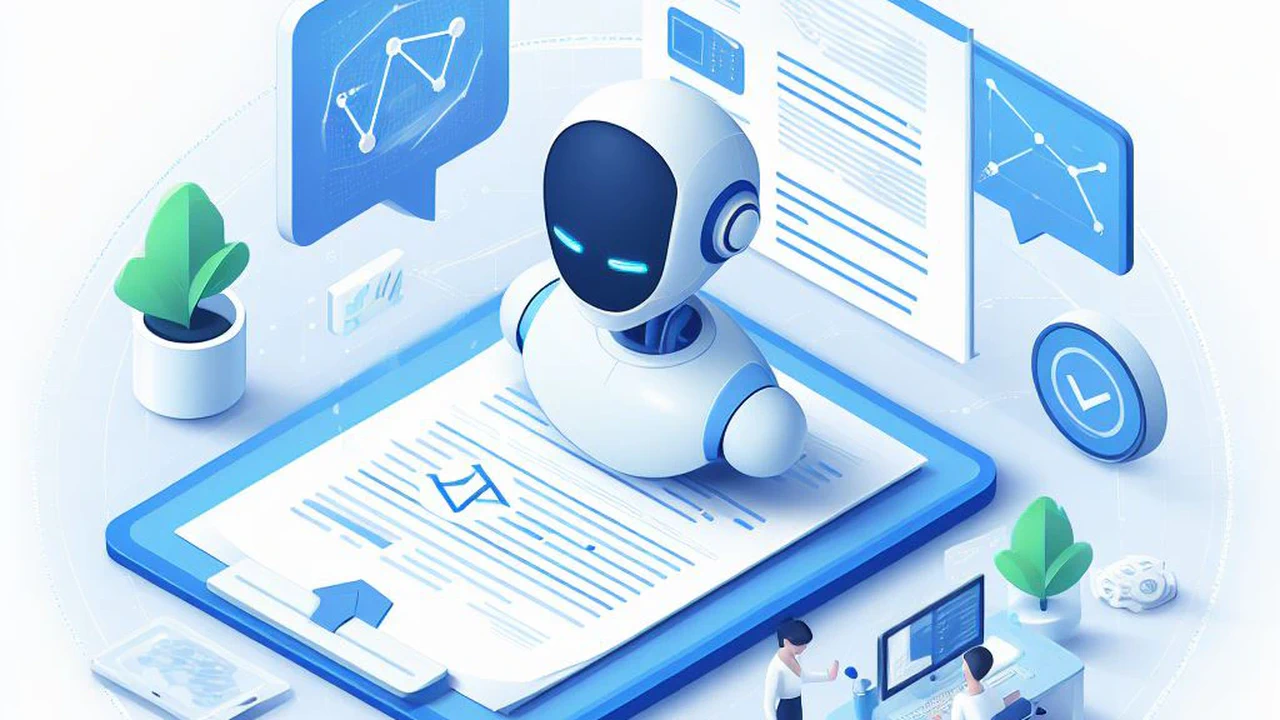
- Papers
Papers is Alpha. This content is part of an effort to make research more accessible, and (most likely) has lost some details from the original. You can find the original paper here.
Introduction
Artificial Intelligence (AI) has taken a `participatory turn’, with the reasoning that participation provides a means to incorporate wider publics into the development and deployment of AI systems. The greater attention to participatory methods, participatory design, and the emerging imaginary of participatory AI, follows as a response to the changing attitudes towards AI’s role in our societies, in light of the documented harms that have emerged in the areas of security, justice, employment, and healthcare, among others. The field of artificial intelligence is faced with the need to evolve its development practices—characterized currently as technically-focused, representationally imbalanced, and non-participatory—if it is to meet the optimistic vision of AI intended to deeply support human agency and enhance prosperity. Participation has a vital role to play in aligning AI towards prosperity, especially of the most marginalized, but requires a deeper interrogation of its scope and limitations, uses and misuses, and the place of participation within the broader AI development ecosystem.
A growing body of work has shown the different roles and formats that participation can take in the development of AI, including: new approaches to technical development in NLP in healthcare, in the development of alternative design toolkits and processes, and methods that range from structured interviews to citizens juries. In these cases, participation is meant to move beyond individual opinion to center the values of inclusion, plurality, collective safety, and ownership, subsequently shifting the relationship from one of designer-and-user to one of co-designers and co-creators. Participation is expected to lead to systems of self-determination and community empowerment. Yet, caution has been raised about the possibility of `participation-washing’, where efforts are mischaracterized under the banner of participation, are weakly-executed, or co-opt the voice of participants to achieve predetermined aims.
In this paper, we advance a view that participation should continue to grow and be refined as a key component of the AI development and deployment lifecycle as a means to empower communities, especially those at the margins of society that AI often disproportionately and negatively impacts. To achieve this through Participatory AI, greater clarity is needed on what participation is, who it is supposed to serve, how it can be used in the specific context of AI, and how it is related to the mechanisms and approaches already available. Our paper makes three contributions towards developing this deeper understanding of participatory AI. Firstly, we situate the participatory process within its broader genealogical development. We develop this historical hindsight in section sect-history, considering histories of participatory development as well as its colonial inheritances and its newer digital forms. Secondly, we present three case studies in section sect-casestudies taken from selected participatory projects to further concretize various forms of existing participatory work. we reframe participation by introducing a characterisation that allows the multiple forms of participation seen in practice to be compared in Appendix B. We then describe potential limitations and concerns of participation in section sect-limitations and conclude in Section sect-conclusion.
Genealogy of Participation
“‘je participe, tu participes, il participe, nous participons, vous participez … ils profitent’ (in English: ‘I participate, you participate, he participates, we participate, you participate … they profit’ )”. [From a French poster by Atelier Populaire,May 1968. V&A Museum collections accession number E.784-2003 ]
This quote appears widely in works related to participation—it poetically captures the cycles of enthusiasm and use of participation and participatory methods as a remedy for many problems of social life, but ending with a sense of disenchantment, exploitation, and with asymmetrical power dynamics in society left unchanged. We see the same enthusiasm for participation in AI at present, which renews this quote’s relevance for the analysis of participatory approaches in AI. The quote points to the many historical lessons upon which new participatory undertakings can draw from, which is often absent in AI research; it also serves as a warning of one type of participatory outcome to avoid. To draw on this experience, in this section, we begin with a summary of participation’s historical roots, look specifically at participation for technological development, and then review the current landscape of participatory AI.
Historical Participation
Over the last century, participatory approaches have risen globally to the fore across all sectors, including in international development, healthcare provision, decision-making about science, democracy, the environment, and social movements for self-determination, among others. This rise is driven by the multitude of benefits associated with participation. Participatory approaches, by engaging citizens in scientific, democratic or environmental decision-making, for example, enables these processes to become transparent, accountable, and responsive to the needs of those who participate. Participatory methods also establish a distinct opportunity to learn from and include knowledge created by the people directly affected by existing or new undertakings. When such collective knowledge is missing, its absence leads to failure leaving projects to be based solely upon technocratic or elite perspectives. Moreover, at its best, participation leads to individual and collective empowerment as well as social and structural change via the cultivation of new skills, social capital, networks, and self-determination among those who contribute. This has the potential to make a sustained positive impact to the welfare and benefit of communities over time.
The desire to unlock these benefits through novel forms of organization played a central role in the development of participatory approaches to research and decision-making, a trend that is most often traced back to the work of Scandinavian researchers in the 1970s and 80s. The ‘Scandinavian approach’ to participation is concerned primarily with the creation of ‘workplace democracy’ understood as a system of structured consultation and dialogue between workers and employees with the aim of giving workers greater control over wages and the allocation of tasks. Building upon this idea, participatory approaches have been used to countenance different kinds of response to the challenges posed to workers by technological innovation. As examples, the Scandanavian Collective Resource Approach helps workers actively manage processes of technological adoption by promoting knowledge-sharing about new technologies, improving the ability of unions to negotiate collectively with employers, and identify mutually beneficial trajectories. The British Socio-Technical Systems Approach to participation was developed to promote the notion of a systems science where new technologies, the workers (with emphasis on their psychological and physical well-being), and the environment that they are embedded in, are held to be an interactive part of a larger system that needs to be collectively managed. The latter school of thought set out to promote workers’ autonomy through their active participation in the design of socio-technical systems as a whole.
The power of participation has led to a proliferation of approaches, including the principle of maximum feasible participation, and one of the most regarded uses in the process of participatory budgeting. Today there are numerous tools and processes available for anyone to build on the established practices across a range of participatory methods, whether they include Delphi interviews, citizen’s juries, role-playing exercises and scenarios,workshops, force field analyses, or visual imagery (like those in the participatory development toolkit), alongside the professionalization of participation through organisations like the International Association for Public Participation.
Despite these advancements, historical analysis of the roots of participation reveal some of its failings and shortcomings. Long before calls for participation in the workplace, the notion of participation played a central role in the administration of the British empire. The “Dual Mandate in British Tropical Africa” colonial system of the 1920s was rooted in the co-optation of traditional rules and authority structures. By establishing a hierarchical division of power that was enforced using the authority of local rules and chiefs, colonial projects claimed their legitimacy under the veneer of participation. They mandated local people to abide by colonial rules, turning participation in governance into a form of colonial power.
The risk that participation could simply mask uneven power relations, making it easier to perpetuate a dynamic that is fundamentally extractive remains a major concern. One of the most astute critique in this vein was raised bythat established the now widespread image of the ladder of participation that provided a linear model with which to characterise participation, from extractive to empowering. Although the ladder was powerful, the key critiqueraised was one of power. And this critique has been extended further, labelling the fervour for participation in all sectors as a form tyranny, pushing out other approaches for equity that would be more appropriate while using participatory methods to facilitate an illegitimate and unjust exercise of power. Aswrites:“… participation without redistribution of power is an empty and frustrating process for the powerless. It allows the powerholders to claim that all sides were considered, but makes it possible for only some of those sides to benefit. It maintains the status quo.”
Participation for Technological Innovation
Of relevance to machine learning research, are the specific roles that calls for participation have played in the context of computing and technological innovation. In the U.S., participatory design was widely adopted by large corporations creating office technologies in the 1970s and 80s. The key idea was to increase the quality of products, and to reduce time and costs, by bridging the gap between those doing the design (removed from day to day use) and those that were designed for by involving end-users in the process. Participation in this sense was primarily conceived of as technical work done by participants for the sake of economic efficiency; an aspiration that fit well with larger organizational goals. While this approach yielded some benefits for consumers via an improved product, the value of participation was limited: it did not need to benefit those engaged in co-design and was very unlikely to empower them.
One salient question centers upon whether participation in the technology development pipeline necessarily requires those involved to be actively engaged in the process. For those who focus on participation in the form of activism, movement-building and community initiatives, activeengagement is essential. Yet, othersdefine participation more widely, so that it encompasses types of incidental participation that arises by simply being part of an environment or process that involves others. This phenomenon is increasingly pronounced in digital media environments, e.g., having one’s data harvested and used in AI development by virtue of “participating” in social media, sometimes referred to as mediated participation, participation through the medium of technology. Yet this type of “passive” participation has increasingly been linked to privacy violations and surveillance.Furthermore, to support the ideal of a “participatory condition” of society and technological development requires a degree of agency and intentionality. To participate, requires knowing that one is participating.
The Emergence of Participatory AI
While the participatory mechanisms have served as a constant backdrop for the development of modern technologies, it’s emergence within the context of artificial intelligence (AI) and machine learning based applications specifically have been relatively recent. Given its origins as a more speculative academic and industrial technological endeavor, the initial cycles of AI research largely missed the prior waves of participatory research that other technologies of comparable vintage (e.g. personal computing, internet networking, computer software). However, the shift away from logic-based AI systems towards more data-driven paradigms such Deep Learningas well as new infrastructure for capturing and leveraging human-generated data (e.g. Amazon Mturk) prompted greater demand for “non-expert” participation in the construction of AI systems.
One significant adoption of non-expert participation was in the construction of large scale benchmark datasets such as ImageNet, where the research team utilized over 49,000 workers from Amazon’s mechanical turk (Mturk) platform across 167 countriesto perform image recognition tasks to filter and validate the roughly 14 million images in the dataset. Despite this effort being quite broad in its “participation”, the highly variable ethical and documentation standardsfor data enrichment or moderation tasks means that these contributors often fail to be discussed when publishing the final artefacts or protected by conventional research participant standards (e.g. Beneficence, Respect for Persons, Justice). Other area has been in the form of content moderation, where non-expert participants are used to review misinformation or graphic media to prohibit the display of harmful content on internet platforms (e.g YouTube, Facebook, Twitter) but also serve as labelled training data for machine learning classifiers deployed to expedite policy enforcement.The proliferation of machine learning across multiple industries has further ingrained and expanded the general data enrichment and moderation paradigm, but the abuses and concentration of these forms of “Ghost Work" in low income countries and peoples have also been extensively documented in recent years.
In parallel to the expansion of data enrichment and moderation, problematic applications of machine learning tools in high stakes domains such as criminal justice, healthcare, and hiringhave prompted both researchers, civil society, and regulators to increasingly urge greater use participatory methods to mitigate sociotechnical risksnot addressed by algorithmic adjustments or transformations. The recent upswing in participatory tools have varied in approach and point in the machine learning product lifecycle, including: auditing methods for machine learning based interventions, public consultation methods such as citizen juriesor joint problem formulation, information disclosures such as model cardsor datasheets, and artefact co-development. A central tension of this this recent wave of “participatory” is whether these mechanisms should merely serve to aid in the refinement of relevant machine learning sytem or rather emphasize lived experience as a critical form of knowledge and employ experiential learning as a force for community empowerment and advance algorithmic equityor ensure wider humanitarian or societal benefits. The heavy influence of industry stakeholders calling for greater participation without resolving these tensions has led to concerns of `participation-washing’ and calls for a greater need to focus on broader social structures and uneven power asymmetries, as well as the limits of participation in specific applications, such as healthcare.
While the advancement of an emergent subfield of “Participatory AI” has its own critical questions and tensions which are important to further contexualize, the field needs to continue to reflect both its instrumental and broader purposes. The sections below focus exploring three specific areas:
Standards: Despite many activities applying the label of participatory, there are yet no clear consensus on what minimum set of standards or dimensions one should use to assess or evaluate a given potential participatory mechanism. Though not an exhaustive list, attributes such as the degree of Reciprocity , Reflexivity , and Empowerment , as well as the Duration of a task are applicable and salient considerations for all participatory mechanisms. Please see the list of questions in Appendix A that further aid the reflexive process for those embarking on participatory activities.
Goals: There is no single unified vision of what Participatory AI tools are intend to achieve, but rather a bundle of overlapping goals and motivations. These include algorithmic performance improvements, process improvements, and collective exploration . This is further explored in the Appendix B. While each of these objectives are participatory to some degree, the composition of the stakeholders and relative degree of influence in ultimately shaping the development and impact of a given machine learning system vary significantly. Thus, researchers and developers must ensure that the forms of participatory mechanisms utilized align with the downstream technical, ethical and sociotechnical risks.
Limitations: Invoking both lessons from history and contemporary cases, we will discuss some emerging limitations of utilizing participatory methods as a means of mitigating technical, ethical and sociotechnical risks. These include concerns of participatory mechanisms serving as a viable substitute for legitimate forms of democratic governance and regulation, co-optation of mechanisms in asymmetrical stakeholder settings, and conflation with other social goals such as inclusion or equity. See Section sect-limitations for more.
Below, we present three “sites” or case studies of Participatory AI across the machine learning lifecycle to explore the substantive areas outlined above. The decision to utilize a case study-based approach is intentional, aiming to provide a deeper understanding of the substantive questions in the context of existing or recent cases. Our hope is that this approach will lend a greater appreciation for the nuance and complexity implementing participatory mechanisms in this setting often presents to all the relevant stakeholders. Each case begins with a background description before presenting a contextual analysis. Through an investigation of existing participatory practices, we aim to offer a wider lens on the use of participatory mechanisms in the AI/ML context, where those goals can be attained through participatory approaches, and a clear understanding of potential limitations such that future efforts can hopefully fully realize the impact of meaningfully incorporating participation in AI/ML development and use.
Three Case Studies in Participatory AI
Participatory activities, process, and projects exist in a variety of forms. Within the AI for social good field, for example, participatory activities are evoked as a means to improve AI systems that impact communities where, ideally, impacted groups take part as stakeholders through participatory design and implementation. Participation hasalso been instrumental in designing and building algorithms that serve communities for purposes such as operating on-demand food donation transportation serviceas well as for building tools, for instance, (Turkopticon) that allow low wage workers — Amazon Mechanical Turkers, in this case — to evaluate their relationships with employers and support one another. Similarly, algorithmic tools that optimize worker well-being through participatory design and algorithmic tool building has been put forward by. In collecting, documenting, and archiving, sociocultural data needed to train and validate machine learning systems, participatory approaches can be critical for representing the “non-elites, the grassroots, the marginalized” in datasets, as outlined by. For justice oriented design of technology, participatory approaches provide the means for marginalized communities to organize grassroots movements, challenge structural inequalities and uneven power dynamics allowing communities to build the kind of technology that benefits such communities. Abstaining from participation can also be a form of participation in another related practice , as shown in, who analysed older adults who refused to participate in technological intervention evaluation. Participatory projects, process, and objectives, therefore, are diverse and multifaceted. Below, we present three case studies that illustrate three various models of participation.
Case 1: Machine translation for African languages
Description Over 400 participants from more than 20 countries have been self-organizing through an online community. Some of the projects that have emerged from this community focus on the inclusion of traditionally omitted “low-resourced” African languages within the broader machine translation (MT) research agenda. The projects sought to ensure that MT work for low-resourced languages is driven by communities who speak those languages. There were no prerequisites placed on participation or fixed roles assigned to participants but rather the roles emerged organically and participatory input was sought at every level of the process from language speaking to model building and participants moved fluidly between different roles. The research process was defined collaboratively and iteratively. Meeting agendas were public and democratically voted on. Language data was crowd-sourced, annotated, evaluated, analyzed and interpreted by participants (from participants). The specific project on MT for African languages produced 46 benchmarks for machine translation from English to 39 African languages and from 3 different languages into English.
Analysis
The MT project by the Masakhane NLP community illustrates a grassroots (or bottom up) attempt at using participatory mechanism to build new systems improve the underlying performance of existing NLP systems through the inclusion of traditionally under-resourced African languages. The Masakhane MT project sought to increase the degree of empowerment for the stakeholders involved in the project. In this context, Empowerment reflects the degree of impact the participants have in shaping the participatory relationship, and ultimately the project or product. An empowering process is one that is often Reciprocal : it is bi-directional, emergent, and dynamic, and one where core decisions or artefacts are informed by active participation rather than one based on the idea of placating a community or notions of saviourism.For example, in this case, the idea is to not only crowd-source activities such as crowd-sourcing of language data, participant evaluation of model output, and production of benchmarks but also to create and foster avenues and forums to veto or voice objections.
Having said that, although the project is built on the idea that MT for low-resourced languages should be done by the language speakers themselves, for language speakers, based on community values and interests, it is still possible to see how the research, datasets and tools may be co-opted and monopolized by commercial actors to improve products or models without supporting the broader grassroots effort or the community’s interests or needs. As a result the primary beneficiaries of participatory data sourcing may not be speakers of `low-resourced’ languages but actors with access to such sufficient data and compute resources, thus gaining financial benefits, control and legitimacy off of such participatory efforts.
Case 2: Fighting for Māori data rights
Description Through participatory initiatives that took place over 10 days in 2018 as part of the Te Hiku NLP project, the Māori community in New Zealand both recorded and annotated 300 hours of audio data of the Te Reo Māori language. This is enough data to build tools such as spell-checkers, grammar assistants, speech recognition, and speech-to-text technology. However, although the data originated from the Māori speakers across New Zealand and was annotated and cleaned by the Māori community itself, Western based data sharing/open data initiatives meant that the Māori community had to explicitly prevent corporate entities from getting hold of the dataset. The community thus established the Māori Data Sovereignty Protocolsin order to take control of their data and technology. Sharing their data, the Māori argued, is to invite commercial actors to shape the future of their language through tools developed by those without connection to the language. By not sharing their data, the Māori argue they are able to maintaining their autonomy and right to self-determination. They insist that, if any technology is to be built using such community sourced data, it must directly and primarily benefit the Māori people. Accordingly, such technology needs to be built by the Māori community itself since they hold the expert knowledge and experience of the language.
Analysis
The Māori case study is an illuminating example that brings together participatory mechanisms as means for methodological innovation while offering reciprocity to the relevant stakeholders. It is a process that prioritizes the net benefit of participants, especially those disproportionately impacted by oppressive social structures, who often carry the burdens of negative impacts of technologyand reflecting a fair or proportionate return for the value of the participatory engagement. This is of particularly importance when seeking to utilize participatory mechanisms to achieve methodological innovation, or where the process yields unique insights that can inform new or innovative technological artefacts (as opposed to a means to achieve a particular pre-determined technical objective).
Because the data originates from the language speakers themselves and is annotated and cleaned by the Māori community, existing laws around data sovereigntyoften require that those communities are key decision makers for any downstream applications. In this case, the Māori are committed to the view that any project created using Māori data must directly benefit and needs to be carried out by the Māori themselves. This high degree of reciprocity between stakeholders lead to a case where the needs, goals, benefits and interests of the community is central to participatory mechanism itself. This case study goes further than others by providing avenues for foregrounding reciprocity and refusal (when they are not aligned with the participants values, interests and benefits).
Case 3: Participatory dataset documentation
Description A team of researchers put forward participatory methods and processes for dataset documentation — The Data Card Playbook — which they view as the route to creating responsible AI systems [https://pair-code.github.io/datacardsplaybook/]. According to the team, the Playbook can play a central role in improving dataset quality, validity and reproducibility, all critical aspects of better performing, more accurate, and transparent AI systems. The Playbook comprises of three components – Essentials, Module one, and Module two – all activities supplemented by ready to download templates and instructions. These participatory activities cover guidance ranging from tracking progress, identifying stakeholders, characterizing target audiences for the Data Card, to evaluate and fine-tune documentation, all presented and organized in a detailed and systematic way. The Playbook aims to make datasets accessible to a wider set of stakeholders. The Playbook is presented as a people-centered approach to dataset documentation, subsequently, with the aim of informing and making AI products and research more transparent.
Analysis
This case study encapsulates the kind of participatory activities that support participation as a form of algorithmic performance and/or dataset quality improvement. An indirect benefit of this approach is that mechanisms designed to explore the space of a given artefact or process inevitably offer a potential for Reflexivity , critical evaluation and meaningful feedback. Reflexivity as part of a participatory process is a critical element for improving trust between stakeholders and conveying a sense legitimacy of the ultimate artefact.
However, because the central drive for these specific participatory practices are motivated by objectives such as dataset quality improvement, the participants are assigned pre-defined roles and very clear tasks. Dataset quality and transparent dataset documentation indeed impact the performance and accuracy of AI systems, which can all play a role in the larger goal of fair, inclusive, and just AI systems. Nonetheless, this form of participation focuses on fine-grained activities that come with pre-defined goals and objectives means that there is little room (if at all any) for co-exploring, co-creating, and/or negotiating the larger objectives, reflections, and implication of AI systems. There is no guarantee that an AI system that is created using improved and better datasets with the help of the Data Card Playbook cannot be used in applications that harm, disenfranchise, and unjustly target the most marginalised in society. Computer vision systems that are used to target refugees or marginalized communities in any society, for example, result in a net harm to the targeted regardless of their improved performance. Participation for algorithmic performance improvement is not necessarily equipped to deal with such concerns.
Limitations and Concerns
Like any method, participation has limitations, and we briefly explore these here, and also refer to the large body of work in these topics. Effective participation should serve specific purposes and should not be conflated with other tasks and activities, such as consultation, inclusion, or labour. Moreover, participation cannot be expected to provide a solution for all concerns, and is not a solution for all problems. When used in considered ways, participation can be an important tool in the responsible development of AI. We consider here the role of participation in relation to democracy, its conflation with other activities, concerns on cooptation of participatory activities, the challenges of measuring the effectiveness of participatory efforts, and the challenges of balancing expertise and incentives.
Democratic governance. In democratic societies, it is useful to think of democracy as an apparatus that responds to the right of citizens to determine the shape of practices that govern their lives. Consequently, participation is not the best mechanism for decisions/values/norms that are better decided and codified by democratic institutions, governance and laws. In a democratic system, participants are rights-holders: the people to whom decision-makers are accountable, and the body in which authority is ultimately vested. This distinction is important when an undertaking involves matters of significant public concern or modalities of operation, such as the coercive enforcement of law, that require stronger forms of validation and legitimacy. Participatory activities convened by private actors or parallel institutions, cannot stand in for democratic politics, and participatory AI should not aspire to do so or be perceived to meet this function.
Conflation with other activities. By acknowledging participation’s limitations, we can refine what it does and does not entail. As one example, inclusion is often conflated with participation. Being included might have practical consequences on the ability of people and groups to engage in participatory processes. But inclusion is not necessarily participation, since any individual can be included in a group, yet not participate, e.g., by never voting, writing, or acting. Inclusion is then related, but in some ways different from participation, and needs its own attention, which also depends on an understanding of any systemic and social barriers in place (e.g., racism, patriarchy, wealth exclusion). Attempts to include can also be exclusionary. When we invite people to participate it is never everyone: some people are always excluded. Typically those excluded are the very worst-off, those with low literacy, those who do not have the time to seek out participatory opportunities, are not members of the right networks, etc. At other times exclusion is needed for safe, open participatory action. And the purposeful abstention, collective refusal, dissent, or silent protest are themselves forms of participation (e.g., as illustrated by the Maori data rights case study).
Cooptation. Concerns remain of participation becoming a mechanism of cooptation. Specific concerns are raised through current economic and capitalist models that seek to dissolve social relations and behaviours into commodities that are then open to monetization. The history of colonial tactics showed how traditional participatory structures were co-opted to claim legitimacy. The case study on machine translation for African languages raises related concerns, where the efforts of grassroots participatory actions, and their data-sharing initiatives, leaves opens the door for cooptation, where corporate actors can use the results of participatory efforts towards corporate benefits. The potential for corporate actors to capitalize on such efforts and build products that maximize profits, with little benefit to communities remains open. In such circumstances, not only are those that participated disempowered, but corporations then emerge as the legitimate arbiters of African languages and subsequently language technology.
Effectiveness and Measurement. One core concern with participatory methods is that it is difficult to measure and provide attribution to the positive benefits of participation. The type of participation described in Appendix B are likely to result in benefits (though real) that are gradual and intangible, e.g., a sense of empowerment, knowledge transfer, creation of social capital, and social reform. In particular, participation that enables in-depth understanding and iterative co-learning can defy quantification and measurement. Investing in such types of participation may appear wasteful when outcomes are measured using blunt instruments such as cost-benefit analysis, and it could instead be the limitations of the metrics we use to evaluate participatory approaches that are an obstacle to the effective use of participatory approaches. The problem of measurement of impact and a general monitoring, evaluation and learning (MEL) framework is generally difficult, so also points to areas for further research to effectively make participatory methods part of regular practice.
Expertise and Incentives. One aim of participatory methods is to spread knowledge about technical systems and their impacts. This involves the knowledge of technical experts, but also the local knowledge embedded in the lived-experience of communities. There is an epistemic burden on all stakeholders in the participatory process to gather enough information to reason, questions, act or decide. The need then to always learn and gather information requires participatory approaches that occur at different time frames, over various duration, and with different groups. Participation necessitates an assessment of the incentives involved, which can become distorted by epistemic burden, fundamentally affecting the participatory process. Put simply, participatory methods cannot rely on simplified assumptions about the reasons people have for engaging in a participatory process. This returns to the need to challenge uneven power distributions and oppressive social structures, as well as the ways that `community’ itself can hide power dynamics.
Conclusion
To characterise AI as participatory is to acknowledge that the communities and publics beyond technical designers haveknowledge, expertise and interests that are essential to the development of AI that aims to strengthen justice and prosperity. Participation is then an essential component in achieving these aims, yet hype and misunderstanding of the participation’s role risks reducing its effectiveness and the possibility of greater harm and exploitation of participants. This paper contributes towards clarifying the understanding and role of participation in AI, situating participation within its historical development, as central to contending with systems of power, as seeking forms of vibrant participation, and a as set of methods that has limitations and specific uses.
Participation in AI is a broad set of practices, but whether we use participation for algorithmic improvement, methodological innovation, or collective exploration, they can be characterised along axes of empowerment and reflexive assessment, along which we seek to move away from transactional engagements towards forms of vibrant participation that are in constant engagement with their publics and increase community knowledge and empowerment. As the case studies show, there are desirable forms of participation that are already available that we can draw inspiration from. Participation takes many different from across the AI pipeline, but for researchers, a key aim is to build the reflexive process that the probe questions hoped to initiate. New AI research and products will increasingly rely onparticipation for its claims to safety, legitimacy and responsibility and we hope this paper provides part of the clarity needed to effectively incorporate participatory methods; and where we find participation lacking, we hope to provide a basis for critique, which is itself a powerful form of participation.
We would like to thank Nenad Tomašev, Sean Legassick, and Courtney Biles for the invaluable comments on an earlier version of the paper. We would also like thank the EAAMO reviewers for their feedback.
Appendix
Reflexive Assessment of Participatory Practices
As outlined in earlier subsections, participatory efforts in AI may draw from different motivations, may have different objectives, and are characterized by different attributes that influence their effectiveness and utility for the communities they are meant to help. What makes participation meaningful may vary depending on its sociotechnical contexts, but we argue that it is critical nonetheless to maintain a deep reflexive exercise on the various objectives and characteristics of a planned participatory effort. This is particularly important when participation is called for by researchers, technologists and institutions in positions of power.
Below, we provide a set of questions to clarify and make explicit the goals, objectives, and limitations of any particular participatory activity. These questions are not meant to serve as an exhaustive checklist, rather as a tool to help guide researchers and technologists towards a reflexive exercise. We include questions here that are relevant across most, if not all, contexts of AI interventions, however specific contexts might call for the inclusion of additional questions.
Do the project the goals that motivate a participatory effort seek to support community interests?
What efforts will go into building trust with the people and communities that are involved in participatory initiatives?
When there is lack of trust, how is it understood in its historical and structural context (e.g., effects of racism and discrimination)?
What efforts will be taken to manage and mitigate the effects of the power imbalance between the participants and the project team?
What efforts will be taken to manage and mitigate the effects of the power differential within the participant group(s)?
What efforts will be taken to ensure a transparent and open conversation between the participants and the project team?
What mechanism are put in place to allow participants to question the existence of the product/tool itself, rather than helping to reduce harms or improve benefits?
In what ways will the participation process allow for disagreement?
How do participants experience the process of participation?
What do participants own and how do they benefit?
In what ways is the participatory process more than a collection of individual experiences?
Will the participatory effort be a one-off engagement with the community, or a recurring/long-term engagement?
At what step or phase of the development process will participation with communities take place?
Did participants have the opportunity to refuse participation or withdraw from the process without causing direct or indirect harm to themselves or their communities?
Will there be flexibility in the participatory process to influence decisions made in prior phases?
Could the data source or curation decisions be changed, and data be re-collected based on insights from your participatory efforts?
Could the plans for evaluation of performance or harms be updated based on insights from your participatory efforts?
Current Modes of Participation in AI
There is no single unified understanding of the term or vision of what participation is supposed to achieve. Rather, participation is a concept that encompasses a cluster of attitudes, practices, and goals, ranging from focusing on improving model performance, accuracy and data quality to building social reforms and redistribution of power and resources. A single universal definition and vision vision of what participatory AI could could become is therefore futile. In this section, we offer a characterization of participation in order to clearly articulate the implications and consequences of different forms of participation in ML projects. We present three broad categories of participation, not with a sharp delineation and boundaries, but as overlapping categories morphing into each other. Our characterization investigates participation in terms of its driving motivations and objectives.
Participation for algorithmic performance improvement
Participation of this type aims to leverage participation as a means to improve the quality, robustness, or diversity of one or more technical components of an existing system such as a dataset or model. This might include practices such as community data labeling and annotation, or collective data entry and cleaning. These kinds of practices tend to have clear and pre-defined roles, often set out by the researcher or tech developer for the “participant”, “modeller” and other stakeholders. Tasks for participants tend to be discreet microtasks or one-off involvements, although they may also involve longer term engagements.
Pre-defined and relatively rigid roles for participants enable certain kinds of objectives, but also foreclose others. These types of practices are relatively unambiguous and can have benefits such as improvement of AI model accuracy, efficiency, and robustness via improving data quality. They can also elevate the inclusion and better representation of subjects and communities (in datasets) that are historically marginalized in technology development. For instance, data annotation done by communities that are subject matter experts of certain topics can contribute to a net improvement of AI models and potentially be a net benefit to these communities themselves. But, data from marginalized communities also has the potential to be be misunderstood and misinterpreted., for example, hired formerly gang-involved youths as domain experts that are best suited to contextualize Twitter data from same domain in order to create a more inclusive and community-informed ML systems.
Because the impact of participants is tightly scoped to particular components of a system or system behavior, overall community benefit is not guaranteed. Furthermore, the participants are often not provided with the overall picture of the larger system. Communication between developers, designers, and participants, tends to be limited and one-way, in that the participant has little room for negotiation or access to the larger goal or the downstream impact of their “participation”. Participants may not even be aware of how their individual actions impact the overall outcomes of the system. From this perspective, participation is piecemeal and potentially extractive in the sense that it may not result in a net benefit to participants, or lead to technology that benefits those at the margins of society.
Participation for process improvement
In other uses, participation is prioritized as a methodology that provides unique insights that can inform the overall design process (not as a means to achieve a particular pre-determined technical objective). Examples of this type include inclusive design, user-centered design, co-design, participatory design, human-in-the-loop model development, citizen science, and crowdsourcing. This form of participation can vary from a discreet one-off involvement to a short term recurring participation. Participation takes place in the form of activities like user feedback, surveys, focus groups, and user experience (UX) studies (see). This type of participation can be found in academic research settings, non-profit and activist organization (e.g., the participatory initiatives from the Turing Institute [https://decidim.org/ andhttps://www.turing.ac.uk/research/research-projects/citizen-science-platform-autistica] and the UN [https://www.unodc.org/unodc/en/alternative-development/particpatoryapproaches.html] for example), as well as in industry product development settings [https://pair-code.github.io/datacardsplaybook/].
This form of participation also facilitates certain types of opportunities, but also forecloses others. When done in a service or product-design setting, such participation is a means to include the beliefs, attitudes, and needs of customers who may have been marginalized or for whom the product is not working in their best interest. There are circumstances in which this form can benefit both the company (making profit on a better product) and the individuals and communities involved (e.g., see initiatives such as Racial Equity in Everyday Products [https://design.google/library/racial-equity-everyday-products/] and How anyone can make Maps more accessible [https://africa.googleblog.com/2021/05/how-anyone-can-make-maps-more-accessible.html]). While there is some flexibility to discuss, iterate and change the overall goals of a project or product, those who design and create tools often set the agenda and make major decisions. In general, this form lends itself to some level of active participation on how a project, product, or service is designed with an underlying aim of mutual benefit for the participant and those seeking participants. Still, this form of participation neither allows questions such as whether the design or service should exist at all nor goes so far as to challenge power asymmetries and oppressive structures that are at play. Participation for process improvement is more reciprocal (than participation for algorithmic improvement), but the boundaries of the project remain relatively fixed by the designers and bigger organizational goals and participation is expected to happen within those bounds without opportunity to question or change those bounds.
Participation for collective exploration
Participation of this type aims to organize a project (a practice or an activity) around the needs, goals, and futures of a particular community. Participants self-organize or are included as representatives of a community to which they belong, and the activities that constitute the means of participation are designed to generate an understanding of the views, needs, and perspectives of the group. Core concerns of communities shape the central agenda, product, or service and subsequently how participation is collectively organized or how participants are selected and engaged. This type of participation tackles question such as who participates, why, how, who benefits, what are the larger objectives of participation, as well as the downstream implications for a product or service (seefor example).
In this form of participation, creating, planning, decision making and building are an ongoing and emergent processes. They unfold over time, in sometimes unanticipated and unpredictable ways, through continual discussion among participants, facilitators and various stakeholders. In its extreme form, this form of participation can be mainly exploratory and a medium that enables in-depth understanding of each other and the previously unfamiliar, where people engage in active and iterative co-learning. Here, participation is not merely a means to a goal; participation is a main goal in and of itself. Participation in this form is seen as not merely a way to problem solve but rather as a rewarding and valuable activity and a process in and of itself (but also one that may result in a product or service as a by product). This form of participation is rarely found within AI. The core motivation and objectives of this form of participation are somewhat incompatible with the core values of AI research, which include efficiency, performance, accuracy, novelty, and beating state-of-the-art.
Participation for collective exploration can lead to the attainment of the goals set out by other forms of participation, but also has significant implications for how a project is structured and what a project may or may not ultimately achieve. Participation as collective exploration requires prioritizing the expertise that is gained through local knowledge and lived experience. While this approach can accommodate both methodological innovation and fine grained objectives such as model/data improvement, such outcomes would be by-products of processes, not an originating and orienting focus. This form necessarily takes time, and may come in conflict with rapid research and design expectations.
Decisions at every stage of the process — from boundary definition, co-deliberation, co-conceptualization of problems, to design and development of tools/products/prototypes to data collection and annotation and everything in between — are made through active involvement of participants. The case study `Fighting Fighting Māori data rights’ provides an example of a form of activity that is participatory throughout. As this form of participation is driven by the needs, goals, and futures of a particular community, the need for a project, product or tool can be questioned and/or entirely discarded if it conflicts such community goals and needs. This form of participation asks not only who is/ought to participate and at what level, but also participate to what ends? Questions such as “Is participation to enable better surveillance? To improve harmful systems? To create an anti-racist model?" form a core part of it. This form of participation, because no question is off the table, accommodates, and is sympathetic to, challenging uneven power dynamics and hierarchical and oppressive social structures. This form of participation is slow paced, unfolds over a long period of time, and physical space and interaction are important. It requires a significant investment in resources, money and time while the outcome may not be measurable in monetary terms (compared to participation for algorithmic performance improvement, for instance, where results are immediate).
Bibliography
1@article{asaro2000transforming,
2 publisher = {Elsevier},
3 year = {2000},
4 pages = {257--290},
5 number = {4},
6 volume = {10},
7 journal = {Accounting, Management and Information Technologies},
8 author = {Asaro, Peter M},
9 title = {Transforming society by transforming technology: the science and politics of participatory design},
10}
11
12@book{chilvers2015remaking,
13 publisher = {Routledge},
14 year = {2015},
15 author = {Chilvers, Jason and Kearnes, Matthew},
16 title = {Remaking participation: Science, environment and emergent publics},
17}
18
19@book{barney2016participatory,
20 publisher = {U of Minnesota Press},
21 year = {2016},
22 volume = {51},
23 author = {Barney, Darin and Coleman, Gabriella and Ross, Christine and Sterne, Jonathan and Tembeck, Tamar},
24 title = {The participatory condition in the digital age},
25}
26
27@article{martin2020participatory,
28 year = {2020},
29 journal = {arXiv preprint arXiv:2005.07572},
30 author = {Martin Jr, Donald and Prabhakaran, Vinodkumar and Kuhlberg, Jill and Smart, Andrew and Isaac, William S},
31 title = {Participatory problem formulation for fairer machine learning through community based system dynamics},
32}
33
34@book{greenbaum1991design,
35 publisher = {CRC Press},
36 year = {1991},
37 author = {Greenbaum, Joan and Kyng, Morten},
38 title = {Design at work: Cooperative design of computer systems},
39}
40
41@book{lugard1922dual,
42 publisher = {Routledge},
43 year = {1922},
44 author = {Lugard, Lord Frederick JD},
45 title = {The dual mandate in British tropical Africa},
46}
47
48@article{nekoto2020participatory,
49 year = {2020},
50 journal = {arXiv preprint arXiv:2010.02353},
51 author = {Nekoto, Wilhelmina and Marivate, Vukosi and Matsila, Tshinondiwa and Fasubaa, Timi and Kolawole, Tajudeen and Fagbohungbe, Taiwo and Akinola, Solomon Oluwole and Muhammad, Shamsuddeen Hassan and Kabongo, Salomon and Osei, Salomey and others},
52 title = {Participatory research for low-resourced machine translation: A case study in african languages},
53}
54
55@inproceedings{katellToward2020,
56 series = {FAT* '20},
57 location = {Barcelona, Spain},
58 numpages = {11},
59 pages = {45–55},
60 booktitle = {Proceedings of the 2020 Conference on Fairness, Accountability, and Transparency},
61 address = {New York, NY, USA},
62 publisher = {Association for Computing Machinery},
63 isbn = {9781450369367},
64 year = {2020},
65 title = {Toward Situated Interventions for Algorithmic Equity: Lessons from the Field},
66 author = {Katell, Michael and Young, Meg and Dailey, Dharma and Herman, Bernease and Guetler, Vivian and Tam, Aaron and Bintz, Corinne and Raz, Daniella and Krafft, P. M.},
67}
68
69@inproceedings{abebe2021narratives,
70 year = {2021},
71 pages = {329--341},
72 booktitle = {Proceedings of the 2021 ACM Conference on Fairness, Accountability, and Transparency},
73 author = {Abebe, Rediet and Aruleba, Kehinde and Birhane, Abeba and Kingsley, Sara and Obaido, George and Remy, Sekou L and Sadagopan, Swathi},
74 title = {Narratives and counternarratives on data sharing in Africa},
75}
76
77@article{sloane2020participation,
78 year = {2020},
79 journal = {arXiv preprint arXiv:2007.02423},
80 author = {Sloane, Mona and Moss, Emanuel and Awomolo, Olaitan and Forlano, Laura},
81 title = {Participation is not a design fix for machine learning},
82}
83
84@article{balaram2018artificial,
85 year = {2018},
86 journal = {London: RSA. https://www. thersa. org/discover/publications-and-articles/reports/artificial-intelligence-realpublic-engagement},
87 author = {Balaram, Brhmie and Greenham, Tony and Leonard, Jasmine},
88 title = {Artificial Intelligence: real public engagement},
89}
90
91@article{ehn1987collective,
92 publisher = {Aldershot, Avebury},
93 year = {1987},
94 pages = {17--57},
95 journal = {Computers and democracy},
96 author = {Ehn, Pelle and Kyng, Morten},
97 title = {The collective resource approach to systems design},
98}
99
100@inproceedings{sambasivan2021everyone,
101 year = {2021},
102 pages = {1--15},
103 booktitle = {proceedings of the 2021 CHI Conference on Human Factors in Computing Systems},
104 author = {Sambasivan, Nithya and Kapania, Shivani and Highfill, Hannah and Akrong, Diana and Paritosh, Praveen and Aroyo, Lora M},
105 title = {“Everyone wants to do the model work, not the data work”: Data Cascades in High-Stakes AI},
106}
107
108@article{frey2020artificial,
109 publisher = {SAGE Publications Sage CA: Los Angeles, CA},
110 year = {2020},
111 pages = {42--56},
112 number = {1},
113 volume = {38},
114 journal = {Social Science Computer Review},
115 author = {Frey, William R and Patton, Desmond U and Gaskell, Michael B and McGregor, Kyle A},
116 title = {Artificial intelligence and inclusion: Formerly gang-involved youth as domain experts for analyzing unstructured twitter data},
117}
118
119@article{baum2006participatory,
120 publisher = {BMJ Publishing Group},
121 year = {2006},
122 pages = {854},
123 number = {10},
124 volume = {60},
125 journal = {Journal of epidemiology and community health},
126 author = {Baum, Fran and MacDougall, Colin and Smith, Danielle},
127 title = {Participatory action research},
128}
129
130@article{lee2019webuildai,
131 publisher = {ACM New York, NY, USA},
132 year = {2019},
133 pages = {1--35},
134 number = {CSCW},
135 volume = {3},
136 journal = {Proceedings of the ACM on Human-Computer Interaction},
137 author = {Lee, Min Kyung and Kusbit, Daniel and Kahng, Anson and Kim, Ji Tae and Yuan, Xinran and Chan, Allissa and See, Daniel and Noothigattu, Ritesh and Lee, Siheon and Psomas, Alexandros and others},
138 title = {WeBuildAI: Participatory framework for algorithmic governance},
139}
140
141@article{feyisa2020characterizing,
142 publisher = {Elsevier},
143 year = {2020},
144 pages = {105595},
145 volume = {175},
146 journal = {Computers and Electronics in Agriculture},
147 author = {Feyisa, Gudina Legese and Palao, Leo Kris and Nelson, Andy and Gumma, Murali Krishna and Paliwal, Ambica and Win, Khin Thawda and Nge, Khin Htar and Johnson, David E},
148 title = {Characterizing and mapping cropping patterns in a complex agro-ecosystem: An iterative participatory mapping procedure using machine learning algorithms and MODIS vegetation indices},
149}
150
151@inproceedings{jo2020lessons,
152 year = {2020},
153 pages = {306--316},
154 booktitle = {Proceedings of the 2020 Conference on Fairness, Accountability, and Transparency},
155 author = {Jo, Eun Seo and Gebru, Timnit},
156 title = {Lessons from archives: Strategies for collecting sociocultural data in machine learning},
157}
158
159@inproceedings{ishida2007augmented,
160 year = {2007},
161 pages = {1341--1346},
162 volume = {7},
163 booktitle = {IJCAI},
164 author = {Ishida, Toru and Nakajima, Yuu and Murakami, Yohei and Nakanishi, Hideyuki},
165 title = {Augmented Experiment: Participatory Design with Multiagent Simulation.},
166}
167
168@misc{groves2017remaking,
169 publisher = {Taylor \& Francis},
170 year = {2017},
171 author = {Groves, Christopher},
172 title = {Remaking participation: Science, environment and emergent publics},
173}
174
175@article{dearden2008participatory,
176 year = {2008},
177 author = {Dearden, Andy and Rizvi, Haider},
178 title = {Participatory design and participatory development: a comparative review},
179}
180
181@article{chambers1994origins,
182 publisher = {Elsevier},
183 year = {1994},
184 pages = {953--969},
185 number = {7},
186 volume = {22},
187 journal = {World development},
188 author = {Chambers, Robert},
189 title = {The origins and practice of participatory rural appraisal},
190}
191
192@book{ferguson1994anti,
193 publisher = {U of Minnesota Press},
194 year = {1994},
195 author = {Ferguson, James},
196 title = {The anti-politics machine:" development," depoliticization, and bureaucratic power in Lesotho},
197}
198
199@book{mitchell2002rule,
200 publisher = {University of California Press},
201 year = {2002},
202 author = {Mitchell, Timothy},
203 title = {Rule of experts},
204}
205
206@book{sen2009idea,
207 publisher = {Harvard University Press},
208 year = {2009},
209 author = {Sen, Amartya Kumar},
210 title = {The idea of justice},
211}
212
213@article{farmer2001community,
214 publisher = {World Health Organization},
215 year = {2001},
216 pages = {1145},
217 number = {12},
218 volume = {79},
219 journal = {Bulletin of the World Health Organization},
220 author = {Farmer, Paul and L{\'e}andre, Fernet and Mukherjee, Joia and Gupta, Rajesh and Tarter, Laura and Kim, Jim Yong},
221 title = {Community-based treatment of advanced HIV disease: introducing DOT-HAART (directly observed therapy with highly active antiretroviral therapy).},
222}
223
224@article{kraft1994collective,
225 year = {1994},
226 pages = {4},
227 number = {1},
228 volume = {6},
229 journal = {Scandinavian Journal of Information Systems},
230 author = {Kraft, Philip and Bansler, J{\o}rgen P},
231 title = {The collective resource approach: the Scandinavian experience},
232}
233
234@book{zuboff2019age,
235 publisher = {Profile books},
236 year = {2019},
237 author = {Zuboff, Shoshana},
238 title = {The age of surveillance capitalism: The fight for a human future at the new frontier of power},
239}
240
241@book{veliz2020privacy,
242 publisher = {Random House Australia},
243 year = {2020},
244 author = {V{\'e}liz, Carissa},
245 title = {Privacy is power},
246}
247
248@article{van2021trading,
249 publisher = {Oxford University Press},
250 year = {2021},
251 pages = {2128--2138},
252 number = {10},
253 volume = {28},
254 journal = {Journal of the American Medical Informatics Association},
255 author = {van der Veer, Sabine N and Riste, Lisa and Cheraghi-Sohi, Sudeh and Phipps, Denham L and Tully, Mary P and Bozentko, Kyle and Atwood, Sarah and Hubbard, Alex and Wiper, Carl and Oswald, Malcolm and others},
256 title = {Trading off accuracy and explainability in AI decision-making: findings from 2 citizens’ juries},
257}
258
259@article{frey2019place,
260 publisher = {ACM New York, NY, USA},
261 year = {2019},
262 pages = {1--31},
263 number = {CSCW},
264 volume = {3},
265 journal = {Proceedings of the ACM on Human-Computer Interaction},
266 author = {Frey, Seth and Krafft, PM and Keegan, Brian C},
267 title = {" This Place Does What It Was Built For" Designing Digital Institutions for Participatory Change},
268}
269
270@article{birhane2021values,
271 year = {2021},
272 journal = {arXiv preprint arXiv:2106.15590},
273 author = {Birhane, Abeba and Kalluri, Pratyusha and Card, Dallas and Agnew, William and Dotan, Ravit and Bao, Michelle},
274 title = {The values encoded in machine learning research},
275}
276
277@article{arnstein1969ladder,
278 publisher = {Taylor \& Francis},
279 year = {1969},
280 pages = {216--224},
281 number = {4},
282 volume = {35},
283 journal = {Journal of the American Institute of planners},
284 author = {Arnstein, Sherry R},
285 title = {A ladder of citizen participation},
286}
287
288@book{kelty2020participant,
289 publisher = {University of Chicago Press},
290 year = {2020},
291 author = {Kelty, Christopher M},
292 title = {The participant: A century of participation in four stories},
293}
294
295@article{freire1996pedagogy,
296 year = {1996},
297 journal = {New York: Continuum},
298 author = {Freire, Paolo},
299 title = {Pedagogy of the oppressed (revised)},
300}
301
302@article{moynihan1969maximum,
303 publisher = {ERIC},
304 year = {1969},
305 author = {Moynihan, Daniel P},
306 title = {Maximum Feasible Misunderstanding; Community Action in the War on Poverty.},
307}
308
309@article{cabannes2004participatory,
310 publisher = {Sage Publications Sage CA: Thousand Oaks, CA},
311 year = {2004},
312 pages = {27--46},
313 number = {1},
314 volume = {16},
315 journal = {Environment and urbanization},
316 author = {Cabannes, Yves},
317 title = {Participatory budgeting: a significant contribution to participatory democracy},
318}
319
320@inproceedings{kelty2017toolkit,
321 year = {2017},
322 publisher = {Limn},
323 volume = {9},
324 booktitle = {Little Development Devices / Humanitarian Goods},
325 author = {Kelty, Christopher M},
326 title = {The Participatory Development Toolkit},
327}
328
329@article{bondi2021envisioning,
330 year = {2021},
331 journal = {arXiv preprint arXiv:2105.01774},
332 author = {Bondi, Elizabeth and Xu, Lily and Acosta-Navas, Diana and Killian, Jackson A},
333 title = {Envisioning Communities: A Participatory Approach Towards AI for Social Good},
334}
335
336@article{berditchevskaiaparticipatory,
337 year = {2020},
338 author = {Berditchevskaia, Aleks and Malliaraki, Eirini and Peach, Kathy},
339 title = {Participatory AI for humanitarian innovation},
340}
341
342@article{chan2021limits,
343 year = {2021},
344 journal = {arXiv preprint arXiv:2102.01265},
345 author = {Chan, Alan and Okolo, Chinasa T and Terner, Zachary and Wang, Angelina},
346 title = {The Limits of Global Inclusion in AI Development},
347}
348
349@article{donia2021co,
350 publisher = {SAGE Publications Sage UK: London, England},
351 year = {2021},
352 pages = {20539517211065248},
353 number = {2},
354 volume = {8},
355 journal = {Big Data \& Society},
356 author = {Donia, Joseph and Shaw, James A},
357 title = {Co-design and ethical artificial intelligence for health: An agenda for critical research and practice},
358}
359
360@article{quick2011distinguishing,
361 publisher = {SAGE Publications Sage CA: Los Angeles, CA},
362 year = {2011},
363 pages = {272--290},
364 number = {3},
365 volume = {31},
366 journal = {Journal of planning education and research},
367 author = {Quick, Kathryn S and Feldman, Martha S},
368 title = {Distinguishing participation and inclusion},
369}
370
371@article{spivak2003can,
372 year = {2003},
373 pages = {42--58},
374 number = {27},
375 volume = {14},
376 journal = {Die Philosophin},
377 author = {Spivak, Gayatri Chakravorty},
378 title = {Can the subaltern speak?},
379}
380
381@book{couldry2019costs,
382 publisher = {Stanford University Press},
383 year = {2019},
384 author = {Couldry, Nick and Mejias, Ulises A},
385 title = {The costs of connection},
386}
387
388@article{leeparticipatory,
389 author = {Lee, Min Kyung and Nigam, Ishan and Zhang, Angie and Afriyie, Joel and Qin, Zhizhen and Gao, Sicun},
390 title = {Participatory Algorithmic Management for Worker Well-Being},
391}
392
393@book{costanza2020design,
394 year = {2020},
395 author = {Costanza-Chock, Sasha},
396 title = {Design justice: Community-led practices to build the worlds we need},
397}
398
399@inproceedings{waycott2016not,
400 year = {2016},
401 pages = {745--757},
402 booktitle = {Proceedings of the 2016 CHI Conference on Human Factors in Computing Systems},
403 author = {Waycott, Jenny and Vetere, Frank and Pedell, Sonja and Morgans, Amee and Ozanne, Elizabeth and Kulik, Lars},
404 title = {Not for me: Older adults choosing not to participate in a social isolation intervention},
405}
406
407@inproceedings{irani2013turkopticon,
408 year = {2013},
409 pages = {611--620},
410 booktitle = {Proceedings of the SIGCHI conference on human factors in computing systems},
411 author = {Irani, Lilly C and Silberman, M Six},
412 title = {Turkopticon: Interrupting worker invisibility in amazon mechanical turk},
413}
414
415@inproceedings{pierre2021getting,
416 year = {2021},
417 pages = {1--11},
418 booktitle = {Proceedings of the 2021 CHI Conference on Human Factors in Computing Systems},
419 author = {Pierre, Jennifer and Crooks, Roderic and Currie, Morgan and Paris, Britt and Pasquetto, Irene},
420 title = {Getting Ourselves Together: Data-centered participatory design research \& epistemic burden},
421}
422
423@book{toyama2015geek,
424 publisher = {PublicAffairs},
425 year = {2015},
426 author = {Toyama, Kentaro},
427 title = {Geek heresy: Rescuing social change from the cult of technology},
428}
429
430@article{gabriel2022toward,
431 publisher = {MIT Press One Rogers Street, Cambridge, MA 02142-1209, USA journals-info~…},
432 year = {2022},
433 pages = {218--231},
434 number = {2},
435 volume = {151},
436 journal = {Daedalus},
437 author = {Gabriel, Iason},
438 title = {Toward a Theory of Justice for Artificial Intelligence},
439}
440
441@article{scheall2021priority,
442 publisher = {Cambridge University Press},
443 year = {2021},
444 pages = {726--737},
445 number = {4},
446 volume = {18},
447 journal = {Episteme},
448 author = {Scheall, Scott and Crutchfield, Parker},
449 title = {The priority of the epistemic},
450}
451
452@incollection{muller2012participatory,
453 publisher = {CRC Press},
454 year = {2012},
455 pages = {1125--1153},
456 booktitle = {The Human--Computer Interaction Handbook},
457 author = {Muller, Michael J and Druin, Allison},
458 title = {Participatory design: The third space in human--computer interaction},
459}
460
461@article{van2015participatory,
462 year = {2015},
463 pages = {41--66},
464 journal = {Handbook of Ethics, Values, and Technological Design: Sources, Theory, Values and Application Domains},
465 author = {Van der Velden, Maja and M{\"o}rtberg, Christina and others},
466 title = {Participatory design and design for values},
467}
468
469@article{harrington2020forgotten,
470 publisher = {ACM New York, NY, USA},
471 year = {2020},
472 pages = {24--29},
473 number = {3},
474 volume = {27},
475 journal = {Interactions},
476 author = {Harrington, Christina N},
477 title = {The forgotten margins: what is community-based participatory health design telling us?},
478}
479
480@article{arana2021citizen,
481 year = {2021},
482 journal = {arXiv preprint arXiv:2103.00508},
483 author = {Arana-Catania, Miguel and Van Lier, Felix-Anselm and Procter, Rob and Tkachenko, Nataliya and He, Yulan and Zubiaga, Arkaitz and Liakata, Maria},
484 title = {Citizen participation and machine learning for a better democracy},
485}
486
487@article{weber2015participatory,
488 publisher = {Internet Society},
489 year = {2015},
490 volume = {15},
491 journal = {Proc. USEC},
492 author = {Weber, Susanne and Harbach, Marian and Smith, Matthew},
493 title = {Participatory design for security-related user interfaces},
494}
495
496@article{majale2008employment,
497 publisher = {Elsevier},
498 year = {2008},
499 pages = {270--282},
500 number = {2},
501 volume = {32},
502 journal = {Habitat International},
503 author = {Majale, Michael},
504 title = {Employment creation through participatory urban planning and slum upgrading: The case of Kitale, Kenya},
505}
506
507@misc{maori21,
508 year = {2021},
509 journal = {Wired},
510 author = {Graham, Tim},
511 url = {https://www.wired.co.uk/article/maori-language-tech},
512 title = {Māori are trying to save their language from Big Tech},
513}
514
515@inproceedings{abernethy2018activeremediation,
516 year = {2018},
517 pages = {5--14},
518 booktitle = {Proceedings of the 24th ACM SIGKDD International Conference on Knowledge Discovery \& Data Mining},
519 author = {Abernethy, Jacob and Chojnacki, Alex and Farahi, Arya and Schwartz, Eric and Webb, Jared},
520 title = {Activeremediation: The search for lead pipes in flint, michigan},
521}
522
523@misc{madrigal2019,
524 year = {2019},
525 journal = {The Atlantic},
526 author = {Madrigal, Alexis C.},
527 url = {https://www.theatlantic.com/technology/archive/2019/01/how-machine-learning-found-flints-lead-pipes/578692/},
528 title = {How a Feel-Good AI Story Went Wrong in Flint},
529}
530
531@book{cooke2001participation,
532 publisher = {Zed books},
533 year = {2001},
534 author = {Cooke, Bill and Kothari, Uma},
535 title = {Participation: The new tyranny?},
536}
537
538@article{mackenzie2012value,
539 publisher = {Elsevier},
540 year = {2012},
541 pages = {11--21},
542 volume = {474},
543 journal = {Journal of hydrology},
544 author = {Mackenzie, John and Tan, Poh-Ling and Hoverman, Suzanne and Baldwin, Claudia},
545 title = {The value and limitations of participatory action research methodology},
546}
547
548@article{mansuri2012localizing,
549 publisher = {World Bank Publications},
550 year = {2012},
551 author = {Mansuri, Ghazala and Rao, Vijayendra},
552 title = {Localizing development: Does participation work?},
553}
554
555@misc{ahmed2020,
556 year = {2020},
557 author = {Ahmed, Alex},
558 url = {https://medium.com/care-labor-and-ai/we-will-not-be-pacified-through-participation-aa757ccf79a0},
559 title = {We Will Not Be Pacified Through Participation},
560}
561
562@article{denton2021genealogy,
563 publisher = {SAGE Publications Sage UK: London, England},
564 year = {2021},
565 pages = {20539517211035955},
566 number = {2},
567 volume = {8},
568 journal = {Big Data \& Society},
569 author = {Denton, Emily and Hanna, Alex and Amironesei, Razvan and Smart, Andrew and Nicole, Hilary},
570 title = {On the genealogy of machine learning datasets: A critical history of ImageNet},
571}
572
573@inproceedings{deng2009imagenet,
574 organization = {Ieee},
575 year = {2009},
576 pages = {248--255},
577 booktitle = {2009 IEEE conference on computer vision and pattern recognition},
578 author = {Deng, Jia and Dong, Wei and Socher, Richard and Li, Li-Jia and Li, Kai and Fei-Fei, Li},
579 title = {Imagenet: A large-scale hierarchical image database},
580}
581
582@article{denton2020bringing,
583 year = {2020},
584 journal = {arXiv preprint arXiv:2007.07399},
585 author = {Denton, Emily and Hanna, Alex and Amironesei, Razvan and Smart, Andrew and Nicole, Hilary and Scheuerman, Morgan Klaus},
586 title = {Bringing the people back in: Contesting benchmark machine learning datasets},
587}
588
589@inproceedings{geiger2020garbage,
590 year = {2020},
591 pages = {325--336},
592 booktitle = {Proceedings of the 2020 Conference on Fairness, Accountability, and Transparency},
593 author = {Geiger, R Stuart and Yu, Kevin and Yang, Yanlai and Dai, Mindy and Qiu, Jie and Tang, Rebekah and Huang, Jenny},
594 title = {Garbage in, garbage out? Do machine learning application papers in social computing report where human-labeled training data comes from?},
595}
596
597@book{gray2019ghost,
598 publisher = {Eamon Dolan Books},
599 year = {2019},
600 author = {Gray, Mary L and Suri, Siddharth},
601 title = {Ghost work: How to stop Silicon Valley from building a new global underclass},
602}
603
604@article{mohamed2020decolonial,
605 publisher = {Springer},
606 year = {2020},
607 pages = {659--684},
608 number = {4},
609 volume = {33},
610 journal = {Philosophy \& Technology},
611 author = {Mohamed, Shakir and Png, Marie-Therese and Isaac, William},
612 title = {Decolonial AI: Decolonial theory as sociotechnical foresight in artificial intelligence},
613}
614
615@incollection{roberts2019behind,
616 publisher = {Yale University Press},
617 year = {2019},
618 booktitle = {Behind the Screen},
619 author = {Roberts, Sarah T},
620 title = {Behind the screen},
621}
622
623@article{lecun2015deep,
624 publisher = {Nature Publishing Group},
625 year = {2015},
626 pages = {436--444},
627 number = {7553},
628 volume = {521},
629 journal = {nature},
630 author = {LeCun, Yann and Bengio, Yoshua and Hinton, Geoffrey},
631 title = {Deep learning},
632}
633
634@inproceedings{selbst2019fairness,
635 year = {2019},
636 pages = {59--68},
637 booktitle = {Proceedings of the conference on fairness, accountability, and transparency},
638 author = {Selbst, Andrew D and Boyd, Danah and Friedler, Sorelle A and Venkatasubramanian, Suresh and Vertesi, Janet},
639 title = {Fairness and abstraction in sociotechnical systems},
640}
641
642@inproceedings{mitchell2019model,
643 year = {2019},
644 pages = {220--229},
645 booktitle = {Proceedings of the conference on fairness, accountability, and transparency},
646 author = {Mitchell, Margaret and Wu, Simone and Zaldivar, Andrew and Barnes, Parker and Vasserman, Lucy and Hutchinson, Ben and Spitzer, Elena and Raji, Inioluwa Deborah and Gebru, Timnit},
647 title = {Model cards for model reporting},
648}
649
650@article{gebru2021datasheets,
651 publisher = {ACM New York, NY, USA},
652 year = {2021},
653 pages = {86--92},
654 number = {12},
655 volume = {64},
656 journal = {Communications of the ACM},
657 author = {Gebru, Timnit and Morgenstern, Jamie and Vecchione, Briana and Vaughan, Jennifer Wortman and Wallach, Hanna and Iii, Hal Daum{\'e} and Crawford, Kate},
658 title = {Datasheets for datasets},
659}
660
661@article{holland2018dataset,
662 year = {2018},
663 journal = {arXiv preprint arXiv:1805.03677},
664 author = {Holland, Sarah and Hosny, Ahmed and Newman, Sarah and Joseph, Joshua and Chmielinski, Kasia},
665 title = {The dataset nutrition label: A framework to drive higher data quality standards},
666}
667
668@inproceedings{hutchinson2021towards,
669 year = {2021},
670 pages = {560--575},
671 booktitle = {Proceedings of the 2021 ACM Conference on Fairness, Accountability, and Transparency},
672 author = {Hutchinson, Ben and Smart, Andrew and Hanna, Alex and Denton, Emily and Greer, Christina and Kjartansson, Oddur and Barnes, Parker and Mitchell, Margaret},
673 title = {Towards accountability for machine learning datasets: Practices from software engineering and infrastructure},
674}
675
676@inproceedings{holstein2019improving,
677 year = {2019},
678 pages = {1--16},
679 booktitle = {Proceedings of the 2019 CHI conference on human factors in computing systems},
680 author = {Holstein, Kenneth and Wortman Vaughan, Jennifer and Daum{\'e} III, Hal and Dudik, Miro and Wallach, Hanna},
681 title = {Improving fairness in machine learning systems: What do industry practitioners need?},
682}
683
684@article{halfaker2020ores,
685 publisher = {ACM New York, NY, USA},
686 year = {2020},
687 pages = {1--37},
688 number = {CSCW2},
689 volume = {4},
690 journal = {Proceedings of the ACM on Human-Computer Interaction},
691 author = {Halfaker, Aaron and Geiger, R Stuart},
692 title = {Ores: Lowering barriers with participatory machine learning in wikipedia},
693}
694
695@article{sendak2020real,
696 publisher = {JMIR Publications Inc., Toronto, Canada},
697 year = {2020},
698 pages = {e15182},
699 number = {7},
700 volume = {8},
701 journal = {JMIR medical informatics},
702 author = {Sendak, Mark P and Ratliff, William and Sarro, Dina and Alderton, Elizabeth and Futoma, Joseph and Gao, Michael and Nichols, Marshall and Revoir, Mike and Yashar, Faraz and Miller, Corinne and others},
703 title = {Real-world integration of a sepsis deep learning technology into routine clinical care: implementation study},
704}
705
706@article{mhlambi2020rationality,
707 year = {2020},
708 volume = {9},
709 journal = {Carr Center for Human Rights Policy Discussion Paper Series},
710 author = {Mhlambi, Sabelo},
711 title = {From rationality to relationality: ubuntu as an ethical and human rights framework for artificial intelligence governance},
712}
713
714@article{couldry2021decolonial,
715 publisher = {Taylor \& Francis},
716 year = {2021},
717 pages = {1--17},
718 journal = {Information, Communication \& Society},
719 author = {Couldry, Nick and Mejias, Ulises Ali},
720 title = {The decolonial turn in data and technology research: what is at stake and where is it heading?},
721}
722
723@article{birhane2021algorithmic,
724 publisher = {Elsevier},
725 year = {2021},
726 pages = {100205},
727 number = {2},
728 volume = {2},
729 journal = {Patterns},
730 author = {Birhane, Abeba},
731 title = {Algorithmic injustice: a relational ethics approach},
732}
733
734@inproceedings{green2019good,
735 year = {2019},
736 volume = {17},
737 booktitle = {Proceedings of the AI for Social Good workshop at NeurIPS},
738 author = {Green, Ben},
739 title = {Good” isn’t good enough},
740}
741
742@article{lum2016predict,
743 publisher = {Wiley Online Library},
744 year = {2016},
745 pages = {14--19},
746 number = {5},
747 volume = {13},
748 journal = {Significance},
749 author = {Lum, Kristian and Isaac, William},
750 title = {To predict and serve?},
751}
752
753@article{richardson2019dirty,
754 publisher = {HeinOnline},
755 year = {2019},
756 pages = {15},
757 volume = {94},
758 journal = {NYUL Rev. Online},
759 author = {Richardson, Rashida and Schultz, Jason M and Crawford, Kate},
760 title = {Dirty data, bad predictions: How civil rights violations impact police data, predictive policing systems, and justice},
761}
762
763@article{bennett2018algorithmic,
764 publisher = {Taylor \& Francis},
765 year = {2018},
766 pages = {806--822},
767 number = {7},
768 volume = {28},
769 journal = {Policing and society},
770 author = {Bennett Moses, Lyria and Chan, Janet},
771 title = {Algorithmic prediction in policing: assumptions, evaluation, and accountability},
772}
773
774@article{benjamin2019assessing,
775 publisher = {American Association for the Advancement of Science},
776 year = {2019},
777 pages = {421--422},
778 number = {6464},
779 volume = {366},
780 journal = {Science},
781 author = {Benjamin, Ruha},
782 title = {Assessing risk, automating racism},
783}
784
785@article{banerjee2021reading,
786 year = {2021},
787 journal = {arXiv preprint arXiv:2107.10356},
788 author = {Banerjee, Imon and Bhimireddy, Ananth Reddy and Burns, John L and Celi, Leo Anthony and Chen, Li-Ching and Correa, Ramon and Dullerud, Natalie and Ghassemi, Marzyeh and Huang, Shih-Cheng and Kuo, Po-Chih and others},
789 title = {Reading Race: AI Recognises Patient's Racial Identity In Medical Images},
790}
791
792@inproceedings{raghavan2020mitigating,
793 year = {2020},
794 pages = {469--481},
795 booktitle = {Proceedings of the 2020 conference on fairness, accountability, and transparency},
796 author = {Raghavan, Manish and Barocas, Solon and Kleinberg, Jon and Levy, Karen},
797 title = {Mitigating bias in algorithmic hiring: Evaluating claims and practices},
798}
799
800@article{ajunwa2019auditing,
801 year = {2019},
802 author = {Ajunwa, Ifeoma},
803 title = {An Auditing Imperative for Automated Hiring},
804}
805
806@article{rainie2019indigenous,
807 publisher = {African Minds and the International Development Research Centre (IDRC)},
808 year = {2019},
809 author = {Rainie, Stephanie Carroll and Kukutai, Tahu and Walter, Maggie and Figueroa-Rodr{\'\i}guez, Oscar Luis and Walker, Jennifer and Axelsson, Per},
810 title = {Indigenous data sovereignty},
811}
812
813@article{gabriel2017effective,
814 publisher = {Wiley Online Library},
815 year = {2017},
816 pages = {457--473},
817 number = {4},
818 volume = {34},
819 journal = {Journal of Applied Philosophy},
820 author = {Gabriel, Iason},
821 title = {Effective altruism and its critics},
822}
Attribution
arXiv:2209.07572v1
[cs.CY]
License: cc-by-4.0