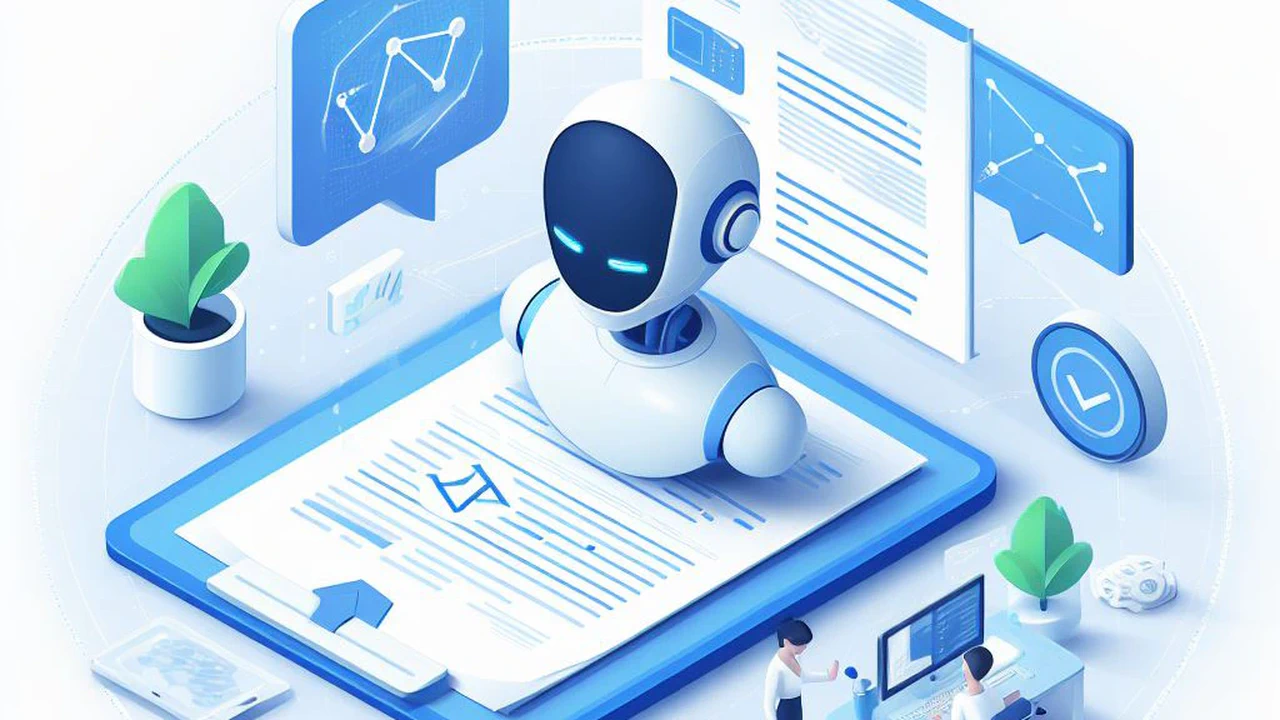
- Papers
Papers is Alpha. This content is part of an effort to make research more accessible, and (most likely) has lost some details from the original. You can find the original paper here.
Introduction
In our increasingly data-driven society, artificial intelligence (AI) has become pervasive for decision-making in a wide range of fields. AI has immense potential to make positive change in fields ranging from agricultureto healthcare. However, as with any tool, AI can also cause great harm if developed and used improperly or inappropriately; regardless of whether this is done unintentionally or maliciously. The consequences of bad AI practice can range from bias affecting underprivileged or underrepresented groups, to financial harm, to physical and mental harm.
The need to develop AI in a way that benefits human life and societies has lead to the emergence of responsible AI (RAI). RAI is fundamentally the field of applying ethics to the development and utilization of AI, to ensure that AI systems and used for the good of humanity. As with all moral philosophy, there is no single consensus on what makes AI ethical and therefore responsible. However, efforts have been made to establish RAI characteristics, frameworks, and guidelines in academia, industry, and goverments and political bodies. Frequently identified pillars of RAI across these diverse sources include fairness, robustness, transparency, accountability, privacy, and safety.
Explainability is also considered as a pillar of RAI by many works, often connected to transparency. Explainability is broadly considered by the field of explainable AI (XAI), which is focused on providing humans with insight into the reasoning of AI models during their decision-making processes. Researchers in the XAI domain have developed methods for producing explanations that include text-based, visual, and feature importancemethods.
Given that XAI techniques improve transparency, it is understandable that some researchers have classed explainability as a pillar of RAI. However, our review of the literature finds that considering XAI as one discrete pillar of RAI, separate to all others, is insufficient to capture the potential impact of explainability on RAI. In this review, we find substantial evidence that XAI should instead be considered as the foundation of RAI; this key difference is shown in Fig. fig-pillars.
In our review, XAI is shown to support assessment of fairness, robustness, and safety, thus supporting responsible AI operation and enabling improvement as needed. The transparency offered by XAI is shown to improve interpretability and understability of AI through human evaluation in several studies, which in turn supports accountability for decision making in critical sectors such as financeand law. Finally, it is illustrated that XAI can be combined with privacy-preserving techniques to ensure that the requirement of privacy is met without compromising on other RAI requirements.
Original Contributions
The literature contains several high-quality reviews on the topics of XAI and RAI; however, these are typically considered as separate topics. In this work, we fill a key gap in the literature by illustrating that XAI is foundational to RAI. The key contributions of this work are as follows:
- We conduct a broad-scoping review of the literature on both XAI and RAI, highlighting the technologies, principals, and frameworks developed by previous works.
- We propose a novel framework that considers explainable AI as the foundation of responsible AI. This is in contrast to previous works, which have considered XAI as a single pillar of RAI.
- We demonstrate that XAI is foundational to the RAI principles of fairness, robustness, transparency, accountability, privacy and safety through rigorous exploration of the XAI literature.
- Building upon our findings, we illustrate real-world use cases where XAI directly supports RAI in applications within the key fields of generative AI, healthcare, and transportation.
Comparison to Other Works
The field of XAI has been extremely active in recent years, and responsible AI has also become a hot topic. As such, several high-quality surveys have examined these two topics. However, the concept of XAI as the foundational technology of RAI has not been considered in previous works. This is shown in Table tab-literaturecomparison, where we compare several recent surveys in the literature. We exclude surveys that review XAI and/or RAI in a domain-specific context (i.e., healthcare or finance).
Comparison of recent surveys on explainable or responsible AI. Key: The topic is explored in depth. The topic is briefly mentioned. The topic is not discussed.
Table Label: tab-literaturecomparison
Download PDF to view tableResponsible AI has been considered in recent surveys by Kaur _et al._and Li et al.. In both of these works, the literature on RAI was reviewed and findings were used to propose principals and guidelines for the development and deployment of RAI. In each work, explainability was identified as a characteristic of RAI but was not explored in depth. Characteristics of transparency, accountability, fairness, and robustness were identified by each work.
Another work by Ashok _et al._sought to outline broader ethical principles for digital technology development, including but not limited to AI. They ultimately proposed 14 ethical characteristics, which included accountability, fairness, and privacy. Explainability was not discussed.
RAI was explored through the lens of multiple critical use cases in another recent survey by Anagnostou et al.. Their work first explored the problems in critical sectors including healthcare and transportation, before identifying several characteristics required of RAI. These characteristics were similar to other works and included transparency, accountability, and fairness. Explainability was mentioned as a strategy for supporting transparency, but was not explored in depth.
Dwivedi _et al._recently conducted a survey that explored explainability techniques and provides guidance on Python programming packages that allow for implementation. Similarly, a recent review by Minh _et al._explored XAI techniques in depth, with some discussion of applications. Furthermore, a recent survey by Ahmed _et al._discussed AI, XAI, and use cases of XAI in Industry 4.0. However, these three works did not discuss responsible or ethical AI concepts.
Another recent survey conducted by Saeed _et al._explored XAI with a particular focus on future challenges. They also briefly discuss how XAI can be used to create trustworthy AI systems, which supports RAI. Perhaps the survey that most considered the relationship between XAI and RAI was conducted by Barredo Arrieta et al.. They comprehensively reviewed the literature on XAI, before discussing RAI as a separate but related topic. They briefly highlight how XAI could support RAI in terms of transparency and fairness. However, their review overlooked the usefulness of XAI in areas of RAI including robustness.
Overall, the literature is rich with surveys on RAI and XAI separately. However, there is relatively little discussion in the literature of the strong relationship between these two topics. In this review, we fill that gap in the literature by investigating how XAI can be used to create AI systems that align with existing RAI frameworks and characteristics.
Structure of the Paper
The remainder of this paper is structured as follows: Section 2 addresses the question of what makes AI explainable, and explores the types and applications of explainable AI. Section 3 then discusses responsible AI, including why responsibility is important and the characteristics that make AI responsible. Section 4 then examines how the pillars of responsible AI can be achieved through explainability. In Section 5, we present several real-world use cases to highlight the importance of explainability to produce responsible and trustworthy AI for sectors including healthcare and transportation. We present recommendations for future works in the AI domain in Section 6, before concluding the paper in Section 7. The key contents of this paper are illustrated in Fig. fig-structure.
Explainable AI
What is Explainable AI?
Explainable AI (XAI) describes strategies and processes used to make AI models more understandable and interpretable to their developers and end-users, without significant compromise on performance. Audience is critical to consider; strategies utilised to understand a model as the developer may differ from those needed to explain a model to non-technical end users. The key motivations for explainability are improved transparency and trust in AI models, particularly those used in critical applications.
The need for explainable AI is primarily due to the increasing complexity of AI models. Early AI models, such as linear and logistic regression algorithms, can be considered as explainable AI models due to being understandable by design. In such models, the internal mechanisms linking input to output can be readily visualised and interpreted. However, these models are often limited in terms of performance; their simplicity prevents them from accurately interpreting complex data.
As computing resources have improved, increasingly complex AI algorithms have been developed to support better interpretation of complex data. Advanced AI algorithms that continue to grow in prevalence include deep neural networks (DNNs) and random forest. Such models have demonstrated high performance in many domains, however their complexity limits insight into what happens in between input and output; they are not inherently understandable. The increase of high-performing yet opaque models, often termed black-box models, has lead to the substantially increasing interest in XAI for interpreting complex models.
The following subsections dive deeper into the existing literature on XAI, focusing on both explainability by design, where models are intentionally designed to be inherently interpretable, and post-hoc explainability, whereby the model is retrospectively explained after its development or use. Within both types of explainability, there are methods designed to provide local explanations (i.e., explain a single prediction) or global explanations (i.e., explain the general decision-making process of the model). Additionally, some strategies are model-agnostic (i.e., they can be applied to all or many models), while others are model-specific (they can only be applied to a select model). We introduce these terms here as they are relevant to our discussions in the next two subsections.
Explainability by Design
Several AI models can be considered explainable by design due to their simplicity; their inner workings are transparent and thus inherently interpretable. Models that fall into this category include linear regression, generalised additive models, logistic regression, decision trees, k-nearest neighbour, and low-dimensionality support vector machines.
Linear Regression
Linear regression (LR) seeks to find a line of best fit to continuous data, as illustrated in Fig. fig-linreg. In its simplest form with one independent variable, this type of regression is widely understood and thus inherently explainable. Multivariate LR is more common in the literature as it can consider multiple independent variables, each of which can be explained via individual LR plots. This is still relatively interpretable for lower numbers of variables, however weightings of variables may need to be explained. Despite their advantages in explainability, the performance of LR is limited by its inability to capture non-linear relationships, or complex relationships between variables. Nonetheless, multivariate LR remains in use with recent works utilising it to identify radiomics features associated with clinical outcomes, water and wastewater forecasting, and pandemic preparedness assessment.
Generalised Additive Models
Generalised additive models (GAMs) overlap with linear regression in that they attempt to fit a line to the data. However, they differ in that they are not constrained to linear features. They are comprised of multiple `splines’ - non-linear smooth functions - added together to give an overall line describing the data, as illustrated in Fig. fig-gamreg. While there is some complexity in their development, their decisions can be readily explained to the end-user through illustrations of the fitted GAM lines for each variable considered; as with linear regression, interpretabiilty decreases as the number of variables increases. Due to their ability to capture non-linear relationships, GAMs have recently been used for applications including predicting time to crop maturity, electrical load forecasting, and bushfire prediction.
Logistic Regression
Commonly used for classification problems, logistic regression finds a logistic function that best fits to a given set of data. The binary classification case is shown in Fig. fig-logreg; the threshold (grey) line splits the outputs into the two classes. This can again be clearly illustrated and explained. Multiple logistic regression can be performed where multiple variables exist, remaining explainable for lower numbers of variables. Multiclass logistic regression is more complex, however recent papers have attempted to improve explainability through weight heat-maps. Logistic regression has been relatively popular in XAI literature, used for applications including image recognition, telehealth record sentiment analysis, adverse reaction to medication prediction, and predicting student outcomes. However, logistic regression is often outperformed by more advanced models.
Decision Trees
Decision trees (DTs) utilise multiple input features to make a series of decisions resulting in an output. The features are typically `branching’ points, with the tree splitting into separate paths after each feature is considered. The is illustrated by Fig. fig-dt, which shows a simple DT for choosing a sport to play based on weather conditions. DTs are explainable due to their clear logical flow, resembling flowcharts that are common in many industries. However, they are limited in their implementation and have become less popular in the literature. DT ensemble methods such as random forest (RF) are more common in the literature, however explainability decreases as the number of trees increases; many RF papers explain their models using post-hoc methodsdiscussed in the next section.
k-Nearest Neighbour
In $k$ -nearest neighbour (KNN) models, a prediction is made by identifying the k closest datapoints to the new datapoint of interest, and using their values to determine what output value should be assigned to a new input. In the classification example illustrated in Fig. fig-simpleknn, this would result in the new data being classified as Class 2. KNN can also be used for regression tasks. The premise of using proximate data to make a decision is intuitive, and can be easily visualised where k is small. However, as the value of k increases, the interpretability of the model decreases. The simplicity of KNN also leads to limitations, including susceptibility to unimportant features and ignoring the distances of each neighbour. Classic KNN is primarily seen in the literature for benchmarking or validating feature selection algorithms, however KNN variants have been recently used in applications including severity assessment for Parkinson’s diseaseand prediction of cardiovascular disease.
Support Vector Machines
Support vector machines (SVMs) are generally not considered to be explainable, as they are challenging to visualise or explain for higher dimensionality inputs. However, we argue that this is also true of algorithms such as linear regression. Low-dimensionality SVMs split data into classes based on a line or hyperplane. For simple binary classification scenarios with up to three features (i.e., three dimensions), the clusters and separating line or plane can be readily illustrated to the end user; an example for 2D binary classification is given in Fig. fig-simplesvm. Due to the interpretability of low-dimensionality SVM, some early studies have sought to represent complex data in lower-dimensional feature spaces so that explainable 3D SVM can be utilised.
Post-Hoc Explainability
Permutation Importance
Permutation importance is a model-agnostic explainability technique that seeks to quantify feature importances in a global context, applied after a model is trained. It involves randomly shuffling all values for a particular feature and assessing whether this has an impact on model performance. The output of permutation importance is a list of weights indicating how important each feature is. These weights represent the impact of shuffling the particular feature on a metric of interest. An example output for a house pricing task is shown in Fig. fig-permutation, with features that caused the highest increase in prediction error considered to be the most important. Permutation importance is a computationally expensive technique, however both the output and the method itself are easily understood. This has lead to it being widely used in applications including disease biomarker discovery, digital soil mapping, wildfire susceptibility analysis, and prediction of biohydrogen production efficiency. A key limitation is that its results can be misleading where two variables are highly correlated; shuffling one may have little effect on the output due to the presence of the other.
Partial Dependence Plots and Individual Conditional Expectation
Partial dependence plots (PDPs) and individual conditional expectation (ICE) plots are model-agnostic methods which seek to understand the changes in prediction if a single feature is modified in the global context. After a model is trained, the particular feature is modified to assess the impact on the output. ICE plots illustrate the impact of the feature for every instance in the database; e.g., every instance is plotted as a single line. PDP plots find the average of the impact across all features, and therefore result in a single line. An example for the impact of land size on house pricing is illustrated in Fig. fig-iceandpdp.
ICE and PDP each have advantages and disadvantages. ICE can reveal heterogeneous effects, such as when changing a feature has a negative effect on half of the instances, but a positive effect in the other half. In such a case, PDP would display a flat, horizontal line - a result that is misleading and not useful. However, the interpretability of ICE decreases as the number of instances increases; plots become crowded, and it becomes harder to separate instances or identify atypical instances. PDP avoids this problem by illustrating an average.
PDP is also useful in in graphing the dependencies of two features. As shown in Fig. fig-2dpdp, PDP can be used to generate contour plots that illustrate the relationship of two features to the output of interest - in our example, the relationship of house and land size to house price. This cannot be achieved with ICE, as the large number of overlapping contours would not be interpretable.
As they each have their respective benefits, ICE and PDP are commonly applied together; they have seen use in applications including acute kidney injury risk assessment, identification of factors in crash severity, water stream degradation assessment, and mortality risk prediction in intensive care settings. PDP has also been commonly applied alone in the literature. Recent works have applied PDP to applications including biochar yield prediction, concrete strength prediction, crop yield assessment, and deterioration in hepatitis patients.
Shapley Additive Explanations
Shapley Additive Explanations (SHAP)is a model-agnostic explainability approach that uses a game theory approach to assess the impact on model performance when different combinations of features (players') participate in the prediction (
game’). It assesses all combinations of features and uses an additive approach to summarise the impact of each feature.
SHAP can be utilised in several different ways. Examples in the global context are illustrated by Figs. fig-shapbar and fig-shapbee. In Fig. fig-shapbar, feature importances as determined by SHAP are simply graphed as bar charts. Meanwhile, Fig. fig-shapbee shows a `beeswarm’ chart. Features are listed in order of determined importance. Each dot represents a single instance, and its colour indicates whether the feature had a low (blue) through to high (red) value; for example, large distances to the CBD are shown to have a negative impact on the output of house prices.
SHAP can also be used in a local context. For an individual prediction using feature-based data, force plots can be generated to explain which features contributes the final output, including the direction in which they contributed - as shown in Fig. fig-shapforce. For image-based data, heatmaps can be generated to illustrate the regions of an image that SHAP considers most important when making a classification. Examples of these are seen in Figs. fig-shapimage; these examples were generated using the pre-trained ResNet modelfor ImageNet data. The helicopter was incorrectly labelled as a warplane, but the presence of blue squares indicates a lower level of confidence. This transparency can help maintain trust even where predictions are wrong.
SHAP has been extremely popular in recent literature due to the range of clearly interpretable graphical methods that can be implemented to understand its results. SHAP has been used to explain feature importances in applications including mortality risk assessment, concrete creep prediction, plastic waste forecasting, wastewater processing analytics, electric vehicle route planning, and fault detection for rotating machinery. SHAP has also been applied for image explanations in a wide range of settings, including brain tumour diagnosis, offshore oil slick identification, and spam image detection.
Local Interpretable Model-Agnostic Explanations
Local Interpretable Model-Agnostic Explanations (LIME)is an explainability tool that does what its name suggests - offers model-agnostic explanations in a local context. It modifies a single data sample by altering features, and then fits a linear model to the pertubed dataset in the vicinity of the original data sample. This is then used to generate explanations that are accurate for the local context, but invalid in the global context.
LIME returns scores for feature importances in the local context, and thus many graphical methods for interpretation resemble those seen with previous feature importance methods. Fig. fig-limefeature illustrates LIME’s graphical outputs when assessing a single prediction; it displays the overall prediction, a list of the most important features, and a `waterfall’ graph of the most important features. Features on the right pushed the predicted house price higher, while those on the left pushed the prediction lower. LIME can also be used on image and text data, highlighting regions of an image or words within a document that contributes to an overall output prediction, as shown in Fig. fig-limeimage. For each example in Fig. fig-limeimage, the visible section in the left image indicates the areas that contributed towards the prediction. The green and red areas highlighted in the right image are those that had positive and negative impacts on the prediction, respectively.
Alongside SHAP, LIME is one of the most popular explainability methods in the literature. LIME has been used on feature-based data for applications including activity recognition with wearable sensors, steel beam damage prediction, bankruptcy prediction, and stock market forecasting. It has also been broadly used for image and waveform classification tasks including heartbeat detection from electrocardiogram, COVID-19 detection in chest x-rays, and quality assessment for augmented synthetic aperture radar images. Finally, LIME has been used for text analysis tasks including depression identification from symptom self-reportingand identification of resource needs following natural disaster based on Twitter posts.
Class Activation Mapping
Class activation mapping (CAM) is a model-specific method for providing local explanations of image-based predictions made by CNNs. Classical CAM by inserting a global average pooling (GAP) layer after the final convolutional layer of a convolutional neural network (CNN), and weighting the GAP outputs to generate a heatmap. An example of outputs is illustrated in Fig. fig-camfig, with the red hotter' areas contributing the most to a prediction, through to blue
cooler’ areas which contribute the least.
Classical CAM has also inspired a large number of variants in recent years, including Score-CAM, Gradient-weighted CAM (Grad-CAM), and HiResCAM. One recent paper proposed a CAM method using SHAP scores. The key purpose of each variant has been to produce visualisations that more faithfully illustrate a model’s decision making process. However, it is challenging to prove superiority of one method against another without extensive manual comparison of explanations by end-users; this has not been broadly conducted in the literature.
Variants of CAM have been utilised in a wide range of image classification papers, with applications including tree mapping for forest management, Alzheimer’s disease diagnosis from structural magnetic resonance imagery, manufacturing cost prediction from computer-aided designs, and guided rehabilitation training.
Other Techniques
In the above subsections, we have examined some of the most prevalent XAI techniques in the literature. However, there are many additional techniques that are gaining interest in the literature. One example is counterfactuals, a group of model-agnostic local explanation techniques that seek to explain what would need to be different for the outcome to have changed; these are particularly useful for binary classification tasks.
Anchors focus on building rule-based model simplifications that capture the key features and identify if-then rules that explain predictions. Rules are developed in the global context, and can then be used to explain local predictions. Anchors have also inspired related approaches such as the recently proposed CASTLE (cluster-aided space transformation for local explanations), which has been shown to outperform its predecessor on a number of databases.
Emerging AI systems such as generative AI have also posed challenges for explainability, as the underlying models have high complexity. In the natural language processing (NLP) context, one recent work adapted SHAP scores for the language context, highlighting text that indicated COVID-19 misinformation. Another novel method determined layer-wise relevance propagation (LRP) for each component of a transformer model, using this to generate heatmaps with higher accuracy than CAM approaches. This approach was also demonstrated to work in the NLP context, and has been applied to problems such as COVID-19 screening using chest radiography.
Responsible AI
What is Responsible AI?
Responsible AI (RAI) describes the principles by which AI systems are developed, deployed, and utilised in order to comply with legal and ethical standards. As laws and ethics vary from region to region, there is no singular definition of what makes AI responsible; however, many stakeholders have sought to define frameworks to guide responsible AI development.
In much recent literature, RAI has been used synonymously with related terms such as trustworthy AI, ethical AI, and fair or equitable AI. However, we suggest that RAI encompasses these concepts; that truly responsible AI must be trustworthy, fair, and adhere to ethical norms, amongst other characteristics. Based on our review of the literature on RAI and related terms, this section illustrates the importance of responsibility in AI and defines six essential pillars of responsibility.
Importance of Responsibility
AI systems are becoming increasingly prevalent in everyday life, used in applications ranging from media recommendation systems to self-driving vehicles. The dangers of irresponsibly developed AI in safety-critical applications are evident: if autonomous vehicles, weapon systems, or automated medication delivery devices fail to operate as intended, then lives can be lost or irreparably altered. It is essential that AI systems making safety-critical decisions are designed responsibly to ensure that they are reliable, accountable, and free from biases.
In other domains, the dangers of irresponsible AI design are less obvious but can be equally serious. One notable example is facial recognition, which is used by law enforcement, retail stores, and sporting venues. In each of these settings, facial recognition technology is primarily being used to identify perpetrators of crime or misdemeanours. However, researchers have found that commercial facial recognition tools have higher error rates when identifying women and racial or ethnic minorities compared to light-skinned men. Thus, innocent civilians belonging to marginalized groups are more likely to be wrongly criminalized due to mistaken identification. Additionally, predictive policing algorithms trained on images of previously arrested persons have been shown to exhibit racial biases. It is widely theorised that biases in facial recognition algorithms are largely due to data imbalance; minority groups are often underrepresented in data used to train commercial facial recognition models, and overrepresented in data used to train predictive policing models. Thus, irresponsible AI can reproduce and reinforce human biases in justice systems, contributing to a cycle of discriminatory arrest practices.
Facial recognition is just one example of where AI systems can have life-altering consequences. Medical AI diagnosis systems can fail to accurately diagnose conditions in gender, racial, and ethnic minorities, largely due to these groups being underrepresented in medicine and medical data until the last century. Hiring algorithms have also been shown to exhibit biases based on gender, ethnicity, age, disability, and sexuality due to human biases. Even where these characteristics are removed from a resume, NLP approaches can still discriminate against minority groups based on sociolinguistic patterns. Automated lending algorithms are also known to exhibit biases in terms of approval rates and maximum loan sizes for minority groups.
Aside from the potentially life-altering consequences of biased AI, there are also risks of physical, mental and social harm associated directly with some types of AI. Generative AI systems are increasingly popular in a range of fields, ranging from education to psychology. However, there are many recent and historical examples of generative AIs producing biased musings and hate speech, perpetuating misinformation, or responding inappropriately to mental health crises. There is also some evidence that human-chatbot relationships can become dysfunctional and lead to social or mental harm. Even state-of-the-art models such as ChatGPT are already being shown to have dangers; one recent work demonstrated that ChatGPT provided misleading information about mental health disorders and treatments, while another recent work suggests that ChatGPT provides “correct but inadequate” information about medical conditions.
Even seemingly innocuous recommendation systems can have dangers. Some studies have found that recommendation systems on some media platforms can lead to users being guided towards problematic content based on their previous media engagement. Amplification of misinformation and extremism has been found to be particularly prevalent on YouTube, creating `filter bubbles’ where users are predominantly recommended content that confirms their existing views. Research has found that the filter bubble can be easily burst through providing more balanced recommendations, however this is not being implemented by all platforms.
Overall, there are many ways that AI can cause harm - socially, financially, mentally, and physically. The potential of AI to cause harm is the key motivation for RAI. Each of the substantial risks outlined in this section can be mitigated through strategies to open the black box of AI in order to better develop, deploy and use AI responsibly - for the good of society and environments.
Pillars of Responsibility
In this section, we propose a framework comprised of six critical characteristics for responsible AI: fairness, robustness, transparency, accountability, privacy, and safety. Each of these pillars has been identified as critical to RAI based on our review of RAI policies and frameworks recently proposed in academia, industry, and governance, as highlighted in the following subsections. The identification of these key pillars then guides our discussions in Section 4, where we examine how XAI is foundational to all pillars of RAI.
To overview the prevalence of each pillar in the literature, Table tab-raipillars summarises the frequency with which various sources include a particular pillar in their own responsible AI framework or guidelines. In terms of academic sources, we focus on four recent frameworks published in reputable journals. In terms of government and political sources, we have considered AI frameworks and guidelines developed by government and political bodies in eight diverse regions - namely Australia’s Commonwealth Scientific and Industrial Research Organisation (CSIRO), the United States’ (US) National Institute of Standards and Technology (NIST), China’s Ministry of Science and Technology (MOST), India’s INDIAai, Japan’s Ministry of Economy Trade and Industry (METI), Saudi Arabia’s Saudi Authority for Data and Artificial Intelligence (SADAI), the United Kingdom’s (UK) Office for Artificial Intelligence, and the European Union’s (EU) High-Level Expert Group on Artificial Intelligence (AI HLEG). Lastly, we considered RAI principles from six industry sources that are prevalent in the AI space, namely Google, Microsoft, Meta, Samsung, IBM, and Amazon.
Quantifying the number of considered sources that included each pillar in their respective RAI frameworks or guidelines. A total of 4 academic sources, 8 government and political sources, and 6 industry sources were consulted. Academic sources were papers recently published in reputable journals, government and political sources were selected to be representative of a wide range of global regions, and industry sources were selected based on their prevalence in the AI domain.
Table Label: tab-raipillars
Download PDF to view tableFairness
Fairness of an AI system ensures that the model treats people and scenarios equitably, without discrimination. Several recent works on RAI have identified fairness as a key attribute of responsibility. Another work by Mehrabi _et al._conducted a focused survey on bias and fairness in AI, arguing that fairness is the most critical issue facing successful development and deployment of AI systems.
Four of the considered industry sources specifically named fairness as a fundamental principle of RAI. Of the two that didn’t explicitly name fairness, the concept is still present - Google’s related principle is to “avoid creating or reinforcing unfair bias”, while Samsung discusses fairness under their principle of “Diversity & Inclusion”.
Government sources from around the world unanimously agree that fairness and non-discrimination is compulsory for AI systems. However, it is worth noting that not all sources define what fairness means in their regional context.
Overall, the principle of fairness is perhaps the most agreed upon of all the pillars of RAI. Fairness is explicitly mentioned by all consulted sources across academia, governing bodies, and industry.
Robustness
Robustness of an AI system ensures that the model is accurate across all potential use cases, and resilient against malicious attacks. This concept is perhaps the most familiar to AI developers and users; there has long been significant value placed on ensuring that AI systems are robust, reliable, and resilient.
The pillar of robustness has been identified by most academic sources. One work directly names robustness as a key principle of RAI, focusing on robustness against adversarial attacks. Meanwhile, another work identifies the related concept of “technical robustness and safety” as critical; they suggest that a system meets this criteria if it performs as expected, is resilient against attacks, and can recover from failure without causing harm. Additionally, the related concept of reliability is mentioned in one work as a means of ensuring that AI is understandable.
In industry, robustness is widely considered but terminology varies. Meta, IBM, and Amazonall name robustness as a key principal of RAI, while Microsoft uses the terminology of reliability. Googledo not directly discuss reliability or responsibility, but under the principle of ensuring safety they mention the need to “avoid unintended results that create risks of harm”. This is somewhat related to robustness, but does not cover all elements of the concept. Interestingly, Samsungdo not discuss robustness, reliability, or accuracy in their RAI framework.
Most government and political bodies note the need for robustness in RAI systems. Robustness is noted as key for responsibility by government bodies in the US, EU, Japan, and Saudi Arabia. Those that did not discuss robustness instead noted that the strongly related concept of reliability is critical for responsible AI systems.
Overall, robustness and reliability are considered across academia, industry, and governing bodies to be essential for the development of ethical and responsible AI.
Transparency
Transparency is the principle of ensuring that the decisions made by an AI system are able to be described and reproduced. For transparency to be achieved, descriptions should be accessible and understandable to users, developers, and other stakeholders.
The principle of transparency in RAI is largely agreed upon by academic, government, and industry sources. In terms of academic sources, three works name transparency as an essential pillar of RAI. Another work also discussed the need for transparency in the context of the overarching principle of “intelligibility”. Each of these works note that transparency also supports the identification of issues within a model that might cause failures or biases.
In industry, transparency is often mentioned but individual definitions vary. Microsoft, IBM, and Samsungeach note transparency as a key RAI principle in the sense of a model being understandable to users, however Samsung stipulates that this applies only where it does not compromise corporate competitiveness. Amazonstate that models should be transparent to the extent that users can decide whether or how to use them, while Meta’s policyfocuses on transparency around how data is used. Google’s stance on transparency is vague, mentioning that AI systems should feature “relevant explanations” and “provide appropriate transparency and control over the use of data”, but this is in the context of accountability and privacy principles.
Government agencies are in consensus regarding transparency - all eight considered government and political bodies noted transparency as a critical feature of responsible AI. Most noted the need for transparency primarily for supporting auditability and for ensuring that end users know how decisions are being made about them by an AI system.
Accountability
Accountability of an AI system ensures that a model can justify its decisions. An AI system is accountable if its functionality and decisions can be explained to users, governing bodies, and other stakeholders to ensure compliance with laws and ethical standards. Accountability also means that AI should be subject to feedback and appeals from users and governing bodies, with mechanisms for remediation in place where issues arise.
In terms of academic literature, accountability is discussed extensively in all considered works. One work notes that the principle of transparency can support accountability, however does not guarantee it. Academic literature emphasises the need for AI systems to be made accountable to ensure that they can be made liable for their decisions.
Industry sources also commonly include accountability in their principles, but implementations vary. Meta notes accountability and governance as critical for RAI, with internal self-governance measures in place along with processes for user appeals. Google explicitly states that RAI should “be accountable to people”, further clarifying that this includes providing explanations and opportunities for feedback and appeal. Conversely, Microsoft states that people should be accountable for AI systems. Amazon mentions the related principle of governance as key for RAI, but provides no clear guidelines on how this should be implemented. IBM discusses accountability and governance extensively in their stance on AI ethics, noting that governance to ensure accountability should occur both internally and externally; however, accountability is not one of their five primary principles of RAI. Samsung does not include accountability or governance in their RAI framework.
Accountability is also considered as a key principle of RAI by all government and political bodies considered, but descriptions of accountability vary. RAI principles from governing bodies in the US, China, India, Japan and Australia focus on holding people accountable for AI systems. Meanwhile, holding AI itself accountable is the focus of accountability principles proposed by political bodies in the EUand the UK. RAI principles from Saudi Arabia’s SADAI considers accountability of both people and technology, indicating that people across the AI supply chain should be accountable for AI systems, as should the system itself. RAI criteria from the US, UK, and Japanconsider transparency and accountability as a combined principle of RAI, while all other bodies consider accountability as an individual principle.
Overall, accountability is another prevalent pillar of RAI in the literature. It is clear that AI systems must be accountable for their decisions in order for systems and developers to meet legal and ethical requirements.
Privacy
Privacy in AI systems relates to ensuring that sensitive information used in training, validating, testing, or using the model remains private. An AI system should not be able to be `reverse engineered’ to reveal private information about persons in the training data, nor should it be manipulable to reveal sensitive information about a person through malicious inputs.
Privacy is named as a key principle of RAI by all considered academic sources. The definitions of privacy in all instances refer to ensuring that sensitive data are not revealed. Emphasis is broadly placed on ensuring that data is protected at all stages of the AI pipeline.
Similarly, all considered industry sources mention the concepts of privacy, data protection, or security in their RAI principles. Implementations of privacy vary somewhat between companies. Meta specify that their privacy approach requires them “assess privacy risks that involve the collection, use, or sharing of people’s information”, however they do not specify how data sharing or collection will be disclosed. Conversely, Google notes the need for consent and notice of how data is used or shared, and IBM notes the need for at least notice. Policies from Microsoft, Amazon and Samsung focus on preventing data breaches.
All considered government and political sources also noted the need for privacy in RAI. Privacy is directly named as an RAI principle by six bodies, while the US NIST used the terminology `privacy-enhancing’. The UK government emphasised the related concepts of data protection and security. All definitions focused on preserving individual privacy with strong emphasis on data security. The US NIST’s documentationalso notes that “privacy-related risks may overlap with security, bias, and transparency,” highlighting the challenges of ensuring transparency and fairness without compromising privacy.
Overall, the principle of privacy is well agreed upon - all considered academic, government, and industry sources note it as essential. However, it is worth noting that there are varied descriptions of how privacy should be considered amongst industry sources.
Safety
Safety of an AI system is focused on ensuring that it does not harm people, environments, and societies. The need for safety to prevent harm is clear in a range of applications, from mental health chatbots to autonomous driving systems.
All considered literature sources discussed safety, to varying degrees. One workconsidered the principles of robustness and safety together - arguing that a robust system would prevent harm by preventing failures. Other works discussed safety separately, with one noting the need for real-time protectionsto mitigate safety issues should an AI fail.
Industry also broadly agrees that safety is a critical issue. Metaand Microsoftboth group safety with either robustness or reliability in their frameworks. Guidelines presented by Samsung do not explicitly name safety, but do discuss limiting potential harm to humans, environments and ecosystems. Google states that AI should “be built and tested for safety”. Interestingly, neither IBM nor Amazon include safety in their guidelines.
All government and political bodies discuss safety. The US’s NIST names safety as an attribute of RAI, while guidelines from China, Japan, and the UKalso discuss safety or minimisation of harm. Australia’s CSIROaddresses safety under the principle of “Do no harm”, stating that civilian AI systems should be designed to minimise harm and negative outcomes. Government and political bodies from India, the EU, and Saudi Arabiaall group safety with robustness or reliability, again highlighting a link between these two pillars.
Overall, safety is widely agreed to be critical for RAI. Interestingly, many sources grouped safety with robustness or reliability. However, an AI model could be robust without being safe if it is designed to do harm. As such, we argue that safety should be considered as a discrete pillar of RAI.
Summary
Our review of current academic, industrial, and government frameworks and policies on RAI has revealed that there are six critical pillars for RAI: Fairness, Robustness, Transparency, Accountabiilty, Privacy, and Safety.
Interestingly, we found that government and political bodies are leading the push for responsible AI. In all instances, government and political bodies had substantial guidelines in place for the development of responsible and ethical AI. Additionally, government bodies from a diverse group of regions all agreed on these six fundamental principles of RAI.
Overall, this section has highlighted that these six principles are critical in ensuring that AI is developed, deployed, and used responsibly. However, a significant question remains: how do we address these pillars to create responsible AI systems? In the next section, we propose an answer: the use of XAI to underpin truly responsible AI.
Explainable is Responsible
In this section, we investigate the use of explainability to enable responsibility. In particular, we have presented strong evidence illustrating that explainability is foundational for each of the six pillars of responsibility identified in the previous section. Through our analysis, we determine that XAI is foundational for RAI; it would not be possible for RAI to exist without it.
Fairness
In order to achieve fairness in AI systems, developers must first be able to identify when bias or discrimination occur so that the problem can be rectified. Explainability techniques are highly suited to revealing a model’s biases, both at global and local levels. Consider a home loan example: if explainability tools such as SHAP or LIME are applied and reveal that a person’s gender was the largest contributor towards an AI system deciding to decline their home loan application, then this reveals a clear bias that must be corrected. This intuition has led to explainability being broadly considered in the literature for promoting development of fair AI systems.
One recent work examined the fairness of AI models including RF, KNN, and DTs trained to predict recidivism in juveniles convicted of a crime. Their analysis used LIME scoring alongside accuracy and fairness metrics, comparing models trained with only static inputs (those that cannot be changed, such as sex and nationality), only dynamic inputs (those that can be changed, such as social support and school performance), and a combination of both. Using LIME scoring, they identified that AI models made decisions largely based on static variables where these were available; the models were learning to be biased. Further analysis showed that disparity metrics also increased where static variables were included - specifically, the likelihood of someone being falsely identified as recidivist was significantly higher amongst men and foreigners. A trade-off between accuracy and fairness was identified, with the feature encoding strategy of learning fair representations (LFR) identified as a candidate for mitigating bias. In this instance, the explanations provided by LIME were essential in revealing the bias of all developed AI models, and thus enabling bias mitigation strategies to be applied and assessed.
In the medical domain, one recent work proposed a framework based on their custom XAI tool for auditing medical diagnostics systems. Their proposed XAI tool was inspired by both LIME and anchors, generating a local `neighbourhood’ of pertubations, and thereafter extracting rules to provide as output explanations. The overall framework initially calculates disparity scores for different groups (including groups based on sex, ethnicity, and insurance status) based on the Wasserstein distance between predictions and the ground truth. Their custom XAI tool is then utilised to explain system misclassifications. They suggest that this allows for assessment of fairness at two stages: firstly, by visualising the disparity scores and assessing whether any one group suffers from higher disparity than another, and secondly, but inspecting local explanations of misclassifications to assess whether the model demonstrated biases in making those predictions. Overall, their proposed system supports fairness in an auditable way.
In another recent work, an interactive and explainable tool was developed for loan decision making. The prototype was based on a logistic regression model with interfaces that explain feature importances at global and local levels based on model weights, as well as enabling comparison to similar applications. The interactive component of the prototype allows non-technical end users to mark a decision as fair' or
not fair’, and adjust weightings on different features to see if a decision changes; these labels and suggested weight changes were then utilised to adjust the model. Prior fair' and
not fair’ labels are incorporated into explanations; users can see how many times the model’s predictions were labelled as fair' and
not fair’ overall, as well as seeing whether similar applications were deemed to be fairly assessed by the model or not. Additionally, when the suggested weight changes were incorporated into the model, it was found to increase fairness based on disparate impact (DI) assessment. Overall, this is an interesting approach that shows promise in incorporating human feedback from end-users to improve the fairness of models, however the authors did caution that there is always a risk of people introducing their own biases to the model.
Another work that sought to enable human `fairness debugging’ through a proposed system called Gopher, which seeks to quantify and explain model bias, utilising an approach of data subset removal and fairness metrics to analyse which types of data are causing biases and make suggestions on how to correct these biases. Explanations are made in the form of lists of patterns (groups of one or more co-occurring features) responsible for biases, with additional explanations provided as to possible updates or data removals that would reduce the bias of the model. The effectiveness of this approach was demonstrated on multiple well-known databases.
Overall, the use of explainability for the purpose of supporting fairness has become established in the literature. Several works used explanation tools like LIME and SHAP to inspect whether particular features are being unfairly emphasised by a model. Meanwhile, several works propose systems that instead assess and explain fairness to developers and end-users, with the intention of receiving feedback and iterating the model to improve fairness. It is clear from the literature that XAI strongly supports the fairness principle of RAI.
Robustness
Robustness has been a key focus for many works utilising explainable AI. Explainability can help to reveal whether a model behaves consistently with similar inputs, as well as being robust against adversarial attacks.
In one recent work, a novel explainability technique entitled Similarity Difference and Uniqueness (SIDU) was proposed for producing heatmap explanations of image data that are robust against adversarial attacks. SIDU extracts the final layer of a CNN and calculates similarity and difference masks relatingto different feature applications. These are then fused to generate a final heatmap. In their experiments, SIDU produced explanations that were strongly aligned with human-grounded evaluation via eye tracking, outperforming prevalent literature models including Grad-CAM. To assess robustness, noise was introduced to input images, with CNN model predictions then explained using SIDU and competing literature models. SIDU explanations remained consistent as noise levels increased, while Grad-CAM explanations rapidly declined with increased noise. Their work demonstrates that explanations can be used to assess whether a model is robust against noise, and that explainability tools themselves can be enhanced to improve robustness.
Another recent work examined the relationship between robustness and explainability from a different angle, exploring whether robust models are inherently explainable. Their study used clean and adversarial data to train models for improved robustness, as well as training equivalent non-robust models with only clean data. They then examined how closely salience maps produced by the models matched target salience maps. Across two open-access databases, it was shown that salience maps for the robust models were more closely aligned with target salience maps than those of the non-robust models. This indicates that models designed to be robust have more inherently interpretable salience maps, potentially eliminating the need for post-hoc heatmap explanations where robustness is achieved.
Explainability has also been used to quantify robustness, with one study proposing a robustness metric based on counterfactual explanations. Their proposed Counterfactual Explanation-based Robustness Score (CERScore) operates on the principal that when comparing two AI models, the model where counterfactuals are further away on average from the input instances is the more robust model. CERScore is also model-agnostic and can be applied with no knowledge of the underyling architecture, a significant advantage over previous benchmark robustness metrics. Through a series of experiments on established AI models, the CERScore authors demonstrated that their proposed metric produced robustness scores consistent with literature benchmarks, indicating that explainability can be used to quantify robustness.
XAI has also been used to fine-tune models to improve their robustness. In one recent study, a DNN model was trained to identify malware using adversarial training and XAI-based fine tuning. Following initial adversarial training, SHAP scores were calculated for the considered input features. A new database was then developed using input features and their corresponding SHAP scores to create a second adversarial database. This was then utilised to train the final DNN model. Testing showed that the XAI-based fine-tuning approach improved the accuracy of the model on unseen data, compared to using solely traditional adversarial training approaches.
Overall, recent literature has demonstrated that explainability can be utilised both to quantify robustness and to provide robustness. Both of these areas of robustness are critical to RAI. Quantification of robustness is a useful metric for RAI, as it allows comparison between candidate models. Additionally, the use of XAI to make models that are more robust is essential to RAI, as it supports development of models are resilient against adversarial attacks and invalid inputs.
Transparency
Transparency is perhaps the most self-evident characteristic of RAI that XAI can provide; the key purpose of XAI is to open the black-box of AI to improve transparency and comprehensibility. To achieve transparency, models must provide explanations that are interpretable and understandable to end-users. Transparency may include clarifying the inner workings of a black-box model, or explaining how a model made a decision in a way that users find acceptable and meaningful.
Several recent studies have sought to understand whether XAI explanations are transparent, interpretable, or understandable to end-users. In one such study, a counterfactual-based explanation strategy was developed to explain decisions made by a chest X-ray CNN classifier to expert radiologists. To assess the transparency of their approach, they utilised survey questions and free-text responses to assess whether explanations improved the understandability and trust in their proposed XAI system, compared to no explanation, saliency maps, and cycleGAN. Based on a 5-point Likert scale, experts rated the counterfactual explanations the highest for understandability and justifiability. Additionally, all explainability tools trialled were rated significantly higher for understability than the no-explanation case. Several free-text comments also verified that the experts had a better understanding of the AI model following counterfactual explanation.
In another study, text-based explanations were generated by analysing the decision pathways of a DT model trained to assess behavioural anomalies and cognitive status in a smart home residential care setting. Clinicians were asked to complete a Likert scale survey to rate their experience with the provided explanations. Questions about transparency were rated highly, with all clinicians agreeing that explanations were easily understandable and essential to understanding how the model classified anomalies for individual patients. Most clinicians reported that the explanations would assist them in determining whether assessments made by the model were correct.
In a study focusing on the education sector, a RF model was developed to make decisions on admissions to graduate school, with the decisions then explained using LIME, local SHAP, global SHAP, and PDP. University students with a range of AI experience were then surveyed using a Likert scale approach to determine whether explanations improved the understandability of the model. PDP and LIME were both found to significantly improve understandability amongst both AI novices and students with some AI experience. SHAP showed some improvement in understandability, but to a lesser extent. However, it is unclear which graphical format was used to present SHAP scores, so the chosen method may have contributed to the lower ranking.
Another recent study sought to understand what level of transparency gave users the most confidence in an autonomous vehicle’s driving decisions. They developed a confidence metric derived from case-based reasoning, which utilised prior situations to determine an autonomous vehicle’s confidence in a current situation. The model’s confidence in a given situationwas presented to a non-expert cohort, using several different text structures to explain the confidence score. Survey participants were asked to rank which text structure they found most useful. Results of the survey found that the best-perceived explanation included the confidence level along with general information about prior situations. Explanations that provided confidence in addition to current or future situation information were also well received. The worst-ranked explanation was one that provided the confidence level only, with no transparency about how that confidence level was reached. These results indicate that transparency via text explanations of model confidence were critical to improving acceptability of the proposed AI approach.
Overall, recent literature that has sought to quantify transparency, understandability, or interpretability of AI systems have indicated a strong link between explanations and transparency. A wide range of explanation approaches have been examined by the literature, including text-based explanations, visual explanations, and feature-based explanations; each has been shown to improve transparency to some degree. Explanations have also been shown to support transparency for a wide range of users, with varied levels of domain and AI expertise.
Accountability
Accountability requires AI models to justify their decisions to ensure that they can be audited and assessed for compliance with legal and governance requirements. This principle is related to transparency, as models must be able to explain their decisions to be accountable.
One sector where accountability is critical is finance. In one recent study, an auditable pipeline based on various XAI techniques was proposed to provide transparent and accountable credit scoring. The authors note that logistic regression is popular in credit risk scoring due to the inherent explainability of these models that has lead to acceptance amongst regulators, however note that logistic regression does not perform as strongly as advanced AI techniques for this task. To address this issue, they proposed a pipeline that begins with global explanations using feature permutation importance, followed by assessment of the most important features with PDP and ICE plots. From there, local instances are then able to be explained using LIME, SHAP, and another variant of SHAP. They indicate that this pipeline explains advanced AI algorithms in a manner that satisfies prevalent regulatory and legal requirements for transparency, accountability, and privacy.
There is also a need for accountable AI systems to establish liability in legal cases. One recent work identifies XAI as highly suitable for providing a `forensic toolset’ that can help establish liability of AI systems in a range of settings. In particular, they illustrate a case study where an autonomous vehicle changes lanes to avoid a collision with car A, ultimately causing a collision with car B. The authors demonstrate that the use of XAI tools can help establish why the AI model made the decision, thus establishing factual and legal causation as is required by many common law systems. Ultimately, this enables legal systems to hold AI systems accountable for their actions, and thereafter to determine whether the AI system in question or another party is at fault for an accident. It is suggested that LIME, ICE, and PDP are critical XAI tools for understanding the global model, while SHAP is essential to understand individual decisions; this is consistent with suggestions made by the credit scoring study discussed above.
In the medical sector, an auditable pipeline was recently developed for prediction of oxygen requirement in COVID-19 patients, based on GradCAM explanations of features identified in chest x-ray input images. Their technique included the development of model-derived atlases for similar feature patches and similar overall predictions, based on areas that GradCAM highlighted as important. When a prediction is made, the clinician is presented with 8 similar patches from the atlas alongside the test patch, a GradCAM heatmap of the overall x-ray, a table comparing the test x-ray with several similar x-rays both visually and with feature-based similarity scores, and finally a table showing the model’s confidence in each feature it detects. Clinicians can also select feature labels of interest to receive further characterization of the given disease. The use of example-based explanations and numerical scores for confidence makes the decisions made by AI highly auditable and accountable, both to expert clinicians and regulatory bodies.
Overall, it is clear that accountability is critical in a wide range of sectors to ensure that AI models can be held to the same standards as humans. The literature indicates that XAI can provide an appropriate level of accountability and auditability across several key sectors, which contributes significantly towards the development of RAI systems.
Privacy
Privacy is critical for AI systems, as no sensitive information should be accessible to users of an AI system. Achieving privacy while maintaining transparency has previously been identified as a key challenge in developing responsible AI systems.
In standard use, most techniques for global and local explanation are inherently privacy-preserving as they do not explicitly reveal identifiable information about data in the training set. However, limited recent studies have identified that targeted adversarial attacks on CAM-based XAI models have a nominally increased chance of success in breaching privacy through training image reconstructionor model duplicationcompared to equivalent models without explanations.
Critically, these attacks were successful against both explainable and non-explainable models; thus data protection and privacy-preserving techniques are essential for developing responsible AI. However, such techniques make AI more opaque and thus are in conflict with transparency and accountability principles for RAI. This brings us full-circle back to XAI: several recent works have demonstrated that XAI techniques continue to provide robust and meaningful explanations where data protection and privacy-preserving techniques have been applied, thus supporting privacy to be incorporated into RAI systems without compromising on other RAI principles.
In one such work, federated learning was applied to develop a model for heart arrhythmia classification from electrocardiogram (ECG) signals before explainability was applied. Federated learning preserves privacy by enabling individual insitutions to train local models, with these models then shared to a central node for aggregation. The aggregated model is then returned to institutions for use. This enables the development of more robust models without direct data sharing, and thus protects against data inversion attacks. In the ECG study, federated learning was shown to improve accuracy in detecting key ECG features and classifying arrhythmia compared to local models, even where signals were noisy. Explanations were provided using GradCAM, providing heatmaps of the most important regions in a signal. Model duplication is still feasible, however reconstruction of local models is unlikely if weight sharing is protected.
In another work, swarm learning was applied to develop a model for cancer diagnosis from histopathology slides. Swarm learning is similar to federated learning in that models are trained locally first, however aggregation of models occurs by sharing trained local models in a peer-to-peer structure; this distributed and decentralized architecture ensures that the failure of a single node does not lead to failure of the entire network. The cancer histopathology work demonstrated that this enabled the development of swarm models that exceeded the performance of local models across all criteria of interest. To explain model predictions, heatmaps were generated to illustrate regions of interest that lead to a prediction. As with federated learning, this approach is robust against data inversion attacks as the final aggregate model has no knowledge of training data. The decentralized nature of swarm learning also ensures higher robustness in the event of a node failure.
Another approach to privacy preservation is the notion of differential privacy, where data is anonymized by injecting noise. One recent study explored whether this approach had a significant impact on SHAP values, using various open-access databases, data pertubation methods, and AI models. Their findings showed that SHAP values were minimally affected by data protection techniques. Simple linear models were more affected than more advanced models such as support vector regression, however overall still provided meaningful explanations. Based on their findings, the authors concluded that data protection and explanation are not mutually exclusive.
Differential privacy was also considered in an image context by a recent work that used image compression to remove spatial and edge information in order to privatise data prior to training. Their work considered three image-based use cases: chest x-ray classification, cervix type classification, and glaucoma detection. Their work indicated that high accuracy could still be achieved with compressed images. Salience map explanations were also able to provide meaningful information about relevant areas of an image without access to the original uncompressed image. Overall, this approach improves privacy as original images are not used for model training.
Overall, XAI enables privacy in an indirect manner. AI systems are vulnerable to many adversarial attacks, and privacy-preserving techniques can compromise RAI principles such as transparency and accountability. XAI thus supports privacy by enabling privacy-preserving learning and data protection techniques to be implemented without violating the other key requirements of RAI.
Safety
Safety of humans, environments and societies is critical for AI systems, particularly in high-risk settings such as autonomous driving and healthcare. As XAI helps reveal the inner workings of an AI model, it can similarly assist in revealing safety risks. Additionally, XAI systems can be developed to sit above existing AI systems to provide safety in terms of detecting network intrusion or other attacks, protecting the overall pipeline from adversarial attacks and thus supporting RAI.
In one recent work, the need for XAI in ensuring safety in healthcare systems was examined through a case study on predicting when a patient should be extubated from mechanical ventilation in intensive care. In this application, safety is of the utmost importance as early extubation can lead to emergency re-intubation, while late extubation increases risk of complications including pneumonia. Their work identified that XAI techniques are useful for verifying safety in several stages of AI development. In particular, they highlight that feature importance and counterfactual example methods support safety in AI. Feature importances can allow clinicians to assess whether the model utilised features that meet clinical expectations, improving confidence that the model makes decisions that will benefit the patient. Meanwhile, counterfactual explanations can be used to determine how many features would need to change (and by how much) in order to change the decision. This allows clinicians to evaluate the confidence of the model in its decision, which in turn supports patient safety as clinicians can make informed decisions about whether the model can be trusted in a particular instance or whether further investigation is needed.
In the autonomous driving domain, XAI has been applied in one recent work to compare the performance of three AI models classifying traffic signs where camera failures such as broken lens, ice coverage, or dead pixels occur. Their study evaluated the performance decreases for several types of lens failure, identifying that the model based on AlexNetwas the strongest performer across most lens failure modes. To understand this further, LIME heatmaps were applied to traffic sign classification outputs. This illustrated that predictions made by the AlexNet model used features distributed over a larger section of the inputted image compared to the other models. The authors hypothesise that this leads to the higher camera failure tolerance of AlexNet, as the features of interest are less likely to be obscured by the defect if they are widely spread throughout the image. In this context, XAI supports safe autonomous driving by enabling identification of the traffic sign model that performs most reliably under adverse conditions.
Another recent work reviewed the field of goal-driven AI systems (GDAIs), which include robots and other agents operating independently to fulfil their individual goals. In particular, they examined the need for explainable GDAIs in safety-critical applications to ensure that GDAIs do not cause harm to humans or environments. Their work identified that communication of actions via explanations such as CAM, SHAP, and textual description are essential for communicating the decisions of GDAIs. Through their analysis, the authors identify explanations support human-computer interaction, and thus support safety by enabling early detection of problems and thus early intervention to prevent failures, which is particularly crucial for safety-critical applications.
XAI can also be used to create a layer of protection against adversarial attacks on digital systems that employ AI. In one recent study, explainability techniques were utilised as part of an intrusion detection system for recognising attacks on Internet of Things (IoT) networks. Using a pipeline including rule-based explanations, LIME, and SHAP, the proposed intrusion detection system accurately detects intruder or compromised nodes on the IoT network, with global and local explanations provided to show how the decision was made so that human experts can decide on a response path. The use of XAI in this context helps to protect any AI models operating on the IoT network data from attack, as rapid and interpretable detection of intruders greatly reduces the risk of the IoT network being poisoned by malicious data injection.
Overall, explainability strongly supports safety across diverse AI applications. XAI techniques improve safety by enabling human experts to review decisions and decide whether they were made reasonably. Additionally, XAI can be applied on top of other AI systems to detect security risks such as network intrusions, reducing the risk of adversarial attacks. XAI is thus an important tool for ensuring that the RAI principle of safety is met.
Lessons Learned
Through our exploration of the literature, it was found that XAI is foundational to each key pillar of RAI. The relationship of XAI to the principles of transparency and accountability was found to be straightforward. The primary intention of explanation is to make models more interpretable, and several works have established that users perceive XAI to be more transparent, understandable, and trustworthy. Transparency is also the first step towards accountability, where AI models need to be able to explain and justify their decisions to relevant stakeholders. Works to date have established that XAI is essential in making AI accountable and, where necessary, liable for its actions in critical sectors from autonomous driving to healthcare.
Accountability is also connected to the principles of fairness and safety. In our exploration of the literature, it was found that XAI tools can highlight societal biases learned from historic data. Human-in-the-loop fairness debugging was also discussed, with human users able to provide feedback on potential biases based on model explanations, as a form of fairness accountability. Human-in-the-loop debugging was also considered in the safety context, with explanations provided by goal-driven AIs to human safety auditors. In turn, humans could provide feedback to the AI models that would improve the safety and failure tolerance of the systems. Similarly, safety-critical applications in healthand autonomous drivingwere supported by XAI through the provision of explanations to domain experts, who could then assess how and why the model is making a decision and determine whether that decision is appropriate.
Safety is also tied to the principle of robustness; a model that is less likely to fail, is less likely to cause harm in safety-critical applications. XAI was found to support the RAI principle of robustness in several direct and indirect ways. In particular, XAI has been used quantify robustness, enable robustness comparison between models, and support fine-tuning of models to enhance robustness. Interestingly, one study also identified that robust models have a higher degree of inherent explainability, indicating a strong relationship between these principles.
In our analysis of XAI robustness literature, one study highlighted that certain explanations remain accurate in the face of adversarial attacks that utilise perturbed inputs to compromise a model. The resilience of XAI to adversaries was considered by several works seeking to improve privacy. In some works, it was found that XAI could still successfully explain decisions where user privacy was protected by perturbing the dataset such that adversarial attacks would not be able to unveil genuine and identifiable information. Another key approach for preserving privacy was distributed learning, both in federatedand swarm learning structures, where local models are trained on private data, shared securely, and then aggregated into a final model for the end-user. In this context, XAI supported privacy indirectly by enabling models to continue meeting other RAI requirements whilst using an otherwise opaque method of learning.
In this section, we identified that research into XAI and RAI is an active field that remains in its infancy. The works highlighted have firmly established that XAI and RAI are inseparable concepts, however much remains to be explored in how best to utilise XAI to create truly responsible AI systems. Notably, relatively few works sought to develop frameworks or metrics for assessing the quality of explanations as they pertain to AI responsibility. Additionally, many XAI works to date considered only a subset of the six principles of responsible AI. Thus, there is still a need to create AI systems that are truly responsible in all attributes. XAI for RAI is a significant and growing field, and one which remains open for further exploration.
Use Cases
Having now established that XAI is foundational to RAI, this section presents several short case studies that illustrate how explainability can be utilised to create responsible AI. In particular, we present case studies in content generation, healthcare, and transport - domains where responsibility is essential to ensure that AI acts for the benefit of humanity and minimising risk of harm.
Generative AI
Generative AI (GAI) has recently become a prevalent topic in the literature and media, largely due to the emergence of large-language generative models such as ChatGPT. GAI has previously been found to exhibit biases, produce disinformationor misleading information. These issues are barriers to GAI meeting RAI requirements, and can pose substantial harm to users.
Explainable AI could be leveraged to make GAI systems more responsible. Consider a user who asks a GAI model a question about clinical depression. The model might provide information about symptoms and treatment paths, however without explanation the user cannot ascertain that the information is correct. XAI could be used to explain GAI outputs by providing local explanations that highlight how it understood the prompt, similar to previous research that used LIME for text analysis tasks. This would allow users to assess whether their query has been appropriately understood by the GAI model.
In terms of the generated output, text-based explanations where the GAI model generates an output and an explanation of how it chose this output are suitable. This approach has been highlighted by one early work in the GAI/XAI space. Case-based reasoning can also be used to evaluate the similarity between the users prompts, previous prompts, and legitimate sources to assign confidence weightings to different sections of its generated output. For example, a GAI model responding to a question about clinical depression may have higher confidence in some symptoms than others based on its training set, and this could be highlighted using colour coding - similar to a previous approach utilised in decision-making systems. Additionally, example-based approaches could be utilised by GAI models to effectively cite their sources. Example-based approaches have previously been utilised in healthcare applications such as chest x-ray assessment to provide examples of similar chest x-rays and their diagnoses. In the GAI context, example-based explainability could highlight relevant excerpts from the literature - in our clinical depression example, the GAI model could list five sources that describe the symptom of `fatigue’ when a user hovers over the word.
Having GAI models explain their outputs supports responsibility across all key pillars. For the user, explanation of prompt understanding and output generation provides transparency that supports them in identifying whether information is correct. This can enhance user safety and interpretation in many settings. From the developer perspective, explanations make the model auditable for RAI attributes so that it can be held accountable for its actions and continuously improved. For example, explanations of prompts would allow model auditors to phrase a question in many different ways, assessing model robustness to phrasing changes. Additionally, auditors could examine explanations of prompts and outputs for signs of unfair bias, dangerous information or advice, and privacy breaches such as reconstructing sensitive data from the training set. Using XAI to ensure transparency and accountability in GAI can thus support iterative development to enhance the fairness, privacy, safety, and robustness of these models.
Overall, GAI has significant potential to cause harm if not developed responsibly. In this case study, we have shown how XAI can serve as the foundation for responsible GAIs that better serve humanity. This field is novel with few works published to date, offering much opportunity for future research.
Medical Diagnostics
The field of medical diagnostics is one where AI holds much potential. In some scenarios, AI has been shown to outperform expert clinicians, partly due to their ability to learn from large datasets. Responsible AI development is critical in healthcare due to the sensitive nature of the underlying data, and the potential for significant harm to humans if incorrect decisions are made.
One potential source for harm in medical AI models arises from historic databases reflecting societal biases. Without caution, AI models can learn the same biases and thus make potentially harmful decisions. Consider the case of attention-deficit/hyperactivity disorder (ADHD). Due to societal biases, ADHD has historically been underdiagnosed in women and racial minorities. ADHD diagnosis with AI is an active field of research that has shown promising results, however a recent work cautioned that certain inputs can introduce unfair bias into models. XAI can assist in ensuring fairness in such models, with global and local explanations helping to reveal if the model places weight on parameters that perpetuate societal biases. For feature-based data types, explanations such as counterfactuals, SHAP, and LIME are strong candidates, while CAM would be suitable for image data.
Similarly, model robustness can be assessed using XAI techniques. By quantifying how significantly the data would need to change for the prediction to change using a technique such as CERSCore, the robustness of a model can be assessed. This also supports fairness and safety, as robust models will not make decisions based on one or two excessively weighted features.
Global and local explanations also make the model inherently more transparent, and support accountability. Clinicians and regulatory bodies can review the decisions made by AI models to ensure that the model operates in accordance with clinical knowledge and legal requirements. For individual patients, clinicians can utilise local explanations to understand the confidence of the model, and thus make assessment on the correctness of the decision. This also improves patient safety, as the interaction between AI and clinicians further supports accurate ADHD diagnosis.
In the medical context, the concepts of safety and privacy are intertwined due to the sensitive nature of medical data. Federated learning and swarm learning are of interest in the medical spaceas these methods eliminate the need for data sharing between institutions; however, distributed learning makes models more opaque. Fortunately, XAI still provides robust, transparent and meaningful explanations where distributed learning has been utilised - enabling privacy-preserving training to be implemented without compromising other responsibility requirements.
Overall, XAI is critical for RAI in healthcare, due to the direct impact of AI decisions on human life. In this case study, we have illustrated how XAI can be utilised to develop an RAI system for ADHD diagnosis. The concepts discussed here are applicable to many other diagnostic use cases.
Autonomous Vehicles
Autonomous vehicles (AVs), vessels and aircraft pose significant risk to human life if not developed responsibly. AI systems in this domain must be able to make rapid decisions, and poor decisions can lead to injury or loss of life - both for passengers and bystanders.
XAI is essential for ensuring that AVs are piloted by RAIs. Transparency about decision making on the road can help to support user trust, and enable the human driver to regain manual control if needed. For example, if an AV speeds up after seeing a new speed limit sign, this decision could be explained to the user by showing the sign and decision, and highlighting the regions that lead to the decision using an approach such as CAM. If the decision is incorrect, the user could make manual speed adjustment and report the error to the car manufacturer. Similarly, feature-based explanations of speed changes based on weather conditions using LIME or a similar method would support the user in assessing the suitability of the decision made.
This transparency makes the AV accountable to the user and to car manufacturers. XAI can further ensure accountability when events such as collisions resulting in injury occur, as explanations of how and why parameters were used to make a decision can aid in determining whether the AI model acted reasonably and in line with legal requirements.
Explanations used to ensure transparency and accountability also support robustness and safety. Users can make informed decisions about whether the AV has made a good choice, and feedback can be provided to vehicle manufacturers for iterative improvement to enhance robustness and safety. Legal accountability further encourages manufacturers to ensure that their systems are resilient and safe. During the development stage, XAI can also be utilised to compare the robustness of AI models, to identify the system that makes the safest decisions in various contexts.
Fairness in the AV context has primarily been considered in terms of the Moral Machine Experiment (MME), which placed humans in the metaphorical driver’s seat of an AV that was guaranteed to collide with someone - and asked them to choose which person or group of people the car should hit. This experiment revealed human preferences contain biases based on attributes such as sex, age, and societal status. Thus, AVs are at risk of inheriting biases that could put life at risk. While the MME presents an extreme case, it is clear that there is a need to explain the decisions made by AVs - both for legal accountability purposes in extreme events, and to ensure that we are not sharing the road with vehicles that are prejudiced. Explained decisions can be evaluated for any potential biases, and this in turn can be used to iteratively improve the fairness of systems.
The final attribute to consider for RAIs in autonomous driving is privacy. Large datasets are essential for ensuring that AI models for autonomous driving perform well, however many companies would be unwilling to share proprietary data. Swarm learning may be more acceptable to companies, as they could share local models in a peer-to-peer structure with only companies they choose. Another aspect of privacy is user privacy; users will have varying preferences regarding the sharing of sensitive information such as location. Fortunately, this can largely be addressed by implementing differential privacy techniques. XAI again supports privacy by ensuring that all other attributes of RAI continue to be met when privacy-preserving techniques are implemented.
Overall, the need for RAI to drive AVs is clear. AVs are making continuous and rapid decisions, and many of their decisions can mean life or death for passengers and bystanders. The transparency provided by XAI is essential for investigating decisions that AVs make to ensure that they operate robustly, safely, and fairly. This also enables AVs to be held accountable for their actions should accidents occur. XAI also responsible AVs by enabling privacy-preserving techniques to be implemented without compromising on transparency and accountability. In this case study, we further illustrate that XAI and RAI are inseparable concepts. When AI is behind the wheel, it is essential that XAI techniques are in the passenger seat.
Opportunities for Future Work
This review of the literature has clearly demonstrated that XAI is foundational to the development of RAI systems. However, this field remains in its infancy, with much opportunity for future research. In this section, we outline several key directions for future research in this critical domain.
Quantifying responsibility: One notable direction for future research is in quantifying responsibility to enable comparison between different AI systems. Some early works have proposed metrics for assessing attributes such as fairnessand robustness, however these have not been widely validated. Similarly, some works have sought to assess transparency by surveying users of XAI models, however each of these works used different surveys. A significant gap remains in terms of developing clear frameworks, guidelines, or metrics for assessing transparency. Similarly, metrics or methods for assessing accountability, privacy, and safety remain open research topics. Development of clear metrics or methods for quantifying responsibility would be a valuable addition to the literature. Validated strategies for quantifying responsibility could then be utilised to assess different XAI techniques to determine which are the most responsible, and subsequently develop new XAI techniques that enhance responsibility further.
Improving explanations: The field of XAI has been rapidly growing, however relatively few studies have sought to analyse whether the explanations provided by their proposed techniques are understandable and acceptable to humans users. These characteristics are essential in ensuring that XAI tools are contributing to responsibility. There remains a significant gap in the literature in determining how humans interpret data, and subsequently developing XAI systems that are in line with human understanding. The development of databases and methods for capturing human attention to data would greatly support research in this area.
Considering all pillars of responsibility: In this review, it is clear that most works on XAI for RAI focus on only one pillar of responsibility. Future research would benefit from utilising XAI techniques to improve responsibility in more than one area. The case studies presented have highlighted that this is feasible, particularly given certain XAI techniques and approaches can address many pillars of RAI simultaneously. While there certainly remains a need for ongoing research that seeks to improve responsibility with respect to certain pillars, developers of AI systems should seek to meet all pillars of RAI using XAI tools, as AI cannot be truly responsible if it addresses some pillars of RAI at the expense of others.
Responsible GAI: In terms of domain-specific research, our review indicates that explainability and responsibility of generative AI is under-explored. Given the prevalence and controversy surrounding generative AI systems such as ChatGPT in the literature and popular media, there is a significant research opportunity in implementing and continuing to develop XAI techniques that will lay the foundation for responsible GAI models that incorporate all pillars of RAI.
Overall, the field of XAI for RAI offers significant opportunities to future researchers. Research to date has illustrated that XAI is foundational to RAI, however there remains much research opportunity in areas such as quantifying responsibility, enhancing explanations to be more responsible, developing RAI models that address all pillars of responsibility, and finally applying XAI techniques to support development of RAI in rapidly evolving and exciting fields such as generative AI.
Conclusion
In this work, we conducted a scoping review of current XAI and RAI literature, and presented an evidence-based argument that XAI is foundational to RAI across all key pillars of responsibility. Our review began with XAI, exploring methods for explainability by design and post-hoc explainability. We identified approaches for feature-based, image-based, and text-based explanations at both local and global levels, with illustrative examples provided for each prevalent method.
We then examined the literature on RAI, considering sources from academic literature, government and political bodies, and large technology companies. Based on our findings, we identified six key pillars of responsibility: fairness, robustness, transparency, accountability, privacy, and safety. Interestingly, it was also identified that governments are leading academia and industry on the push for responsible AI, with diverse government and political bodies from across the globe having substantial guidelines in place for the development of responsible AI.
With the fields of XAI and RAI established, our review then explored the relationship between XAI and RAI. Based on the evidence found, we identified that XAI is foundational to all pillars of responsibility. XAI supports AI systems to be more transparent and accountable without compromising privacy, which in turn supports the principles of fairness, robustness, and safety. It is therefore clear that XAI is the essential foundation for truly responsible AI.
To further illustrate the importance of XAI for RAI in practical contexts, we present several timely use cases. We first showed how XAI is essential for developing responsible GAI models that can explain their understanding of a prompt and how they produced their outputs. Next, we turned to the medical domain and highlighted how XAI can be utilised to develop RAI diagnostics models that overcome historical biases and better serve humanity. Finally, we explored the use of XAI to ensure that autonomous vehicles act responsibly, highlighting how they can use XAI to communicate their decisions with passengers, manufacturers, and legal authorities.
Based on our thorough review of cutting-edge research on XAI as the foundational tool of RAI, we next presented a series of future research directions informed by our lessons learned. It was identified that this emerging field has much research potential, with opportunities present in developing methods and metrics to quantify responsibility, further improvements to XAI for RAI, development of systems that are responsible across all key pillars of RAI, and lastly in the application of XAI techniques to novel and exciting domains, including generative AI.
Bibliography
1@article{dwivedi2023,
2 year = {2023},
3 volume = {55},
4 pages = {1--33},
5 url = {https://doi.org/10.1145/3561048},
6 title = {{Explainable AI (XAI): Core Ideas, Techniques, and Solutions}},
7 publisher = {ACM},
8 number = {9},
9 month = {jan},
10 keywords = { interpretable AI, programming framework, software toolkits,Explainable artificial intelligence},
11 journal = {ACM Comput. Surv.},
12 issn = {0360-0300},
13 doi = {10.1145/3561048},
14 author = {Dwivedi, Rudresh and Dave, Devam and Naik, Het and Singhal, Smiti and Omer, Rana and Patel, Pankesh and Qian, Bin and Wen, Zhenyu and Shah, Tejal and Morgan, Graham and Ranjan, Rajiv},
15 address = {New York, NY, USA},
16 abstract = {As our dependence on intelligent machines continues to grow, so does the demand for more transparent and interpretable models. In addition, the ability to explain the model generally is now the gold standard for building trust and deployment of artificial intelligence systems in critical domains. Explainable artificial intelligence (XAI) aims to provide a suite of machine learning techniques that enable human users to understand, appropriately trust, and produce more explainable models. Selecting an appropriate approach for building an XAI-enabled application requires a clear understanding of the core ideas within XAI and the associated programming frameworks. We survey state-of-the-art programming techniques for XAI and present the different phases of XAI in a typical machine learning development process. We classify the various XAI approaches and, using this taxonomy, discuss the key differences among the existing XAI techniques. Furthermore, concrete examples are used to describe these techniques that are mapped to programming frameworks and software toolkits. It is the intention that this survey will help stakeholders in selecting the appropriate approaches, programming frameworks, and software toolkits by comparing them through the lens of the presented taxonomy.},
17}
18
19@article{Li2023,
20 year = {2023},
21 volume = {55},
22 title = {{Trustworthy ai: From principles to practices}},
23 publisher = {ACM New York, NY},
24 pages = {1--46},
25 number = {9},
26 journal = {ACM Computing Surveys},
27 issn = {0360-0300},
28 author = {Li, Bo and Qi, Peng and Liu, Bo and Di, Shuai and Liu, Jingen and Pei, Jiquan and Yi, Jinfeng and Zhou, Bowen},
29}
30
31@article{BarredoArrieta2020,
32 year = {2020},
33 volume = {58},
34 url = {https://www.sciencedirect.com/science/article/pii/S1566253519308103},
35 title = {{Explainable Artificial Intelligence (XAI): Concepts, taxonomies, opportunities and challenges toward responsible AI}},
36 pages = {82--115},
37 mendeley-groups = {Responsible AI survey},
38 keywords = {Accountability,Comprehensibility,Data Fusion,Deep Learning,Explainable Artificial Intelligence,Fairness,Interpretability,Machine Learning,Privacy,Responsible Artificial Intelligence,Transparency},
39 journal = {Information Fusion},
40 issn = {1566-2535},
41 doi = {https://doi.org/10.1016/j.inffus.2019.12.012},
42 author = {{Barredo Arrieta}, Alejandro and D{\'{i}}az-Rodr{\'{i}}guez, Natalia and {Del Ser}, Javier and Bennetot, Adrien and Tabik, Siham and Barbado, Alberto and Garcia, Salvador and Gil-Lopez, Sergio and Molina, Daniel and Benjamins, Richard and Chatila, Raja and Herrera, Francisco},
43 abstract = {In the last few years, Artificial Intelligence (AI) has achieved a notable momentum that, if harnessed appropriately, may deliver the best of expectations over many application sectors across the field. For this to occur shortly in Machine Learning, the entire community stands in front of the barrier of explainability, an inherent problem of the latest techniques brought by sub-symbolism (e.g. ensembles or Deep Neural Networks) that were not present in the last hype of AI (namely, expert systems and rule based models). Paradigms underlying this problem fall within the so-called eXplainable AI (XAI) field, which is widely acknowledged as a crucial feature for the practical deployment of AI models. The overview presented in this article examines the existing literature and contributions already done in the field of XAI, including a prospect toward what is yet to be reached. For this purpose we summarize previous efforts made to define explainability in Machine Learning, establishing a novel definition of explainable Machine Learning that covers such prior conceptual propositions with a major focus on the audience for which the explainability is sought. Departing from this definition, we propose and discuss about a taxonomy of recent contributions related to the explainability of different Machine Learning models, including those aimed at explaining Deep Learning methods for which a second dedicated taxonomy is built and examined in detail. This critical literature analysis serves as the motivating background for a series of challenges faced by XAI, such as the interesting crossroads of data fusion and explainability. Our prospects lead toward the concept of Responsible Artificial Intelligence, namely, a methodology for the large-scale implementation of AI methods in real organizations with fairness, model explainability and accountability at its core. Our ultimate goal is to provide newcomers to the field of XAI with a thorough taxonomy that can serve as reference material in order to stimulate future research advances, but also to encourage experts and professionals from other disciplines to embrace the benefits of AI in their activity sectors, without any prior bias for its lack of interpretability.},
44}
45
46@article{kaur2022,
47 year = {2022},
48 volume = {55},
49 url = {https://doi.org/10.1145/3491209},
50 title = {{Trustworthy Artificial Intelligence: A Review}},
51 publisher = {ACM},
52 pages = {1--38},
53 number = {2},
54 month = {jan},
55 mendeley-groups = {Responsible AI survey},
56 keywords = { Artificial intelligence, acceptance, accountability, explainability, explainable AI, fairness, machine learning, privacy, trustworthy AI,black-box problem},
57 journal = {ACM Comput. Surv.},
58 issn = {0360-0300},
59 doi = {10.1145/3491209},
60 author = {Kaur, Davinder and Uslu, Suleyman and Rittichier, Kaley J and Durresi, Arjan},
61 address = {New York, NY, USA},
62 abstract = {Artificial intelligence (AI) and algorithmic decision making are having a profound impact on our daily lives. These systems are vastly used in different high-stakes applications like healthcare, business, government, education, and justice, moving us toward a more algorithmic society. However, despite so many advantages of these systems, they sometimes directly or indirectly cause harm to the users and society. Therefore, it has become essential to make these systems safe, reliable, and trustworthy. Several requirements, such as fairness, explainability, accountability, reliability, and acceptance, have been proposed in this direction to make these systems trustworthy. This survey analyzes all of these different requirements through the lens of the literature. It provides an overview of different approaches that can help mitigate AI risks and increase trust and acceptance of the systems by utilizing the users and society. It also discusses existing strategies for validating and verifying these systems and the current standardization efforts for trustworthy AI. Finally, we present a holistic view of the recent advancements in trustworthy AI to help the interested researchers grasp the crucial facets of the topic efficiently and offer possible future research directions.},
63}
64
65@article{Anagnostou2022,
66 year = {2022},
67 volume = {24},
68 url = {https://doi.org/10.1007/s10676-022-09634-1},
69 title = {{Characteristics and challenges in the industries towards responsible AI: a systematic literature review}},
70 pages = {37},
71 number = {3},
72 journal = {Ethics and Information Technology},
73 issn = {1572-8439},
74 doi = {10.1007/s10676-022-09634-1},
75 author = {Anagnostou, Marianna and Karvounidou, Olga and Katritzidaki, Chrysovalantou and Kechagia, Christina and Melidou, Kyriaki and Mpeza, Eleni and Konstantinidis, Ioannis and Kapantai, Eleni and Berberidis, Christos and Magnisalis, Ioannis and Peristeras, Vassilios},
76 abstract = {Today humanity is in the midst of the massive expansion of new and fundamental technology, represented by advanced artificial intelligence (AI) systems. The ongoing revolution of these technologies and their profound impact across various sectors, has triggered discussions about the characteristics and values that should guide their use and development in a responsible manner. In this paper, we conduct a systematic literature review with the aim of pointing out existing challenges and required principles in AI-based systems in different industries. We discuss our findings and provide general recommendations to be considered during AI deployment in production. The results have shown many gaps and concerns towards responsible AI and integration of complex AI models in the industry that the research community could address.},
77}
78
79@article{Minh2022,
80 year = {2022},
81 volume = {55},
82 url = {https://doi.org/10.1007/s10462-021-10088-y},
83 title = {{Explainable artificial intelligence: a comprehensive review}},
84 pages = {3503--3568},
85 number = {5},
86 mendeley-groups = {Responsible AI survey},
87 journal = {Artificial Intelligence Review},
88 issn = {1573-7462},
89 doi = {10.1007/s10462-021-10088-y},
90 author = {Minh, Dang and Wang, H Xiang and Li, Y Fen and Nguyen, Tan N},
91 abstract = {Thanks to the exponential growth in computing power and vast amounts of data, artificial intelligence (AI) has witnessed remarkable developments in recent years, enabling it to be ubiquitously adopted in our daily lives. Even though AI-powered systems have brought competitive advantages, the black-box nature makes them lack transparency and prevents them from explaining their decisions. This issue has motivated the introduction of explainable artificial intelligence (XAI), which promotes AI algorithms that can show their internal process and explain how they made decisions. The number of XAI research has increased significantly in recent years, but there lacks a unified and comprehensive review of the latest XAI progress. This review aims to bridge the gap by discovering the critical perspectives of the rapidly growing body of research associated with XAI. After offering the readers a solid XAI background, we analyze and review various XAI methods, which are grouped into (i) pre-modeling explainability, (ii) interpretable model, and (iii) post-modeling explainability. We also pay attention to the current methods that dedicate to interpret and analyze deep learning methods. In addition, we systematically discuss various XAI challenges, such as the trade-off between the performance and the explainability, evaluation methods, security, and policy. Finally, we show the standard approaches that are leveraged to deal with the mentioned challenges.},
92}
93
94@article{Ashok2022,
95 year = {2022},
96 volume = {62},
97 url = {https://www.sciencedirect.com/science/article/pii/S0268401221001262},
98 title = {{Ethical framework for Artificial Intelligence and Digital technologies}},
99 pages = {102433},
100 mendeley-groups = {Responsible AI survey},
101 keywords = {Artificial Intelligence (AI) ethics,Digital ethics,Digital technologies and archetypes,Ontological framework,PRISMA,Systematic literature review},
102 journal = {International Journal of Information Management},
103 issn = {0268-4012},
104 doi = {https://doi.org/10.1016/j.ijinfomgt.2021.102433},
105 author = {Ashok, Mona and Madan, Rohit and Joha, Anton and Sivarajah, Uthayasankar},
106 abstract = {The use of Artificial Intelligence (AI) in Digital technologies (DT) is proliferating a profound socio-technical transformation. Governments and AI scholarship have endorsed key AI principles but lack direction at the implementation level. Through a systematic literature review of 59 papers, this paper contributes to the critical debate on the ethical use of AI in DTs beyond high-level AI principles. To our knowledge, this is the first paper that identifies 14 digital ethics implications for the use of AI in seven DT archetypes using a novel ontological framework (physical, cognitive, information, and governance). The paper presents key findings of the review and a conceptual model with twelve propositions highlighting the impact of digital ethics implications on societal impact, as moderated by DT archetypes and mediated by organisational impact. The implications of intelligibility, accountability, fairness, and autonomy (under the cognitive domain), and privacy (under the information domain) are the most widely discussed in our sample. Furthermore, ethical implications related to the governance domain are shown to be generally applicable for most DT archetypes. Implications under the physical domain are less prominent when it comes to AI diffusion with one exception (safety). The key findings and resulting conceptual model have academic and professional implications.},
107}
108
109@misc{IndiaAI2022,
110 year = {2022},
111 url = {https://indiaai.gov.in/responsible-ai/homepage},
112 title = {{Responsible AI Principles}},
113 author = {{India AI} and {National Association of Software and Service Companies (India)}},
114}
115
116@article{Ahmed2022,
117 year = {2022},
118 volume = {18},
119 title = {{From Artificial Intelligence to Explainable Artificial Intelligence in Industry 4.0: A Survey on What, How, and Where}},
120 pages = {5031--5042},
121 number = {8},
122 journal = {IEEE Transactions on Industrial Informatics},
123 issn = {1941-0050 VO - 18},
124 doi = {10.1109/TII.2022.3146552},
125 author = {Ahmed, I and Jeon, G and Piccialli, F},
126}
127
128@article{Saeed2023,
129 year = {2023},
130 volume = {263},
131 url = {https://www.sciencedirect.com/science/article/pii/S0950705123000230},
132 title = {{Explainable AI (XAI): A systematic meta-survey of current challenges and future opportunities}},
133 pages = {110273},
134 keywords = {Black-box,Deep learning,Explainable AI (XAI),Interpretable AI,Machine learning,Meta-survey,Responsible AI},
135 journal = {Knowledge-Based Systems},
136 issn = {0950-7051},
137 doi = {https://doi.org/10.1016/j.knosys.2023.110273},
138 author = {Saeed, Waddah and Omlin, Christian},
139 abstract = {The past decade has seen significant progress in artificial intelligence (AI), which has resulted in algorithms being adopted for resolving a variety of problems. However, this success has been met by increasing model complexity and employing black-box AI models that lack transparency. In response to this need, Explainable AI (XAI) has been proposed to make AI more transparent and thus advance the adoption of AI in critical domains. Although there are several reviews of XAI topics in the literature that have identified challenges and potential research directions of XAI, these challenges and research directions are scattered. This study, hence, presents a systematic meta-survey of challenges and future research directions in XAI organized in two themes: (1) general challenges and research directions of XAI and (2) challenges and research directions of XAI based on machine learning life cycle's phases: design, development, and deployment. We believe that our meta-survey contributes to XAI literature by providing a guide for future exploration in the XAI area.},
140}
141
142@article{Adadi2018,
143 year = {2018},
144 volume = {6},
145 title = {{Peeking Inside the Black-Box: A Survey on Explainable Artificial Intelligence (XAI)}},
146 pages = {52138--52160},
147 mendeley-groups = {Responsible AI survey},
148 journal = {IEEE Access},
149 issn = {2169-3536 VO - 6},
150 doi = {10.1109/ACCESS.2018.2870052},
151 author = {Adadi, A and Berrada, M},
152}
153
154@misc{Elsevier2023,
155 year = {2023},
156 urldate = {2023-02-02},
157 url = {https://www.scopus.com/},
158 title = {{Scopus}},
159 mendeley-groups = {Responsible AI survey},
160 author = {Elsevier},
161}
162
163@article{Bennetot2022,
164 year = {2022},
165 volume = {258},
166 url = {https://www.sciencedirect.com/science/article/pii/S0950705122010401},
167 title = {{Greybox XAI: A Neural-Symbolic learning framework to produce interpretable predictions for image classification}},
168 pages = {109947},
169 mendeley-groups = {Responsible AI survey},
170 keywords = {Compositional models,Computer vision,Deep learning,Explainable artificial intelligence,Neural-symbolic learning and reasoning,Part-based object classification},
171 journal = {Knowledge-Based Systems},
172 issn = {0950-7051},
173 doi = {https://doi.org/10.1016/j.knosys.2022.109947},
174 author = {Bennetot, Adrien and Franchi, Gianni and Ser, Javier Del and Chatila, Raja and D{\'{i}}az-Rodr{\'{i}}guez, Natalia},
175 abstract = {Although Deep Neural Networks (DNNs) have great generalization and prediction capabilities, their functioning does not allow a detailed explanation of their behavior. Opaque deep learning models are increasingly used to make important predictions in critical environments, and the danger is that they make and use predictions that cannot be justified or legitimized. Several eXplainable Artificial Intelligence (XAI) methods that separate explanations from machine learning models have emerged, but have shortcomings in faithfulness to the model actual functioning and robustness. As a result, there is a widespread agreement on the importance of endowing Deep Learning models with explanatory capabilities so that they can themselves provide an answer to why a particular prediction was made. First, we address the problem of the lack of universal criteria for XAI by formalizing what an explanation is. We also introduced a set of axioms and definitions to clarify XAI from a mathematical perspective. Finally, we present the Greybox XAI, a framework that composes a DNN and a transparent model thanks to the use of a symbolic Knowledge Base (KB). We extract a KB from the dataset and use it to train a transparent model (i.e., a logistic regression). An encoder–decoder architecture is trained on RGB images to produce an output similar to the KB used by the transparent model. Once the two models are trained independently, they are used compositionally to form an explainable predictive model. We show how this new architecture is accurate and explainable in several datasets.},
176}
177
178@article{Obeid2020,
179 year = {2020},
180 volume = {27},
181 url = {https://doi.org/10.1093/jamia/ocaa105},
182 title = {{An artificial intelligence approach to COVID-19 infection risk assessment in virtual visits: A case report}},
183 pages = {1321--1325},
184 number = {8},
185 month = {aug},
186 mendeley-groups = {Responsible AI survey},
187 journal = {Journal of the American Medical Informatics Association},
188 issn = {1527-974X},
189 doi = {10.1093/jamia/ocaa105},
190 author = {Obeid, Jihad S and Davis, Matthew and Turner, Matthew and Meystre, Stephane M and Heider, Paul M and O'Bryan, Edward C and Lenert, Leslie A},
191 abstract = {In an effort to improve the efficiency of computer algorithms applied to screening for coronavirus disease 2019 (COVID-19) testing, we used natural language processing and artificial intelligence–based methods with unstructured patient data collected through telehealth visits.After segmenting and parsing documents, we conducted analysis of overrepresented words in patient symptoms. We then developed a word embedding–based convolutional neural network for predicting COVID-19 test results based on patients' self-reported symptoms.Text analytics revealed that concepts such as smell and taste were more prevalent than expected in patients testing positive. As a result, screening algorithms were adapted to include these symptoms. The deep learning model yielded an area under the receiver-operating characteristic curve of 0.729 for predicting positive results and was subsequently applied to prioritize testing appointment scheduling.Informatics tools such as natural language processing and artificial intelligence methods can have significant clinical impacts when applied to data streams early in the development of clinical systems for outbreak response.},
192}
193
194@article{Jang2022,
195 year = {2022},
196 volume = {27},
197 url = {https://doi.org/10.1007/s10639-022-11120-6},
198 title = {{Practical early prediction of students' performance using machine learning and eXplainable AI}},
199 pages = {12855--12889},
200 number = {9},
201 mendeley-groups = {Responsible AI survey},
202 journal = {Education and Information Technologies},
203 issn = {1573-7608},
204 doi = {10.1007/s10639-022-11120-6},
205 author = {Jang, Yeonju and Choi, Seongyune and Jung, Heeseok and Kim, Hyeoncheol},
206 abstract = {Predicting students' performance in advance could help assist the learning process; if “at-risk” students can be identified early on, educators can provide them with the necessary educational support. Despite this potential advantage, the technology for predicting students' performance has not been widely used in education due to practical limitations. We propose a practical method to predict students' performance in the educational environment using machine learning and explainable artificial intelligence (XAI) techniques. We conducted qualitative research to ascertain the perspectives of educational stakeholders. Twelve people, including educators, parents of K-12 students, and policymakers, participated in a focus group interview. The initial practical features were chosen based on the participants' responses. Then, a final version of the practical features was selected through correlation analysis. In addition, to verify whether at-risk students could be distinguished using the selected features, we experimented with various machine learning algorithms: Logistic Regression, Decision Tree, Random Forest, Multi-Layer Perceptron, Support Vector Machine, XGBoost, LightGBM, VTC, and STC. As a result of the experiment, Logistic Regression showed the best overall performance. Finally, information intended to help each student was visually provided using the XAI technique.},
207}
208
209@article{Kostopoulos2021,
210 year = {2021},
211 volume = {9},
212 title = {{Interpretable Models for Early Prediction of Certification in MOOCs: A Case Study on a MOOC for Smart City Professionals}},
213 pages = {165881--165891},
214 mendeley-groups = {Responsible AI survey},
215 journal = {IEEE Access},
216 issn = {2169-3536 VO - 9},
217 doi = {10.1109/ACCESS.2021.3134787},
218 author = {Kostopoulos, G and Panagiotakopoulos, T and Kotsiantis, S and Pierrakeas, C and Kameas, A},
219}
220
221@article{Zhan2020,
222 year = {2020},
223 volume = {104},
224 url = {https://www.sciencedirect.com/science/article/pii/S0933365719301216},
225 title = {{Detecting potential signals of adverse drug events from prescription data}},
226 pages = {101839},
227 mendeley-groups = {Responsible AI survey},
228 keywords = {Adverse drug events (ADEs),Case-crossover,Logistic regression,Prescription data},
229 journal = {Artificial Intelligence in Medicine},
230 issn = {0933-3657},
231 doi = {https://doi.org/10.1016/j.artmed.2020.101839},
232 author = {Zhan, Chen and Roughead, Elizabeth and Liu, Lin and Pratt, Nicole and Li, Jiuyong},
233 abstract = {Adverse drug events (ADEs) may occur and lead to severe consequences for the public, even though clinical trials are conducted in the stage of pre-market. Computational methods are still needed to fulfil the task of pharmacosurveillance. In post-market surveillance, the spontaneous reporting system (SRS) has been widely used to detect suspicious associations between medicines and ADEs. However, the passive mechanism of SRS leads to the hysteresis in ADE detection by SRS based methods, not mentioning the acknowledged problem of under-reporting and duplicate reporting in SRS. Therefore, there is a growing demand for other complementary methods utilising different types of healthcare data to assist with global pharmacosurveillance. Among those data sources, prescription data is of proved usefulness for pharmacosurveillance. However, few works have used prescription data for signalling ADEs. In this paper, we propose a data-driven method to discover medicines that are responsible for a given ADE purely from prescription data. Our method uses a logistic regression model to evaluate the associations between up to hundreds of suspected medicines and an ADE spontaneously and selects the medicines possessing the most significant associations via Lasso regularisation. To prepare data for training the logistic regression model, we adapt the design of the case-crossover study to construct case time and control time windows for the extraction of medicine use information. While the case time window can be readily determined, we propose several criteria to select the suitable control time windows providing the maximum power of comparisons. In order to address confounding situations, we have considered diverse factors in medicine utilisation in terms of the temporal effect of medicine and the frequency of prescription, as well as the individual effect of patients on the occurrence of an ADE. To assess the performance of the proposed method, we conducted a case study with a real-world prescription dataset. Validated by the existing domain knowledge, our method successfully traced a wide range of medicines that are potentially responsible for the ADE. Further experiments were also carried out according to a recognised gold standard, our method achieved a sensitivity of 65.9% and specificity of 96.2%.},
234}
235
236@article{Anand2021,
237 year = {2021},
238 volume = {255},
239 url = {https://doi.org/10.1002/path.5773},
240 title = {{Weakly supervised learning on unannotated H\&E-stained slides predicts BRAF mutation in thyroid cancer with high accuracy}},
241 publisher = {John Wiley & Sons, Ltd},
242 pages = {232--242},
243 number = {3},
244 month = {nov},
245 mendeley-groups = {Responsible AI survey},
246 keywords = {BRAF V600E,H&E,computational pathology,deep learning,thyroid cancer,weakly supervised learning},
247 journal = {The Journal of Pathology},
248 issn = {0022-3417},
249 doi = {https://doi.org/10.1002/path.5773},
250 author = {Anand, Deepak and Yashashwi, Kumar and Kumar, Neeraj and Rane, Swapnil and Gann, Peter H and Sethi, Amit},
251 annote = {https://doi.org/10.1002/path.5773},
252 abstract = {Abstract Deep neural networks (DNNs) that predict mutational status from H&E slides of cancers can enable inexpensive and timely precision oncology. Although expert knowledge is reliable for annotating regions informative of malignancy and other known histologic patterns (strong supervision), it is unreliable for identifying regions informative of mutational status. This poses a serious impediment to obtaining higher prognostic accuracy and discovering new knowledge of pathobiology. We used a weakly supervised learning technique to train a DNN to predict BRAF V600E mutational status, determined using DNA testing, in H&E-stained images of thyroid cancer tissue without regional annotations. Our discovery cohort was a tissue microarray of only 85 patients from a single hospital. On a large independent external cohort of 444 patients from other hospitals, the trained model gave an area under the receiver operating characteristic curve of 0.98 (95% CI 0.97?1.00), which is much higher than the previously reported results for detecting any mutation using H&E by DNNs trained using strong supervision. We also developed a visualization technique that can automatically highlight regions the DNN found most informative for predicting mutational status. Our visualization is spatially granular and highly specific in highlighting strong negative and positive regions and moves us toward explainable artificial intelligence. Using t-tests, we confirmed that the proportions of follicular or papillary histology and oncocytic cytology, as noted for each patient by a pathologist who was blinded to the mutational status, were significantly different between mutated and wildtype patients. However, based solely on these features noted by the pathologist, a logistic regression classifier gave an average area under the receiver operating characteristic curve of 0.78 in five-fold cross-validation, which is much lower than that obtained using the DNN. These results highlight the potential of weakly supervised learning for training DNN models for problems where the informative visual patterns and their locations are not known a priori. ? 2021 The Pathological Society of Great Britain and Ireland. Published by John Wiley & Sons, Ltd.},
253}
254
255@article{Aslam2022,
256 year = {2022},
257 volume = {2022},
258 url = {https://doi.org/10.1155/2022/1558381},
259 title = {{Anomaly Detection Using Explainable Random Forest for the Prediction of Undesirable Events in Oil Wells}},
260 publisher = {Hindawi},
261 pages = {1558381},
262 mendeley-groups = {Responsible AI survey},
263 journal = {Applied Computational Intelligence and Soft Computing},
264 issn = {1687-9724},
265 doi = {10.1155/2022/1558381},
266 author = {Aslam, Nida and Khan, Irfan Ullah and Alansari, Aisha and Alrammah, Marah and Alghwairy, Atheer and Alqahtani, Rahaf and Alqahtani, Razan and Almushikes, Maryam and Hashim, Mohammed A L},
267 abstract = {The worldwide demand for oil has been rising rapidly for many decades, being the first indicator of economic development. Oil is extracted from underneath reservoirs found below land or ocean using oil wells. An offshore oil well is an oil well type where a wellbore is drilled underneath the ocean bed to obtain oil to the surface that demands more stability than other oil wells. The sensors of oil wells generate massive amounts of multivariate time-series data for surveillance engineers to analyze manually and have continuous insight into drilling operations. The manual analysis of data is challenging and time-consuming. Additionally, it can lead to several faulty events that could increase costs and production losses since the engineers tend to focus on the analysis rather than detecting the faulty events. Recently, machine learning (ML) techniques have significantly solved enormous real-time data anomaly problems by decreasing the data engineers’ interaction processes. Accordingly, this study aimed to utilize ML techniques to reduce the time spent manually to establish rules that detect abnormalities in oil wells, leading to rapid and more precise detection. Four ML algorithms were utilized, including random forest (RF), logistic regression (LR), k-nearest neighbor (K-NN), and decision tree (DT). The dataset used in this study suffers from the class imbalance issue; therefore, experiments were conducted using the original and sampled datasets. The empirical results demonstrated promising outcomes, where RF achieved the highest accuracy, recall, precision, F1-score, and AUC of 99.60%, 99.64%, 99.91%, 99.77%, and 1.00, respectively, using the sampled data, and 99.84%, 99.91%, 99.91%, 99.91%, and 1.00, respectively, using the original data. Besides, the study employed Explainable Artificial Intelligence (XAI) to enable surveillance engineers to interpret black box models to understand the causes of abnormalities. The proposed models can be used to successfully identify anomalous events in the oil wells.},
268}
269
270@misc{Marcillo2021,
271 year = {2021},
272 volume = {11},
273 title = {{Implementation of a Generalized Additive Model (GAM) for Soybean Maturity Prediction in African Environments}},
274 number = {6},
275 keywords = {Africa,generalized additive model (GAM),photoperiod,soybean,temperature},
276 isbn = {2073-4395},
277 doi = {10.3390/agronomy11061043},
278 booktitle = {Agronomy},
279 author = {Marcillo, Guillermo S and Martin, Nicolas F and Diers, Brian W and {Da Fonseca Santos}, Michelle and Leles, Erica P and Chigeza, Godfree and Francischini, Josy H},
280 abstract = {Time to maturity (TTM) is an important trait in soybean breeding programs. However, soybeans are a relatively new crop in Africa. As such, TTM information for soybeans is not yet as well defined as in other major producing areas. Multi-environment trials (METs) allow breeders to analyze crop performance across diverse conditions, but also pose statistical challenges (e.g., unbalanced data). Modern statistical methods, e.g., generalized additive models (GAMs), can flexibly smooth a range of responses while retaining observations that could be lost under other approaches. We leveraged 5 years of data from an MET breeding program in Africa to identify the best geographical and seasonal variables to explain site and genotypic differences in soybean TTM. Using soybean cycle features (e.g., minimum temperature, daylength) along with trial geolocation (longitude, latitude), a GAM predicted soybean TTM within 10 days of the average observed TTM (RMSE = 10.3; x = 109 days post-planting). Furthermore, we found significant differences between cultivars (p < 0.05) in TTM sensitivity to minimum temperature and daylength. Our results show potential to advance the design of maturity systems that enhance soybean planting and breeding decisions in Africa.},
281}
282
283@article{Pachauri2022,
284 year = {2022},
285 volume = {10},
286 title = {{Electrical Energy Prediction of Combined Cycle Power Plant Using Gradient Boosted Generalized Additive Model}},
287 pages = {24566--24577},
288 journal = {IEEE Access},
289 issn = {2169-3536 VO - 10},
290 doi = {10.1109/ACCESS.2022.3153720},
291 author = {Pachauri, N and Ahn, C W},
292}
293
294@article{Phelps2021,
295 year = {2021},
296 volume = {30},
297 url = {https://doi.org/10.1071/WF20139},
298 title = {{Comparing calibrated statistical and machine learning methods for wildland fire occurrence prediction: a case study of human-caused fires in Lac La Biche, Alberta, Canada}},
299 pages = {850--870},
300 number = {11},
301 keywords = {Keywords: artificial intelligence, classification, ensemble, forest fire occurrence prediction, generalised additive model, human-caused, supervised learning.},
302 journal = {International Journal of Wildland Fire},
303 author = {Phelps, Nathan and Woolford, Douglas G},
304 abstract = {Wildland fire occurrence prediction (FOP) modelling supports fire management decisions, such as suppression resource pre-positioning and the routeing of detection patrols. Common empirical modelling methods for FOP include both model-based (statistical modelling) and algorithmic-based (machine learning) approaches. However, it was recently shown that many machine learning models in FOP literature are not suitable for fire management operations because of overprediction if not properly calibrated to output true probabilities. We present methods for properly calibrating statistical and machine learning models for fine-scale, spatially explicit daily FOP followed by a case-study comparison of human-caused FOP modelling in the Lac La Biche region of Alberta, Canada, using data from 1996 to 2016. Calibrated bagged classification trees, random forests, neural networks, logistic regression models and logistic generalised additive models (GAMs) are compared in order to assess the pros and cons of these approaches when properly calibrated. Results suggest that logistic GAMs can have similar performance to machine learning models for FOP. Hence, we advocate that the pros and cons of different modelling approaches should be discussed with fire management practitioners when determining which models to use operationally because statistical methods are commonly viewed as more interpretable than machine learning methods. },
305}
306
307@article{Jia2022,
308 pages = {115024},
309 year = {2022},
310 volume = {314},
311 url = {https://www.scopus.com/inward/record.uri?eid=2-s2.0-85128328223&doi=10.1016%2Fj.jenvman.2022.115024&partnerID=40&md5=379a6bb97d7d3e63197e53447e28dcaa},
312 title = {{Changes in water use and wastewater generation influenced by the COVID-19 pandemic: A case study of China}},
313 journal = {Journal of Environmental Management},
314 doi = {10.1016/j.jenvman.2022.115024},
315 author = {Jia, X and Shahzad, K and Kleme{\v{s}}, J J and Jia, X},
316 annote = {Export Date: 03 February 2023; Cited By: 5},
317}
318
319@article{Li2022,
320 year = {2022},
321 volume = {13},
322 url = {https://www.scopus.com/inward/record.uri?eid=2-s2.0-85127604139&doi=10.3390%2Ff13030473&partnerID=40&md5=4566be2023fba1a3724883775a683e6f},
323 title = {{Experiencing Urban Green and Blue Spaces in Urban Wetlands as a Nature-Based Solution to Promote Positive Emotions}},
324 number = {3},
325 journal = {Forests},
326 doi = {10.3390/f13030473},
327 author = {Li, H and Peng, J and Jiao, Y and Ai, S},
328 annote = {Export Date: 03 February 2023; Cited By: 8},
329}
330
331@article{Bollyky2022,
332 year = {2022},
333 url = {https://www.scopus.com/inward/record.uri?eid=2-s2.0-85124985797&doi=10.1016%2FS0140-6736%2822%2900172-6&partnerID=40&md5=dac2e452b8cbbee07f899f92fb98833a},
334 pages = {1489--1512},
335 issue = {10334},
336 volume = {399},
337 title = {{Pandemic preparedness and COVID-19: an exploratory analysis of infection and fatality rates, and contextual factors associated with preparedness in 177 countries, from Jan 1, 2020, to Sept 30, 2021}},
338 journal = {The Lancet},
339 doi = {10.1016/S0140-6736(22)00172-6},
340 author = {Bollyky, T J and Hulland, E N and Barber, R M and Collins, J K and Kiernan, S and Moses, M and Pigott, D M and {Reiner Jr}, R C and Sorensen, R J D and Abbafati, C and Adolph, C and Allorant, A and Amlag, J O and Aravkin, A Y and Bang-Jensen, B and Carter, A and Castellano, R and Castro, E and Chakrabarti, S and Combs, E and Dai, X and Dangel, W J and Dapper, C and Deen, A and Duncan, B B and Earl, L and Erickson, M and Ewald, S B and Fedosseeva, T and Ferrari, A J and Flaxman, A D and Fullman, N and Gakidou, E and Galal, B and Gallagher, J and Giles, J R and Guo, G and He, J and Helak, M and Huntley, B M and Idrisov, B and Johanns, C and LeGrand, K E and Letourneau, I D and Lindstrom, A and Linebarger, E and Lotufo, P A and Lozano, R and Magistro, B and Malta, D C and M{\aa}nsson, J and {Mantilla Herrera}, A M and Marinho, F and Mirkuzie, A H and Mokdad, A H and Monasta, L and Naik, P and Nomura, S and O'Halloran, J K and Odell, C M and Olana, L T and Ostroff, S M and Pasovic, M and Passos, V.M.D.A. and Penberthy, L and Reinke, G and Santomauro, D F and Schmidt, M I and Sholokhov, A and Spurlock, E and Troeger, C E and Varavikova, E and Vo, A T and Vos, T and Walcott, R and Walker, A and Wigley, S D and Wiysonge, C S and Worku, N A and Wu, Y and {Wulf Hanson}, S and Zheng, P and Hay, S I and Murray, C J L and Dieleman, J L},
341 annote = {Export Date: 03 February 2023; Cited By: 20},
342}
343
344@article{Salonia2022,
345 year = {2022},
346 volume = {10},
347 url = {https://www.scopus.com/inward/record.uri?eid=2-s2.0-85113842768&doi=10.1111%2Fandr.13097&partnerID=40&md5=942ad6e3178dc7a8280099857d73f29e},
348 title = {{Testosterone in males with COVID-19: A 7-month cohort study}},
349 pages = {34--41},
350 number = {1},
351 journal = {Andrology},
352 doi = {10.1111/andr.13097},
353 author = {Salonia, A and Pontillo, M and Capogrosso, P and Gregori, S and Carenzi, C and Ferrara, A M and Rowe, I and Boeri, L and Larcher, A and Ramirez, G A and Tresoldi, C and Locatelli, M and Cavalli, G and Dagna, L and Castagna, A and Zangrillo, A and Tresoldi, M and Landoni, G and Rovere-Querini, P and Ciceri, F and Montorsi, F},
354 annote = {Export Date: 03 February 2023; Cited By: 20},
355}
356
357@article{Granata2022,
358 year = {2022},
359 volume = {14},
360 url = {https://www.scopus.com/inward/record.uri?eid=2-s2.0-85125244688&doi=10.3390%2Fcancers14051239&partnerID=40&md5=f350947557e204839ec06df0df6ed886},
361 title = {{EOB-MR Based Radiomics Analysis to Assess Clinical Outcomes following Liver Resection in Colorectal Liver Metastases}},
362 pages = {1239},
363 number = {5},
364 journal = {Cancers},
365 doi = {10.3390/cancers14051239},
366 author = {Granata, V and Fusco, R and {De Muzio}, F and Cutolo, C and Setola, S V and Dell'aversana, F and Ottaiano, A and Nasti, G and Grassi, R and Pilone, V and Miele, V and Brunese, M C and Tatangelo, F and Izzo, F and Petrillo, A},
367 annote = {Export Date: 03 February 2023; Cited By: 11},
368}
369
370@article{Zhang2022,
371 year = {2022},
372 volume = {33},
373 title = {{Improving EEG Decoding via Clustering-Based Multitask Feature Learning}},
374 pages = {3587--3597},
375 number = {8},
376 journal = {IEEE Transactions on Neural Networks and Learning Systems},
377 issn = {2162-2388 VO - 33},
378 doi = {10.1109/TNNLS.2021.3053576},
379 author = {Zhang, Y and Zhou, T and Wu, W and Xie, H and Zhu, H and Zhou, G and Cichocki, A},
380}
381
382@article{Uddin2022,
383 year = {2022},
384 volume = {12},
385 title = {{Comparative performance analysis of K-nearest neighbour (KNN) algorithm and its different variants for disease prediction}},
386 publisher = {Springer},
387 pages = {1--11},
388 number = {1},
389 journal = {Scientific Reports},
390 issn = {2045-2322},
391 author = {Uddin, Shahadat and Haque, Ibtisham and Lu, Haohui and Moni, Mohammad Ali and Gide, Ergun},
392}
393
394@article{Song2021,
395 year = {2021},
396 volume = {112},
397 pages = {107804},
398 url = {https://doi.org/10.1016/j.patcog.2020.107804},
399 title = {{Feature selection using bare-bones particle swarm optimization with mutual information}},
400 journal = {Pattern Recognition},
401 doi = {10.1016/j.patcog.2020.107804},
402 author = {Song, X.-F. and Zhang, Y and Gong, D.-W. and Sun, X.-Y.},
403 annote = {Export Date: 06 February 2023; Cited By: 68},
404}
405
406@article{Tubishat2021,
407 year = {2021},
408 volume = {164},
409 url = {https://doi.org/10.1016/j.eswa.2020.113873},
410 pages = {113873},
411 title = {{Dynamic Salp swarm algorithm for feature selection}},
412 journal = {Expert Systems with Applications},
413 doi = {10.1016/j.eswa.2020.113873},
414 author = {Tubishat, M and Ja'afar, S and Alswaitti, M and Mirjalili, S and Idris, N and Ismail, M A and Omar, M S},
415 annote = {Export Date: 06 February 2023; Cited By: 78},
416}
417
418@article{Zhao2022,
419 year = {2022},
420 volume = {189},
421 url = {https://www.sciencedirect.com/science/article/pii/S0957417421014433},
422 title = {{Severity level diagnosis of Parkinson's disease by ensemble K-nearest neighbor under imbalanced data}},
423 pages = {116113},
424 keywords = {Imbalanced data,K-nearest neighbor,Parkinson's disease,Severity level diagnosis},
425 journal = {Expert Systems with Applications},
426 issn = {0957-4174},
427 doi = {https://doi.org/10.1016/j.eswa.2021.116113},
428 author = {Zhao, Huan and Wang, Ruixue and Lei, Yaguo and Liao, Wei-Hsin and Cao, Hongmei and Cao, Junyi},
429 abstract = {The reliable and explainable diagnosis of severity level for Parkinson's disease (PD) is significant for the therapy. Nonetheless, there are little data for severe PD patients but abundant data for slight PD patients, and this imbalanced distribution reduces the accuracy of diagnosis. Besides, the intrinsic differences for different severity levels are still unclear due to the individual differences and similarity of gait. To figure out the gait differences toward the development of PD severity level, gait features like time and force features as well as their coefficient of variance and asymmetry index have been extracted and compared. To overcome the imbalance influence during the severity level diagnosis, an ensemble K-nearest neighbor (EnKNN) is proposed. The K-nearest neighbor algorithm is applied to construct the base classifiers with extracted features, then the weight of each base classifier is calculated by the G-mean score and the F-measure. Finally, base classifiers are integrated by weight voting. Results show that the proposed EnKNN can achieve an average accuracy of 95.02% (0.44%) for PD severity level diagnosis overwhelming the imbalanced distribution of data. Additionally, some gait features exhibit distinct change with the increase of PD severity level which helps to a reliable and explainable diagnosis.},
430}
431
432@article{Ghosh2021,
433 year = {2021},
434 volume = {9},
435 url = {https://www.scopus.com/inward/record.uri?eid=2-s2.0-85100517914&doi=10.1109%2FACCESS.2021.3053759&partnerID=40&md5=a1375a73257449ef0d9477f37e85c8bb},
436 title = {{Efficient prediction of cardiovascular disease using machine learning algorithms with relief and lasso feature selection techniques}},
437 pages = {19304--19326},
438 journal = {IEEE Access},
439 doi = {10.1109/ACCESS.2021.3053759},
440 author = {Ghosh, P and Azam, S and Jonkman, M and Karim, A and Shamrat, F.M.J.M. and Ignatious, E and Shultana, S and Beeravolu, A R and {De Boer}, F},
441 annote = {Export Date: 06 February 2023; Cited By: 102},
442}
443
444@article{Peng2021,
445 year = {2021},
446 volume = {45},
447 url = {https://doi.org/10.1007/s10916-021-01736-5},
448 title = {{An Explainable Artificial Intelligence Framework for the Deterioration Risk Prediction of Hepatitis Patients}},
449 pages = {61},
450 number = {5},
451 journal = {Journal of Medical Systems},
452 doi = {10.1007/s10916-021-01736-5},
453 author = {Peng, J and Zou, K and Zhou, M and Teng, Y and Zhu, X and Zhang, F and Xu, J},
454 annote = {Export Date: 06 February 2023; Cited By: 19},
455}
456
457@article{Wang2021,
458 year = {2021},
459 volume = {761},
460 url = {https://doi.org/10.1016/j.scitotenv.2020.144057},
461 title = {{Predicting stream water quality under different urban development pattern scenarios with an interpretable machine learning approach}},
462 journal = {Science of the Total Environment},
463 pages = {144057},
464 doi = {10.1016/j.scitotenv.2020.144057},
465 author = {Wang, R and Kim, J.-H. and Li, M.-H.},
466 annote = {Export Date: 06 February 2023; Cited By: 47},
467}
468
469@article{Liang2022,
470 year = {2022},
471 pages = {104295},
472 volume = {125},
473 url = {https://doi.org/10.1016/j.cemconcomp.2021.104295},
474 title = {{Interpretable Ensemble-Machine-Learning models for predicting creep behavior of concrete}},
475 journal = {Cement and Concrete Composites},
476 doi = {10.1016/j.cemconcomp.2021.104295},
477 author = {Liang, M and Chang, Z and Wan, Z and Gan, Y and Schlangen, E and {\v{S}}avija, B},
478 annote = {Export Date: 06 February 2023; Cited By: 37},
479}
480
481@inproceedings{Ensign2018,
482 address = {New York},
483 year = {2018},
484 title = {{Runaway feedback loops in predictive policing}},
485 publisher = {PMLR},
486 pages = {160--171},
487 isbn = {2640-3498},
488 booktitle = {Proceedings of Machine Learning Research 2018},
489 author = {Ensign, Danielle and Friedler, Sorelle A and Neville, Scott and Scheidegger, Carlos and Venkatasubramanian, Suresh},
490}
491
492@misc{Dastin2019,
493 year = {2019},
494 url = {https://www.reuters.com/article/us-amazon-com-jobs-automation-insight/amazon-scraps-secret-ai-recruiting-tool-that-showed-bias-against-women-idUSKCN1MK08G},
495 title = {{Amazon scraps secret AI recruiting tool that showed bias against women}},
496 booktitle = {Reuters},
497 author = {Dastin, Jeffrey},
498}
499
500@article{Ashta2021,
501 year = {2021},
502 volume = {30},
503 url = {https://doi.org/10.1002/jsc.2404},
504 title = {{Artificial intelligence and fintech: An overview of opportunities and risks for banking, investments, and microfinance}},
505 publisher = {John Wiley & Sons, Ltd},
506 pages = {211--222},
507 number = {3},
508 month = {may},
509 keywords = {artificial intelligence,banking,financial markets,fintech,microfinance},
510 journal = {Strategic Change},
511 issn = {1086-1718},
512 doi = {https://doi.org/10.1002/jsc.2404},
513 author = {Ashta, Arvind and Herrmann, Heinz},
514 annote = {https://doi.org/10.1002/jsc.2404},
515 abstract = {Abstract Artificial Intelligence (AI) is creating a rush of opportunities in the financial sector, but financial organizations need to be aware of the risks inherent in the use of this technology. Financial organizations are integrating AI in their operations: in-house, outsourced, or ecosystem-based. The growth of AI-based fintech firms has encouraged several mergers and acquisitions among financial service providers and wealth managers as they grapple with volatility, uncertainty, complexity, and ambiguity. AI's unique promise of combined cost reduction and increased differentiation makes it generally attractive across the board. However, perhaps other than fraud detection, these benefits depend on the scale of an organization. Risk arises from nonrepresentative data, bias inherent in representative data, choice of algorithms, and human decisions, based on their AI interpretations (and whether humans are involved at all once AI has been unleashed). Risk reduction requires a vigilant division of labour between AI and humans for the foreseeable future.},
516}
517
518@article{Laestadius2022,
519 year = {2022},
520 url = {https://doi.org/10.1177/14614448221142007},
521 title = {{Too human and not human enough: A grounded theory analysis of mental health harms from emotional dependence on the social chatbot Replika}},
522 publisher = {SAGE Publications},
523 pages = {14614448221142007},
524 month = {dec},
525 journal = {New Media \& Society},
526 volume = {26},
527 issn = {1461-4448},
528 doi = {10.1177/14614448221142007},
529 author = {Laestadius, Linnea and Bishop, Andrea and Gonzalez, Michael and Illen{\v{c}}{\'{i}}k, Diana and Campos-Castillo, Celeste},
530 annote = {doi: 10.1177/14614448221142007},
531 abstract = {Social chatbot (SC) applications offering social companionship and basic therapy tools have grown in popularity for emotional, social, and psychological support. While use appears to offer mental health benefits, few studies unpack the potential for harms. Our grounded theory study analyzes mental health experiences with the popular SC application Replika. We identified mental health relevant posts made in the r/Replika Reddit community between 2017 and 2021 (n?=?582). We find evidence of harms, facilitated via emotional dependence on Replika that resembles patterns seen in human?human relationships. Unlike other forms of technology dependency, this dependency is marked by role-taking, whereby users felt that Replika had its own needs and emotions to which the user must attend. While prior research suggests human?chatbot and human?human interactions may not resemble each other, we identify social and technological factors that promote parallels and suggest ways to balance the benefits and risks of SCs.},
532}
533
534@article{Rinta-Kahila2022,
535 year = {2022},
536 volume = {31},
537 url = {https://doi.org/10.1080/0960085X.2021.1960905},
538 title = {{Algorithmic decision-making and system destructiveness: A case of automatic debt recovery}},
539 publisher = {Taylor & Francis},
540 pages = {313--338},
541 number = {3},
542 month = {may},
543 journal = {European Journal of Information Systems},
544 issn = {0960-085X},
545 doi = {10.1080/0960085X.2021.1960905},
546 author = {Rinta-Kahila, Tapani and Someh, Ida and Gillespie, Nicole and Indulska, Marta and Gregor, Shirley},
547 annote = {doi: 10.1080/0960085X.2021.1960905},
548}
549
550@article{Norori2021,
551 year = {2021},
552 volume = {2},
553 url = {https://www.sciencedirect.com/science/article/pii/S2666389921002026},
554 title = {{Addressing bias in big data and AI for health care: A call for open science}},
555 pages = {100347},
556 number = {10},
557 keywords = {artificial intelligence,bias,data standards,deep learning,health care,open science,participatory science},
558 journal = {Patterns},
559 issn = {2666-3899},
560 doi = {https://doi.org/10.1016/j.patter.2021.100347},
561 author = {Norori, Natalia and Hu, Qiyang and Aellen, Florence Marcelle and Faraci, Francesca Dalia and Tzovara, Athina},
562 abstract = {Summary Artificial intelligence (AI) has an astonishing potential in assisting clinical decision making and revolutionizing the field of health care. A major open challenge that AI will need to address before its integration in the clinical routine is that of algorithmic bias. Most AI algorithms need big datasets to learn from, but several groups of the human population have a long history of being absent or misrepresented in existing biomedical datasets. If the training data is misrepresentative of the population variability, AI is prone to reinforcing bias, which can lead to fatal outcomes, misdiagnoses, and lack of generalization. Here, we describe the challenges in rendering AI algorithms fairer, and we propose concrete steps for addressing bias using tools from the field of open science.},
563}
564
565@article{Trocin2021,
566 year = {2021},
567 issue = {3},
568 volume = {23},
569 url = {https://doi.org/10.1007/s10796-021-10146-4},
570 pages = {1--19},
571 title = {{Responsible AI for Digital Health: a Synthesis and a Research Agenda}},
572 journal = {Information Systems Frontiers},
573 issn = {1572-9419},
574 doi = {10.1007/s10796-021-10146-4},
575 author = {Trocin, Cristina and Mikalef, Patrick and Papamitsiou, Zacharoula and Conboy, Kieran},
576 abstract = {Responsible AI is concerned with the design, implementation and use of ethical, transparent, and accountable AI technology in order to reduce biases, promote fairness, equality, and to help facilitate interpretability and explainability of outcomes, which are particularly pertinent in a healthcare context. However, the extant literature on health AI reveals significant issues regarding each of the areas of responsible AI, posing moral and ethical consequences. This is particularly concerning in a health context where lives are at stake and where there are significant sensitivities that are not as pertinent in other domains outside of health. This calls for a comprehensive analysis of health AI using responsible AI concepts as a structural lens. A systematic literature review supported our data collection and sampling procedure, the corresponding analysis, and extraction of research themes helped us provide an evidence-based foundation. We contribute with a systematic description and explanation of the intellectual structure of Responsible AI in digital health and develop an agenda for future research.},
577}
578
579@misc{Samsung2023,
580 year = {2023},
581 url = {https://www.samsung.com/latin_en/sustainability/digital-responsibility/ai-ethics/},
582 title = {{Samsung AI Principles}},
583 author = {Samsung},
584}
585
586@misc{Google2023,
587 year = {2023},
588 url = {https://ai.google/principles/},
589 title = {{Artificial Intelligence at Google: Our Principles}},
590 author = {Google},
591}
592
593@misc{Microsoft2023,
594 year = {2023},
595 url = {https://www.microsoft.com/en-us/ai/responsible-ai?activetab=pivot1%3Aprimaryr6},
596 title = {{Responsible AI}},
597 author = {Microsoft},
598}
599
600@misc{DefenseInnovationUnit2023,
601 year = {2023},
602 url = {https://www.diu.mil/responsible-ai-guidelines},
603 title = {{Responsible AI Guidelines}},
604 author = {{Defense Innovation Unit}},
605}
606
607@misc{CSIRO2023,
608 year = {2023},
609 url = {https://www.csiro.au/en/research/technology-space/ai/responsible-ai},
610 title = {{Responsible artificial intelligence}},
611 author = {CSIRO},
612}
613
614@incollection{shap,
615 url = {http://papers.nips.cc/paper/7062-a-unified-approach-to-interpreting-model-predictions.pdf},
616 publisher = {Curran Associates, Inc.},
617 address = {Long Beach, CA, USA},
618 year = {2017},
619 pages = {4765--4774},
620 booktitle = {Advances in Neural Information Processing Systems 30},
621 author = {Lundberg, Scott M and Lee, Su-In},
622 title = {A Unified Approach to Interpreting Model Predictions},
623}
624
625@inproceedings{lime,
626 year = {2016},
627 pages = {1135--1144},
628 address = {San Francisco, CA, USA},
629 publisher = {ACM},
630 booktitle = {Proceedings of the 22nd {ACM} {SIGKDD} International Conference on Knowledge Discovery and Data Mining, August 13-17, 2016},
631 title = {"Why Should {I} Trust You?": Explaining the Predictions of Any Classifier},
632 author = {Marco Tulio Ribeiro and Sameer Singh and Carlos Guestrin},
633}
634
635@inproceedings{gradcam,
636 year = {2017},
637 pages = {618--626},
638 month = {Oct},
639 address = {Venice, Italy},
640 publisher = {Curran Associates, Inc.},
641 booktitle = {Proceedings of the IEEE International Conference on Computer Vision (ICCV)},
642 title = {Grad-CAM: Visual Explanations From Deep Networks via Gradient-Based Localization},
643 author = {Selvaraju, Ramprasaath R. and Cogswell, Michael and Das, Abhishek and Vedantam, Ramakrishna and Parikh, Devi and Batra, Dhruv},
644}
645
646@article{Shin2021,
647 year = {2021},
648 volume = {146},
649 url = {https://www.sciencedirect.com/science/article/pii/S1071581920301531},
650 title = {{The effects of explainability and causability on perception, trust, and acceptance: Implications for explainable AI}},
651 pages = {102551},
652 mendeley-groups = {Responsible AI survey},
653 keywords = {Causability,Explainable Ai,Explanatorycues,Glassbox,Human-ai interaction,Human-centeredAI,Interpretability,Trust,Understandability},
654 journal = {International Journal of Human-Computer Studies},
655 issn = {1071-5819},
656 doi = {https://doi.org/10.1016/j.ijhcs.2020.102551},
657 author = {Shin, Donghee},
658 abstract = {Artificial intelligence and algorithmic decision-making processes are increasingly criticized for their black-box nature. Explainable AI approaches to trace human-interpretable decision processes from algorithms have been explored. Yet, little is known about algorithmic explainability from a human factors' perspective. From the perspective of user interpretability and understandability, this study examines the effect of explainability in AI on user trust and attitudes toward AI. It conceptualizes causability as an antecedent of explainability and as a key cue of an algorithm and examines them in relation to trust by testing how they affect user perceived performance of AI-driven services. The results show the dual roles of causability and explainability in terms of its underlying links to trust and subsequent user behaviors. Explanations of why certain news articles are recommended generate users trust whereas causability of to what extent they can understand the explanations affords users emotional confidence. Causability lends the justification for what and how should be explained as it determines the relative importance of the properties of explainability. The results have implications for the inclusion of causability and explanatory cues in AI systems, which help to increase trust and help users to assess the quality of explanations. Causable explainable AI will help people understand the decision-making process of AI algorithms by bringing transparency and accountability into AI systems.},
659}
660
661@article{Xiang2022,
662 year = {2022},
663 url = {https://doi.org/10.1080/0144929X.2021.2024252},
664 title = {{AI tools for debunking online spam reviews? Trust of younger and older adults in AI detection criteria}},
665 publisher = {Taylor & Francis},
666 pages = {1--20},
667 month = {jan},
668 mendeley-groups = {Responsible AI survey},
669 journal = {Behaviour \& Information Technology},
670 issn = {0144-929X},
671 doi = {10.1080/0144929X.2021.2024252},
672 author = {Xiang, Honglian and Zhou, Jia and Xie, Bingjun},
673 annote = {doi: 10.1080/0144929X.2021.2024252},
674 volume = {1},
675}
676
677@article{Alam2021,
678 year = {2021},
679 volume = {21},
680 url = {https://doi.org/10.1186/s12911-021-01542-6},
681 title = {{Examining the effect of explanation on satisfaction and trust in AI diagnostic systems}},
682 pages = {178},
683 number = {1},
684 mendeley-groups = {Responsible AI survey},
685 journal = {BMC Medical Informatics and Decision Making},
686 issn = {1472-6947},
687 doi = {10.1186/s12911-021-01542-6},
688 author = {Alam, Lamia and Mueller, Shane},
689 abstract = {Artificial Intelligence has the potential to revolutionize healthcare, and it is increasingly being deployed to support and assist medical diagnosis. One potential application of AI is as the first point of contact for patients, replacing initial diagnoses prior to sending a patient to a specialist, allowing health care professionals to focus on more challenging and critical aspects of treatment. But for AI systems to succeed in this role, it will not be enough for them to merely provide accurate diagnoses and predictions. In addition, it will need to provide explanations (both to physicians and patients) about why the diagnoses are made. Without this, accurate and correct diagnoses and treatments might otherwise be ignored or rejected.},
690}
691
692@inproceedings{Arous2021,
693 year = {2021},
694 volume = {7},
695 url = {https://www.scopus.com/inward/record.uri?eid=2-s2.0-85129363314&partnerID=40&md5=56f214b67454396f0aea9aa933bad621},
696 title = {{MARTA: Leveraging Human Rationales for Explainable Text Classification}},
697 pages = {5868--5876},
698 mendeley-groups = {Responsible AI survey},
699 publisher = {AAAI Press},
700 address = {Vancouver, Canada},
701 booktitle = {35th AAAI Conference on Artificial Intelligence, AAAI 2021},
702 author = {Arous, I and Dolamic, L and Yang, J and Bhardwaj, A and Cuccu, G and Cudr{\'{e}}-Mauroux, P},
703 annote = {Export Date: 07 February 2023; Cited By: 9},
704}
705
706@article{Chun2022,
707 year = {2022},
708 volume = {37},
709 url = {https://www.scopus.com/inward/record.uri?eid=2-s2.0-85119894593&doi=10.1111%2Fmice.12793&partnerID=40&md5=a42a78d8bea81191ffdd1e95588e525a},
710 title = {{A deep learning-based image captioning method to automatically generate comprehensive explanations of bridge damage}},
711 pages = {1387--1401},
712 number = {11},
713 mendeley-groups = {Responsible AI survey},
714 journal = {Computer-Aided Civil and Infrastructure Engineering},
715 doi = {10.1111/mice.12793},
716 author = {Chun, P.-J. and Yamane, T and Maemura, Y},
717 annote = {Export Date: 07 February 2023; Cited By: 12},
718}
719
720@article{Loh2022,
721 year = {2022},
722 volume = {226},
723 url = {https://www.sciencedirect.com/science/article/pii/S0169260722005429},
724 title = {{Application of explainable artificial intelligence for healthcare: A systematic review of the last decade (2011–2022)}},
725 pages = {107161},
726 keywords = {Attention mechanism,CBR,Deep learning,EBM,Expert system,Explainable artificial intelligence (XAI),GradCAM,Healthcare,LIME,LRP,Machine learning,PRISMA,Rule-based,SHAP,Saliency map},
727 journal = {Computer Methods and Programs in Biomedicine},
728 issn = {0169-2607},
729 doi = {https://doi.org/10.1016/j.cmpb.2022.107161},
730 author = {Loh, Hui Wen and Ooi, Chui Ping and Seoni, Silvia and Barua, Prabal Datta and Molinari, Filippo and Acharya, U Rajendra},
731 abstract = {Background and objectives Artificial intelligence (AI) has branched out to various applications in healthcare, such as health services management, predictive medicine, clinical decision-making, and patient data and diagnostics. Although AI models have achieved human-like performance, their use is still limited because they are seen as a black box. This lack of trust remains the main reason for their low use in practice, especially in healthcare. Hence, explainable artificial intelligence (XAI) has been introduced as a technique that can provide confidence in the model's prediction by explaining how the prediction is derived, thereby encouraging the use of AI systems in healthcare. The primary goal of this review is to provide areas of healthcare that require more attention from the XAI research community. Methods Multiple journal databases were thoroughly searched using PRISMA guidelines 2020. Studies that do not appear in Q1 journals, which are highly credible, were excluded. Results In this review, we surveyed 99 Q1 articles covering the following XAI techniques: SHAP, LIME, GradCAM, LRP, Fuzzy classifier, EBM, CBR, rule-based systems, and others. Conclusion We discovered that detecting abnormalities in 1D biosignals and identifying key text in clinical notes are areas that require more attention from the XAI research community. We hope this is review will encourage the development of a holistic cloud system for a smart city.},
732}
733
734@article{Saraswat2022,
735 year = {2022},
736 volume = {10},
737 title = {{Explainable AI for Healthcare 5.0: Opportunities and Challenges}},
738 pages = {84486--84517},
739 journal = {IEEE Access},
740 issn = {2169-3536 VO - 10},
741 doi = {10.1109/ACCESS.2022.3197671},
742 author = {Saraswat, D and Bhattacharya, P and Verma, A and Prasad, V K and Tanwar, S and Sharma, G and Bokoro, P N and Sharma, R},
743}
744
745@article{Muhammad2021,
746 year = {2021},
747 volume = {22},
748 url = {https://www.scopus.com/inward/record.uri?eid=2-s2.0-85097934346&doi=10.1109%2FTITS.2020.3032227&partnerID=40&md5=028d8fa7c3275b8a895671192c6414ef},
749 title = {{Deep Learning for Safe Autonomous Driving: Current Challenges and Future Directions}},
750 pages = {4316--4336},
751 number = {7},
752 journal = {IEEE Transactions on Intelligent Transportation Systems},
753 doi = {10.1109/TITS.2020.3032227},
754 author = {Muhammad, K and Ullah, A and Lloret, J and Ser, J D and {De Albuquerque}, V H C},
755 annote = {Export Date: 07 February 2023; Cited By: 57},
756}
757
758@article{Himeur2021,
759 year = {2021},
760 volume = {287},
761 pages = {116601},
762 url = {https://doi.org/10.1016/j.apenergy.2021.116601},
763 title = {{Artificial intelligence based anomaly detection of energy consumption in buildings: A review, current trends and new perspectives}},
764 journal = {Applied Energy},
765 doi = {10.1016/j.apenergy.2021.116601},
766 author = {Himeur, Y and Ghanem, K and Alsalemi, A and Bensaali, F and Amira, A},
767 annote = {Export Date: 07 February 2023; Cited By: 121},
768}
769
770@article{Piccialli2021,
771 year = {2021},
772 volume = {66},
773 url = {https://www.sciencedirect.com/science/article/pii/S1566253520303651},
774 title = {{A survey on deep learning in medicine: Why, how and when?}},
775 pages = {111--137},
776 keywords = {Artificial intelligence,Data science,Deep learning,Medicine,Neural networks},
777 journal = {Information Fusion},
778 issn = {1566-2535},
779 doi = {https://doi.org/10.1016/j.inffus.2020.09.006},
780 author = {Piccialli, Francesco and Somma, Vittorio Di and Giampaolo, Fabio and Cuomo, Salvatore and Fortino, Giancarlo},
781 abstract = {New technologies are transforming medicine, and this revolution starts with data. Health data, clinical images, genome sequences, data on prescribed therapies and results obtained, data that each of us has helped to create. Although the first uses of artificial intelligence (AI) in medicine date back to the 1980s, it is only with the beginning of the new millennium that there has been an explosion of interest in this sector worldwide. We are therefore witnessing the exponential growth of health-related information with the result that traditional analysis techniques are not suitable for satisfactorily management of this vast amount of data. AI applications (especially Deep Learning), on the other hand, are naturally predisposed to cope with this explosion of data, as they always work better as the amount of training data increases, a phase necessary to build the optimal neural network for a given clinical problem. This paper proposes a comprehensive and in-depth study of Deep Learning methodologies and applications in medicine. An in-depth analysis of the literature is presented; how, where and why Deep Learning models are applied in medicine are discussed and reviewed. Finally, current challenges and future research directions are outlined and analysed.},
782}
783
784@misc{StanfordVisionLab2020,
785 year = {2020},
786 url = {https://www.image-net.org/},
787 title = {{ImageNet}},
788 booktitle = {Stanford University},
789 author = {{Stanford Vision Lab}},
790}
791
792@misc{Keras2023,
793 year = {2023},
794 url = {https://keras.io/api/applications/vgg/},
795 title = {{VGG16 and VGG19}},
796 author = {Keras},
797}
798
799@misc{TensorFlow2023,
800 year = {2023},
801 url = {https://www.tensorflow.org/api_docs/python/tf/keras/applications/resnet50/ResNet50},
802 title = {{ResNet50}},
803 author = {TensorFlow},
804}
805
806@article{Zhang2021,
807 year = {2021},
808 volume = {127},
809 url = {https://doi.org/10.1016/j.ecolind.2021.107735},
810 pages = {107735},
811 title = {{Deep neural networks for global wildfire susceptibility modelling}},
812 journal = {Ecological Indicators},
813 doi = {10.1016/j.ecolind.2021.107735},
814 author = {Zhang, G and Wang, M and Liu, K},
815 annote = {Export Date: 10 February 2023; Cited By: 30},
816}
817
818@article{Hosseinzadeh2022,
819 year = {2022},
820 volume = {343},
821 url = {https://doi.org/10.1016/j.biortech.2021.126111},
822 pages = {126111},
823 title = {{Machine learning modeling and analysis of biohydrogen production from wastewater by dark fermentation process}},
824 journal = {Bioresource Technology},
825 doi = {10.1016/j.biortech.2021.126111},
826 author = {Hosseinzadeh, A and Zhou, J L and Altaee, A and Li, D},
827 annote = {Export Date: 10 February 2023; Cited By: 30},
828}
829
830@article{Mi2021,
831 year = {2021},
832 volume = {12},
833 pages = {3008},
834 url = {https://doi.org/10.1038/s41467-021-22756-2},
835 title = {{Permutation-based identification of important biomarkers for complex diseases via machine learning models}},
836 number = {1},
837 journal = {Nature Communications},
838 doi = {10.1038/s41467-021-22756-2},
839 author = {Mi, X and Zou, B and Zou, F and Hu, J},
840 annote = {Export Date: 10 February 2023; Cited By: 17},
841}
842
843@article{Taghizadeh-Mehrjardi2021,
844 pages = {115108},
845 year = {2021},
846 volume = {399},
847 url = {https://doi.org/10.1016/j.geoderma.2021.115108},
848 title = {{Enhancing the accuracy of machine learning models using the super learner technique in digital soil mapping}},
849 journal = {Geoderma},
850 doi = {10.1016/j.geoderma.2021.115108},
851 author = {Taghizadeh-Mehrjardi, R and Hamzehpour, N and Hassanzadeh, M and Heung, B and {Ghebleh Goydaragh}, M and Schmidt, K and Scholten, T},
852 annote = {Export Date: 10 February 2023; Cited By: 24},
853}
854
855@article{QuanTran2022,
856 year = {2022},
857 volume = {323},
858 pages = {126578},
859 url = {https://doi.org/10.1016/j.conbuildmat.2022.126578},
860 title = {{Evaluating compressive strength of concrete made with recycled concrete aggregates using machine learning approach}},
861 journal = {Construction and Building Materials},
862 doi = {10.1016/j.conbuildmat.2022.126578},
863 author = {{Quan Tran}, V and {Quoc Dang}, V and {Si Ho}, L},
864 annote = {Export Date: 10 February 2023; Cited By: 28},
865}
866
867@article{Khan2022,
868 year = {2022},
869 pages = {127215},
870 volume = {355},
871 url = {https://doi.org/10.1016/j.biortech.2022.127215},
872 title = {{Artificial neural networks for the prediction of biochar yield: A comparative study of metaheuristic algorithms}},
873 journal = {Bioresource Technology},
874 doi = {10.1016/j.biortech.2022.127215},
875 author = {Khan, M and Ullah, Z and Ma{\v{s}}ek, O and {Raza Naqvi}, S and {Nouman Aslam Khan}, M},
876 annote = {Export Date: 10 February 2023; Cited By: 16},
877}
878
879@article{Chen2021,
880 year = {2021},
881 volume = {87},
882 url = {https://doi.org/10.1016/j.tourman.2021.104357},
883 pages = {104357},
884 title = {{Customer purchase forecasting for online tourism: A data-driven method with multiplex behavior data}},
885 journal = {Tourism Management},
886 doi = {10.1016/j.tourman.2021.104357},
887 author = {Chen, S.-X. and Wang, X.-K. and Zhang, H.-Y. and Wang, J.-Q. and Peng, J.-J.},
888 annote = {Export Date: 10 February 2023; Cited By: 8},
889}
890
891@article{Zhi2022,
892 year = {2022},
893 volume = {327},
894 pages = {109216},
895 url = {https://www.scopus.com/inward/record.uri?eid=2-s2.0-85139828907&doi=10.1016%2Fj.agrformet.2022.109216&partnerID=40&md5=5b6099601cee14364bfa6598de111fbf},
896 title = {{Identifying the determinants of crop yields in China since 1952 and its policy implications}},
897 journal = {Agricultural and Forest Meteorology},
898 doi = {10.1016/j.agrformet.2022.109216},
899 author = {Zhi, J and Cao, X and Zhang, Z and Qin, T and Qu, L and Qi, L and Ge, L and Guo, A and Wang, X and Da, C and Sun, Y and Liu, W and Zhang, H and Fu, X},
900 annote = {Export Date: 10 February 2023; Cited By: 1},
901}
902
903@article{Mangalathu2022,
904 year = {2022},
905 pages = {112883},
906 volume = {250},
907 url = {https://doi.org/10.1016/j.engstruct.2021.112883},
908 title = {{Machine-learning interpretability techniques for seismic performance assessment of infrastructure systems}},
909 journal = {Engineering Structures},
910 doi = {10.1016/j.engstruct.2021.112883},
911 author = {Mangalathu, S and Karthikeyan, K and Feng, D.-C. and Jeon, J.-S.},
912 annote = {Export Date: 10 February 2023; Cited By: 26},
913}
914
915@article{Safaei2022,
916 year = {2022},
917 pages = {0262895},
918 volume = {17},
919 url = {https://doi.org/10.1371/journal.pone.0262895},
920 title = {{E-CatBoost: An efficient machine learning framework for predicting ICU mortality using the eICU Collaborative Research Database}},
921 number = {5 May},
922 journal = {PLoS ONE},
923 doi = {10.1371/journal.pone.0262895},
924 author = {Safaei, N and Safaei, B and Seyedekrami, S and Talafidaryani, M and Masoud, A and Wang, S and Li, Q and Moqri, M},
925 annote = {Export Date: 10 February 2023; Cited By: 1},
926}
927
928@article{Maloney2022,
929 year = {2022},
930 volume = {322},
931 pages = {116068},
932 url = {https://doi.org/10.1016/j.jenvman.2022.116068},
933 title = {{Explainable machine learning improves interpretability in the predictive modeling of biological stream conditions in the Chesapeake Bay Watershed, USA}},
934 journal = {Journal of Environmental Management},
935 doi = {10.1016/j.jenvman.2022.116068},
936 author = {Maloney, K O and Buchanan, C and Jepsen, R D and Krause, K P and Cashman, M J and Gressler, B P and Young, J A and Schmid, M},
937 annote = {Export Date: 10 February 2023; Cited By: 1},
938}
939
940@article{Afshar2022,
941 year = {2022},
942 volume = {12},
943 pages = {11476},
944 url = {https://doi.org/10.1038/s41598-022-15693-7},
945 title = {{Application of Extremely Randomised Trees for exploring influential factors on variant crash severity data}},
946 number = {1},
947 journal = {Scientific Reports},
948 doi = {10.1038/s41598-022-15693-7},
949 author = {Afshar, F and Seyedabrishami, S and Moridpour, S},
950 annote = {Export Date: 10 February 2023; Cited By: 1},
951}
952
953@article{Kim2021,
954 year = {2021},
955 volume = {23},
956 pages = {e24120},
957 url = {https://doi.org/10.2196/24120},
958 title = {{Real-time clinical decision support based on recurrent neural networks for in-hospital acute kidney injury: External validation and model interpretation}},
959 number = {4},
960 journal = {Journal of Medical Internet Research},
961 doi = {10.2196/24120},
962 author = {Kim, K and Yang, H and Yi, J and Son, H.-E. and Ryu, J.-Y. and Kim, Y C and Jeong, J C and Chin, H J and Na, K Y and Chae, D.-W. and Han, S S and Kim, S},
963 annote = {Export Date: 10 February 2023; Cited By: 7},
964}
965
966@article{Baker2021,
967 year = {2021},
968 volume = {134},
969 url = {https://www.sciencedirect.com/science/article/pii/S0010482521003152},
970 title = {{Hybridized neural networks for non-invasive and continuous mortality risk assessment in neonates}},
971 pages = {104521},
972 mendeley-groups = {Neonatal and Paediatric results,Neonatal and Paediatric results/Shortlist},
973 keywords = {Intensive care,Machine learning,Mortality risk prediction,Neonatal mortality,Neural networks,Prognostics},
974 journal = {Comp. Biol. Med.},
975 issn = {0010-4825},
976 doi = {https://doi.org/10.1016/j.compbiomed.2021.104521},
977 author = {Baker, Stephanie and Xiang, Wei and Atkinson, Ian},
978 abstract = {Premature birth is the primary risk factor in neonatal deaths, with the majority of extremely premature babies cared for in neonatal intensive care units (NICUs). Mortality risk prediction in this setting can greatly improve patient outcomes and resource utilization. However, existing schemes often require laborious medical testing and calculation, and are typically only calculated once at admission. In this work, we propose a shallow hybrid neural network for the prediction of mortality risk in 3-day, 7-day, and 14-day risk windows using only birthweight, gestational age, sex, and heart rate (HR) and respiratory rate (RR) information from a 12-h window. As such, this scheme is capable of continuously updating mortality risk assessment, enabling analysis of health trends and responses to treatment. The highest performing scheme was the network that considered mortality risk within 3 days, with this scheme outperforming state-of-the-art works in the literature and achieving an area under the receiver-operator curve (AUROC) of 0.9336 with standard deviation of 0.0337 across 5 folds of cross-validation. As such, we conclude that our proposed scheme could readily be used for continuously-updating mortality risk prediction in NICU environments.},
979}
980
981@article{Gaur2022,
982 year = {2022},
983 pages = {822666},
984 volume = {13},
985 url = {https://doi.org/10.3389/fgene.2022.822666},
986 title = {{Explanation-Driven Deep Learning Model for Prediction of Brain Tumour Status Using MRI Image Data}},
987 journal = {Frontiers in Genetics},
988 doi = {10.3389/fgene.2022.822666},
989 author = {Gaur, L and Bhandari, M and Razdan, T and Mallik, S and Zhao, Z},
990 annote = {Export Date: 10 February 2023; Cited By: 6},
991}
992
993@inproceedings{Zhang2022Spam,
994 year = {2022},
995 title = {{Explainable Artificial Intelligence to Detect Image Spam Using Convolutional Neural Network}},
996 pages = {1--5},
997 isbn = { VO -},
998 doi = {10.1109/ICCR56254.2022.9995839},
999 address = {Dubai, UAE},
1000 publisher = {Curran Associates},
1001 booktitle = {2022 International Conference on Cyber Resilience (ICCR)},
1002 author = {Zhang, Z and Damiani, E and Hamadi, H A and Yeun, C Y and Taher, F},
1003}
1004
1005@article{Amri2022,
1006 year = {2022},
1007 volume = {14},
1008 url = {https://doi.org/10.3390/rs14153565},
1009 title = {{Offshore Oil Slick Detection: From Photo-Interpreter to Explainable Multi-Modal Deep Learning Models Using SAR Images and Contextual Data}},
1010 pages = {3565},
1011 number = {15},
1012 journal = {Remote Sensing},
1013 doi = {10.3390/rs14153565},
1014 author = {Amri, E and Dardouillet, P and Benoit, A and Courteille, H and Bolon, P and Dubucq, D and Credoz, A},
1015 annote = {Export Date: 10 February 2023; Cited By: 1},
1016}
1017
1018@article{Brito2022,
1019 year = {2022},
1020 volume = {163},
1021 url = {https://doi.org/10.1016/j.ymssp.2021.108105},
1022 title = {{An explainable artificial intelligence approach for unsupervised fault detection and diagnosis in rotating machinery}},
1023 journal = {Mechanical Systems and Signal Processing},
1024 pages = {108105},
1025 doi = {10.1016/j.ymssp.2021.108105},
1026 author = {Brito, L C and Susto, G A and Brito, J N and Duarte, M A V},
1027 annote = {Export Date: 10 February 2023; Cited By: 42},
1028}
1029
1030@article{Ullah2022,
1031 year = {2022},
1032 volume = {46},
1033 url = {https://www.scopus.com/inward/record.uri?eid=2-s2.0-85131357493&doi=10.1002%2Fer.8219&partnerID=40&md5=a9cfd82891e5a368242c90a3f68d3822},
1034 title = {{Prediction of electric vehicle charging duration time using ensemble machine learning algorithm and Shapley additive explanations}},
1035 pages = {15211--15230},
1036 number = {11},
1037 journal = {International Journal of Energy Research},
1038 doi = {10.1002/er.8219},
1039 author = {Ullah, I and Liu, K and Yamamoto, T and Zahid, M and Jamal, A},
1040 annote = {Export Date: 10 February 2023; Cited By: 19},
1041}
1042
1043@article{Wang2022,
1044 year = {2022},
1045 volume = {301},
1046 pages = {113941},
1047 url = {https://doi.org/10.1016/j.jenvman.2021.113941},
1048 title = {{Towards better process management in wastewater treatment plants: Process analytics based on SHAP values for tree-based machine learning methods}},
1049 journal = {Journal of Environmental Management},
1050 doi = {10.1016/j.jenvman.2021.113941},
1051 author = {Wang, D and Thun{\'{e}}ll, S and Lindberg, U and Jiang, L and Trygg, J and Tysklind, M},
1052 annote = {Export Date: 10 February 2023; Cited By: 20},
1053}
1054
1055@article{Fan2022,
1056 year = {2022},
1057 pages = {127330},
1058 volume = {424},
1059 url = {https://doi.org/10.1016/j.jhazmat.2021.127330},
1060 title = {{Forecasting plastic waste generation and interventions for environmental hazard mitigation}},
1061 journal = {Journal of Hazardous Materials},
1062 doi = {10.1016/j.jhazmat.2021.127330},
1063 author = {Fan, Y V and Jiang, P and Tan, R R and Aviso, K B and You, F and Zhao, X and Lee, C T and Kleme{\v{s}}, J J},
1064 annote = {Export Date: 10 February 2023; Cited By: 23},
1065}
1066
1067@article{Uddin2022depression,
1068 year = {2022},
1069 volume = {34},
1070 url = {https://doi.org/10.1007/s00521-021-06426-4},
1071 title = {{Deep learning for prediction of depressive symptoms in a large textual dataset}},
1072 pages = {721--744},
1073 number = {1},
1074 journal = {Neural Computing and Applications},
1075 issn = {1433-3058},
1076 doi = {10.1007/s00521-021-06426-4},
1077 author = {Uddin, Md Zia and Dysthe, Kim Kristoffer and F{\o}lstad, Asbj{\o}rn and Brandtzaeg, Petter Bae},
1078 abstract = {Depression is a common illness worldwide with potentially severe implications. Early identification of depressive symptoms is a crucial first step towards assessment, intervention, and relapse prevention. With an increase in data sets with relevance for depression, and the advancement of machine learning, there is a potential to develop intelligent systems to detect symptoms of depression in written material. This work proposes an efficient approach using Long Short-Term Memory (LSTM)-based Recurrent Neural Network (RNN) to identify texts describing self-perceived symptoms of depression. The approach is applied on a large dataset from a public online information channel for young people in Norway. The dataset consists of youth's own text-based questions on this information channel. Features are then provided from a one-hot process on robust features extracted from the reflection of possible symptoms of depression pre-defined by medical and psychological experts. The features are better than conventional approaches, which are mostly based on the word frequencies (i.e., some topmost frequent words are chosen as features from the whole text dataset and applied to model the underlying events in any text message) rather than symptoms. Then, a deep learning approach is applied (i.e., RNN) to train the time-sequential features discriminating texts describing depression symptoms from posts with no such descriptions (non-depression posts). Finally, the trained RNN is used to automatically predict depression posts. The system is compared against conventional approaches where it achieved superior performance than others. The linear discriminant space clearly reveals the robustness of the features by generating better clustering than other traditional features. Besides, since the features are based on the possible symptoms of depression, the system may generate meaningful explanations of the decision from machine learning models using an explainable Artificial Intelligence (XAI) algorithm called Local Interpretable Model-Agnostic Explanations (LIME). The proposed depression symptom feature-based approach shows superior performance compared to the traditional general word frequency-based approaches where frequency of the features gets more importance than the specific symptoms of depression. Although the proposed approach is applied on a Norwegian dataset, a similar robust approach can be applied on other depression datasets developed in other languages with proper annotations and symptom-based feature extraction. Thus, the depression prediction approach can be adopted to contribute to develop better mental health care technologies such as intelligent chatbots.},
1079}
1080
1081@article{Behl2021,
1082 year = {2021},
1083 volume = {55},
1084 url = {https://www.sciencedirect.com/science/article/pii/S2212420921000674},
1085 title = {{Twitter for disaster relief through sentiment analysis for COVID-19 and natural hazard crises}},
1086 pages = {102101},
1087 keywords = {COVID-19 preparedness,Deep learning,Disaster management,Sentiment analysis},
1088 journal = {International Journal of Disaster Risk Reduction},
1089 issn = {2212-4209},
1090 doi = {https://doi.org/10.1016/j.ijdrr.2021.102101},
1091 author = {Behl, Shivam and Rao, Aman and Aggarwal, Sahil and Chadha, Sakshi and Pannu, H S},
1092 abstract = {In emergencies and disasters, large numbers of people require basic needs and medical attention. In such situations, online social media comes as a possible solution to aid the current disaster management methods. In this paper, supervised learning approaches are compared for the multi-class classification of Twitter data. A careful setting of Multilayer Perceptron (MLP) network layers and the optimizer has shown promising results for classification of tweets into three categories i.e. ‘resource needs', ‘resource availability', and ‘others' being neutral and of no useful information. Public data of Nepal Earthquake (2015) and Italy Earthquake (2016) have been used for training and validation of the models, and original COVID-19 data is acquired, annotated, and used for testing. Detailed data analysis of tweets collected during different disasters has also been incorporated in the paper. The proposed model has been able to achieve 83% classification accuracy on the original COVID-19 dataset. Local Interpretable Model-Agnostic Explanations (LIME) is used to explain the behavior and shortcomings model on COVID-19 data. This paper provides a simple choice for real-world applications and a good starting point for future research.},
1093}
1094
1095@article{Neves2021,
1096 year = {2021},
1097 volume = {133},
1098 url = {https://www.sciencedirect.com/science/article/pii/S0010482521001876},
1099 title = {{Interpretable heartbeat classification using local model-agnostic explanations on ECGs}},
1100 pages = {104393},
1101 keywords = {Electrocardiogram,Explainable artificial intelligence,Heartbeat classification,Human–AI interfaces,Machine learning,Model-agnostic method,Time series,Usability,Visual explanations},
1102 journal = {Computers in Biology and Medicine},
1103 issn = {0010-4825},
1104 doi = {https://doi.org/10.1016/j.compbiomed.2021.104393},
1105 author = {Neves, In{\^{e}}s and Folgado, Duarte and Santos, Sara and Barandas, Mar{\'{i}}lia and Campagner, Andrea and Ronzio, Luca and Cabitza, Federico and Gamboa, Hugo},
1106 abstract = {Treatment and prevention of cardiovascular diseases often rely on Electrocardiogram (ECG) interpretation. Dependent on the physician's variability, ECG interpretation is subjective and prone to errors. Machine learning models are often developed and used to support doctors; however, their lack of interpretability stands as one of the main drawbacks of their widespread operation. This paper focuses on an Explainable Artificial Intelligence (XAI) solution to make heartbeat classification more explainable using several state-of-the-art model-agnostic methods. We introduce a high-level conceptual framework for explainable time series and propose an original method that adds temporal dependency between time samples using the time series' derivative. The results were validated in the MIT-BIH arrhythmia dataset: we performed a performance's analysis to evaluate whether the explanations fit the model's behaviour; and employed the 1-D Jaccard's index to compare the subsequences extracted from an interpretable model and the XAI methods used. Our results show that the use of the raw signal and its derivative includes temporal dependency between samples to promote classification explanation. A small but informative user study concludes this study to evaluate the potential of the visual explanations produced by our original method for being adopted in real-world clinical settings, either as diagnostic aids or training resource.},
1107}
1108
1109@article{Uddin2021,
1110 year = {2021},
1111 volume = {11},
1112 url = {https://doi.org/10.1038/s41598-021-95947-y},
1113 title = {{Human activity recognition using wearable sensors, discriminant analysis, and long short-term memory-based neural structured learning}},
1114 pages = {16455},
1115 number = {1},
1116 journal = {Scientific Reports},
1117 issn = {2045-2322},
1118 doi = {10.1038/s41598-021-95947-y},
1119 author = {Uddin, Md Zia and Soylu, Ahmet},
1120 abstract = {Healthcare using body sensor data has been getting huge research attentions by a wide range of researchers because of its good practical applications such as smart health care systems. For instance, smart wearable sensor-based behavior recognition system can observe elderly people in a smart eldercare environment to improve their lifestyle and can also help them by warning about forthcoming unprecedented events such as falls or other health risk, to prolong their independent life. Although there are many ways of using distinguished sensors to observe behavior of people, wearable sensors mostly provide reliable data in this regard to monitor the individual's functionality and lifestyle. In this paper, we propose a body sensor-based activity modeling and recognition system using time-sequential information-based deep Neural Structured Learning (NSL), a promising deep learning algorithm. First, we obtain data from multiple wearable sensors while the subjects conduct several daily activities. Once the data is collected, the time-sequential information then go through some statistical feature processing. Furthermore, kernel-based discriminant analysis (KDA) is applied to see the better clustering of the features from different activity classes by minimizing inner-class scatterings while maximizing inter-class scatterings of the samples. The robust time-sequential features are then applied with Neural Structured Learning (NSL) based on Long Short-Term Memory (LSTM), for activity modeling. The proposed approach achieved around 99% recall rate on a public dataset. It is also compared to existing different conventional machine learning methods such as typical Deep Belief Network (DBN), Convolutional Neural Network (CNN), and Recurrent Neural Network (RNN) where they yielded the maximum recall rate of 94%. Furthermore, a fast and efficient explainable Artificial Intelligence (XAI) algorithm, Local Interpretable Model-Agnostic Explanations (LIME) is used to explain and check the machine learning decisions. The robust activity recognition system can be adopted for understanding peoples' behavior in their daily life in different environments such as homes, clinics, and offices.},
1121}
1122
1123@article{Onchis2021,
1124 year = {2021},
1125 volume = {125},
1126 url = {https://www.sciencedirect.com/science/article/pii/S0166361520305935},
1127 title = {{Stable and explainable deep learning damage prediction for prismatic cantilever steel beam}},
1128 pages = {103359},
1129 keywords = {Cantilever beam,Damage detection,LIME,SHAP,Stability-fit compensation index,XAI},
1130 journal = {Computers in Industry},
1131 issn = {0166-3615},
1132 doi = {https://doi.org/10.1016/j.compind.2020.103359},
1133 author = {Onchis, Darian M and Gillich, Gilbert-Rainer},
1134 abstract = {Deep learning models fulfill the goal of characterizing the condition of beams in an non-invasive manner by accurately classifying accelerometer data. But the high probabilistic accuracy achieved on the validation set, while being a necessary indicator is usually not sufficient in most operational situations. With the occurrence of a damage, the reliable prediction must be also explainable in human terms incorporating the features that generated that particular result. This will enhance the trust and also the possibility of correction for the future functioning conditions. For obtaining the interpretable model, we correlate model agnostic global and local explanations with the use of the LIME and respectively the SHAP algorithm. Since the local explanations might be unstable, we introduce a compound stability-fit compensation index as a quality indicator in order to accept an explanation. This index is computed using both the condition number and the R2 fit indicator. Extensive testing, showed us the benefits of our method to completely and trustfully characterize the location and the depth of damaged beams.},
1135}
1136
1137@article{Park2021,
1138 year = {2021},
1139 volume = {9},
1140 url = {https://www.scopus.com/inward/record.uri?eid=2-s2.0-85114744771&doi=10.1109%2FACCESS.2021.3110270&partnerID=40&md5=5e2d86c72e0ff6e155eea1487dfb9fe6},
1141 title = {{Explainability of Machine Learning Models for Bankruptcy Prediction}},
1142 pages = {124887--124899},
1143 journal = {IEEE Access},
1144 doi = {10.1109/ACCESS.2021.3110270},
1145 author = {Park, M S and Son, H and Hyun, C and Hwang, H J},
1146 annote = {Export Date: 10 February 2023; Cited By: 6},
1147}
1148
1149@article{Wu2022,
1150 year = {2022},
1151 volume = {191},
1152 url = {https://www.sciencedirect.com/science/article/pii/S0957417421015669},
1153 title = {{Jointly modeling transfer learning of industrial chain information and deep learning for stock prediction}},
1154 pages = {116257},
1155 keywords = {Deep learning,Industrial chain,Stock prediction,Transfer learning},
1156 journal = {Expert Systems with Applications},
1157 issn = {0957-4174},
1158 doi = {https://doi.org/10.1016/j.eswa.2021.116257},
1159 author = {Wu, Dingming and Wang, Xiaolong and Wu, Shaocong},
1160 abstract = {The prediction of stock price has always been a main challenge. The time series of stock price tends to exhibit very strong nonlinear characteristics. In recent years, with the rapid development of deep learning, the ability to automatically extract nonlinear features has significantly attracted scholars' attention. However, the majority of the relevant studies have concentrated on prediction of the changes of stock market based on the data of the specific stock (e.g., transaction data, financial data, etc.), while those studies ignored the interaction between stocks of different industries, especially the interaction between the stocks of upstream enterprises and downstream enterprises in the industrial chain. This paper aims to propose a combination of transfer learning of industrial chain information and deep learning models, including multilayer perceptron (MLP), recurrent neural network (RNN), long short-term memory (LSTM), and gated recurrent unit (GRU), for stock market prediction. These models are used to predict the trend of the 379 stock market indices by industry in China, and the DM test was employed for validation of the prediction results. It can be concluded that RNNs are not necessarily such an optimal choice for the prediction when dealing with specific time series data, and it could be justified by using the local interpretable model-agnostic explanations (LIME) algorithm. Hence, the MLP was selected to effectively improve the accuracy of the prediction of the stock market indices based on the transfer learning of industrial chain information. The investment strategy is constructed according to the prediction results, and the yield of maturity exceeds that of the buy-and-hold strategy.},
1161}
1162
1163@article{Ahsan2021,
1164 year = {2021},
1165 volume = {9},
1166 url = {https://www.scopus.com/inward/record.uri?eid=2-s2.0-85113747043&doi=10.3390%2Fhealthcare9091099&partnerID=40&md5=5d93872707e5b8bd9f9177b1c66d9586},
1167 title = {{Detection of covid-19 patients from ct scan and chest x-ray data using modified mobilenetv2 and lime}},
1168 number = {9},
1169 journal = {Healthcare (Switzerland)},
1170 pages = {1099},
1171 doi = {10.3390/healthcare9091099},
1172 author = {Ahsan, M M and Nazim, R and Siddique, Z and Huebner, P},
1173 annote = {Export Date: 10 February 2023; Cited By: 24},
1174}
1175
1176@article{Zhu2022,
1177 year = {2022},
1178 pages = {204},
1179 volume = {14},
1180 url = {https://www.scopus.com/inward/record.uri?eid=2-s2.0-85122214616&doi=10.3390%2Frs14010204&partnerID=40&md5=c28c63e9a7b2dcbf2842335919ec02c3},
1181 title = {{LIME-Based Data Selection Method for SAR Images Generation Using GAN}},
1182 number = {1},
1183 journal = {Remote Sensing},
1184 doi = {10.3390/rs14010204},
1185 author = {Zhu, M and Zang, B and Ding, L and Lei, T and Feng, Z and Fan, J},
1186 annote = {Export Date: 10 February 2023; Cited By: 3},
1187}
1188
1189@article{Ayling2022,
1190 year = {2022},
1191 volume = {2},
1192 url = {https://doi.org/10.1007/s43681-021-00084-x},
1193 title = {{Putting AI ethics to work: are the tools fit for purpose?}},
1194 pages = {405--429},
1195 number = {3},
1196 journal = {AI and Ethics},
1197 issn = {2730-5961},
1198 doi = {10.1007/s43681-021-00084-x},
1199 author = {Ayling, Jacqui and Chapman, Adriane},
1200 abstract = {Bias, unfairness and lack of transparency and accountability in Artificial Intelligence (AI) systems, and the potential for the misuse of predictive models for decision-making have raised concerns about the ethical impact and unintended consequences of new technologies for society across every sector where data-driven innovation is taking place. This paper reviews the landscape of suggested ethical frameworks with a focus on those which go beyond high-level statements of principles and offer practical tools for application of these principles in the production and deployment of systems. This work provides an assessment of these practical frameworks with the lens of known best practices for impact assessment and audit of technology. We review other historical uses of risk assessments and audits and create a typology that allows us to compare current AI ethics tools to Best Practices found in previous methodologies from technology, environment, privacy, finance and engineering. We analyse current AI ethics tools and their support for diverse stakeholders and components of the AI development and deployment lifecycle as well as the types of tools used to facilitate use. From this, we identify gaps in current AI ethics tools in auditing and risk assessment that should be considered going forward.},
1201}
1202
1203@article{Ti2021,
1204 year = {2021},
1205 volume = {15},
1206 url = {https://journals.lww.com/journaladdictionmedicine/Fulltext/2021/04000/Towards_Equitable_AI_Interventions_for_People_Who.3.aspx},
1207 title = {{Towards Equitable AI Interventions for People Who Use Drugs: Key Areas That Require Ethical Investment}},
1208 number = {2},
1209 keywords = {artificial intelligence ,drug use ,ethics ,intervention},
1210 journal = {Journal of Addiction Medicine},
1211 issn = {1932-0620},
1212 author = {Ti, Lianping and Ho, Anita and Knight, Rod},
1213 abstract = {There has been growing investment in artificial intelligence (AI) interventions to combat the opioid-driven overdose epidemic plaguing North America. Although the evidence for the use of technology and AI in medicine is mounting, there are a number of ethical, social, and political implications that need to be considered when designing AI interventions. In this commentary, we describe 2 key areas that will require ethical deliberation in order to ensure that AI is being applied ethically with socially vulnerable populations such as people who use drugs: (1) perpetuation of biases in data and (2) consent. We offer ways forward to guide and provide opportunities for interventionists to develop substance use-related AI technologies that account for the inherent biases embedded within conventional data systems. This includes a discussion of how other data generation techniques (eg, qualitative and community-based approaches) can be integrated within AI intervention development efforts to mitigate the limitations of relying on electronic health record data. Finally, we emphasize the need to involve people who use drugs as stakeholders in all phases of AI intervention development.},
1214}
1215
1216@article{Robert2020,
1217 year = {2020},
1218 volume = {35},
1219 url = {https://doi.org/10.1080/07370024.2020.1735391},
1220 title = {{Designing fair AI for managing employees in organizations: a review, critique, and design agenda}},
1221 publisher = {Taylor & Francis},
1222 pages = {545--575},
1223 number = {5-6},
1224 month = {nov},
1225 journal = {Human–Computer Interaction},
1226 issn = {0737-0024},
1227 doi = {10.1080/07370024.2020.1735391},
1228 author = {Robert, Lionel P and Pierce, Casey and Marquis, Liz and Kim, Sangmi and Alahmad, Rasha},
1229 annote = {doi: 10.1080/07370024.2020.1735391},
1230}
1231
1232@article{Draelos2020,
1233 year = {2020},
1234 pages = {1},
1235 volume = {1},
1236 title = {{HiResCam: Faithful location representation in visual attention for explainable 3D medical image classification}},
1237 journal = {arXiv preprint arXiv:2011.08891},
1238 author = {Draelos, Rachel Lea and Carin, Lawrence},
1239}
1240
1241@inproceedings{Zheng2022,
1242 year = {2022},
1243 address = {Tel Aviv, Israel},
1244 title = {{Shap-CAM: Visual Explanations for Convolutional Neural Networks Based on Shapley Value}},
1245 publisher = {Springer},
1246 pages = {459--474},
1247 booktitle = {Computer Vision–ECCV 2022: 17th European Conference},
1248 author = {Zheng, Quan and Wang, Ziwei and Zhou, Jie and Lu, Jiwen},
1249}
1250
1251@inproceedings{Selvaraju2017,
1252 year = {2017},
1253 pages = {618--626},
1254 month = {Oct},
1255 title = {Grad-CAM: Visual Explanations From Deep Networks via Gradient-Based Localization},
1256 address = {Venice, Italy},
1257 publisher = {Curran Associates, Inc.},
1258 booktitle = {Proceedings of the IEEE International Conference on Computer Vision (ICCV)},
1259 author = {Selvaraju, Ramprasaath R and Cogswell, Michael and Das, Abhishek and Vedantam, Ramakrishna and Parikh, Devi and Batra, Dhruv},
1260}
1261
1262@inproceedings{Wang2020,
1263 year = {2020},
1264 address = {Nashville, USA},
1265 title = {{Score-CAM: Score-weighted visual explanations for convolutional neural networks}},
1266 publisher = {Curran Associates},
1267 pages = {24--25},
1268 booktitle = {Proceedings of the IEEE/CVF conference on computer vision and pattern recognition workshops},
1269 author = {Wang, Haofan and Wang, Zifan and Du, Mengnan and Yang, Fan and Zhang, Zijian and Ding, Sirui and Mardziel, Piotr and Hu, Xia},
1270}
1271
1272@article{Qiu2022,
1273 year = {2022},
1274 volume = {72},
1275 pages = {103323},
1276 url = {https://doi.org/10.1016/j.bspc.2021.103323},
1277 title = {{Pose-guided matching based on deep learning for assessing quality of action on rehabilitation training}},
1278 journal = {Biomedical Signal Processing and Control},
1279 doi = {10.1016/j.bspc.2021.103323},
1280 author = {Qiu, Y and Wang, J and Jin, Z and Chen, H and Zhang, M and Guo, L},
1281 annote = {Export Date: 13 February 2023; Cited By: 26},
1282}
1283
1284@article{Chen2021cam,
1285 year = {2021},
1286 volume = {47},
1287 url = {https://www.scopus.com/inward/record.uri?eid=2-s2.0-85100523425&doi=10.1016%2Fj.aei.2021.101255&partnerID=40&md5=69ca07a571dd9d01beccae00afe3f692},
1288 title = {{SMD LED chips defect detection using a YOLOV3-dense model}},
1289 journal = {Advanced Engineering Informatics},
1290 doi = {10.1016/j.aei.2021.101255},
1291 author = {Chen, S.-H. and Tsai, C.-C.},
1292 annote = {Export Date: 13 February 2023; Cited By: 27},
1293}
1294
1295@article{Zhang2022cam,
1296 year = {2022},
1297 volume = {26},
1298 url = {https://www.scopus.com/inward/record.uri?eid=2-s2.0-85103296576&doi=10.1109%2FJBHI.2021.3066832&partnerID=40&md5=714c987c03c881bb9e9ebeff57ac5def},
1299 title = {{An Explainable 3D Residual Self-Attention Deep Neural Network for Joint Atrophy Localization and Alzheimer's Disease Diagnosis Using Structural MRI}},
1300 pages = {5289--5297},
1301 number = {11},
1302 journal = {IEEE Journal of Biomedical and Health Informatics},
1303 doi = {10.1109/JBHI.2021.3066832},
1304 author = {Zhang, X and Han, L and Zhu, W and Sun, L and Zhang, D},
1305 annote = {Export Date: 13 February 2023; Cited By: 26},
1306}
1307
1308@article{Yoo2021,
1309 year = {2021},
1310 volume = {183},
1311 url = {https://www.scopus.com/inward/record.uri?eid=2-s2.0-85108271372&doi=10.1016%2Fj.eswa.2021.115430&partnerID=40&md5=3a4b9ae15242fd3b15c1ec7200f79ab6},
1312 pages = {115430},
1313 title = {{Explainable artificial intelligence for manufacturing cost estimation and machining feature visualization}},
1314 journal = {Expert Systems with Applications},
1315 doi = {10.1016/j.eswa.2021.115430},
1316 author = {Yoo, S and Kang, N},
1317 annote = {Export Date: 13 February 2023; Cited By: 26},
1318}
1319
1320@article{Onishi2021,
1321 year = {2021},
1322 pages = {903},
1323 volume = {11},
1324 url = {https://doi.org/10.1038/s41598-020-79653-9},
1325 title = {{Explainable identification and mapping of trees using UAV RGB image and deep learning}},
1326 number = {1},
1327 journal = {Scientific Reports},
1328 doi = {10.1038/s41598-020-79653-9},
1329 author = {Onishi, M and Ise, T},
1330 annote = {Export Date: 13 February 2023; Cited By: 61},
1331}
1332
1333@article{Guidotti2022,
1334 year = {2022},
1335 pages = {1--55},
1336 volume = {36},
1337 url = {https://doi.org/10.1007/s10618-022-00831-6},
1338 title = {{Counterfactual explanations and how to find them: literature review and benchmarking}},
1339 journal = {Data Mining and Knowledge Discovery},
1340 doi = {10.1007/s10618-022-00831-6},
1341 author = {Guidotti, R},
1342 annote = {Export Date: 13 February 2023; Cited By: 10},
1343}
1344
1345@inproceedings{Ribeiro2018,
1346 year = {2018},
1347 volume = {32},
1348 pages = {1},
1349 title = {{Anchors: High-precision model-agnostic explanations}},
1350 address = {New Orleans, Lousiana, USA},
1351 publisher = {AAAI Press},
1352 isbn = {2374-3468},
1353 booktitle = {Proceedings of the AAAI conference on artificial intelligence},
1354 author = {Ribeiro, Marco Tulio and Singh, Sameer and Guestrin, Carlos},
1355}
1356
1357@article{LaGatta2021,
1358 year = {2021},
1359 volume = {179},
1360 url = {https://www.sciencedirect.com/science/article/pii/S0957417421004863},
1361 title = {{CASTLE: Cluster-aided space transformation for local explanations}},
1362 pages = {115045},
1363 keywords = {Artificial Intelligence,Clustering,Machine learning,eXplainable Artificial Intelligence},
1364 journal = {Expert Systems with Applications},
1365 issn = {0957-4174},
1366 doi = {https://doi.org/10.1016/j.eswa.2021.115045},
1367 author = {{La Gatta}, Valerio and Moscato, Vincenzo and Postiglione, Marco and Sperl{\`{i}}, Giancarlo},
1368 abstract = {With Artificial Intelligence becoming part of a rapidly increasing number of industrial applications, more and more requirements about their transparency and trustworthiness are being demanded to AI systems, especially in military, medical and financial domains, where decisions have a huge impact on lives. In this paper, we propose a novel model-agnostic Explainable AI (XAI) technique, named Cluster-aided Space Transformation for Local Explanation (CASTLE), able to provide rule-based explanations based on both the local and global model's workings, i.e. its detailed ”knowledge” in the neighborhood of the target instance and its general knowledge on the training dataset, respectively. The framework has been evaluated on six datasets in terms of temporal efficiency, cluster quality and model significance. Eventually, we asked 36 users to evaluate the explainability of the framework, getting as result an increase of interpretability of 6% with respect to another state-of-the-art technique, named Anchors.},
1369}
1370
1371@article{Ayoub2021,
1372 year = {2021},
1373 volume = {58},
1374 url = {https://doi.org/10.1016/j.ipm.2021.102569},
1375 title = {{Combat COVID-19 infodemic using explainable natural language processing models}},
1376 number = {4},
1377 journal = {Information Processing and Management},
1378 pages = {102569},
1379 doi = {10.1016/j.ipm.2021.102569},
1380 author = {Ayoub, J and Yang, X J and Zhou, F},
1381 annote = {Export Date: 13 February 2023; Cited By: 44},
1382}
1383
1384@inproceedings{Chefer2021,
1385 year = {2021},
1386 title = {{Transformer Interpretability Beyond Attention Visualization}},
1387 publisher = {Curran Associates},
1388 pages = {782--791},
1389 address = {Montreal, Canada},
1390 isbn = {2575-7075 VO},
1391 doi = {10.1109/CVPR46437.2021.00084},
1392 booktitle = {2021 IEEE/CVF Conference on Computer Vision and Pattern Recognition (CVPR)},
1393 author = {Chefer, H and Gur, S and Wolf, L},
1394}
1395
1396@article{Mondal2022,
1397 year = {2022},
1398 volume = {10},
1399 title = {{xViTCOS: Explainable Vision Transformer Based COVID-19 Screening Using Radiography}},
1400 pages = {1--10},
1401 journal = {IEEE Journal of Translational Engineering in Health and Medicine},
1402 issn = {2168-2372 VO - 10},
1403 doi = {10.1109/JTEHM.2021.3134096},
1404 author = {Mondal, A K and Bhattacharjee, A and Singla, P and Prathosh, A P},
1405}
1406
1407@inproceedings{buolamwini2018,
1408 abstract = {Recent studies demonstrate that machine learning algorithms can discriminate based on classes like race and gender. In this work, we present an approach to evaluate bias present in automated facial analysis algorithms and datasets with respect to phenotypic subgroups. Using the dermatologist approved Fitzpatrick Skin Type classification system, we characterize the gender and skin type distribution of two facial analysis benchmarks, IJB-A and Adience. We find that these datasets are overwhelmingly composed of lighter-skinned subjects (79.6% for IJB-A and 86.2% for Adience) and introduce a new facial analysis dataset which is balanced by gender and skin type. We evaluate 3 commercial gender classification systems using our dataset and show that darker-skinned females are the most misclassified group (with error rates of up to 34.7%). The maximum error rate for lighter-skinned males is 0.8%. The substantial disparities in the accuracy of classifying darker females, lighter females, darker males, and lighter males in gender classification systems require urgent attention if commercial companies are to build genuinely fair, transparent and accountable facial analysis algorithms.},
1409 url = {https://proceedings.mlr.press/v81/buolamwini18a.html},
1410 pdf = {http://proceedings.mlr.press/v81/buolamwini18a/buolamwini18a.pdf},
1411 publisher = {PMLR},
1412 month = {23--24 Feb},
1413 series = {Proceedings of Machine Learning Research},
1414 volume = {81},
1415 year = {2018},
1416 pages = {77--91},
1417 booktitle = {Proceedings of the 1st Conference on Fairness, Accountability and Transparency},
1418 author = {Buolamwini, Joy and Gebru, Timnit},
1419 title = {Gender Shades: Intersectional Accuracy Disparities in Commercial Gender Classification},
1420}
1421
1422@article{Bowyer2020,
1423 year = {2020},
1424 volume = {1},
1425 title = {{The “Criminality From Face” Illusion}},
1426 pages = {175--183},
1427 number = {4},
1428 journal = {IEEE Transactions on Technology and Society},
1429 issn = {2637-6415 VO - 1},
1430 doi = {10.1109/TTS.2020.3032321},
1431 author = {Bowyer, K W and King, M C and Scheirer, W J and Vangara, K},
1432}
1433
1434@article{Alikhademi2022,
1435 year = {2022},
1436 volume = {30},
1437 url = {https://doi.org/10.1007/s10506-021-09286-4},
1438 title = {{A review of predictive policing from the perspective of fairness}},
1439 pages = {1--17},
1440 number = {1},
1441 journal = {Artificial Intelligence and Law},
1442 issn = {1572-8382},
1443 doi = {10.1007/s10506-021-09286-4},
1444 author = {Alikhademi, Kiana and Drobina, Emma and Prioleau, Diandra and Richardson, Brianna and Purves, Duncan and Gilbert, Juan E},
1445 abstract = {Machine Learning has become a popular tool in a variety of applications in criminal justice, including sentencing and policing. Media has brought attention to the possibility of predictive policing systems causing disparate impacts and exacerbating social injustices. However, there is little academic research on the importance of fairness in machine learning applications in policing. Although prior research has shown that machine learning models can handle some tasks efficiently, they are susceptible to replicating systemic bias of previous human decision-makers. While there is much research on fair machine learning in general, there is a need to investigate fair machine learning techniques as they pertain to the predictive policing. Therefore, we evaluate the existing publications in the field of fairness in machine learning and predictive policing to arrive at a set of standards for fair predictive policing. We also review the evaluations of ML applications in the area of criminal justice and potential techniques to improve these technologies going forward. We urge that the growing literature on fairness in ML be brought into conversation with the legal and social science concerns being raised about predictive policing. Lastly, in any area, including predictive policing, the pros and cons of the technology need to be evaluated holistically to determine whether and how the technology should be used in policing.},
1446}
1447
1448@article{Malek2022,
1449 year = {2022},
1450 volume = {2},
1451 url = {https://doi.org/10.1007/s43681-022-00137-9},
1452 title = {{Criminal courts' artificial intelligence: the way it reinforces bias and discrimination}},
1453 pages = {233--245},
1454 number = {1},
1455 journal = {AI and Ethics},
1456 issn = {2730-5961},
1457 doi = {10.1007/s43681-022-00137-9},
1458 author = {Malek, Md. Abdul},
1459 abstract = {Embracive, pervasive, and unstoppable global algorithmization greatly influences the deployment of artificial intelligence systems in criminal courts to replace obsolete bail and sentencing practices, reduce recidivism risk, and modernize judicial practices. Since artificial intelligence systems have provably appeared to have the duality of golden promises and potential perils, applying such a system in the justice system also entails some associated risks. Hence, allocating this unchecked-novel resource in judicial domains sparks vigorous debate over their legal and ethical implications. With such backgrounds, this paper examines how and why artificial intelligence systems reinforce bias and discrimination in society and suggest what approach could be an alternative to the current predictive justice mechanisms in use.},
1460}
1461
1462@article{Straw2020,
1463 year = {2020},
1464 volume = {110},
1465 url = {https://www.sciencedirect.com/science/article/pii/S0933365720312306},
1466 title = {{The automation of bias in medical Artificial Intelligence (AI): Decoding the past to create a better future}},
1467 pages = {101965},
1468 keywords = {Artificial intelligence,Bias,Data science,Digital health,Disparities,Health,Healthcare,Inequality,Medicine},
1469 journal = {Artificial Intelligence in Medicine},
1470 issn = {0933-3657},
1471 doi = {https://doi.org/10.1016/j.artmed.2020.101965},
1472 author = {Straw, Isabel},
1473 abstract = {Medicine is at a disciplinary crossroads. With the rapid integration of Artificial Intelligence (AI) into the healthcare field the future care of our patients will depend on the decisions we make now. Demographic healthcare inequalities continue to persist worldwide and the impact of medical biases on different patient groups is still being uncovered by the research community. At a time when clinical AI systems are scaled up in response to the Covid19 pandemic, the role of AI in exacerbating health disparities must be critically reviewed. For AI to account for the past and build a better future, we must first unpack the present and create a new baseline on which to develop these tools. The means by which we move forwards will determine whether we project existing inequity into the future, or whether we reflect on what we hold to be true and challenge ourselves to be better. AI is an opportunity and a mirror for all disciplines to improve their impact on society and for medicine the stakes could not be higher.},
1474}
1475
1476@article{raji2022,
1477 numpages = {8},
1478 pages = {101–108},
1479 month = {dec},
1480 journal = {Commun. ACM},
1481 abstract = {Although algorithmic auditing has emerged as a key strategy to expose systematic biases embedded in software platforms, we struggle to understand the real-world impact of these audits and continue to find it difficult to translate such independent assessments into meaningful corporate accountability. To analyze the impact of publicly naming and disclosing performance results of biased AI systems, we investigate the commercial impact of Gender Shades, the first algorithmic audit of gender- and skin-type performance disparities in commercial facial analysis models. This paper (1) outlines the audit design and structured disclosure procedure used in the Gender Shades study, (2) presents new performance metrics from targeted companies such as IBM, Microsoft, and Megvii (Face++) on the Pilot Parliaments Benchmark (PPB) as of August 2018, (3) provides performance results on PPB by non-target companies such as Amazon and Kairos, and (4) explores differences in company responses as shared through corporate communications that contextualize differences in performance on PPB. Within 7 months of the original audit, we find that all three targets released new application program interface (API) versions. All targets reduced accuracy disparities between males and females and darker- and lighter-skinned subgroups, with the most significant update occurring for the darker-skinned female subgroup that underwent a 17.7--30.4% reduction in error between audit periods. Minimizing these disparities led to a 5.72--8.3% reduction in overall error on the Pilot Parliaments Benchmark (PPB) for target corporation APIs. The overall performance of non-targets Amazon and Kairos lags significantly behind that of the targets, with error rates of 8.66% and 6.60% overall, and error rates of 31.37% and 22.50% for the darker female subgroup, respectively. This is an expanded version of an earlier publication of these results, revised for a more general audience, and updated to include commentary on further developments.},
1482 doi = {10.1145/3571151},
1483 url = {https://doi.org/10.1145/3571151},
1484 issn = {0001-0782},
1485 number = {1},
1486 volume = {66},
1487 address = {New York, NY, USA},
1488 publisher = {ACM},
1489 issue_date = {January 2023},
1490 year = {2022},
1491 title = {Actionable Auditing Revisited: Investigating the Impact of Publicly Naming Biased Performance Results of Commercial AI Products},
1492 author = {Raji, Inioluwa Deborah and Buolamwini, Joy},
1493}
1494
1495@misc{Tesla2023,
1496 year = {2023},
1497 url = {https://www.tesla.com/en_AU/autopilot},
1498 title = {{Future of Driving}},
1499 author = {Tesla},
1500}
1501
1502@misc{Hardesty2019,
1503 year = {2019},
1504 url = {https://www.amazon.science/the-history-of-amazons-recommendation-algorithm},
1505 title = {{The history of Amazon's recommendation algorithm}},
1506 institution = {Amazon Science},
1507 booktitle = {Amazon Science},
1508 author = {Hardesty, L},
1509}
1510
1511@misc{NetflixResearch2022,
1512 year = {2022},
1513 url = {https://research.netflix.com/research-area/machine-learning},
1514 title = {{Machine Learning: Learning how to entertain the world}},
1515 author = {{Netflix Research}},
1516}
1517
1518@misc{Cadillac2023,
1519 year = {2023},
1520 urldate = {https://www.cadillac.com/world-of-cadillac/innovation/super-cruise},
1521 title = {{Innovating the Future of Driving Again}},
1522 author = {Cadillac},
1523}
1524
1525@misc{Bavas2019,
1526 year = {2019},
1527 url = {https://www.abc.net.au/news/2019-06-05/facial-recognition-quietly-switched-on-at-queensland-stadiums/11178334},
1528 title = {{Facial recognition quietly switched on at Queensland stadiums, sparking privacy concerns}},
1529 month = {jun},
1530 booktitle = {ABC News},
1531 author = {Bavas, Josh},
1532 address = {Brisbane, QLD, Australia},
1533}
1534
1535@misc{CHOICE2022,
1536 year = {2022},
1537 url = {https://www.choice.com.au/consumers-and-data/data-collection-and-use/how-your-data-is-used/articles/kmart-bunnings-and-the-good-guys-using-facial-recognition-technology-in-store},
1538 title = {{Kmart, Bunnings and The Good Guys using facial recognition technology in stores}},
1539 author = {CHOICE},
1540}
1541
1542@misc{NewSouthWalesPoliceForce2023,
1543 year = {2023},
1544 url = {https://www.police.nsw.gov.au/crime/terrorism/terrorism_categories/facial_recognition},
1545 title = {{Facial Recognition}},
1546 author = {{New South Wales Police Force}},
1547}
1548
1549@article{Yam2021,
1550 year = {2021},
1551 volume = {23},
1552 url = {https://doi.org/10.1007/s10676-021-09599-7},
1553 title = {{From human resources to human rights: Impact assessments for hiring algorithms}},
1554 pages = {611--623},
1555 number = {4},
1556 journal = {Ethics and Information Technology},
1557 issn = {1572-8439},
1558 doi = {10.1007/s10676-021-09599-7},
1559 author = {Yam, Josephine and Skorburg, Joshua August},
1560 abstract = {Over the years, companies have adopted hiring algorithms because they promise wider job candidate pools, lower recruitment costs and less human bias. Despite these promises, they also bring perils. Using them can inflict unintentional harms on individual human rights. These include the five human rights to work, equality and nondiscrimination, privacy, free expression and free association. Despite the human rights harms of hiring algorithms, the AI ethics literature has predominantly focused on abstract ethical principles. This is problematic for two reasons. First, AI principles have been criticized for being vague and not actionable. Second, the use of vague ethical principles to discuss algorithmic risks does not provide any accountability. This lack of accountability creates an algorithmic accountability gap. Closing this gap is crucial because, without accountability, the use of hiring algorithms can lead to discrimination and unequal access to employment opportunities. This paper makes two contributions to the AI ethics literature. First, it frames the ethical risks of hiring algorithms using international human rights law as a universal standard for determining algorithmic accountability. Second, it evaluates four types of algorithmic impact assessments in terms of how effectively they address the five human rights of job applicants implicated in hiring algorithms. It determines which of the assessments can help companies audit their hiring algorithms and close the algorithmic accountability gap.},
1561}
1562
1563@article{Tilmes2022,
1564 year = {2022},
1565 volume = {24},
1566 url = {https://doi.org/10.1007/s10676-022-09633-2},
1567 title = {{Disability, fairness, and algorithmic bias in AI recruitment}},
1568 pages = {21},
1569 number = {2},
1570 journal = {Ethics and Information Technology},
1571 issn = {1572-8439},
1572 doi = {10.1007/s10676-022-09633-2},
1573 author = {Tilmes, Nicholas},
1574 abstract = {While rapid advances in artificial intelligence (AI) hiring tools promise to transform the workplace, these algorithms risk exacerbating existing biases against marginalized groups. In light of these ethical issues, AI vendors have sought to translate normative concepts such as fairness into measurable, mathematical criteria that can be optimized for. However, questions of disability and access often are omitted from these ongoing discussions about algorithmic bias. In this paper, I argue that the multiplicity of different kinds and intensities of people's disabilities and the fluid, contextual ways in which they manifest point to the limits of algorithmic fairness initiatives. In particular, existing de-biasing measures tend to flatten variance within and among disabled people and abstract away information in ways that reinforce pathologization. While fair machine learning methods can help mitigate certain disparities, I argue that fairness alone is insufficient to secure accessible, inclusive AI. I then outline a disability justice approach, which provides a framework for centering disabled people's experiences and attending to the structures and norms that underpin algorithmic bias.},
1575}
1576
1577@inproceedings{Deshpande2020,
1578 series = {UMAP '20 Adjunct},
1579 location = {Genoa, Italy},
1580 keywords = {term weighting, job recommendation, fair machine learning, TF-IDF},
1581 numpages = {8},
1582 pages = {268–275},
1583 booktitle = {Adjunct Publication of the 28th ACM Conference on User Modeling, Adaptation and Personalization},
1584 abstract = {With increasing diversity in the labor market as well as the work force, employers receive resumes from an increasingly diverse population. However, studies and field experiments have confirmed the presence of bias in the labor market based on gender, race, and ethnicity. Many employers use automated resume screening to filter the many possible matches. Depending on how the automated screening algorithm is trained it can potentially exhibit bias towards a particular population by favoring certain socio-linguistic characteristics. The resume writing style and socio-linguistics are a potential source of bias as they correlate with protected characteristics such as ethnicity. A biased dataset is often translated into biased AI algorithms and de-biasing algorithms are being contemplated. In this work, we study the effects of socio-linguistic bias on resume to job description matching algorithms. We develop a simple technique, called fair-tf-idf, to match resumes with job descriptions in a fair way by mitigating the socio-linguistic bias.},
1585 doi = {10.1145/3386392.3399569},
1586 url = {https://doi.org/10.1145/3386392.3399569},
1587 address = {New York, NY, USA},
1588 publisher = {ACM},
1589 isbn = {9781450379502},
1590 year = {2020},
1591 title = {Mitigating Demographic Bias in AI-Based Resume Filtering},
1592 author = {Deshpande, Ketki V. and Pan, Shimei and Foulds, James R.},
1593}
1594
1595@article{Zou2022,
1596 year = {2022},
1597 url = {https://doi.org/10.1007/s43681-022-00234-9},
1598 title = {{AI and housing discrimination: the case of mortgage applications}},
1599 journal = {AI and Ethics},
1600 issn = {2730-5961},
1601 doi = {10.1007/s43681-022-00234-9},
1602 author = {Zou, Leying and Khern-am-nuai, Warut},
1603 abstract = {Issues surrounding bias and discrimination in housing markets have been acknowledged and discussed both in the literature and in practice. In this study, we investigate this issue specifically in the context of mortgage applications through the lens of an AI-based decision support system. Using the data provided as a part of the Home Mortgage Disclosure Act (HMDA), we first show that ethnicity bias does indeed exist in historical mortgage application approvals, where black applicants are more likely to be declined a mortgage compared with white applicants whose circumstances are otherwise similar. More interestingly, this bias is amplified when an off-the-shelf machine-learning model is used to recommend an approval/denial decision. Finally, when fair machine-learning algorithms are adopted to alleviate such biases, we find that the “fairness” actually leaves all stakeholders—black applicants, white applicants, and mortgage lenders—worse off. Our findings caution against the use of machine-learning models without human involvement when the decision has significant implications for the prediction subjects.},
1604}
1605
1606@misc{Alba2022,
1607 year = {2022},
1608 url = {https://www.bloomberg.com/news/newsletters/2022-12-08/chatgpt-open-ai-s-chatbot-is-spitting-out-biased-sexist-results},
1609 title = {{OpenAI Chatbot Spits Out Biased Musings, Despite Guardrails}},
1610 month = {dec},
1611 booktitle = {Bloomberg},
1612 author = {Alba, Davey},
1613}
1614
1615@misc{Wakefield2016,
1616 year = {2016},
1617 url = {https://www.bbc.com/news/technology-35890188},
1618 title = {{Microsoft chatbot is taught to swear on Twitter}},
1619 month = {mar},
1620 booktitle = {BBC},
1621 author = {Wakefield, Jane},
1622}
1623
1624@misc{Hsu2022,
1625 year = {2022},
1626 url = {https://www.nytimes.com/2023/02/08/technology/ai-chatbots-disinformation.html},
1627 title = {{Disinformation Researchers Raise Alarms About A.I. Chatbots}},
1628 month = {feb},
1629 booktitle = {The New York Times},
1630 author = {Hsu, Tiffany and Thompson, Stuart A},
1631 address = {New York},
1632}
1633
1634@article{Martinengo2022,
1635 year = {2022},
1636 volume = {319},
1637 url = {https://www.sciencedirect.com/science/article/pii/S0165032722010333},
1638 title = {{Evaluation of chatbot-delivered interventions for self-management of depression: Content analysis}},
1639 pages = {598--607},
1640 keywords = {Chatbot,Content analysis,Conversational agent,Depression,Digital health,Mood disorders,mHealth},
1641 journal = {Journal of Affective Disorders},
1642 issn = {0165-0327},
1643 doi = {https://doi.org/10.1016/j.jad.2022.09.028},
1644 author = {Martinengo, Laura and Lum, Elaine and Car, Josip},
1645 abstract = {Background Conversational agents (CAs) or chatbots are increasingly used for depression, anxiety, and wellbeing management. CAs are considered acceptable and helpful. However, little is known about the adequacy of CA responses. This study assessed the structure, content, and user-customization of mental health CA dialogues with users with depression or at risk of suicide. Methods We used content analysis to examine the dialogues of CAs previously included in three assessments of mental health apps (depression education, self-guided cognitive behavioural therapy, and suicide prevention) performed between 2019 and 2020. Two standardized user personas with depression were developed to interact with the CA. All conversations were saved as screenshots, transcribed verbatim, and coded inductively. Results Nine CAs were included. Seven CAs (78%) had Android and iOS versions; five CAs (56%) had at least 500,000 downloads. The analysis generated eight categories: self-introduction, personalization, appropriateness of CA responses, conveying empathy, guiding users through mood-boosting activities, mood monitoring, suicide risk management, and others. CAs could engage in empathic, non-judgemental conversations with users, offer support, and guide psychotherapeutic exercises. Limitations CA evaluations were performed using standardized personas, not real-world users. CAs were included for evaluation only if retrieved in the search strategies associated with the previous assessment studies. Conclusion Assessed CAs offered anonymous, empathic, non-judgemental interactions that align with evidence for face-to-face psychotherapy. CAs from app stores are not suited to provide comprehensive suicide risk management. Further research should evaluate the effectiveness of CA-led interventions in mental health care and in enhancing suicide risk management strategies.},
1646}
1647
1648@article{Yesilada2022Systematic,
1649 year = {2022},
1650 volume = {11},
1651 url = {http://hdl.handle.net/10419/254285},
1652 title = {{Systematic review: YouTube recommendations and problematic content}},
1653 publisher = {Alexander von Humboldt Institute for Internet and Society},
1654 pages = {1--22},
1655 number = {1},
1656 keywords = {300; YouTube; Extremism; Online radicalisation; Recommendation algorithms; Content recommender systems},
1657 journal = {Internet Policy Review},
1658 issn = {2197-6775},
1659 doi = {10.14763/2022.1.1652},
1660 author = {Yesilada, Muhsin and Lewandowsky, Stephan},
1661 address = {Berlin},
1662 abstract = {There has been much concern that social media, in particular YouTube, may facilitate radicalisation and polarisation of online audiences. This systematic review aimed to determine whether the YouTube recommender system facilitates pathways to problematic content such as extremist or radicalising material. The review conducted a narrative synthesis of the papers in this area. It assessed the eligibility of 1,187 studies and excluded studies using the PRISMA process for systematic reviews, leaving a final sample of 23 studies. Overall, 14 studies implicated the YouTube recommender system in facilitating problematic content pathways, seven produced mixed results, and two did not implicate the recommender system. The review's findings indicate that the YouTube recommender system could lead users to problematic content. However, due to limited access and an incomplete understanding of the YouTube recommender system, the models built by researchers might not reflect the actual mechanisms underlying the YouTube recommender system and pathways to problematic content.},
1663}
1664
1665@article{Whittaker2021Recommender,
1666 year = {2021},
1667 volume = {10},
1668 url = {http://hdl.handle.net/10419/235968},
1669 title = {{Recommender systems and the amplification of extremist content}},
1670 publisher = {Alexander von Humboldt Institute for Internet and Society},
1671 pages = {1--29},
1672 number = {2},
1673 keywords = {300; Filter bubble; Online radicalisation; Algorithms; Extremism; Regulation},
1674 journal = {Internet Policy Review},
1675 issn = {2197-6775},
1676 doi = {10.14763/2021.2.1565},
1677 author = {Whittaker, Joe and Looney, Se{\'{a}}n and Reed, Alastair and Votta, Fabio},
1678 address = {Berlin},
1679 abstract = {Policymakers have recently expressed concerns over the role of recommendation algorithms and their role in forming "filter bubbles". This is a particularly prescient concern in the context of extremist content online; these algorithms may promote extremist content at the expense of more moderate voices. In this article, we make two contributions to this debate. Firstly, we provide a novel empirical analysis of three platforms' recommendation systems when interacting with far-right content. We find that one platform-YouTube-does amplify extreme and fringe content, while two-Reddit and Gab-do not. Secondly, we contextualise these findings into the regulatory debate. There are currently few policy instruments for dealing with algorithmic amplification, and those that do exist largely focus on transparency. We argue that policymakers have yet to fully understand the problems inherent in "de-amplifying" legal, borderline content and argue that a co-regulatory approach may offer a route towards tackling many of these challenges.},
1680}
1681
1682@inproceedings{10.1145/3460231.3474241,
1683 series = {RecSys '21},
1684 location = {Amsterdam, Netherlands},
1685 keywords = {youtube, misinformation, audit, personalization, ethics, filter bubble},
1686 numpages = {11},
1687 pages = {1–11},
1688 booktitle = {Proceedings of the 15th ACM Conference on Recommender Systems},
1689 abstract = {The negative effects of misinformation filter bubbles in adaptive systems have been known to researchers for some time. Several studies investigated, most prominently on YouTube, how fast a user can get into a misinformation filter bubble simply by selecting “wrong choices” from the items offered. Yet, no studies so far have investigated what it takes to “burst the bubble”, i.e., revert the bubble enclosure. We present a study in which pre-programmed agents (acting as YouTube users) delve into misinformation filter bubbles by watching misinformation promoting content (for various topics). Then, by watching misinformation debunking content, the agents try to burst the bubbles and reach more balanced recommendation mixes. We recorded the search results and recommendations, which the agents encountered, and analyzed them for the presence of misinformation. Our key finding is that bursting of a filter bubble is possible, albeit it manifests differently from topic to topic. Moreover, we observe that filter bubbles do not truly appear in some situations. We also draw a direct comparison with a previous study. Sadly, we did not find much improvements in misinformation occurrences, despite recent pledges by YouTube.},
1690 doi = {10.1145/3460231.3474241},
1691 url = {https://doi.org/10.1145/3460231.3474241},
1692 address = {New York, NY, USA},
1693 publisher = {ACM},
1694 isbn = {9781450384582},
1695 year = {2021},
1696 title = {An Audit of Misinformation Filter Bubbles on YouTube: Bubble Bursting and Recent Behavior Changes},
1697 author = {Tomlein, Matus and Pecher, Branislav and Simko, Jakub and Srba, Ivan and Moro, Robert and Stefancova, Elena and Kompan, Michal and Hrckova, Andrea and Podrouzek, Juraj and Bielikova, Maria},
1698}
1699
1700@inproceedings{10.1145/3487553.3524674,
1701 series = {WWW '22},
1702 location = {Virtual Event, Lyon, France},
1703 keywords = {stance detection, polarization, sentiment analysis, news recommendation, filter bubbles, echo chambers, German news articles},
1704 numpages = {10},
1705 pages = {448–457},
1706 booktitle = {Companion Proceedings of the Web Conference 2022},
1707 abstract = {News recommender systems are used by online news providers to alleviate information overload and to provide personalized content to users. However, algorithmic news curation has been hypothesized to create filter bubbles and to intensify users’ selective exposure, potentially increasing their vulnerability to polarized opinions and fake news. In this paper, we show how information on news items’ stance and sentiment can be utilized to analyze and quantify the extent to which recommender systems suffer from biases. To that end, we have annotated a German news corpus on the topic of migration using stance detection and sentiment analysis. In an experimental evaluation with four different recommender systems, our results show a slight tendency of all four models for recommending articles with negative sentiments and stances against the topic of refugees and migration. Moreover, we observed a positive correlation between the sentiment and stance bias of the text-based recommenders and the preexisting user bias, which indicates that these systems amplify users’ opinions and decrease the diversity of recommended news. The knowledge-aware model appears to be the least prone to such biases, at the cost of predictive accuracy.},
1708 doi = {10.1145/3487553.3524674},
1709 url = {https://doi.org/10.1145/3487553.3524674},
1710 address = {New York, NY, USA},
1711 publisher = {ACM},
1712 isbn = {9781450391306},
1713 year = {2022},
1714 title = {Towards Analyzing the Bias of News Recommender Systems Using Sentiment and Stance Detection},
1715 author = {Alam, Mehwish and Iana, Andreea and Grote, Alexander and Ludwig, Katharina and M\"{u}ller, Philipp and Paulheim, Heiko},
1716}
1717
1718@inproceedings{Tomlein2021,
1719 series = {RecSys '21},
1720 location = {Amsterdam, Netherlands},
1721 keywords = {youtube, misinformation, audit, personalization, ethics, filter bubble},
1722 numpages = {11},
1723 pages = {1–11},
1724 booktitle = {Proceedings of the 15th ACM Conference on Recommender Systems},
1725 abstract = {The negative effects of misinformation filter bubbles in adaptive systems have been known to researchers for some time. Several studies investigated, most prominently on YouTube, how fast a user can get into a misinformation filter bubble simply by selecting “wrong choices” from the items offered. Yet, no studies so far have investigated what it takes to “burst the bubble”, i.e., revert the bubble enclosure. We present a study in which pre-programmed agents (acting as YouTube users) delve into misinformation filter bubbles by watching misinformation promoting content (for various topics). Then, by watching misinformation debunking content, the agents try to burst the bubbles and reach more balanced recommendation mixes. We recorded the search results and recommendations, which the agents encountered, and analyzed them for the presence of misinformation. Our key finding is that bursting of a filter bubble is possible, albeit it manifests differently from topic to topic. Moreover, we observe that filter bubbles do not truly appear in some situations. We also draw a direct comparison with a previous study. Sadly, we did not find much improvements in misinformation occurrences, despite recent pledges by YouTube.},
1726 doi = {10.1145/3460231.3474241},
1727 url = {https://doi.org/10.1145/3460231.3474241},
1728 address = {New York, NY, USA},
1729 publisher = {ACM},
1730 isbn = {9781450384582},
1731 year = {2021},
1732 title = {An Audit of Misinformation Filter Bubbles on YouTube: Bubble Bursting and Recent Behavior Changes},
1733 author = {Tomlein, Matus and Pecher, Branislav and Simko, Jakub and Srba, Ivan and Moro, Robert and Stefancova, Elena and Kompan, Michal and Hrckova, Andrea and Podrouzek, Juraj and Bielikova, Maria},
1734}
1735
1736@article{Mehrabi2021,
1737 keywords = {machine learning, representation learning, deep learning, natural language processing, Fairness and bias in artificial intelligence},
1738 numpages = {35},
1739 articleno = {115},
1740 month = {jul},
1741 journal = {ACM Comput. Surv.},
1742 abstract = {With the widespread use of artificial intelligence (AI) systems and applications in our everyday lives, accounting for fairness has gained significant importance in designing and engineering of such systems. AI systems can be used in many sensitive environments to make important and life-changing decisions; thus, it is crucial to ensure that these decisions do not reflect discriminatory behavior toward certain groups or populations. More recently some work has been developed in traditional machine learning and deep learning that address such challenges in different subdomains. With the commercialization of these systems, researchers are becoming more aware of the biases that these applications can contain and are attempting to address them. In this survey, we investigated different real-world applications that have shown biases in various ways, and we listed different sources of biases that can affect AI applications. We then created a taxonomy for fairness definitions that machine learning researchers have defined to avoid the existing bias in AI systems. In addition to that, we examined different domains and subdomains in AI showing what researchers have observed with regard to unfair outcomes in the state-of-the-art methods and ways they have tried to address them. There are still many future directions and solutions that can be taken to mitigate the problem of bias in AI systems. We are hoping that this survey will motivate researchers to tackle these issues in the near future by observing existing work in their respective fields.},
1743 doi = {10.1145/3457607},
1744 url = {https://doi.org/10.1145/3457607},
1745 issn = {0360-0300},
1746 number = {6},
1747 volume = {54},
1748 address = {New York, NY, USA},
1749 publisher = {ACM},
1750 issue_date = {July 2022},
1751 year = {2021},
1752 title = {A Survey on Bias and Fairness in Machine Learning},
1753 author = {Mehrabi, Ninareh and Morstatter, Fred and Saxena, Nripsuta and Lerman, Kristina and Galstyan, Aram},
1754}
1755
1756@misc{MetaAI2021,
1757 year = {2021},
1758 urldate = {2023-02-21},
1759 url = {https://ai.facebook.com/blog/facebooks-five-pillars-of-responsible-ai/},
1760 title = {{Facebook's five pillars of Responsible AI}},
1761 author = {{Meta AI}},
1762}
1763
1764@techreport{UKGovernment2021,
1765 year = {2021},
1766 url = {https://assets.publishing.service.gov.uk/government/uploads/system/uploads/attachment_data/file/1020402/National_AI_Strategy_-_PDF_version.pdf},
1767 title = {{National AI Strategy}},
1768 author = {{HM Government (United Kingdom)}},
1769}
1770
1771@techreport{USGovRAI2022,
1772 year = {2022},
1773 url = {https://www.nist.gov/system/files/documents/2022/08/18/AI_RMF_2nd_draft.pdf},
1774 title = {{AI Risk Management Framework: Second Draf}},
1775 author = {{National Institute of Standards and Technology} and {(U.S. Department of Commerce)}},
1776}
1777
1778@techreport{SaudiArabia2022,
1779 year = {2022},
1780 url = {https://istitlaa.ncc.gov.sa/en/transportation/ndmo/aiethicsprinciples/Documents/AI Ethics Principles.pdf},
1781 title = {{AI Ethics Principles}},
1782 author = {{Kingdom of Saudi Arabia}},
1783}
1784
1785@techreport{JapanMIST2022,
1786 year = {2022},
1787 url = {https://www.meti.go.jp/english/press/2022/0128_003.html},
1788 title = {{AI Governance in Japan}},
1789 author = {{Ministry of Economy Trade and Industry (Japan)}},
1790}
1791
1792@techreport{EuropeanCommission2021,
1793 year = {2021},
1794 url = {https://ec.europa.eu/futurium/en/ai-alliance-consultation.1.html},
1795 title = {{Ethics Guidelines for Trustworthy AI}},
1796 institution = {European Union},
1797 author = {{European Commission}},
1798}
1799
1800@techreport{ChinaRAI2022,
1801 year = {2022},
1802 url = {https://www.most.gov.cn/kjbgz/202109/t20210926_177063.html},
1803 title = {{New Generation Artificial Intelligence Code of Ethics}},
1804 author = {{Ministry of Science and Technology of the People's Republic of China}},
1805}
1806
1807@misc{IBM2023,
1808 year = {2023},
1809 url = {https://www.ibm.com/topics/ai-ethics},
1810 title = {{AI Ethics}},
1811 author = {IBM},
1812}
1813
1814@techreport{AmazonWebServices2022,
1815 year = {2022},
1816 url = {https://d1.awsstatic.com/responsible-machine-learning/responsible-use-of-machine-learning-guide.pdf},
1817 title = {{Responsible Use of Machine Learning}},
1818 author = {{Amazon Web Services}},
1819}
1820
1821@techreport{AWS2022,
1822 year = {2022},
1823 url = {https://aws.amazon.com/blogs/machine-learning/introducing-aws-ai-service-cards-a-new-resource-to-enhance-transparency-and-advance-responsible-ai/},
1824 title = {{Introducing AWS AI Service Cards: A new resource to enhance transparency and advance responsible AI}},
1825 institution = {Amazon Web Services},
1826 author = {Philom, Vasi and Hallinan, Peter and {Amazon Web Services}},
1827}
1828
1829@techreport{CsiroRAI2022,
1830 year = {2022},
1831 url = {https://www.csiro.au/en/research/technology-space/ai/AI-Ethics-Framework},
1832 title = {{Artificial Intelligence: Australia's Ethics Framework}},
1833 institution = {Australian Government},
1834 author = {{Commonwealth Scientific and Industrial Research Organisation} and {Data 61}},
1835}
1836
1837@article{Miron2021,
1838 year = {2021},
1839 volume = {29},
1840 url = {https://www.scopus.com/inward/record.uri?eid=2-s2.0-85086109009&doi=10.1007%2Fs10506-020-09268-y&partnerID=40&md5=d13c82484233d7ce2ba4d19d4f4a7e98},
1841 title = {{Evaluating causes of algorithmic bias in juvenile criminal recidivism}},
1842 pages = {111--147},
1843 number = {2},
1844 journal = {Artificial Intelligence and Law},
1845 doi = {10.1007/s10506-020-09268-y},
1846 author = {Miron, M and Tolan, S and G{\'{o}}mez, E and Castillo, C},
1847 annote = {Export Date: 22 February 2023; Cited By: 9},
1848}
1849
1850@misc{Zemel2013,
1851 year = {2013},
1852 volume = {28},
1853 url = {http://proceedings.mlr.press/v28/zemel13.pdf https://proceedings.mlr.press/v28/zemel13.html},
1854 title = {{Learning Fair Representations}},
1855 publisher = {PMLR},
1856 pages = {325--333},
1857 number = {3},
1858 author = {Zemel, Rich and Wu, Yu and Swersky, Kevin and Pitassi, Toni and Learning, Cynthia Dwork B T - Proceedings of the 30th International Conference on Machine},
1859 abstract = {We propose a learning algorithm for fair classification that achieves both group fairness (the proportion of members in a protected group receiving positive classification is identical to the proportion in the population as a whole), and individual fairness (similar individuals should be treated similarly). We formulate fairness as an optimization problem of finding a good representation of the data with two competing goals: to encode the data as well as possible, while simultaneously obfuscating any information about membership in the protected group. We show positive results of our algorithm relative to other known techniques, on three datasets. Moreover, we demonstrate several advantages to our approach. First, our intermediate representation can be used for other classification tasks (i.e., transfer learning is possible); secondly, we take a step toward learning a distance metric which can find important dimensions of the data for classification.},
1860}
1861
1862@article{Panigutti2021,
1863 year = {2021},
1864 volume = {58},
1865 url = {https://www.sciencedirect.com/science/article/pii/S030645732100145X},
1866 title = {{FairLens: Auditing black-box clinical decision support systems}},
1867 pages = {102657},
1868 number = {5},
1869 keywords = {Clinical decision support systems,Fairness and bias in machine learning systems,eXplainable artificial intelligence},
1870 journal = {Information Processing \& Management},
1871 issn = {0306-4573},
1872 doi = {https://doi.org/10.1016/j.ipm.2021.102657},
1873 author = {Panigutti, Cecilia and Perotti, Alan and Panisson, Andr{\'{e}} and Bajardi, Paolo and Pedreschi, Dino},
1874 abstract = {The pervasive application of algorithmic decision-making is raising concerns on the risk of unintended bias in AI systems deployed in critical settings such as healthcare. The detection and mitigation of model bias is a very delicate task that should be tackled with care and involving domain experts in the loop. In this paper we introduce FairLens, a methodology for discovering and explaining biases. We show how this tool can audit a fictional commercial black-box model acting as a clinical decision support system (DSS). In this scenario, the healthcare facility experts can use FairLens on their historical data to discover the biases of the model before incorporating it into the clinical decision flow. FairLens first stratifies the available patient data according to demographic attributes such as age, ethnicity, gender and healthcare insurance; it then assesses the model performance on such groups highlighting the most common misclassifications. Finally, FairLens allows the expert to examine one misclassification of interest by explaining which elements of the affected patients' clinical history drive the model error in the problematic group. We validate FairLens' ability to highlight bias in multilabel clinical DSSs introducing a multilabel-appropriate metric of disparity and proving its efficacy against other standard metrics.},
1875}
1876
1877@article{VanDis2023,
1878 year = {2023},
1879 volume = {614},
1880 title = {{ChatGPT: five priorities for research}},
1881 publisher = {Nature Publishing Group UK London},
1882 pages = {224--226},
1883 number = {7947},
1884 journal = {Nature},
1885 issn = {0028-0836},
1886 author = {van Dis, Eva A M and Bollen, Johan and Zuidema, Willem and van Rooij, Robert and Bockting, Claudi L},
1887}
1888
1889@article{Yeo2023,
1890 year = {2023},
1891 title = {{Assessing the performance of ChatGPT in answering questions regarding cirrhosis and hepatocellular carcinoma}},
1892 publisher = {Cold Spring Harbor Laboratory Press},
1893 pages = {2002--2023},
1894 journal = {medRxiv},
1895 author = {Yeo, Yee Hui and Samaan, Jamil S and Ng, Wee Han and Ting, Peng-Sheng and Trivedi, Hirsh and Vipani, Aarshi and Ayoub, Walid and Yang, Ju Dong and Liran, Omer and Spiegel, Brennan},
1896}
1897
1898@inproceedings{Pradhan2022,
1899 series = {SIGMOD '22},
1900 location = {Philadelphia, PA, USA},
1901 keywords = {explanations, fairness, interpretability, data debugging},
1902 numpages = {15},
1903 pages = {247–261},
1904 booktitle = {Proceedings of the 2022 International Conference on Management of Data},
1905 abstract = {A wide variety of fairness metrics and eXplainable Artificial Intelligence (XAI) approaches have been proposed in the literature to identify bias in machine learning models that are used in critical real-life contexts. However, merely reporting on a model's bias or generating explanations using existing XAI techniques is insufficient to locate and eventually mitigate sources of bias. We introduce Gopher, a system that produces compact, interpretable, and causal explanations for bias or unexpected model behavior by identifying coherent subsets of the training data that are root-causes for this behavior. Specifically, we introduce the concept of causal responsibility that quantifies the extent to which intervening on training data by removing or updating subsets of it can resolve the bias. Building on this concept, we develop an efficient approach for generating the top-k patterns that explain model bias by utilizing techniques from the machine learning (ML) community to approximate causal responsibility, and using pruning rules to manage the large search space for patterns. Our experimental evaluation demonstrates the effectiveness of Gopher in generating interpretable explanations for identifying and debugging sources of bias.},
1906 doi = {10.1145/3514221.3517886},
1907 url = {https://doi.org/10.1145/3514221.3517886},
1908 address = {New York, NY, USA},
1909 publisher = {ACM},
1910 isbn = {9781450392495},
1911 year = {2022},
1912 title = {Interpretable Data-Based Explanations for Fairness Debugging},
1913 author = {Pradhan, Romila and Zhu, Jiongli and Glavic, Boris and Salimi, Babak},
1914}
1915
1916@article{Nakao2022,
1917 keywords = {cultural dimensions, end-users, loan application decisions, AI fairness, explanatory debugging, human-in-the-loop},
1918 numpages = {30},
1919 articleno = {18},
1920 month = {jul},
1921 journal = {ACM Trans. Interact. Intell. Syst.},
1922 abstract = {Ensuring fairness in artificial intelligence (AI) is important to counteract bias and discrimination in far-reaching applications. Recent work has started to investigate how humans judge fairness and how to support machine learning experts in making their AI models fairer. Drawing inspiration from an Explainable AI approach called explanatory debugging used in interactive machine learning, our work explores designing interpretable and interactive human-in-the-loop interfaces that allow ordinary end-users without any technical or domain background to identify potential fairness issues and possibly fix them in the context of loan decisions. Through workshops with end-users, we co-designed and implemented a prototype system that allowed end-users to see why predictions were made, and then to change weights on features to “debug” fairness issues. We evaluated the use of this prototype system through an online study. To investigate the implications of diverse human values about fairness around the globe, we also explored how cultural dimensions might play a role in using this prototype. Our results contribute to the design of interfaces to allow end-users to be involved in judging and addressing AI fairness through a human-in-the-loop approach.},
1923 doi = {10.1145/3514258},
1924 url = {https://doi.org/10.1145/3514258},
1925 issn = {2160-6455},
1926 number = {3},
1927 volume = {12},
1928 address = {New York, NY, USA},
1929 publisher = {ACM},
1930 issue_date = {September 2022},
1931 year = {2022},
1932 title = {Toward Involving End-Users in Interactive Human-in-the-Loop AI Fairness},
1933 author = {Nakao, Yuri and Stumpf, Simone and Ahmed, Subeida and Naseer, Aisha and Strappelli, Lorenzo},
1934}
1935
1936@misc{Yogarajan2022,
1937 year = {2022},
1938 volume = {4 },
1939 url = {https://www.frontiersin.org/articles/10.3389/fcomp.2022.1070493},
1940 title = {{Data and model bias in artificial intelligence for healthcare applications in New Zealand }},
1941 isbn = {2624-9898},
1942 booktitle = {Frontiers in Computer Science },
1943 author = {Yogarajan, Vithya and Dobbie, Gillian and Leitch, Sharon and Keegan, Te Taka and Bensemann, Joshua and Witbrock, Michael and Asrani, Varsha and Reith, David},
1944 abstract = {<sec>IntroductionDevelopments in Artificial Intelligence (AI) are adopted widely in healthcare. However, the introduction and use of AI may come with biases and disparities, resulting in concerns about healthcare access and outcomes for underrepresented indigenous populations. In New Zealand, Māori experience significant inequities in health compared to the non-Indigenous population. This research explores equity concepts and fairness measures concerning AI for healthcare in New Zealand.</sec><sec>MethodsThis research considers data and model bias in NZ-based electronic health records (EHRs). Two very distinct NZ datasets are used in this research, one obtained from one hospital and another from multiple GP practices, where clinicians obtain both datasets. To ensure research equality and fair inclusion of Māori, we combine expertise in Artificial Intelligence (AI), New Zealand clinical context, and te ao Māori. The mitigation of inequity needs to be addressed in data collection, model development, and model deployment. In this paper, we analyze data and algorithmic bias concerning data collection and model development, training and testing using health data collected by experts. We use fairness measures such as disparate impact scores, equal opportunities and equalized odds to analyze tabular data. Furthermore, token frequencies, statistical significance testing and fairness measures for word embeddings, such as WEAT and WEFE frameworks, are used to analyze bias in free-form medical text. The AI model predictions are also explained using SHAP and LIME.</sec><sec>ResultsThis research analyzed fairness metrics for NZ EHRs while considering data and algorithmic bias. We show evidence of bias due to the changes made in algorithmic design. Furthermore, we observe unintentional bias due to the underlying pre-trained models used to represent text data. This research addresses some vital issues while opening up the need and opportunity for future research.</sec><sec>DiscussionsThis research takes early steps toward developing a model of socially responsible and fair AI for New Zealand's population. We provided an overview of reproducible concepts that can be adopted toward any NZ population data. Furthermore, we discuss the gaps and future research avenues that will enable more focused development of fairness measures suitable for the New Zealand population's needs and social structure. One of the primary focuses of this research was ensuring fair inclusions. As such, we combine expertise in AI, clinical knowledge, and the representation of indigenous populations. This inclusion of experts will be vital moving forward, proving a stepping stone toward the integration of AI for better outcomes in healthcare.</sec>},
1945}
1946
1947@article{Sauter2022,
1948 year = {2022},
1949 volume = {22},
1950 url = {https://www.scopus.com/inward/record.uri?eid=2-s2.0-85135129275&doi=10.3390%2Fs22145346&partnerID=40&md5=53f1a9cccfde72138aa95d3c0b093e4b},
1951 title = {{Validating Automatic Concept-Based Explanations for AI-Based Digital Histopathology}},
1952 number = {14},
1953 journal = {Sensors},
1954 doi = {10.3390/s22145346},
1955 author = {Sauter, D and Lodde, G and Nensa, F and Schadendorf, D and Livingstone, E and Kukuk, M},
1956 annote = {Export Date: 07 March 2023; Cited By: 1},
1957}
1958
1959@article{Muddamsetty2022,
1960 year = {2022},
1961 volume = {127},
1962 url = {https://www.scopus.com/inward/record.uri?eid=2-s2.0-85125244939&doi=10.1016%2Fj.patcog.2022.108604&partnerID=40&md5=641a800065266c4b0c6ffdab502a9907},
1963 title = {{Visual explanation of black-box model: Similarity Difference and Uniqueness (SIDU) method}},
1964 journal = {Pattern Recognition},
1965 doi = {10.1016/j.patcog.2022.108604},
1966 author = {Muddamsetty, S M and Jahromi, M N S and Ciontos, A E and Fenoy, L M and Moeslund, T B},
1967 annote = {Export Date: 16 March 2023; Cited By: 4},
1968}
1969
1970@misc{goodfellow2015explaining,
1971 primaryclass = {stat.ML},
1972 archiveprefix = {arXiv},
1973 eprint = {1412.6572},
1974 year = {2015},
1975 author = {Ian J. Goodfellow and Jonathon Shlens and Christian Szegedy},
1976 title = {Explaining and Harnessing Adversarial Examples},
1977}
1978
1979@article{Bai2021,
1980 year = {2021},
1981 volume = {120},
1982 url = {https://www.sciencedirect.com/science/article/pii/S0031320321002892},
1983 title = {{Explainable deep learning for efficient and robust pattern recognition: A survey of recent developments}},
1984 pages = {108102},
1985 keywords = {Adversarial robustness,Explainable deep learning,Network compression and acceleration,Stability in deep learning},
1986 journal = {Pattern Recognition},
1987 issn = {0031-3203},
1988 doi = {https://doi.org/10.1016/j.patcog.2021.108102},
1989 author = {Bai, Xiao and Wang, Xiang and Liu, Xianglong and Liu, Qiang and Song, Jingkuan and Sebe, Nicu and Kim, Been},
1990 abstract = {Deep learning has recently achieved great success in many visual recognition tasks. However, the deep neural networks (DNNs) are often perceived as black-boxes, making their decision less understandable to humans and prohibiting their usage in safety-critical applications. This guest editorial introduces the thirty papers accepted for the Special Issue on Explainable Deep Learning for Efficient and Robust Pattern Recognition. They are grouped into three main categories: explainable deep learning methods, efficient deep learning via model compression and acceleration, as well as robustness and stability in deep learning. For each of the three topics, a survey of the representative works and latest developments is presented, followed by the brief introduction of the accepted papers belonging to this topic. The special issue should be of high relevance to the reader interested in explainable deep learning methods for efficient and robust pattern recognition applications and it helps promoting the future research directions in this field.},
1991}
1992
1993@article{Noack2021,
1994 year = {2021},
1995 volume = {2},
1996 url = {https://doi.org/10.1007/s42979-020-00390-x},
1997 title = {{An Empirical Study on the Relation Between Network Interpretability and Adversarial Robustness}},
1998 pages = {32},
1999 number = {1},
2000 journal = {SN Computer Science},
2001 issn = {2661-8907},
2002 doi = {10.1007/s42979-020-00390-x},
2003 author = {Noack, Adam and Ahern, Isaac and Dou, Dejing and Li, Boyang},
2004 abstract = {Deep neural networks (DNNs) have had many successes, but they suffer from two major issues: (1) a vulnerability to adversarial examples and (2) a tendency to elude human interpretation. Interestingly, recent empirical and theoretical evidence suggests that these two seemingly disparate issues are actually connected. In particular, robust models tend to provide more interpretable gradients than non-robust models. However, whether this relationship works in the opposite direction remains obscure. With this paper, we seek empirical answers to the following question: can models acquire adversarial robustness when they are trained to have interpretable gradients? We introduce a theoretically inspired technique called Interpretation Regularization (IR), which encourages a model's gradients to (1) match the direction of interpretable target salience maps and (2) have small magnitude. To assess model performance and tease apart factors that contribute to adversarial robustness, we conduct extensive experiments on MNIST and CIFAR-10 with both $$\ell _2$$and $$\ell _\infty$$attacks. We demonstrate that training the networks to have interpretable gradients improves their robustness to adversarial perturbations. Applying the network interpretation technique SmoothGrad [59] yields additional performance gains, especially in cross-norm attacks and under heavy perturbations. The results indicate that the interpretability of the model gradients is a crucial factor for adversarial robustness. Code for the experiments can be found at https://github.com/a1noack/interp_regularization.},
2005}
2006
2007@article{deng2012mnist,
2008 publisher = {IEEE},
2009 year = {2012},
2010 pages = {141--142},
2011 number = {6},
2012 volume = {29},
2013 journal = {IEEE Signal Processing Magazine},
2014 author = {Deng, Li},
2015 title = {The mnist database of handwritten digit images for machine learning research},
2016}
2017
2018@article{smoothgrad,
2019 bibsource = {dblp computer science bibliography, https://dblp.org},
2020 biburl = {https://dblp.org/rec/journals/corr/SmilkovTKVW17.bib},
2021 timestamp = {Mon, 13 Aug 2018 16:48:36 +0200},
2022 eprint = {1706.03825},
2023 eprinttype = {arXiv},
2024 url = {http://arxiv.org/abs/1706.03825},
2025 year = {2017},
2026 volume = {abs/1706.03825},
2027 journal = {CoRR},
2028 title = {SmoothGrad: removing noise by adding noise},
2029 author = {Daniel Smilkov and
2030Nikhil Thorat and
2031Been Kim and
2032Fernanda B. Vi{\'{e}}gas and
2033Martin Wattenberg},
2034}
2035
2036@techreport{cifar10,
2037 year = {2009},
2038 institution = {},
2039 title = {Learning multiple layers of features from tiny images},
2040 author = {Alex Krizhevsky},
2041}
2042
2043@inproceedings{SharmaCERTIFAI,
2044 series = {AIES '20},
2045 location = {New York, NY, USA},
2046 keywords = {robust-ness, responsible artificial intelligence, fairness, explainability, machine learning},
2047 numpages = {7},
2048 pages = {166–172},
2049 booktitle = {Proceedings of the AAAI/ACM Conference on AI, Ethics, and Society},
2050 abstract = {Concerns within the machine learning community and external pressures from regulators over the vulnerabilities of machine learning algorithms have spurred on the fields of explainability, robustness, and fairness. Often, issues in explainability, robustness, and fairness are confined to their specific sub-fields and few tools exist for model developers to use to simultaneously build their modeling pipelines in a transparent, accountable, and fair way. This can lead to a bottleneck on the model developer's side as they must juggle multiple methods to evaluate their algorithms. In this paper, we present a single framework for analyzing the robustness, fairness, and explainability of a classifier. The framework, which is based on the generation of counterfactual explanations through a custom genetic algorithm, is flexible, model-agnostic, and does not require access to model internals. The framework allows the user to calculate robustness and fairness scores for individual models and generate explanations for individual predictions which provide a means for actionable recourse (changes to an input to help get a desired outcome). This is the first time that a unified tool has been developed to address three key issues pertaining towards building a responsible artificial intelligence system.},
2051 doi = {10.1145/3375627.3375812},
2052 url = {https://doi.org/10.1145/3375627.3375812},
2053 address = {New York, NY, USA},
2054 publisher = {ACM},
2055 isbn = {9781450371100},
2056 year = {2020},
2057 title = {CERTIFAI: A Common Framework to Provide Explanations and Analyse the Fairness and Robustness of Black-Box Models},
2058 author = {Sharma, Shubham and Henderson, Jette and Ghosh, Joydeep},
2059}
2060
2061@inproceedings{weng2018evaluating,
2062 year = {2018},
2063 publisher = {ICLR},
2064 booktitle = {Sixth International Conference on Learning Representations},
2065 author = {Weng, Tsui Wei and Zhang, Huan and Chen, Pin-Yu and Yi, Jinfeng and Su, Dong and Gao, Yupeng and Hsieh, Cho-Jui and Daniel, Luca},
2066 title = {Evaluating the robustness of neural networks: An extreme value theory approach},
2067}
2068
2069@inproceedings{malik2022xai,
2070 organization = {IEEE},
2071 year = {2022},
2072 pages = {1--8},
2073 booktitle = {2022 IEEE Intl Conf on Dependable, Autonomic and Secure Computing, Intl Conf on Pervasive Intelligence and Computing, Intl Conf on Cloud and Big Data Computing, Intl Conf on Cyber Science and Technology Congress (DASC/PiCom/CBDCom/CyberSciTech)},
2074 author = {Malik, AL-Essa and Andresini, Giuseppina and Appice, Annalisa and Malerba, Donato},
2075 title = {An XAI-based adversarial training approach for cyber-threat detection},
2076}
2077
2078@article{Ding2022,
2079 year = {2022},
2080 volume = {615},
2081 url = {https://www.sciencedirect.com/science/article/pii/S002002552201132X},
2082 title = {{Explainability of artificial intelligence methods, applications and challenges: A comprehensive survey}},
2083 pages = {238--292},
2084 keywords = {Black-box,Deep learning,Explainable AI,Machine learning,Responsible AI,White-box},
2085 journal = {Information Sciences},
2086 issn = {0020-0255},
2087 doi = {https://doi.org/10.1016/j.ins.2022.10.013},
2088 author = {Ding, Weiping and Abdel-Basset, Mohamed and Hawash, Hossam and Ali, Ahmed M},
2089 abstract = {The continuous advancement of Artificial Intelligence (AI) has been revolutionizing the strategy of decision-making in different life domains. Regardless of this achievement, AI algorithms have been built as Black-Boxes, that is as they hide their internal rationality and learning methodology from the human leaving many unanswered questions about how and why the AI decisions are made. The absence of explanation results in a sensible and ethical challenge. Explainable Artificial Intelligence (XAI) is an evolving subfield of AI that emphasizes developing a plethora of tools and techniques for unboxing the Black-Box AI solutions by generating human-comprehensible, insightful, and transparent explanations of AI decisions. This study begins by discussing the primary principles of XAI research, Black-Box problems, the targeted audience, and the related notion of explainability over the historical timeline of the XAI studies and accordingly establishes an innovative definition of explainability that addresses the earlier theoretical proposals. According to an extensive analysis of the literature, this study contributes to the body of knowledge by driving a fine-grained, multi-level, and multi-dimension taxonomy for insightful categorization of XAI studies with the main aim to shed light on the variations and commonalities of existing algorithms paving the way for extra methodological developments. Then, an experimental comparative analysis is presented for the explanation generated by common XAI algorithms applied to different categories of data to highlight their properties, advantages, and flaws. Followingly, this study discusses and categorizes the evaluation metrics for the XAI-generated explanation and the findings show that there is no common consensus on how an explanation must be expressed, and how its quality and dependability should be evaluated. The findings show that XAI can contribute to realizing responsible and trustworthy AI, however, the advantages of interpretability should be technically demonstrated, and complementary procedures and regulations are required to give actionable information that can empower decision-making in real-world applications. Finally, the tutorial is crowned by discussing the open research questions, challenges, and future directions that serve as a roadmap for the AI community to advance the research in XAI and to inspire specialists and practitioners to take the advantage of XAI in different disciplines.},
2090}
2091
2092@inproceedings{Aechtner2022,
2093 year = {2022},
2094 title = {{Comparing User Perception of Explanations Developed with XAI Methods}},
2095 pages = {1--7},
2096 isbn = {1558-4739 VO -},
2097 doi = {10.1109/FUZZ-IEEE55066.2022.9882743},
2098 booktitle = {2022 IEEE International Conference on Fuzzy Systems},
2099 author = {Aechtner, J and Cabrera, L and Katwal, D and Onghena, P and Valenzuela, D P and Wilbik, A},
2100}
2101
2102@article{Waa2020,
2103 year = {2020},
2104 volume = {144},
2105 url = {https://www.sciencedirect.com/science/article/pii/S1071581920300951},
2106 title = {{Interpretable confidence measures for decision support systems}},
2107 pages = {102493},
2108 keywords = {Artificial intelligence,Confidence,Decision support systems,Explainable AI,Interpretable,Interpretable machine learning,Machine learning,Transparency,Trust calibration,User study},
2109 journal = {International Journal of Human-Computer Studies},
2110 issn = {1071-5819},
2111 doi = {https://doi.org/10.1016/j.ijhcs.2020.102493},
2112 author = {van der Waa, Jasper and Schoonderwoerd, Tjeerd and van Diggelen, Jurriaan and Neerincx, Mark},
2113 abstract = {Decision support systems (DSS) have improved significantly but are more complex due to recent advances in Artificial Intelligence. Current XAI methods generate explanations on model behaviour to facilitate a user's understanding, which incites trust in the DSS. However, little focus has been on the development of methods that establish and convey a system's confidence in the advice that it provides. This paper presents a framework for Interpretable Confidence Measures (ICMs). We investigate what properties of a confidence measure are desirable and why, and how an ICM is interpreted by users. In several data sets and user experiments, we evaluate these ideas. The presented framework defines four properties: 1) accuracy or soundness, 2) transparency, 3) explainability and 4) predictability. These characteristics are realized by a case-based reasoning approach to confidence estimation. Example ICMs are proposed for -and evaluated on- multiple data sets. In addition, ICM was evaluated by performing two user experiments. The results show that ICM can be as accurate as other confidence measures, while behaving in a more predictable manner. Also, ICM's underlying idea of case-based reasoning enables generating explanations about the computation of the confidence value, and facilitates user's understandability of the algorithm.},
2114}
2115
2116@article{Singla2023,
2117 year = {2023},
2118 volume = {84},
2119 url = {https://www.sciencedirect.com/science/article/pii/S1361841522003498},
2120 title = {{Explaining the black-box smoothly—A counterfactual approach}},
2121 pages = {102721},
2122 keywords = {Chest X-ray diagnosis,Counterfactual reasoning,Explainable AI,Interpretable machine learning},
2123 journal = {Medical Image Analysis},
2124 issn = {1361-8415},
2125 doi = {https://doi.org/10.1016/j.media.2022.102721},
2126 author = {Singla, Sumedha and Eslami, Motahhare and Pollack, Brian and Wallace, Stephen and Batmanghelich, Kayhan},
2127 abstract = {We propose a BlackBox Counterfactual Explainer, designed to explain image classification models for medical applications. Classical approaches (e.g., , saliency maps) that assess feature importance do not explain how imaging features in important anatomical regions are relevant to the classification decision. Such reasoning is crucial for transparent decision-making in healthcare applications. Our framework explains the decision for a target class by gradually exaggerating the semantic effect of the class in a query image. We adopted a Generative Adversarial Network (GAN) to generate a progressive set of perturbations to a query image, such that the classification decision changes from its original class to its negation. Our proposed loss function preserves essential details (e.g., support devices) in the generated images. We used counterfactual explanations from our framework to audit a classifier trained on a chest X-ray dataset with multiple labels. Clinical evaluation of model explanations is a challenging task. We proposed clinically-relevant quantitative metrics such as cardiothoracic ratio and the score of a healthy costophrenic recess to evaluate our explanations. We used these metrics to quantify the counterfactual changes between the populations with negative and positive decisions for a diagnosis by the given classifier. We conducted a human-grounded experiment with diagnostic radiology residents to compare different styles of explanations (no explanation, saliency map, cycleGAN explanation, and our counterfactual explanation) by evaluating different aspects of explanations: (1) understandability, (2) classifier's decision justification, (3) visual quality, (d) identity preservation, and (5) overall helpfulness of an explanation to the users. Our results show that our counterfactual explanation was the only explanation method that significantly improved the users' understanding of the classifier's decision compared to the no-explanation baseline. Our metrics established a benchmark for evaluating model explanation methods in medical images. Our explanations revealed that the classifier relied on clinically relevant radiographic features for its diagnostic decisions, thus making its decision-making process more transparent to the end-user.},
2128}
2129
2130@inproceedings{Zhu2017,
2131 year = {2017},
2132 title = {{Unpaired Image-to-Image Translation Using Cycle-Consistent Adversarial Networks}},
2133 pages = {2242--2251},
2134 isbn = {2380-7504 VO -},
2135 doi = {10.1109/ICCV.2017.244},
2136 booktitle = {2017 IEEE International Conference on Computer Vision (ICCV)},
2137 author = {Zhu, J -Y. and Park, T and Isola, P and Efros, A A},
2138}
2139
2140@article{Khodabandehloo2021,
2141 year = {2021},
2142 volume = {116},
2143 url = {https://www.sciencedirect.com/science/article/pii/S0167739X20330144},
2144 title = {{HealthXAI: Collaborative and explainable AI for supporting early diagnosis of cognitive decline}},
2145 pages = {168--189},
2146 keywords = {Cognitive decline,Explainable artificial intelligence,Pervasive healthcare,Sensor-based activity recognition},
2147 journal = {Future Generation Computer Systems},
2148 issn = {0167-739X},
2149 doi = {https://doi.org/10.1016/j.future.2020.10.030},
2150 author = {Khodabandehloo, Elham and Riboni, Daniele and Alimohammadi, Abbas},
2151 abstract = {Our aging society claims for innovative tools to early detect symptoms of cognitive decline. Several research efforts are being made to exploit sensorized smart-homes and artificial intelligence (AI) methods to detect a decline of the cognitive functions of the elderly in order to promptly alert practitioners. Even though those tools may provide accurate predictions, they currently provide limited support to clinicians in making a diagnosis. Indeed, most AI systems do not provide any explanation of the reason why a given prediction was computed. Other systems are based on a set of rules that are easy to interpret by a human. However, those rule-based systems can cope with a limited number of abnormal situations, and are not flexible enough to adapt to different users and contextual situations. In this paper, we tackle this challenging problem by proposing a flexible AI system to recognize early symptoms of cognitive decline in smart-homes, which is able to explain the reason of predictions at a fine-grained level. Our method relies on well known clinical indicators that consider subtle and overt behavioral anomalies, as well as spatial disorientation and wandering behaviors. In order to adapt to different individuals and situations, anomalies are recognized using a collaborative approach. We experimented our approach with a large set of real world subjects, including people with MCI and people with dementia. We also implemented a dashboard to allow clinicians to inspect anomalies together with the explanations of predictions. Results show that our system's predictions are significantly correlated to the person's actual diagnosis. Moreover, a preliminary user study with clinicians suggests that the explanation capabilities of our system are useful to improve the task performance and to increase trust. To the best of our knowledge, this is the first work that explores data-driven explainable AI for supporting the diagnosis of cognitive decline.},
2152}
2153
2154@article{Chung2022cxr,
2155 year = {2022},
2156 volume = {12},
2157 url = {https://doi.org/10.1038/s41598-022-24721-5},
2158 title = {{Prediction of oxygen requirement in patients with COVID-19 using a pre-trained chest radiograph xAI model: efficient development of auditable risk prediction models via a fine-tuning approach}},
2159 pages = {21164},
2160 number = {1},
2161 journal = {Scientific Reports},
2162 issn = {2045-2322},
2163 doi = {10.1038/s41598-022-24721-5},
2164 author = {Chung, Joowon and Kim, Doyun and Choi, Jongmun and Yune, Sehyo and Song, Kyoung Doo and Kim, Seonkyoung and Chua, Michelle and Succi, Marc D and Conklin, John and Longo, Maria G Figueiro and Ackman, Jeanne B and Petranovic, Milena and Lev, Michael H and Do, Synho},
2165 abstract = {Risk prediction requires comprehensive integration of clinical information and concurrent radiological findings. We present an upgraded chest radiograph (CXR) explainable artificial intelligence (xAI) model, which was trained on 241,723 well-annotated CXRs obtained prior to the onset of the COVID-19 pandemic. Mean area under the receiver operating characteristic curve (AUROC) for detection of 20 radiographic features was 0.955 },
2166}
2167
2168@article{Bucker2022,
2169 year = {2022},
2170 volume = {73},
2171 url = {https://doi.org/10.1080/01605682.2021.1922098},
2172 title = {{Transparency, auditability, and explainability of machine learning models in credit scoring}},
2173 publisher = {Taylor & Francis},
2174 pages = {70--90},
2175 number = {1},
2176 month = {jan},
2177 journal = {Journal of the Operational Research Society},
2178 issn = {0160-5682},
2179 doi = {10.1080/01605682.2021.1922098},
2180 author = {B{\"{u}}cker, Michael and Szepannek, Gero and Gosiewska, Alicja and Biecek, Przemyslaw},
2181 annote = {doi: 10.1080/01605682.2021.1922098},
2182}
2183
2184@article{Padovan2023,
2185 year = {2023},
2186 volume = {31},
2187 url = {https://doi.org/10.1007/s10506-022-09308-9},
2188 title = {{Black is the new orange: how to determine AI liability}},
2189 pages = {133--167},
2190 number = {1},
2191 journal = {Artificial Intelligence and Law},
2192 issn = {1572-8382},
2193 doi = {10.1007/s10506-022-09308-9},
2194 author = {Padovan, Paulo Henrique and Martins, Clarice Marinho and Reed, Chris},
2195 abstract = {Autonomous artificial intelligence (AI) systems can lead to unpredictable behavior causing loss or damage to individuals. Intricate questions must be resolved to establish how courts determine liability. Until recently, understanding the inner workings of “black boxes” has been exceedingly difficult; however, the use of Explainable Artificial Intelligence (XAI) would help simplify the complex problems that can occur with autonomous AI systems. In this context, this article seeks to provide technical explanations that can be given by XAI, and to show how suitable explanations for liability can be reached in court. It provides an analysis of whether existing liability frameworks, in both civil and common law tort systems, with the support of XAI, can address legal concerns related to AI. Lastly, it claims their further development and adoption should allow AI liability cases to be decided under current legal and regulatory rules until new liability regimes for AI are enacted.},
2196}
2197
2198@article{felzmann2020towards,
2199 publisher = {Springer},
2200 year = {2020},
2201 pages = {3333--3361},
2202 number = {6},
2203 volume = {26},
2204 journal = {Science and Engineering Ethics},
2205 author = {Felzmann, Heike and Fosch-Villaronga, Eduard and Lutz, Christoph and Tam{\`o}-Larrieux, Aurelia},
2206 title = {Towards transparency by design for artificial intelligence},
2207}
2208
2209@inproceedings{zhao2021,
2210 month = {oct},
2211 address = {Los Alamitos, CA, USA},
2212 publisher = {IEEE Computer Society},
2213 url = {https://doi.ieeecomputersociety.org/10.1109/ICCV48922.2021.00072},
2214 doi = {10.1109/ICCV48922.2021.00072},
2215 keywords = {privacy;semantics;data visualization;medical services;predictive models;data models;artificial intelligence},
2216 abstract = {The successful deployment of artificial intelligence (AI) in many domains from healthcare to hiring requires their responsible use, particularly in model explanations and privacy. Explainable artificial intelligence (XAI) provides more information to help users to understand model decisions, yet this additional knowledge exposes additional risks for privacy attacks. Hence, providing explanation harms privacy. We study this risk for image-based model inversion attacks and identified several attack architectures with increasing performance to reconstruct private image data from model explanations. We have developed several multi-modal transposed CNN architectures that achieve significantly higher inversion performance than using the target model prediction only. These XAI-aware inversion models were designed to exploit the spatial knowledge in image explanations. To understand which explanations have higher privacy risk, we analyzed how various explanation types and factors influence inversion performance. In spite of some models not providing explanations, we further demonstrate increased inversion performance even for non-explainable target models by exploiting explanations of surrogate models through attention transfer. This method first inverts an explanation from the target prediction, then reconstructs the target image. These threats highlight the urgent and significant privacy risks of explanations and calls attention for new privacy preservation techniques that balance the dual-requirement for AI explainability and privacy.},
2217 pages = {662-672},
2218 issn = {},
2219 volume = {},
2220 year = {2021},
2221 title = {Exploiting Explanations for Model Inversion Attacks},
2222 booktitle = {2021 IEEE/CVF International Conference on Computer Vision (ICCV)},
2223 author = {X. Zhao and W. Zhang and X. Xiao and B. Lim},
2224}
2225
2226@article{Yan2023,
2227 year = {2023},
2228 volume = {632},
2229 url = {https://www.sciencedirect.com/science/article/pii/S002002552300316X},
2230 title = {{Explanation leaks: Explanation-guided model extraction attacks}},
2231 pages = {269--284},
2232 keywords = {Black-box,Explainable artificial intelligence,Model extraction attacks},
2233 journal = {Information Sciences},
2234 issn = {0020-0255},
2235 doi = {https://doi.org/10.1016/j.ins.2023.03.020},
2236 author = {Yan, Anli and Huang, Teng and Ke, Lishan and Liu, Xiaozhang and Chen, Qi and Dong, Changyu},
2237 abstract = {Explainable artificial intelligence (XAI) is gradually becoming a key component of many artificial intelligence systems. However, such pursuit of transparency may bring potential privacy threats to the model confidentially, as the adversary may obtain more critical information about the model. In this paper, we systematically study how model decision explanations impact model extraction attacks, which aim at stealing the functionalities of a black-box model. Based on the threat models we formulated, an XAI-aware model extraction attack (XaMEA), a novel attack framework that exploits spatial knowledge from decision explanations is proposed. XaMEA is designed to be model-agnostic: it achieves considerable extraction fidelity on arbitrary machine learning (ML) models. Moreover, we proved that this attack is inexorable, even if the target model does not proactively provide model explanations. Various empirical results have also verified the effectiveness of XaMEA and disclosed privacy leakages caused by decision explanations. We hope this work would highlight the need for techniques that better trade off the transparency and privacy of ML models.},
2238}
2239
2240@article{Saldanha2022,
2241 year = {2022},
2242 volume = {28},
2243 url = {https://doi.org/10.1038/s41591-022-01768-5},
2244 title = {{Swarm learning for decentralized artificial intelligence in cancer histopathology}},
2245 pages = {1232--1239},
2246 number = {6},
2247 journal = {Nature Medicine},
2248 issn = {1546-170X},
2249 doi = {10.1038/s41591-022-01768-5},
2250 author = {Saldanha, Oliver Lester and Quirke, Philip and West, Nicholas P and James, Jacqueline A and Loughrey, Maurice B and Grabsch, Heike I and Salto-Tellez, Manuel and Alwers, Elizabeth and Cifci, Didem and {Ghaffari Laleh}, Narmin and Seibel, Tobias and Gray, Richard and Hutchins, Gordon G A and Brenner, Hermann and van Treeck, Marko and Yuan, Tanwei and Brinker, Titus J and Chang-Claude, Jenny and Khader, Firas and Schuppert, Andreas and Luedde, Tom and Trautwein, Christian and Muti, Hannah Sophie and Foersch, Sebastian and Hoffmeister, Michael and Truhn, Daniel and Kather, Jakob Nikolas},
2251 abstract = {Artificial intelligence (AI) can predict the presence of molecular alterations directly from routine histopathology slides. However, training robust AI systems requires large datasets for which data collection faces practical, ethical and legal obstacles. These obstacles could be overcome with swarm learning (SL), in which partners jointly train AI models while avoiding data transfer and monopolistic data governance. Here, we demonstrate the successful use of SL in large, multicentric datasets of gigapixel histopathology images from over 5,000 patients. We show that AI models trained using SL can predict BRAF mutational status and microsatellite instability directly from hematoxylin and eosin (H&E)-stained pathology slides of colorectal cancer. We trained AI models on three patient cohorts from Northern Ireland, Germany and the United States, and validated the prediction performance in two independent datasets from the United Kingdom. Our data show that SL-trained AI models outperform most locally trained models, and perform on par with models that are trained on the merged datasets. In addition, we show that SL-based AI models are data efficient. In the future, SL can be used to train distributed AI models for any histopathology image analysis task, eliminating the need for data transfer.},
2252}
2253
2254@article{Raza2022,
2255 year = {2022},
2256 volume = {236},
2257 url = {https://www.sciencedirect.com/science/article/pii/S0950705121009862},
2258 title = {{Designing ECG monitoring healthcare system with federated transfer learning and explainable AI}},
2259 pages = {107763},
2260 keywords = {Deep learning,Electrocardiography (ECG),Explainable AI (XAI),Federated learning,Privacy,Security},
2261 journal = {Knowledge-Based Systems},
2262 issn = {0950-7051},
2263 doi = {https://doi.org/10.1016/j.knosys.2021.107763},
2264 author = {Raza, Ali and Tran, Kim Phuc and Koehl, Ludovic and Li, Shujun},
2265 abstract = {Deep learning plays a vital role in classifying different arrhythmias using electrocardiography (ECG) data. Nevertheless, training deep learning models normally requires a large amount of data and can lead to privacy concerns. Unfortunately, a large amount of healthcare data cannot be easily collected from a single silo. Additionally, deep learning models are like black-box, with no explainability of the predicted results, which is often required in clinical healthcare. This limits the application of deep learning in real-world health systems. In this paper, to address the above-mentioned challenges, we design a novel end-to-end framework in a federated setting for ECG-based healthcare using explainable artificial intelligence (XAI) and deep convolutional neural networks (CNN). The federated setting is used to solve challenges such as data availability and privacy concerns. Furthermore, the proposed framework effectively classifies different arrhythmias using an autoencoder and a classifier, both based on a CNN. Additionally, we propose an XAI-based module on top of the proposed classifier for interpretability of the classification results, which helps clinical practitioners to interpret the predictions of the classifier and to make quick and reliable decisions. The proposed framework was trained and tested using the baseline Massachusetts Institute of Technology - Boston's Beth Israel Hospital (MIT-BIH) Arrhythmia database. The trained classifier outperformed existing work by achieving accuracy up to 94.5% and 98.9% for arrhythmia detection using noisy and clean data, respectively, with five-fold cross-validation. We also propose a new communication cost reduction method to reduce the communication costs and to enhance the privacy of users' data in the federated setting. While the proposed framework was tested and validated for ECG classification, it is general enough to be extended to many other healthcare applications.},
2266}
2267
2268@misc{Bozorgpanah2022,
2269 year = {2022},
2270 volume = {10},
2271 title = {{Privacy and Explainability: The Effects of Data Protection on Shapley Values}},
2272 number = {6},
2273 keywords = {Shapley values,anonymization,data protection,explainability,machine learning,masking},
2274 isbn = {2227-7080},
2275 doi = {10.3390/technologies10060125},
2276 booktitle = {Technologies},
2277 author = {Bozorgpanah, Aso and Torra, Vicen{\c{c}} and Aliahmadipour, Laya},
2278 abstract = {There is an increasing need to provide explainability for machine learning models. There are different alternatives to provide explainability, for example, local and global methods. One of the approaches is based on Shapley values. Privacy is another critical requirement when dealing with sensitive data. Data-driven machine learning models may lead to disclosure. Data privacy provides several methods for ensuring privacy. In this paper, we study how methods for explainability based on Shapley values are affected by privacy methods. We show that some degree of protection still permits to maintain the information of Shapley values for the four machine learning models studied. Experiments seem to indicate that among the four models, Shapley values of linear models are the most affected ones.},
2279}
2280
2281@article{Gaudio2023,
2282 year = {2023},
2283 volume = {n/a},
2284 url = {https://doi.org/10.1002/widm.1495},
2285 title = {{DeepFixCX: Explainable privacy-preserving image compression for medical image analysis}},
2286 publisher = {John Wiley & Sons, Ltd},
2287 pages = {e1495},
2288 number = {n/a},
2289 month = {mar},
2290 keywords = {compression,deep networks,explainability,medical image analysis,privacy,wavelets},
2291 journal = {WIREs Data Mining and Knowledge Discovery},
2292 issn = {1942-4787},
2293 doi = {https://doi.org/10.1002/widm.1495},
2294 author = {Gaudio, Alex and Smailagic, Asim and Faloutsos, Christos and Mohan, Shreshta and Johnson, Elvin and Liu, Yuhao and Costa, Pedro and Campilho, Aur{\'{e}}lio},
2295 annote = {https://doi.org/10.1002/widm.1495},
2296 abstract = {Abstract Explanations of a model's biases or predictions are essential to medical image analysis. Yet, explainable machine learning approaches for medical image analysis are challenged by needs to preserve privacy of patient data, and by current trends in deep learning to use unsustainably large models and large datasets. We propose DeepFixCX for explainable and privacy-preserving medical image compression that is nimble and performant. We contribute a review of the field and a conceptual framework for simultaneous privacy and explainability via tools of compression. DeepFixCX compresses images without learning by removing or obscuring spatial and edge information. DeepFixCX is ante-hoc explainable and gives privatized post hoc explanations of spatial and edge bias without accessing the original image. DeepFixCX privatizes images to prevent image reconstruction and mitigate patient re-identification. DeepFixCX is nimble. Compression can occur on a laptop CPU or GPU to compress and privatize 1700 images per second of size 320???320. DeepFixCX enables use of low memory MLP classifiers for vision data; permitting small performance loss gives end-to-end MLP performance over 70? faster and batch size over 100? larger. DeepFixCX consistently improves predictive classification performance of a Deep Neural Network (DNN) by 0.02 AUC ROC on Glaucoma and Cervix Type detection datasets, and can improve multi-label chest x-ray classification performance in seven of 10 tested settings. In all three datasets, compression to less than 5% of original number of pixels gives matching or improved performance. Our main novelty is to define an explainability versus privacy problem and address it with lossy compression. This article is categorized under: Fundamental Concepts of Data and Knowledge > Explainable AI Commercial, Legal, and Ethical Issues > Security and Privacy Fundamental Concepts of Data and Knowledge > Big Data Mining},
2297}
2298
2299@article{Jia2022xaihealthcare,
2300 year = {2022},
2301 volume = {10},
2302 title = {{The Role of Explainability in Assuring Safety of Machine Learning in Healthcare}},
2303 pages = {1746--1760},
2304 number = {4},
2305 journal = {IEEE Transactions on Emerging Topics in Computing},
2306 issn = {2168-6750 VO - 10},
2307 doi = {10.1109/TETC.2022.3171314},
2308 author = {Jia, Y and McDermid, J and Lawton, T and Habli, I},
2309}
2310
2311@article{Atif2022,
2312 year = {2022},
2313 volume = {3},
2314 title = {{Robust Traffic Sign Recognition Against Camera Failures}},
2315 pages = {709--722},
2316 journal = {IEEE Open Journal of Intelligent Transportation Systems},
2317 issn = {2687-7813 VO - 3},
2318 doi = {10.1109/OJITS.2022.3213183},
2319 author = {Atif, M and Ceccarelli, A and Zoppi, T and Gharib, M and Bondavalli, A},
2320}
2321
2322@inproceedings{AlexNet,
2323 series = {NIPS'12},
2324 location = {Lake Tahoe, Nevada},
2325 numpages = {9},
2326 pages = {1097–1105},
2327 booktitle = {Proceedings of the 25th International Conference on Neural Information Processing Systems - Volume 1},
2328 abstract = {We trained a large, deep convolutional neural network to classify the 1.2 million high-resolution images in the ImageNet LSVRC-2010 contest into the 1000 different classes. On the test data, we achieved top-1 and top-5 error rates of 37.5},
2329 address = {Red Hook, NY, USA},
2330 publisher = {Curran Associates Inc.},
2331 year = {2012},
2332 title = {ImageNet Classification with Deep Convolutional Neural Networks},
2333 author = {Krizhevsky, Alex and Sutskever, Ilya and Hinton, Geoffrey E.},
2334}
2335
2336@article{Houda2022,
2337 year = {2022},
2338 volume = {3},
2339 title = {{“Why Should I Trust Your IDS?”: An Explainable Deep Learning Framework for Intrusion Detection Systems in Internet of Things Networks}},
2340 pages = {1164--1176},
2341 journal = {IEEE Open Journal of the Communications Society},
2342 issn = {2644-125X VO - 3},
2343 doi = {10.1109/OJCOMS.2022.3188750},
2344 author = {Houda, Z A E and Brik, B and Khoukhi, L},
2345}
2346
2347@article{Sado2023,
2348 keywords = {explainability, explainable AI, goal-driven agents, Accountability, deep neural network, transparency, continual learning},
2349 numpages = {41},
2350 articleno = {211},
2351 month = {feb},
2352 journal = {ACM Comput. Surv.},
2353 abstract = {Recent applications of autonomous agents and robots have brought attention to crucial trust-related challenges associated with the current generation of artificial intelligence (AI) systems. AI systems based on the connectionist deep learning neural network approach lack capabilities of explaining their decisions and actions to others, despite their great successes. Without symbolic interpretation capabilities, they are ‘black boxes’, which renders their choices or actions opaque, making it difficult to trust them in safety-critical applications. The recent stance on the explainability of AI systems has witnessed several approaches to eXplainable Artificial Intelligence (XAI); however, most of the studies have focused on data-driven XAI systems applied in computational sciences. Studies addressing the increasingly pervasive goal-driven agents and robots are sparse at this point in time. This paper reviews approaches on explainable goal-driven intelligent agents and robots, focusing on techniques for explaining and communicating agents’ perceptual functions (e.g., senses, vision) and cognitive reasoning (e.g., beliefs, desires, intentions, plans, and goals) with humans in the loop. The review highlights key strategies that emphasize transparency, understandability, and continual learning for explainability. Finally, the paper presents requirements for explainability and suggests a road map for the possible realization of effective goal-driven explainable agents and robots.},
2354 doi = {10.1145/3564240},
2355 url = {https://doi-org.elibrary.jcu.edu.au/10.1145/3564240},
2356 issn = {0360-0300},
2357 number = {10},
2358 volume = {55},
2359 address = {New York, NY, USA},
2360 publisher = {ACM},
2361 issue_date = {October 2023},
2362 year = {2023},
2363 title = {Explainable Goal-Driven Agents and Robots - A Comprehensive Review},
2364 author = {Sado, Fatai and Loo, Chu Kiong and Liew, Wei Shiung and Kerzel, Matthias and Wermter, Stefan},
2365}
2366
2367@article{clerc2018sex,
2368 publisher = {SAGE Publications Sage UK: London, England},
2369 year = {2018},
2370 pages = {1745506518805641},
2371 volume = {14},
2372 journal = {Women's health},
2373 author = {Clerc Liaudat, Christelle and Vaucher, Paul and De Francesco, Tommaso and Jaunin-Stalder, Nicole and Herzig, Lilli and Verdon, Fran{\c{c}}ois and Favrat, Bernard and Locatelli, Isabella and Clair, Carole},
2374 title = {Sex/gender bias in the management of chest pain in ambulatory care},
2375}
2376
2377@article{Jiang2023,
2378 year = {2023},
2379 volume = {57},
2380 url = {https://doi.org/10.1002/jmri.28427},
2381 title = {{Performance of Artificial Intelligence-Aided Diagnosis System for Clinically Significant Prostate Cancer with MRI: A Diagnostic Comparison Study}},
2382 publisher = {John Wiley & Sons, Ltd},
2383 pages = {1352--1364},
2384 number = {5},
2385 month = {may},
2386 keywords = {artificial intelligence,biparametric MRI,clinically significant prostate cancer,deep learning,the Prostate Imaging Reporting and Data System},
2387 journal = {Journal of Magnetic Resonance Imaging},
2388 issn = {1053-1807},
2389 doi = {https://doi.org/10.1002/jmri.28427},
2390 author = {Jiang, Ke-Wen and Song, Yang and Hou, Ying and Zhi, Rui and Zhang, Jing and Bao, Mei-Ling and Li, Hai and Yan, Xu and Xi, Wei and Zhang, Cheng-Xiu and Yao, Ye-Feng and Yang, Guang and Zhang, Yu-Dong},
2391 annote = {https://doi.org/10.1002/jmri.28427},
2392 abstract = {Background The high level of expertise required for accurate interpretation of prostate MRI. Purpose To develop and test an artificial intelligence (AI) system for diagnosis of clinically significant prostate cancer (CsPC) with MRI. Study Type Retrospective. Subjects One thousand two hundred thirty patients from derivation cohort between Jan 2012 and Oct 2019, and 169 patients from a publicly available data (U-Net: 423 for training/validation and 49 for test and TrumpeNet: 820 for training/validation and 579 for test). Field Strength/Sequence 3.0T/scanners, T2-weighted imaging (T2WI), diffusion-weighted imaging, and apparent diffusion coefficient map. Assessment Close-loop AI system was trained with an Unet for prostate segmentation and a TrumpetNet for CsPC detection. Performance of AI was tested in 410 internal and 169 external sets against 24 radiologists categorizing into junior, general and subspecialist group. Gleason score?>6 was identified as CsPC at pathology. Statistical Tests Area under the receiver operating characteristic curve (AUC-ROC); Delong test; Meta-regression I2 analysis. Results In average, for internal test, AI had lower AUC-ROC than subspecialists (0.85 vs. 0.92, P?<?0.05), and was comparable to junior (0.84, P = 0.76) and general group (0.86, P = 0.35). For external test, both AI (0.86) and subspecialist (0.86) had higher AUC than junior (0.80, P?<?0.05) and general reader (0.83, P?<?0.05). In individual, it revealed moderate diagnostic heterogeneity in 24 readers (Mantel?Haenszel I},
2393}
2394
2395@article{Garb2021,
2396 year = {2021},
2397 volume = {90},
2398 url = {https://www.sciencedirect.com/science/article/pii/S0272735821001306},
2399 title = {{Race bias and gender bias in the diagnosis of psychological disorders}},
2400 pages = {102087},
2401 keywords = {Clinical judgment,Cultural diversity,Diagnosis,Gender bias,Race bias},
2402 journal = {Clinical Psychology Review},
2403 issn = {0272-7358},
2404 doi = {https://doi.org/10.1016/j.cpr.2021.102087},
2405 author = {Garb, Howard N},
2406 abstract = {Bias is said to occur when validity is better for one group than another (e.g., when diagnoses are more valid for male or female clients). This article provides (a) a methodological critique of studies on race bias and gender bias in diagnosis and (b) a narrative review of results from studies with good internal validity. The results suggest that race bias occurs for the diagnosis of conduct disorder, antisocial personality disorder, comorbid substance abuse and mood disorders, eating disorders, posttraumatic stress disorder, and the differential diagnosis of schizophrenia and psychotic affective disorders. Other results suggest that gender bias occurs for the diagnosis of autism spectrum disorder, attention deficit hyperactivity disorder, conduct disorder, and antisocial and histrionic personality disorders. The way that symptoms are expressed (e.g., Black cultural expressions of depression) appears to have a significant effect on diagnoses. It may be possible to decrease bias by expanding the use of (a) mental health screening, (b) self-report measures including some psychological tests, (c) structured interviews, and (d) statistical prediction rules. Finally, evidence exists that (a) the diagnosis of personality disorders should be made using dimensional ratings and (b) training in cultural diversity and debiasing strategies should be provided to mental health professionals.},
2407}
2408
2409@article{Bigman2020,
2410 year = {2020},
2411 volume = {579},
2412 url = {https://doi.org/10.1038/s41586-020-1987-4},
2413 title = {{Life and death decisions of autonomous vehicles}},
2414 pages = {E1--E2},
2415 number = {7797},
2416 journal = {Nature},
2417 issn = {1476-4687},
2418 doi = {10.1038/s41586-020-1987-4},
2419 author = {Bigman, Yochanan E and Gray, Kurt},
2420}
2421
2422@article{Awad2018,
2423 year = {2018},
2424 volume = {563},
2425 url = {https://doi.org/10.1038/s41586-018-0637-6},
2426 title = {{The Moral Machine experiment}},
2427 pages = {59--64},
2428 number = {7729},
2429 journal = {Nature},
2430 issn = {1476-4687},
2431 doi = {10.1038/s41586-018-0637-6},
2432 author = {Awad, Edmond and Dsouza, Sohan and Kim, Richard and Schulz, Jonathan and Henrich, Joseph and Shariff, Azim and Bonnefon, Jean-Fran{\c{c}}ois and Rahwan, Iyad},
2433 abstract = {With the rapid development of artificial intelligence have come concerns about how machines will make moral decisions, and the major challenge of quantifying societal expectations about the ethical principles that should guide machine behaviour. To address this challenge, we deployed the Moral Machine, an online experimental platform designed to explore the moral dilemmas faced by autonomous vehicles. This platform gathered 40 million decisions in ten languages from millions of people in 233 countries and territories. Here we describe the results of this experiment. First, we summarize global moral preferences. Second, we document individual variations in preferences, based on respondents' demographics. Third, we report cross-cultural ethical variation, and uncover three major clusters of countries. Fourth, we show that these differences correlate with modern institutions and deep cultural traits. We discuss how these preferences can contribute to developing global, socially acceptable principles for machine ethics. All data used in this article are publicly available.},
2434}
2435
2436@inproceedings{Khurana2021,
2437 year = {2021},
2438 title = {{ChatrEx: Designing Explainable Chatbot Interfaces for Enhancing Usefulness, Transparency, and Trust}},
2439 pages = {1--11},
2440 isbn = {1943-6106 VO -},
2441 doi = {10.1109/VL/HCC51201.2021.9576440},
2442 booktitle = {2021 IEEE Symposium on Visual Languages and Human-Centric Computing (VL/HCC)},
2443 author = {Khurana, A and Alamzadeh, P and Chilana, P K},
2444}
2445
2446@article{Saiz-Rubio2020,
2447 year = {2020},
2448 volume = {10},
2449 title = {{From smart farming towards agriculture 5.0: A review on crop data management}},
2450 number = {2},
2451 journal = {Agronomy},
2452 doi = {10.3390/agronomy10020207},
2453 author = {Saiz-Rubio, V and Rovira-M{\'{a}}s, F},
2454 annote = {Export Date: 25 April 2023; Cited By: 270},
2455}
2456
2457@article{bakerAIOT,
2458 doi = {10.1109/COMST.2023.3256323},
2459 pages = {1261-1293},
2460 number = {2},
2461 volume = {25},
2462 year = {2023},
2463 title = {Artificial Intelligence of Things for Smarter Healthcare: A Survey of Advancements, Challenges, and Opportunities},
2464 journal = {IEEE Communications Surveys \& Tutorials},
2465 author = {Baker, Stephanie and Xiang, Wei},
2466}
Attribution
arXiv:2312.01555v1
[cs.AI]
License: cc-by-4.0