {10.1145/3351095.3372860},
1169 url = {https://doi.org/10.1145/3351095.3372860},
1170 address = {New York, NY, USA},
1171 publisher = {Association for Computing Machinery},
1172 isbn = {9781450369367},
1173 year = {2020},
1174 title = {From Ethics Washing to Ethics Bashing: A View on Tech Ethics from within Moral Philosophy},
1175 author = {Bietti, Elettra},
1176}
1177
1178@article{shilton2018,
1179 author = {Katie Shilton},
1180 pages = {107-171},
1181 number = {2},
1182 issn = {1551-3955},
1183 doi = {10.1561/1100000073},
1184 title = {Values and Ethics in Human-Computer Interaction},
1185 journal = {Foundations and Trends in Human–Computer Interaction},
1186 volume = {12},
1187 year = {2018},
1188 url = {http://dx.doi.org/10.1561/1100000073},
1189}
1190
1191@inproceedings{Shilton2013,
1192 series = {C\&T '13},
1193 location = {Munich, Germany},
1194 keywords = {virtual community formation, values in design, internet architecture, technology ethics, ethnography},
1195 numpages = {10},
1196 pages = {110–119},
1197 booktitle = {Proceedings of the 6th International Conference on Communities and Technologies},
1198 doi = {10.1145/2482991.2482993},
1199 url = {https://doi.org/10.1145/2482991.2482993},
1200 address = {New York, NY, USA},
1201 publisher = {Association for Computing Machinery},
1202 isbn = {9781450321044},
1203 year = {2013},
1204 title = {Making Space for Values: Communication Values Levers in a Virtual Team},
1205 author = {Shilton, Katie and Koepfler, Jes A.},
1206}
1207
1208@article{Chen_2019,
1209 language = {en},
1210 pages = {40–61},
1211 month = {Jan},
1212 year = {2019},
1213 author = {Chen, Jiawei and Hanrahan, Benjamin V. and Carroll, John M},
1214 journal = {International Journal of Mobile Human Computer Interaction},
1215 number = {1},
1216 doi = {10.4018/IJMHCI.2019010103},
1217 url = {http://services.igi-global.com/resolvedoi/resolve.aspx?doi=10.4018/IJMHCI.2019010103},
1218 issn = {1942-390X, 1942-3918},
1219 volume = {11},
1220 title = {Withshare: A Mobile Application to Support Communzity Coproduction Activities},
1221}
1222
1223@inproceedings{ostrom1999crossing,
1224 year = {1999},
1225 booktitle = {Workshop in Political Theory and Policy Analysis. Ann Arbor MI: University of Michigan Press},
1226 author = {Ostrom, Elinor},
1227 title = {Crossing the great divide. Co-production, synergy \& development, polycentric governance and development},
1228}
1229
1230@inbook{Chen2_2019,
1231 language = {en},
1232 pages = {565–577},
1233 year = {2019},
1234 author = {Chen, Jiawei and Doryab, Afsaneh and Hanrahan, Benjamin V. and Yousfi, Alaaeddine and Beck, Jordan and Wang, Xiying and Bellotti, Victoria and Dey, Anind K. and Carroll, John M.},
1235 publisher = {Springer International Publishing},
1236 booktitle = {Information in Contemporary Society},
1237 doi = {10.1007/978-3-030-15742-5_54},
1238 url = {http://link.springer.com/10.1007/978-3-030-15742-5_54},
1239 isbn = {9783030157418},
1240 volume = {11420},
1241 title = {Context-Aware Coproduction: Implications for Recommendation Algorithms},
1242 address = {Cham},
1243}
1244
1245@misc{ostrom:2009,
1246 year = {2009,
1247howpublished={\url{https://www.nobelprize.org/prizes/economic-sciences/2009/ostrom/lecture/}}},
1248 title = {Elinor Ostrom Prize Lecture},
1249 author = {Ostrom Elinor},
1250}
1251
1252@misc{PAIR:,
1253 year = {2019,
1254howpublished={\url{https://www.pair.withgoogle.com/guidebook/}}},
1255 title = {People + AI Guidebook},
1256 author = {PAIR},
1257}
1258
1259@misc{HAX:,
1260 year = {2022,
1261howpublished={\url{https://www.microsoft.com/en-us/haxtoolkit/}}},
1262 title = {HAX Toolkit},
1263 author = {Microsoft},
1264}
1265
1266@misc{ethical2019,
1267 year = {2019,
1268howpublished={\url{https://ieeexplore.ieee.org/servlet/opac?punumber=9398611}}},
1269 title = {Ethically Aligned Design - A Vision for Prioritizing Human Well-being with Autonomous and Intelligent System},
1270 author = {IEEE},
1271}
1272
1273@incollection{porter2013co,
1274 publisher = {Routledge},
1275 year = {2013},
1276 pages = {163--186},
1277 booktitle = {New public governance, the third sector, and co-production},
1278 author = {Porter, David O},
1279 title = {Co-production and network structures in public education},
1280}
1281
1282@article{Eijk_Steen_2014,
1283 pages = {358–382},
1284 month = {Apr},
1285 year = {2014},
1286 author = {van Eijk, C. J. A. and Steen, T. P. S.},
1287 journal = {Public Management Review},
1288 number = {3},
1289 doi = {10.1080/14719037.2013.841458},
1290 url = {https://doi.org/10.1080/14719037.2013.841458},
1291 issn = {1471-9037},
1292 volume = {16},
1293 title = {Why People Co-Produce: Analysing citizens’ perceptions on co-planning engagement in health care services},
1294}
1295
1296@incollection{cahn2013co,
1297 publisher = {Routledge},
1298 year = {2013},
1299 pages = {147--162},
1300 booktitle = {New public governance, the third sector, and co-production},
1301 author = {Cahn, Edgar S and Gray, Christine},
1302 title = {Co-production from a normative perspective},
1303}
1304
1305@book{Jasanoff_2004c,
1306 language = {eng},
1307 year = {2004},
1308 author = {Jasanoff, Sheila},
1309 publisher = {Routledge},
1310 url = {https://www.taylorfrancis.com/books/edit/10.4324/9780203413845/states-knowledge-sheila-jasanoff},
1311 isbn = {9781134328345},
1312 title = {States of knowledge: the co-production of science and the social order},
1313 address = {London},
1314}
1315
1316@misc{vera2019,
1317 copyright = {arXiv.org perpetual, non-exclusive license},
1318 year = {2019},
1319 publisher = {arXiv},
1320 title = {Enabling Value Sensitive AI Systems through Participatory Design Fictions},
1321 keywords = {Human-Computer Interaction (cs.HC), Artificial Intelligence (cs.AI), FOS: Computer and information sciences, FOS: Computer and information sciences},
1322 author = {Liao, Q. Vera and Muller, Michael},
1323 url = {https://arxiv.org/abs/1912.07381},
1324 doi = {10.48550/ARXIV.1912.07381},
1325}
1326
1327@article{Dourish_Bell_2014,
1328 language = {en},
1329 pages = {769–778},
1330 month = {Apr},
1331 year = {2014},
1332 author = {Dourish, Paul and Bell, Genevieve},
1333 journal = {Personal and Ubiquitous Computing},
1334 number = {4},
1335 doi = {10.1007/s00779-013-0678-7},
1336 url = {http://link.springer.com/10.1007/s00779-013-0678-7},
1337 issn = {1617-4909, 1617-4917},
1338 volume = {18},
1339 title = {“Resistance is futile”: reading science fiction alongside ubiquitous computing},
1340}
1341
1342@inproceedings{borning2012,
1343 series = {CHI '12},
1344 location = {Austin, Texas, USA},
1345 keywords = {value sensitive design, collaborative ethnography, participatory design, feminism, design, culturally-specific values, universal values, qualitative research, voice},
1346 numpages = {10},
1347 pages = {1125–1134},
1348 doi = {10.1145/2207676.2208560},
1349 url = {https://doi.org/10.1145/2207676.2208560},
1350 address = {New York, NY, USA},
1351 publisher = {Association for Computing Machinery},
1352 isbn = {9781450310154},
1353 year = {2012},
1354 title = {Next Steps for Value Sensitive Design},
1355 author = {Borning, Alan and Muller, Michael},
1356}
1357
1358@article{wynne1996may,
1359 year = {1996},
1360 pages = {44},
1361 volume = {40},
1362 journal = {Risk, environment and modernity: Towards a new ecology},
1363 author = {Wynne, Brian},
1364 title = {May the sheep safely graze? A reflexive view of the expert-lay knowledge divide},
1365}
1366
1367@article{jasanoff2001election,
1368 publisher = {Sage Publications London},
1369 year = {2001},
1370 pages = {461--467},
1371 number = {3},
1372 volume = {31},
1373 journal = {Social Studies of Science},
1374 author = {Jasanoff, Sheila},
1375 title = {Election 2000: mechanical error or system failure},
1376}
1377
1378@incollection{jasanoff2004idiom,
1379 isbn = {9780203413845},
1380 publisher = {Routledge},
1381 year = {2004},
1382 pages = {1--12},
1383 booktitle = {States of knowledge},
1384 author = {Jasanoff, Sheila},
1385 title = {The idiom of co-production},
1386}
1387
1388@inproceedings{keegan2016,
1389 series = {CSCW '16},
1390 location = {San Francisco, California, USA},
1391 keywords = {online knowledge collaboration, socio-technical system, routines, organizational practice, sequence analysis, peer production, Wikipedia},
1392 numpages = {15},
1393 pages = {1065–1079},
1394 doi = {10.1145/2818048.2819962},
1395 url = {https://doi.org/10.1145/2818048.2819962},
1396 address = {New York, NY, USA},
1397 publisher = {Association for Computing Machinery},
1398 isbn = {9781450335928},
1399 year = {2016},
1400 title = {Analyzing Organizational Routines in Online Knowledge Collaborations: A Case for Sequence Analysis in CSCW},
1401 author = {Keegan, Brian C. and Lev, Shakked and Arazy, Ofer},
1402}
1403
1404@article{carroll2016,
1405 numpages = {7},
1406 pages = {26–32},
1407 month = {jul},
1408 journal = {Computer},
1409 abstract = {Service coproductions are reciprocal activities with no sharp boundary between providers and recipients; instead, all participants collaborate to enact the service. User participation is validated by recognition instead of extrinsic economic exchange. Mobility and context awareness can be crucial for successful coproductions.},
1410 doi = {10.1109/MC.2016.194},
1411 url = {https://doi.org/10.1109/MC.2016.194},
1412 issn = {0018-9162},
1413 number = {7},
1414 volume = {49},
1415 address = {Washington, DC, USA},
1416 publisher = {IEEE Computer Society Press},
1417 issue_date = {July 2016},
1418 year = {2016},
1419 title = {In Search of Coproduction: Smart Services as Reciprocal Activities},
1420 author = {Carroll, John M. and Chen, Jiawei and Yuan, Chien Wen Tina and Hanrahan, Benjamin V.},
1421}
1422
1423@article{yu2018,
1424 copyright = {arXiv.org perpetual, non-exclusive license},
1425 year = {2018},
1426 publisher = {arXiv},
1427 title = {Building Ethics into Artificial Intelligence},
1428 keywords = {Artificial Intelligence (cs.AI), FOS: Computer and information sciences, FOS: Computer and information sciences},
1429 author = {Yu, Han and Shen, Zhiqi and Miao, Chunyan and Leung, Cyril and Lesser, Victor R. and Yang, Qiang},
1430 url = {https://arxiv.org/abs/1812.02953},
1431 doi = {10.48550/ARXIV.1812.02953},
1432}
1433
1434@article{Shilton_2013,
1435 language = {en},
1436 pages = {374–397},
1437 year = {2013},
1438 author = {Shilton, Katie},
1439 journal = {Science, Technology, \& Human Values},
1440 number = {3},
1441 doi = {10.1177/0162243912436985},
1442 url = {http://journals.sagepub.com/doi/10.1177/0162243912436985},
1443 volume = {38},
1444 title = {Values Levers: Building Ethics into Design},
1445}
1446
1447@article{dewey1939theory,
1448 year = {1939},
1449 journal = {International encyclopedia of unified science},
1450 author = {Dewey, John},
1451 title = {Theory of valuation.},
1452}
1453
1454@inproceedings{dan2022,
1455 series = {DIS '22},
1456 location = {Virtual Event, Australia},
1457 keywords = {values levers, design ethnography, values in design, digital civics},
1458 numpages = {11},
1459 pages = {643–653},
1460 booktitle = {Designing Interactive Systems Conference},
1461 doi = {10.1145/3532106.3533570},
1462 url = {https://doi.org/10.1145/3532106.3533570},
1463 address = {New York, NY, USA},
1464 publisher = {Association for Computing Machinery},
1465 isbn = {9781450393584},
1466 year = {2022},
1467 title = {Critically Engaging with Embedded Values through Constrained Technology Design},
1468 author = {Richardson, Dan and Cumbo, Bronwyn J. and Bartindale, Tom and Varghese, Delvin and Saha, Manika and Saha, Pratyasha and Ahmed, Syed Ishtiaque and Oliver, Gillian C. and Olivier, Patrick},
1469}
1470
1471@inproceedings{Yildirim2022How,
1472 series = {CHI '22},
1473 location = {New Orleans, LA, USA},
1474 keywords = {design, artificial intelligence, User experience, machine learning},
1475 numpages = {13},
1476 articleno = {483},
1477 booktitle = {Proceedings of the 2022 CHI Conference on Human Factors in Computing Systems},
1478 doi = {10.1145/3491102.3517491},
1479 url = {https://doi.org/10.1145/3491102.3517491},
1480 address = {New York, NY, USA},
1481 publisher = {Association for Computing Machinery},
1482 isbn = {9781450391573},
1483 year = {2022},
1484 title = {How Experienced Designers of Enterprise Applications Engage AI as a Design Material},
1485 author = {Yildirim, Nur and Kass, Alex and Tung, Teresa and Upton, Connor and Costello, Donnacha and Giusti, Robert and Lacin, Sinem and Lovic, Sara and O'Neill, James M and Meehan, Rudi O'Reilly and \'{O} Loide\'{a}in, Eoin and Pini, Azzurra and Corcoran, Medb and Hayes, Jeremiah and Cahalane, Diarmuid J and Shivhare, Gaurav and Castoro, Luigi and Caruso, Giovanni and Oh, Changhoon and McCann, James and Forlizzi, Jodi and Zimmerman, John},
1486}
1487
1488@book{Noble_2018,
1489 language = {eng},
1490 year = {2018},
1491 author = {Noble, Safiya Umoja},
1492 publisher = {New York university press},
1493 isbn = {9781479837243},
1494 title = {Algorithms of oppression: how search engines reinforce racism},
1495 address = {New York},
1496}
1497
1498@article{ehsan2021,
1499 bibsource = {dblp computer science bibliography, https://dblp.org},
1500 biburl = {https://dblp.org/rec/journals/corr/abs-2107-13509.bib},
1501 timestamp = {Fri, 30 Jul 2021 13:03:06 +0200},
1502 eprint = {2107.13509},
1503 eprinttype = {arXiv},
1504 url = {https://arxiv.org/abs/2107.13509},
1505 year = {2021},
1506 volume = {abs/2107.13509},
1507 journal = {CoRR},
1508 title = {The Who in Explainable {AI:} How {AI} Background Shapes Perceptions
1509of {AI} Explanations},
1510 author = {Upol Ehsan and
1511Samir Passi and
1512Q. Vera Liao and
1513Larry Chan and
1514I{-}Hsiang Lee and
1515Michael J. Muller and
1516Mark O. Riedl},
1517}
1518
1519@book{Eubanks_2017,
1520 year = {2017},
1521 author = {Eubanks, Virginia},
1522 publisher = {St. Martin’s Press},
1523 callnumber = {HC79.P6 E89 2017},
1524 isbn = {9781250074317},
1525 title = {Automating inequality: how high-tech tools profile, police, and punish the poor},
1526 edition = {First Edition},
1527 address = {New York, NY},
1528}
1529
1530@inproceedings{birhane2022A,
1531 series = {FAccT '22},
1532 location = {Seoul, Republic of Korea},
1533 keywords = {Trends, AI Ethics, Justice, FAccT, AIES},
1534 numpages = {11},
1535 pages = {948–958},
1536 booktitle = {2022 ACM Conference on Fairness, Accountability, and Transparency},
1537 doi = {10.1145/3531146.3533157},
1538 url = {https://doi.org/10.1145/3531146.3533157},
1539 address = {New York, NY, USA},
1540 publisher = {Association for Computing Machinery},
1541 isbn = {9781450393522},
1542 year = {2022},
1543 title = {The Forgotten Margins of AI Ethics},
1544 author = {Birhane, Abeba and Ruane, Elayne and Laurent, Thomas and S. Brown, Matthew and Flowers, Johnathan and Ventresque, Anthony and L. Dancy, Christopher},
1545}
1546
1547@misc{doran2017,
1548 copyright = {arXiv.org perpetual, non-exclusive license},
1549 year = {2017},
1550 publisher = {arXiv},
1551 title = {What Does Explainable AI Really Mean? A New Conceptualization of Perspectives},
1552 keywords = {Artificial Intelligence (cs.AI), FOS: Computer and information sciences, FOS: Computer and information sciences},
1553 author = {Doran, Derek and Schulz, Sarah and Besold, Tarek R.},
1554 url = {https://arxiv.org/abs/1710.00794},
1555 doi = {10.48550/ARXIV.1710.00794},
1556}
1557
1558@book{Fjeld2020,
1559 language = {en},
1560 month = {Jan},
1561 year = {2020},
1562 author = {Fjeld, Jessica and Achten, Nele and Hilligoss, Hannah and Nagy, Adam and Srikumar, Madhulika},
1563 institution = {Social Science Research Network},
1564 number = {3518482},
1565 url = {https://papers.ssrn.com/abstract=3518482},
1566 title = {Principled Artificial Intelligence: Mapping Consensus in Ethical and Rights-Based Approaches to Principles for AI},
1567 type = {SSRN Scholarly Paper},
1568 address = {Rochester, NY},
1569}
1570
1571@inproceedings{passi2019problem,
1572 series = {FAT* '19},
1573 location = {Atlanta, GA, USA},
1574 keywords = {Machine Learning, Data Science, Problem Formulation, Target Variable, Fairness},
1575 numpages = {10},
1576 pages = {39–48},
1577 booktitle = {Proceedings of the Conference on Fairness, Accountability, and Transparency},
1578 doi = {10.1145/3287560.3287567},
1579 url = {https://doi.org/10.1145/3287560.3287567},
1580 address = {New York, NY, USA},
1581 publisher = {Association for Computing Machinery},
1582 isbn = {9781450361255},
1583 year = {2019},
1584 title = {Problem Formulation and Fairness},
1585 author = {Passi, Samir and Barocas, Solon},
1586}
1587
1588@article{arya2019,
1589 bibsource = {dblp computer science bibliography, https://dblp.org},
1590 biburl = {https://dblp.org/rec/journals/corr/abs-1909-03012.bib},
1591 timestamp = {Tue, 17 Sep 2019 11:23:44 +0200},
1592 eprint = {1909.03012},
1593 eprinttype = {arXiv},
1594 url = {http://arxiv.org/abs/1909.03012},
1595 year = {2019},
1596 volume = {abs/1909.03012},
1597 journal = {CoRR},
1598 title = {One Explanation Does Not Fit All: {A} Toolkit and Taxonomy of {AI}
1599Explainability Techniques},
1600 author = {Vijay Arya and
1601Rachel K. E. Bellamy and
1602Pin{-}Yu Chen and
1603Amit Dhurandhar and
1604Michael Hind and
1605Samuel C. Hoffman and
1606Stephanie Houde and
1607Q. Vera Liao and
1608Ronny Luss and
1609Aleksandra Mojsilovic and
1610Sami Mourad and
1611Pablo Pedemonte and
1612Ramya Raghavendra and
1613John T. Richards and
1614Prasanna Sattigeri and
1615Karthikeyan Shanmugam and
1616Moninder Singh and
1617Kush R. Varshney and
1618Dennis Wei and
1619Yunfeng Zhang},
1620}
1621
1622@inproceedings{krafft2021,
1623 series = {FAccT '21},
1624 location = {Virtual Event, Canada},
1625 keywords = {surveillance, accountability, algorithmic equity, Participatory design, algorithmic justice, participatory action research, regulation},
1626 numpages = {10},
1627 pages = {772–781},
1628 booktitle = {Proceedings of the 2021 ACM Conference on Fairness, Accountability, and Transparency},
1629 doi = {10.1145/3442188.3445938},
1630 url = {https://doi.org/10.1145/3442188.3445938},
1631 address = {New York, NY, USA},
1632 publisher = {Association for Computing Machinery},
1633 isbn = {9781450383097},
1634 year = {2021},
1635 title = {An Action-Oriented AI Policy Toolkit for Technology Audits by Community Advocates and Activists},
1636 author = {Krafft, P. M. and Young, Meg and Katell, Michael and Lee, Jennifer E. and Narayan, Shankar and Epstein, Micah and Dailey, Dharma and Herman, Bernease and Tam, Aaron and Guetler, Vivian and Bintz, Corinne and Raz, Daniella and Jobe, Pa Ousman and Putz, Franziska and Robick, Brian and Barghouti, Bissan},
1637}
1638
1639@inproceedings{Katell2020,
1640 url = {https://doi.org/10.1145/3351095.3372874},
1641 address = {New York, NY, USA},
1642 publisher = {Association for Computing Machinery},
1643 isbn = {9781450369367},
1644 year = {2020},
1645 title = {Toward Situated Interventions for Algorithmic Equity: Lessons from the Field},
1646 author = {Katell, Michael and Young, Meg and Dailey, Dharma and Herman, Bernease and Guetler, Vivian and Tam, Aaron and Bintz, Corinne and Raz, Daniella and Krafft, P. M.},
1647}
1648
1649@book{Gray_Suri_2019,
1650 year = {2019},
1651 author = {Gray, Mary L. and Suri, Siddharth},
1652 publisher = {Houghton Mifflin Harcourt},
1653 callnumber = {HD6331},
1654 isbn = {9781328566287},
1655 title = {Ghost work: how to stop Silicon Valley from building a new global underclass},
1656 address = {Boston},
1657}
1658
1659@article{Metcalf_2019,
1660 language = {en},
1661 pages = {449–476},
1662 month = {Jun},
1663 year = {2019},
1664 author = {Metcalf, Jacob and Moss, Emanuel and boyd, danah},
1665 journal = {Social Research: An International Quarterly},
1666 number = {2},
1667 doi = {10.1353/sor.2019.0022},
1668 url = {https://muse.jhu.edu/article/732185},
1669 issn = {1944-768X},
1670 volume = {86},
1671 title = {Owning Ethics: Corporate Logics, Silicon Valley, and the Institutionalization of Ethics},
1672}
1673
1674@book{Friedman_Hendry_2019,
1675 year = {2019},
1676 author = {Friedman, Batya and Hendry, David},
1677 publisher = {The MIT Press},
1678 callnumber = {QA76.9.S88 F75 2019},
1679 isbn = {9780262039536},
1680 title = {Value sensitive design: shaping technology with moral imagination},
1681 address = {Cambridge, Massachusetts},
1682}
1683
1684@article{Eriksson2022,
1685 pages = {830736},
1686 month = {Feb},
1687 year = {2022},
1688 author = {Eriksson, Eva and Nilsson, Elisabet M. and Hansen, Anne-Marie and Bekker, Tilde},
1689 journal = {Frontiers in Computer Science},
1690 doi = {10.3389/fcomp.2022.830736},
1691 url = {https://www.frontiersin.org/articles/10.3389/fcomp.2022.830736/full},
1692 issn = {2624-9898},
1693 volume = {4},
1694 title = {Teaching for Values in Human–Computer Interaction},
1695}
1696
1697@article{sarah2020,
1698 bibsource = {dblp computer science bibliography, https://dblp.org},
1699 biburl = {https://dblp.org/rec/journals/corr/abs-2004-13676.bib},
1700 timestamp = {Sat, 02 May 2020 19:17:26 +0200},
1701 eprint = {2004.13676},
1702 eprinttype = {arXiv},
1703 url = {https://arxiv.org/abs/2004.13676},
1704 year = {2020},
1705 volume = {abs/2004.13676},
1706 journal = {CoRR},
1707 title = {Value-based Engineering for Ethics by Design},
1708 author = {Sarah Spiekermann and
1709Till Winkler},
1710}
1711
1712@book{Weick_2006,
1713 language = {eng},
1714 collection = {Topics in social psychology},
1715 year = {2006},
1716 author = {Weick, Karl E.},
1717 publisher = {McGraw-Hill},
1718 isbn = {9780075548089},
1719 title = {The social psychology of organizing},
1720 series = {Topics in social psychology},
1721 edition = {2. ed., [Nachdr.]},
1722 address = {New York},
1723}
1724
1725@book{Scott_Davis_2016,
1726 language = {eng},
1727 year = {2016},
1728 author = {Scott, W. Richard and Davis, Gerald F.},
1729 publisher = {Routledge},
1730 url = {https://www.taylorfrancis.com/books/mono/10.4324/9781315663371/organizations-organizing-richard-scott-gerald-davis},
1731 isbn = {9781317345923},
1732 title = {Organizations and organizing: rational, natural and open systems perspectives},
1733 edition = {1st ed},
1734 address = {Abingdon, Oxon},
1735}
1736
1737@article{Green_2021,
1738 pages = {209–225},
1739 month = {Sep},
1740 year = {2021},
1741 author = {Green, Ben},
1742 journal = {Journal of Social Computing},
1743 number = {3},
1744 doi = {10.23919/JSC.2021.0018},
1745 url = {https://ieeexplore.ieee.org/document/9684741/},
1746 issn = {2688-5255},
1747 volume = {2},
1748 title = {The Contestation of Tech Ethics: A Sociotechnical Approach to Technology Ethics in Practice},
1749}
1750
1751@inbook{Wagner_2019,
1752 pages = {84–89},
1753 month = {Dec},
1754 year = {2019},
1755 editor = {Bayamlioglu, Emre and Baraliuc, Irina and Janssens, Liisa Albertha Wilhelmina and Hildebrandt, Mireille},
1756 author = {Wagner, Ben},
1757 publisher = {Amsterdam University Press},
1758 booktitle = {BEING PROFILED},
1759 doi = {10.1515/9789048550180-016},
1760 url = {https://www.degruyter.com/document/doi/10.1515/9789048550180-016/html},
1761 isbn = {9789048550180},
1762 title = {Ethics As An Escape From Regulation. From “Ethics-Washing” To Ethics-Shopping?},
1763}
1764
1765@article{Gieryn_1983,
1766 pages = {781},
1767 month = {Dec},
1768 year = {1983},
1769 author = {Gieryn, Thomas F.},
1770 journal = {American Sociological Review},
1771 number = {6},
1772 doi = {10.2307/2095325},
1773 url = {http://www.jstor.org/stable/2095325?origin=crossref},
1774 issn = {00031224},
1775 volume = {48},
1776 title = {Boundary-Work and the Demarcation of Science from Non-Science: Strains and Interests in Professional Ideologies of Scientists},
1777}
1778
1779@article{rakova2021,
1780 keywords = {organizational structure, industry practice, responsible ai},
1781 numpages = {23},
1782 articleno = {7},
1783 month = {apr},
1784 journal = {Proc. ACM Hum.-Comput. Interact.},
1785 doi = {10.1145/3449081},
1786 url = {https://doi.org/10.1145/3449081},
1787 number = {CSCW1},
1788 volume = {5},
1789 address = {New York, NY, USA},
1790 publisher = {Association for Computing Machinery},
1791 issue_date = {April 2021},
1792 year = {2021},
1793 title = {Where Responsible AI Meets Reality: Practitioner Perspectives on Enablers for Shifting Organizational Practices},
1794 author = {Rakova, Bogdana and Yang, Jingying and Cramer, Henriette and Chowdhury, Rumman},
1795}
1796
1797@article{goyal2022your,
1798 publisher = {ACM New York, NY, USA},
1799 year = {2022},
1800 pages = {1--28},
1801 number = {CSCW2},
1802 volume = {6},
1803 journal = {Proceedings of the ACM on Human-Computer Interaction},
1804 author = {Goyal, Nitesh and Kivlichan, Ian D and Rosen, Rachel and Vasserman, Lucy},
1805 title = {Is Your Toxicity My Toxicity? Exploring the Impact of Rater Identity on Toxicity Annotation},
1806}
Attribution
arXiv:2307.10221v1
[cs.AI]
License: cc-by-sa-4.0